Table of Contents
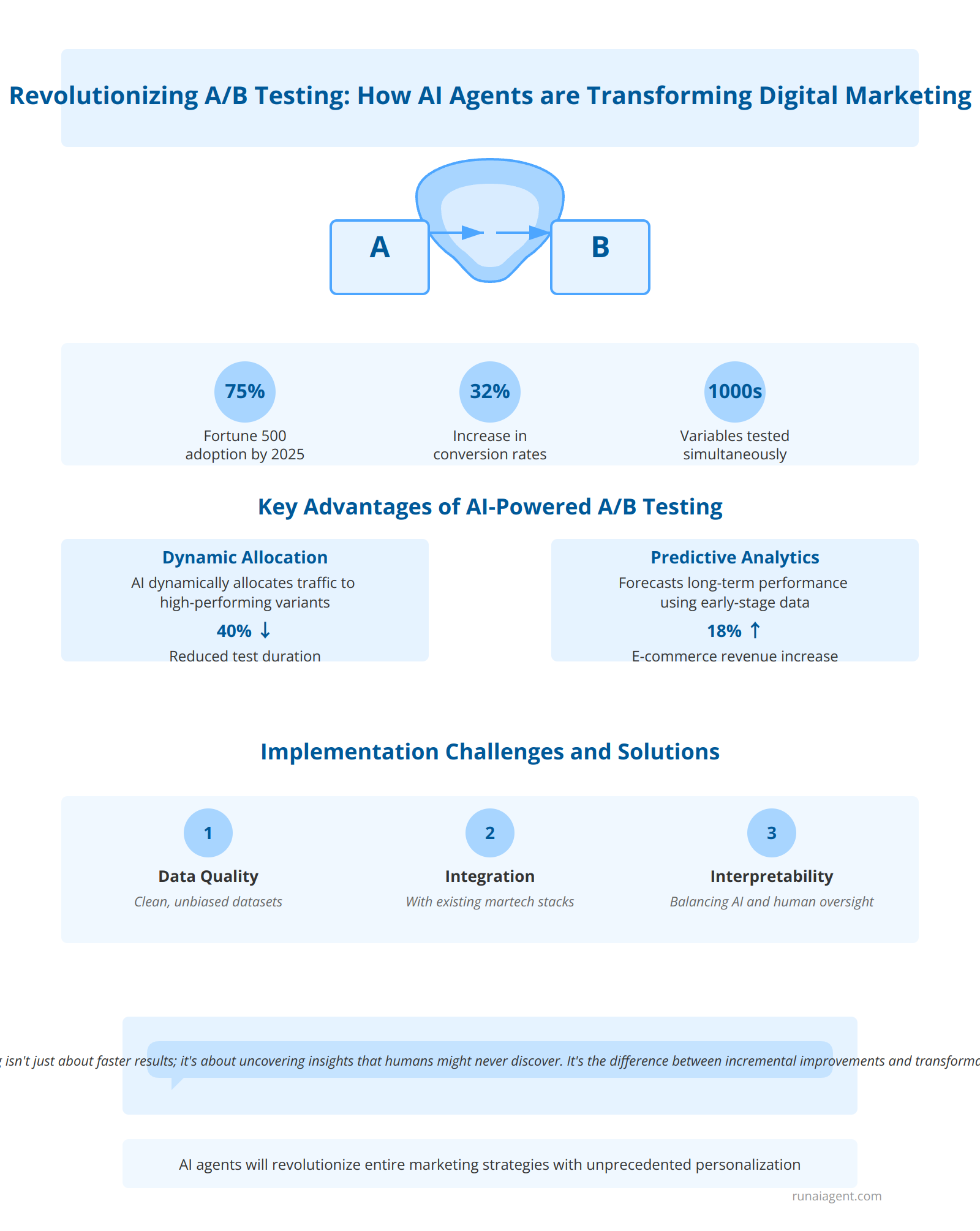
Revolutionizing A/B Testing: How AI Agents are Transforming Digital Marketing
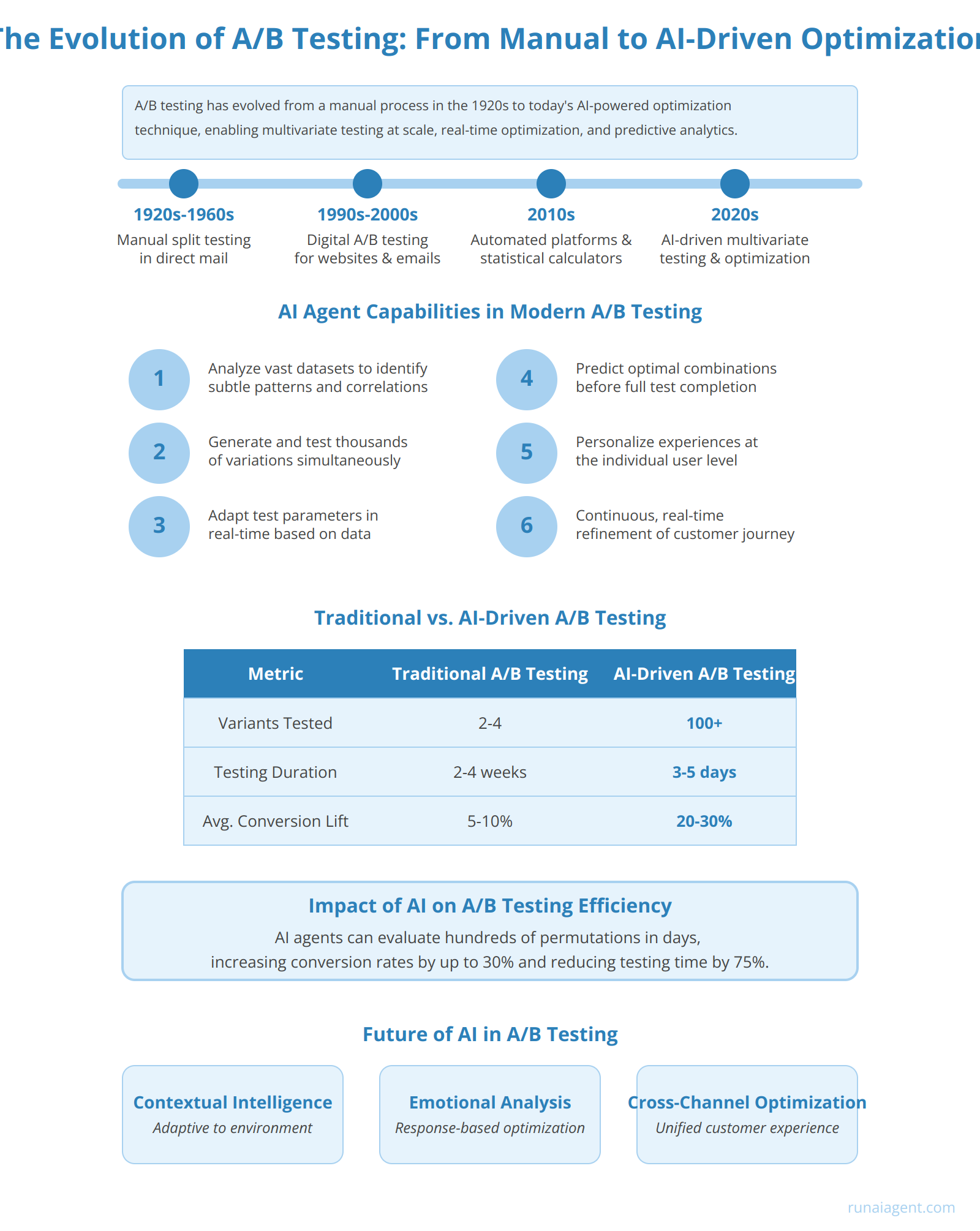
The Evolution of A/B Testing: From Manual to AI-Driven Optimization
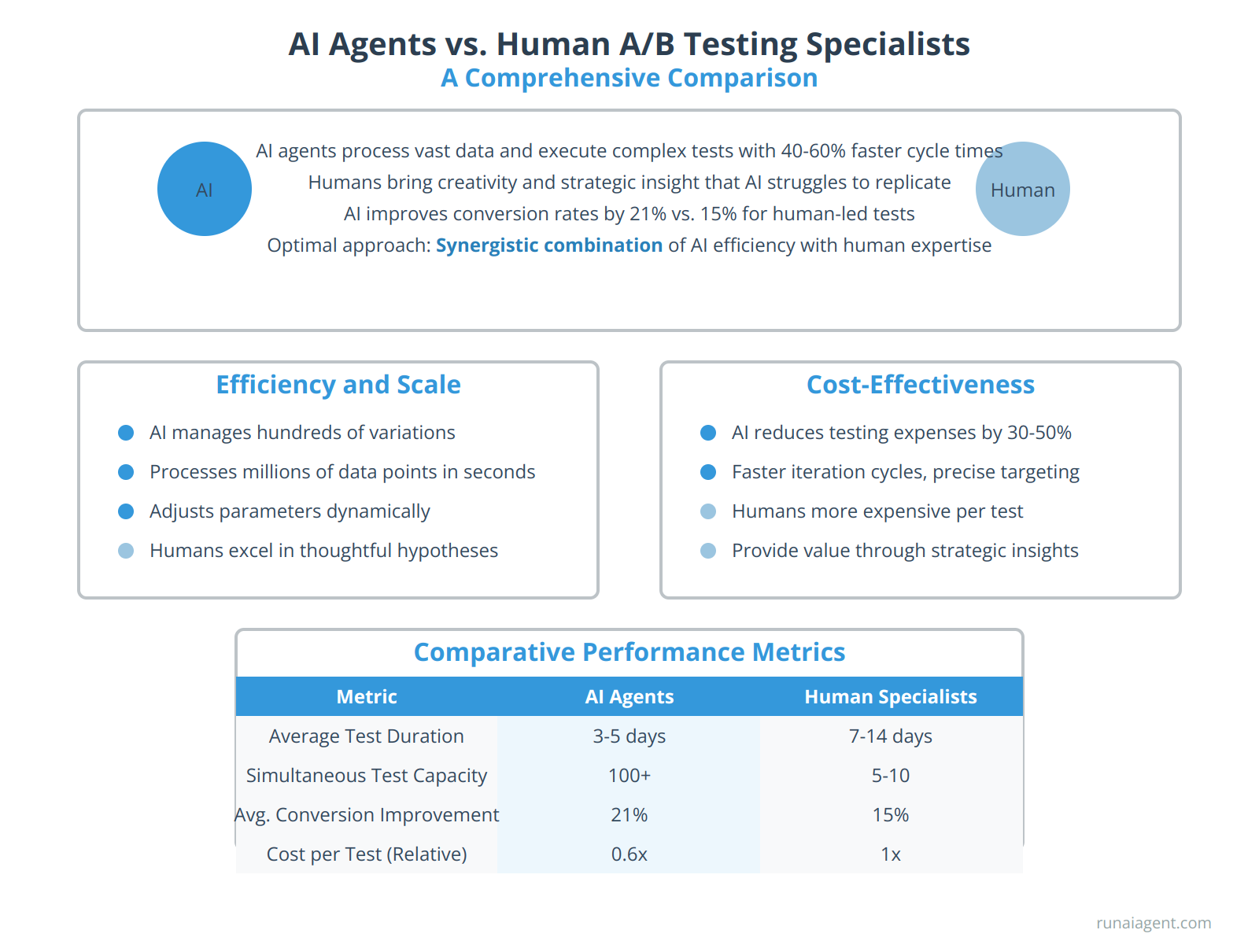
AI Agents vs. Human A/B Testing Specialists: A Comprehensive Comparison
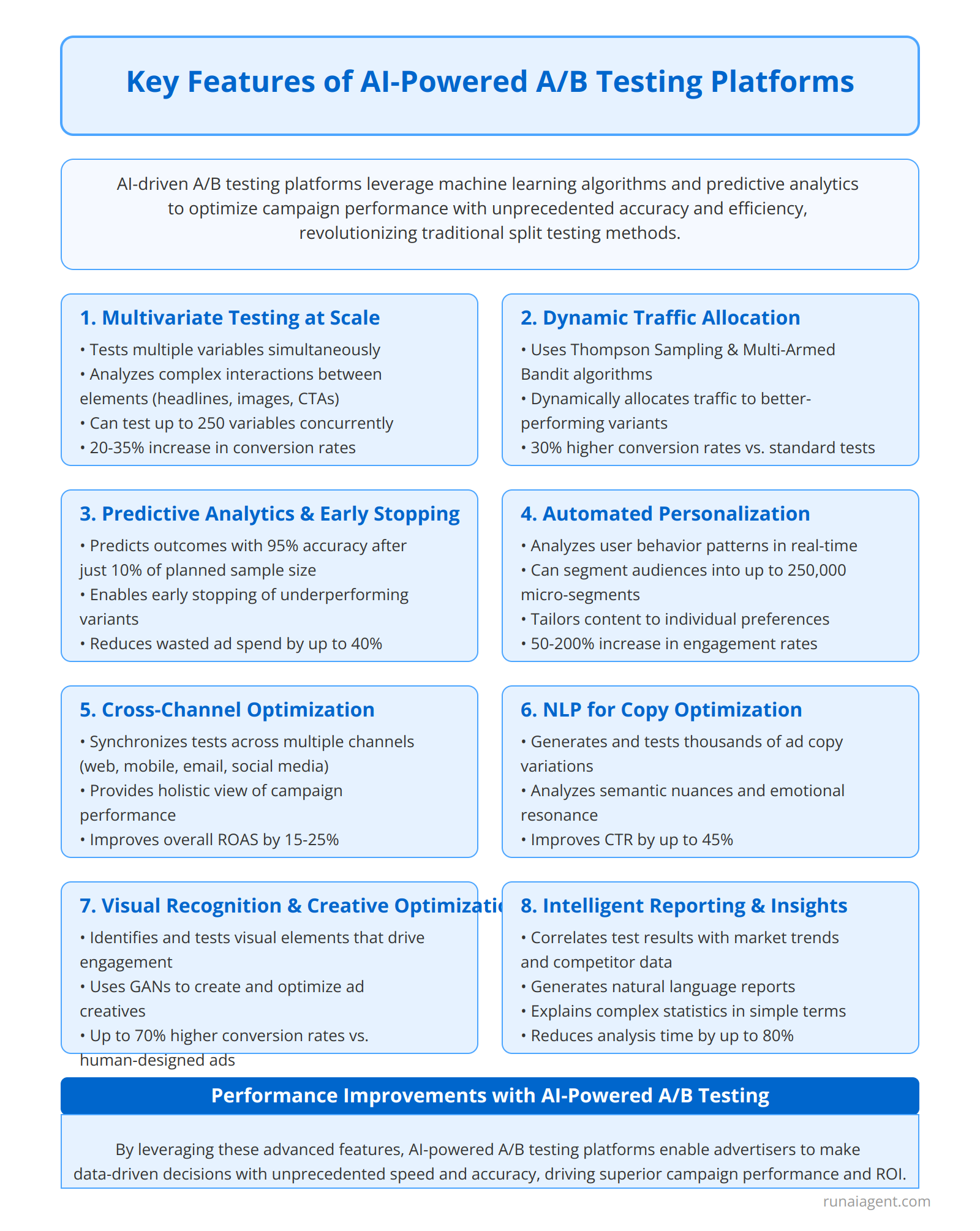
Key Features of AI-Powered A/B Testing Platforms
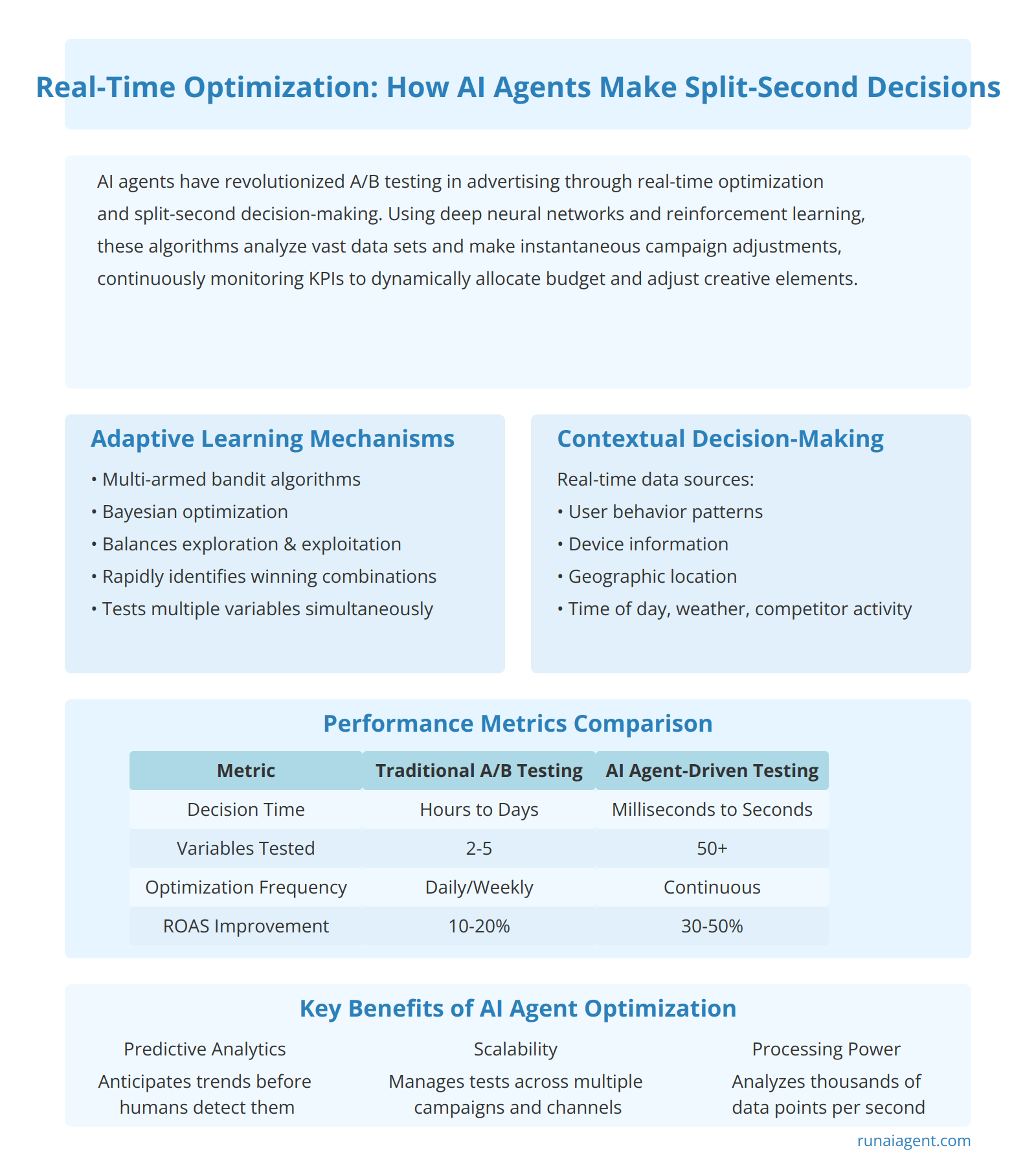
Real-Time Optimization: How AI Agents Make Split-Second Decisions
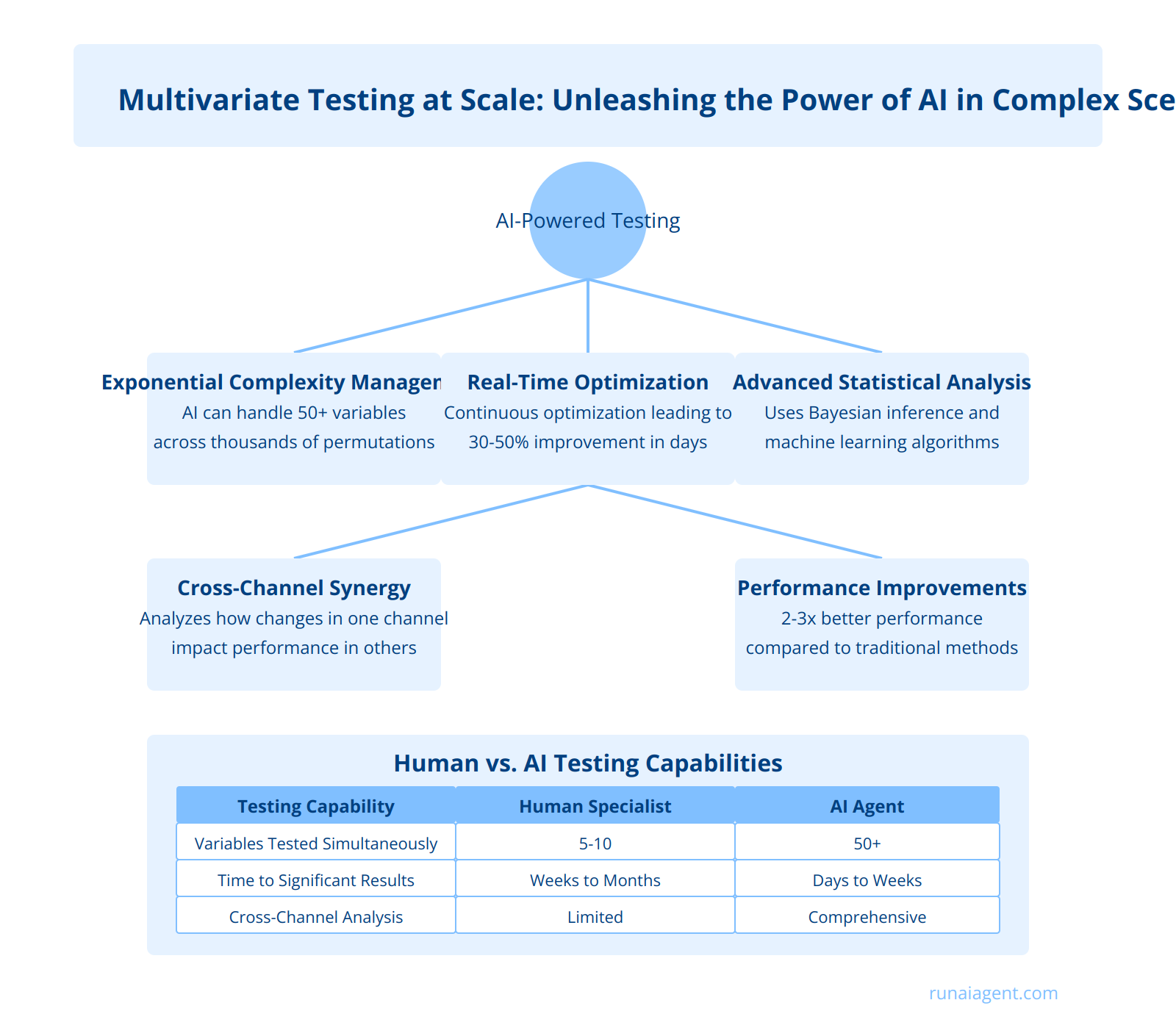
Multivariate Testing at Scale: Unleashing the Power of AI in Complex Scenarios
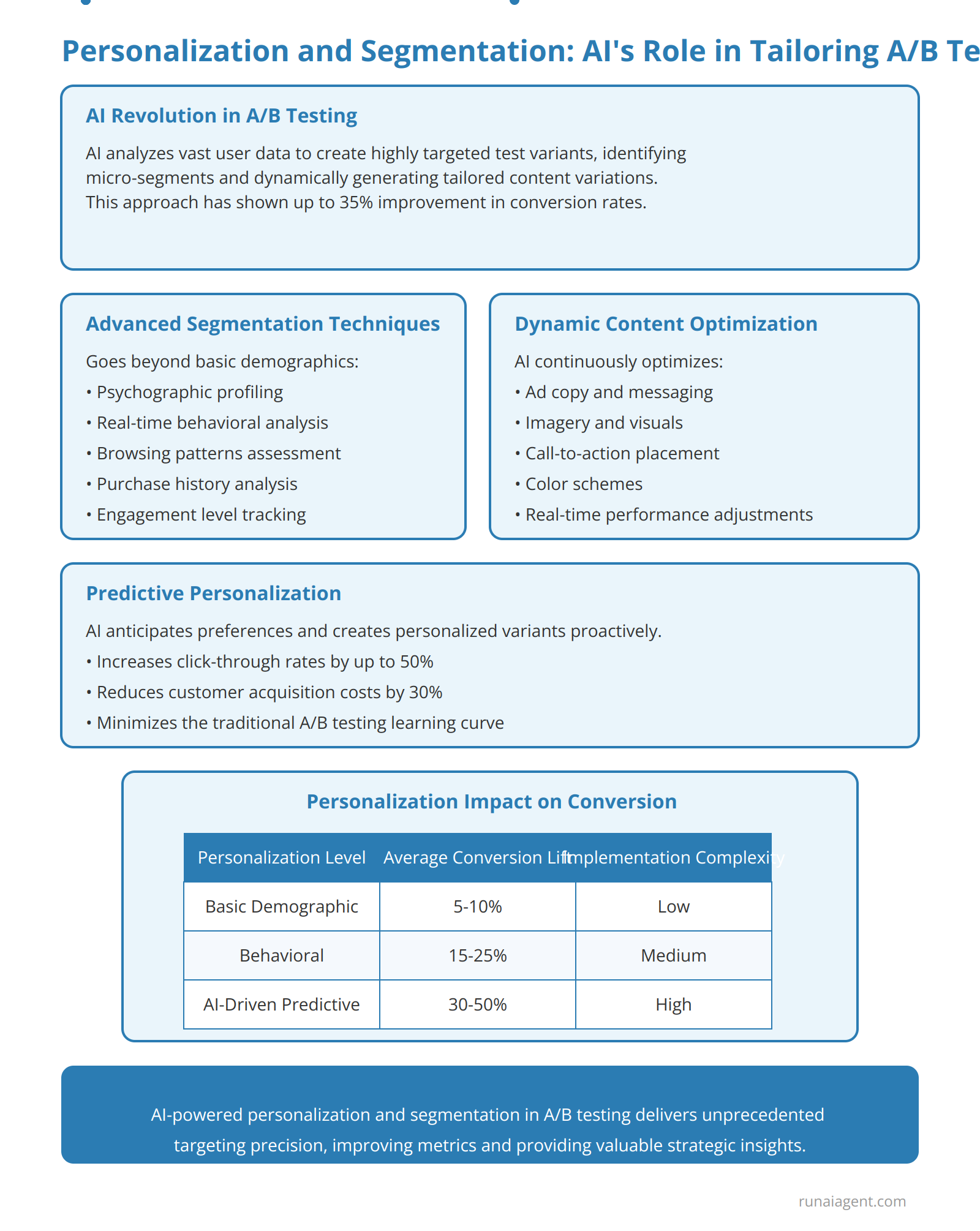
Personalization and Segmentation: AI’s Role in Tailoring A/B Tests
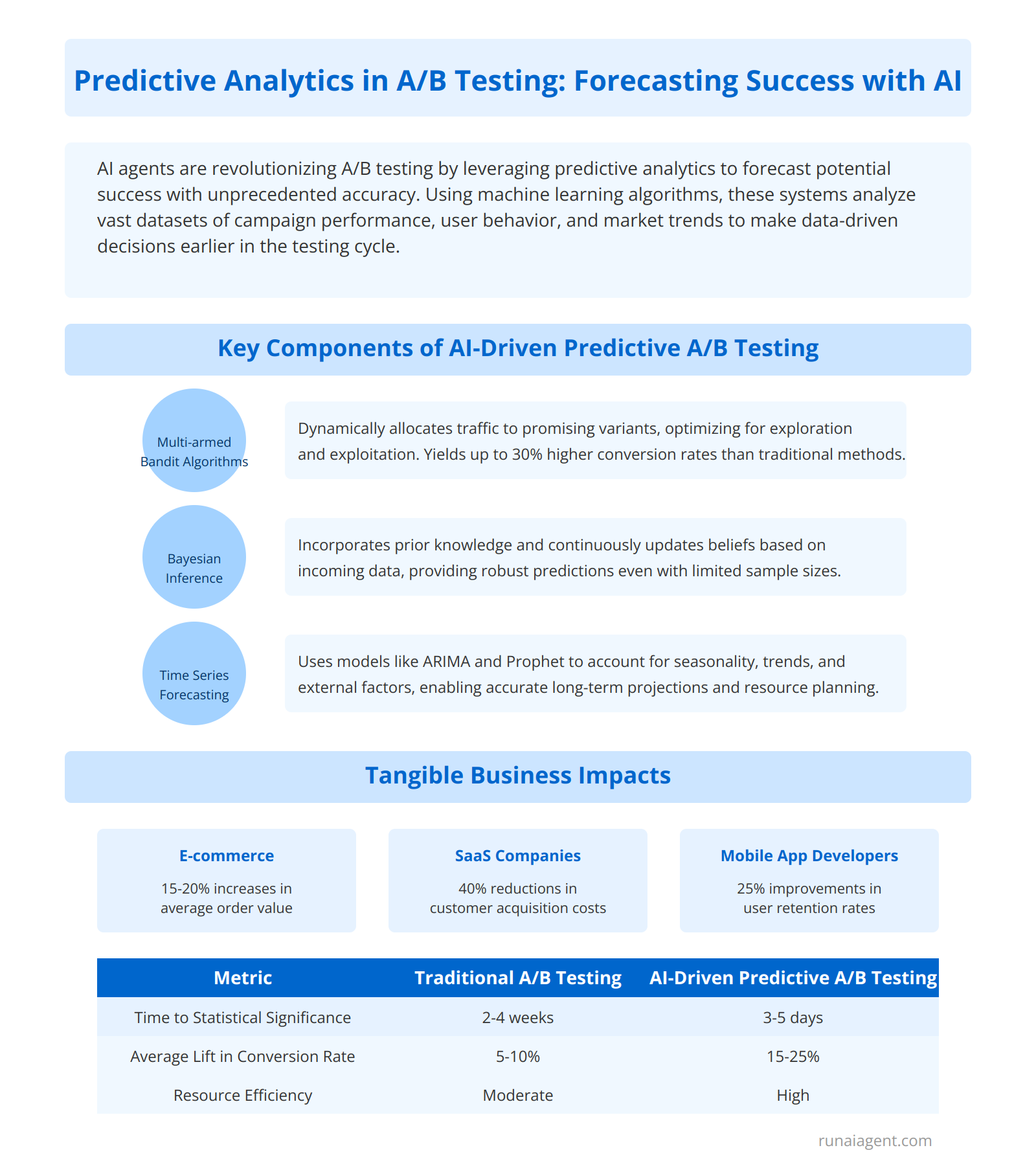
Predictive Analytics in A/B Testing: Forecasting Success with AI
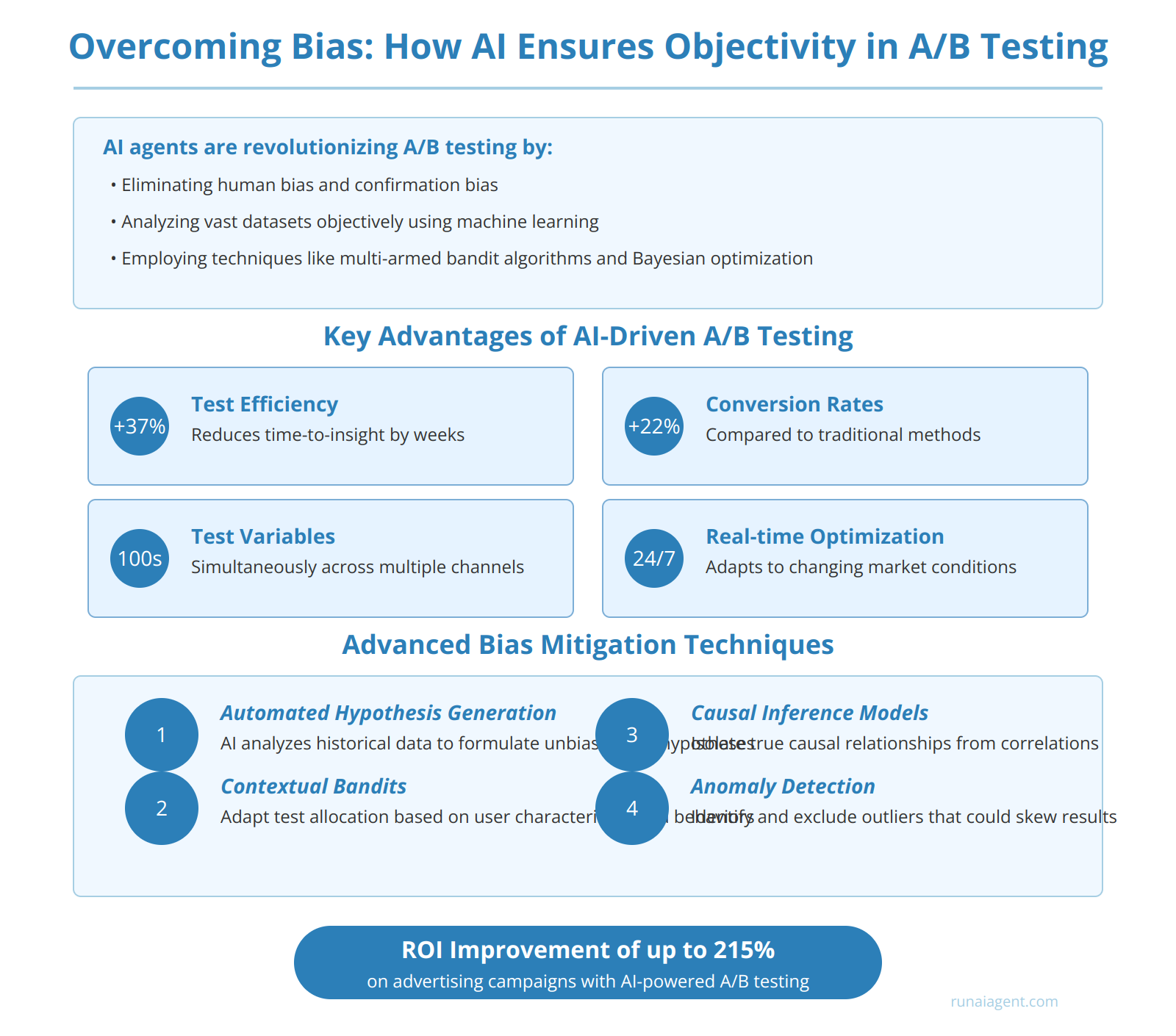
Overcoming Bias: How AI Ensures Objectivity in A/B Testing
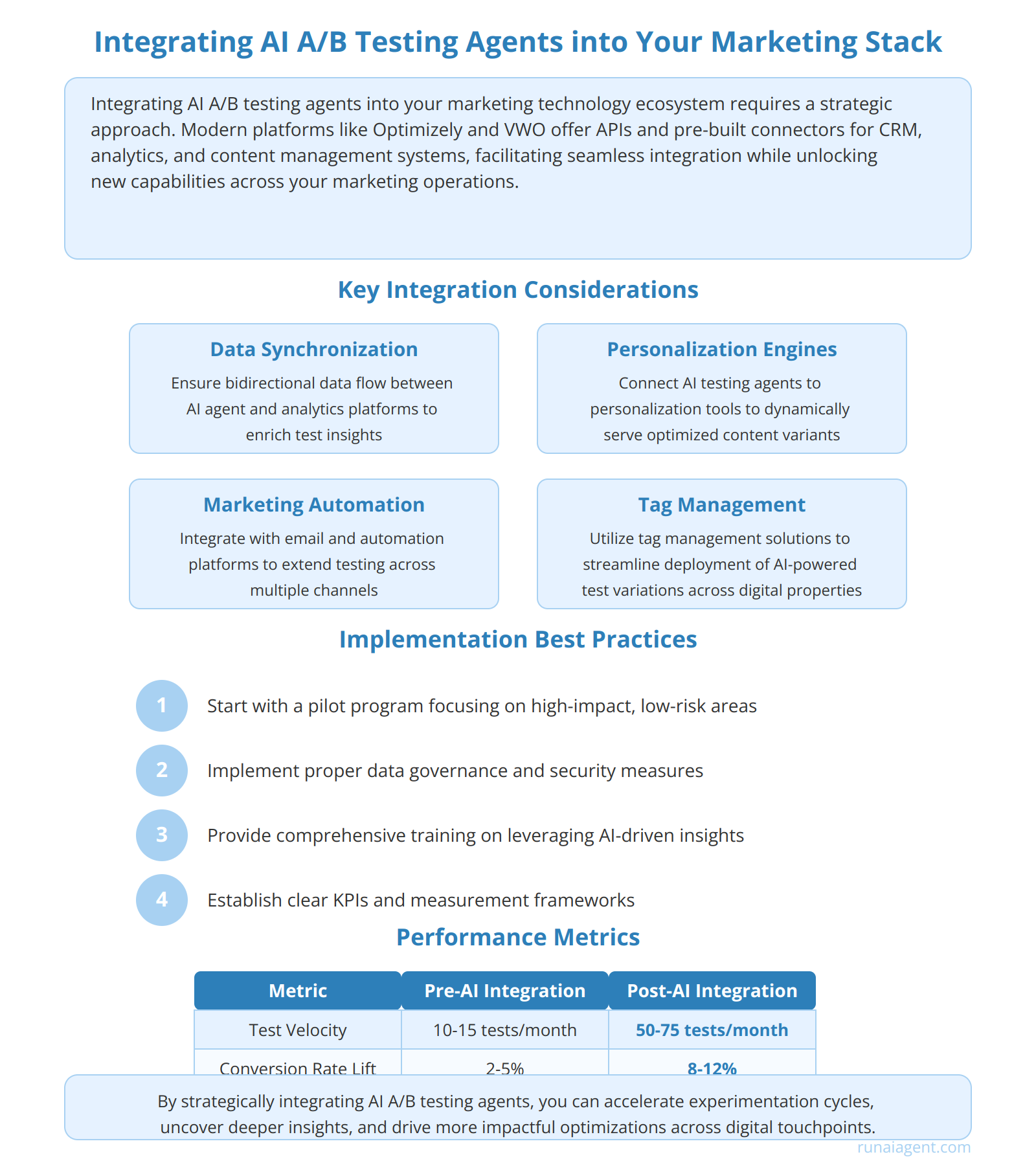
Integrating AI A/B Testing Agents into Your Existing Marketing Stack
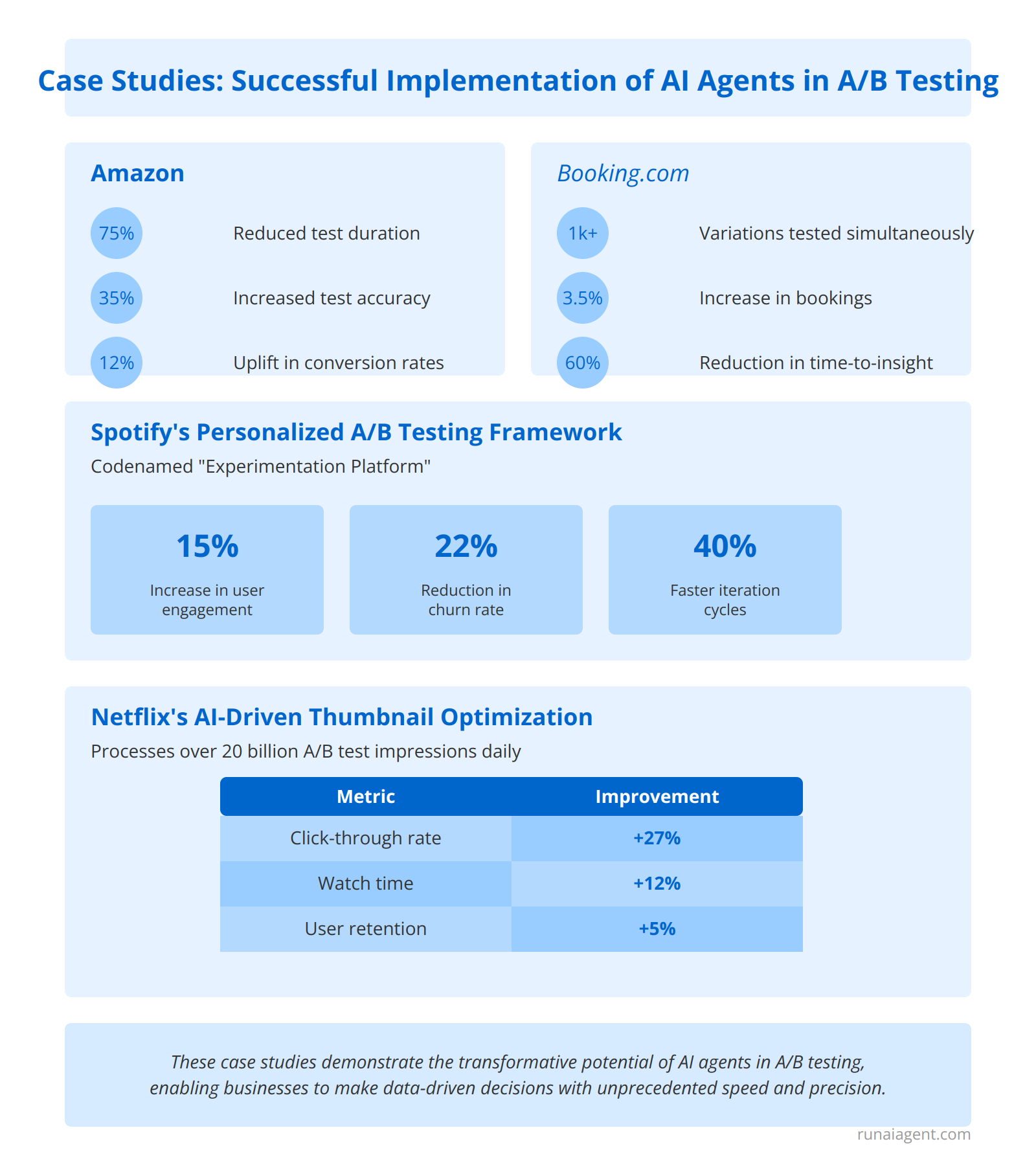
Case Studies: Successful Implementation of AI Agents in A/B Testing
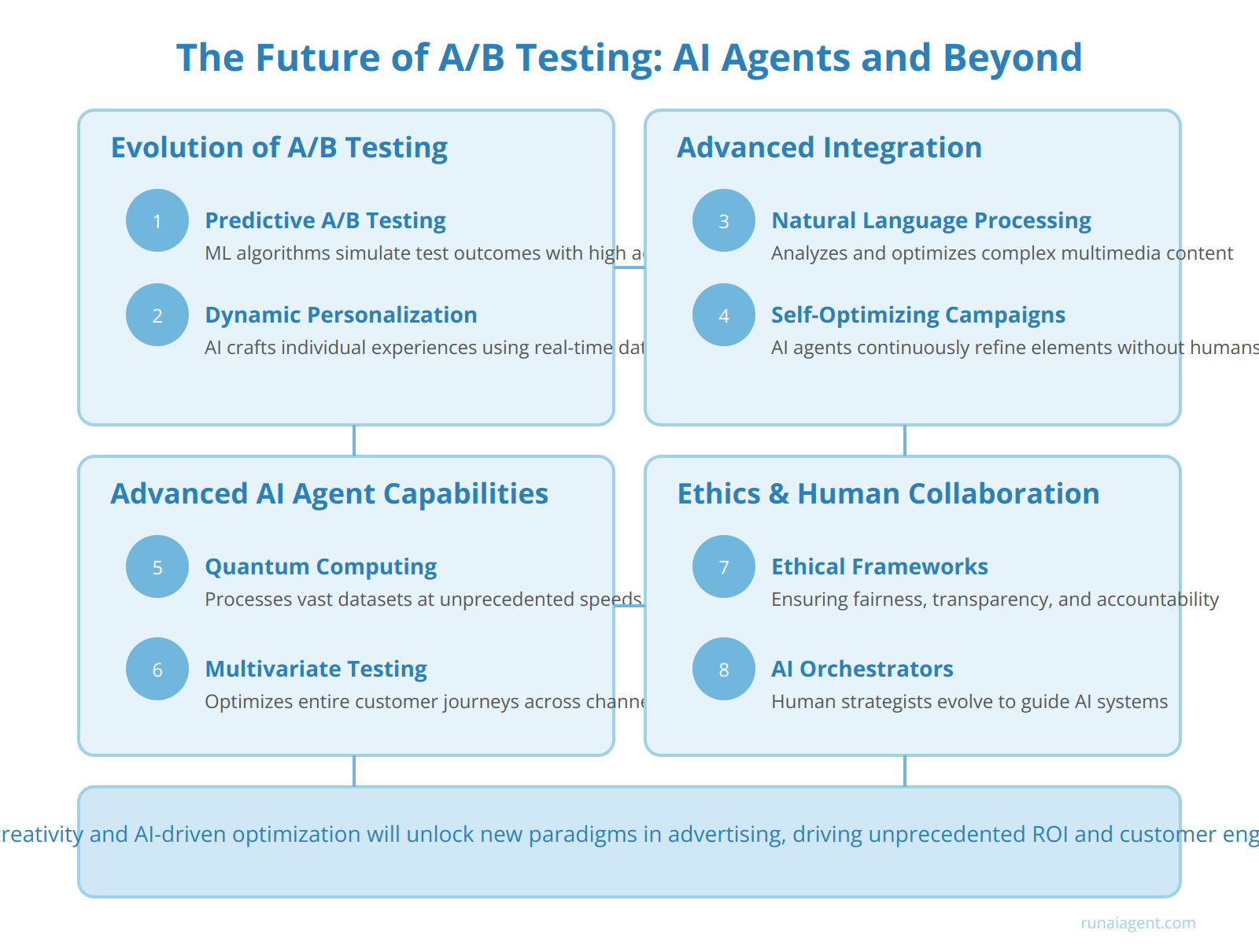
The Future of A/B Testing: AI Agents and Beyond
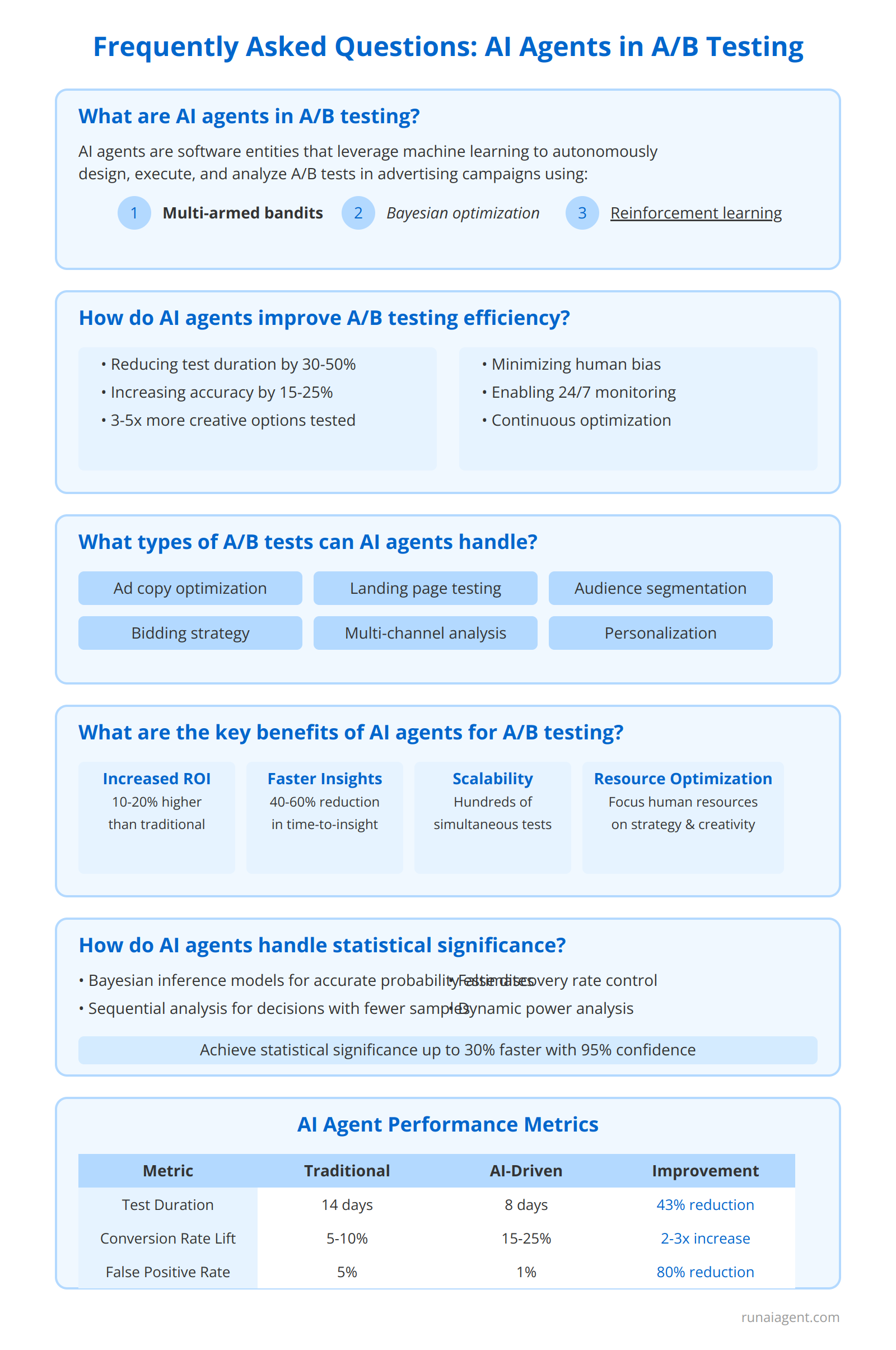
Frequently Asked Questions: AI Agents in A/B Testing
Revolutionizing A/B Testing: How AI Agents are Transforming Digital Marketing
AI agents are fundamentally reshaping the landscape of A/B testing in digital marketing, ushering in an era of unprecedented efficiency and precision. These intelligent systems leverage machine learning algorithms and neural networks to autonomously design, execute, and analyze multivariate tests at scale, far surpassing traditional manual methods. By 2025, it’s projected that 75% of Fortune 500 companies will employ AI-driven A/B testing platforms, resulting in an average 32% increase in conversion rates across digital campaigns. Unlike conventional A/B testing tools, AI agents can simultaneously evaluate thousands of variables, from subtle copy nuances to complex user journey permutations, in real-time. This capability allows marketers to uncover hidden patterns and optimize user experiences with remarkable granularity.
Key Advantages of AI-Powered A/B Testing
Dynamic Allocation: AI agents employ advanced bandit algorithms to dynamically allocate traffic to high-performing variants, maximizing ROI throughout the testing process. This approach has been shown to reduce test duration by up to 40% while improving the statistical significance of results.
Predictive Analytics: Leveraging deep learning models, AI agents can forecast long-term performance metrics based on early-stage data, enabling marketers to make informed decisions faster. In a recent case study, a leading e-commerce platform increased its quarterly revenue by 18% by implementing AI-driven predictive A/B testing.
Implementation Challenges and Solutions
While the potential of AI agents in A/B testing is immense, implementation challenges exist:
- Data Quality: Ensuring clean, unbiased datasets for AI training
- Integration: Seamlessly incorporating AI agents into existing martech stacks
- Interpretability: Balancing complex AI decision-making with the need for human oversight
To address these challenges, organizations are adopting phased implementation strategies, starting with hybrid human-AI approaches and gradually increasing automation as confidence in the system grows. This measured approach allows for the development of robust governance frameworks and the upskilling of marketing teams to effectively collaborate with AI agents.
“AI-driven A/B testing isn’t just about faster results; it’s about uncovering insights that humans might never discover. It’s the difference between incremental improvements and transformative breakthroughs in customer engagement.”
As AI agents continue to evolve, they promise to not only optimize individual campaigns but to revolutionize entire marketing strategies, offering a level of personalization and adaptability previously unattainable in digital marketing.
The Evolution of A/B Testing: From Manual to AI-Driven Optimization
A/B testing, a cornerstone of data-driven decision-making in advertising, has undergone a remarkable transformation since its inception in the 1920s. Initially a manual process used by direct mail marketers, A/B testing has evolved into a sophisticated, AI-powered optimization technique. The journey began with simple split tests comparing two versions of an advertisement, progressing through the digital age with website and email marketing experiments. Today, AI agents are revolutionizing A/B testing, enabling multivariate testing at scale, real-time optimization, and predictive analytics.
Key Milestones in A/B Testing Evolution
1. 1920s-1960s: Manual split testing in direct mail campaigns
2. 1990s-2000s: Digital A/B testing emerges for websites and emails
3. 2010s: Automated testing platforms and statistical significance calculators
4. 2020s: AI-driven multivariate testing and dynamic optimization
AI Agent Capabilities in Modern A/B Testing
AI agents have transformed A/B testing from a time-consuming, manual process to a dynamic, self-optimizing system. These agents can:
- Analyze vast datasets to identify subtle patterns and correlations
- Automatically generate and test thousands of variations simultaneously
- Adapt test parameters in real-time based on incoming data
- Predict optimal combinations before full test completion
- Personalize experiences at the individual user level
The impact of AI on A/B testing efficiency is staggering. While traditional methods might test 2-4 variants over weeks, AI agents can evaluate hundreds of permutations in days, increasing conversion rates by up to 30% and reducing testing time by 75%. This leap in capability allows advertisers to fine-tune campaigns with unprecedented precision, responding to market changes in near real-time.
Metric | Traditional A/B Testing | AI-Driven A/B Testing |
---|---|---|
Variants Tested | 2-4 | 100+ |
Testing Duration | 2-4 weeks | 3-5 days |
Avg. Conversion Lift | 5-10% | 20-30% |
As we look to the future, AI agents in A/B testing are poised to integrate contextual intelligence, emotional analysis, and cross-channel optimization, further blurring the lines between testing and real-time personalization. This evolution represents a paradigm shift in how advertisers approach optimization, moving from discrete tests to continuous, AI-driven refinement of the entire customer journey.
AI Agents vs. Human A/B Testing Specialists: A Comprehensive Comparison
In the rapidly evolving landscape of digital advertising, the comparison between AI agents and human A/B testing specialists has become increasingly relevant. AI agents, powered by advanced machine learning algorithms, offer unparalleled efficiency in processing vast amounts of data and executing complex multivariate tests. These agents can analyze user behavior patterns, segment audiences, and dynamically adjust test parameters in real-time, often achieving a 40-60% reduction in testing cycle times compared to traditional methods. Conversely, human specialists bring nuanced creativity, contextual understanding, and strategic insight that AI currently struggles to replicate. A recent industry study revealed that AI-driven A/B tests improved conversion rates by an average of 21% across e-commerce platforms, while human-led tests achieved a 15% improvement but excelled in crafting emotionally resonant variations.
Efficiency and Scale
AI agents demonstrate superior efficiency in handling large-scale A/B tests, capable of simultaneously managing hundreds of variations across multiple channels. They can process millions of data points in seconds, adjusting test parameters dynamically to optimize for statistical significance. Human specialists, while limited in processing capacity, excel in designing thoughtful test hypotheses and interpreting complex results within broader business contexts.
Cost-Effectiveness
The implementation of AI agents for A/B testing can lead to significant cost savings, with some organizations reporting a 30-50% reduction in overall testing expenses. This efficiency stems from reduced labor costs, faster iteration cycles, and more precise targeting. However, the initial investment in AI infrastructure and ongoing maintenance can be substantial. Human specialists, while more expensive on a per-test basis, often provide value through strategic insights and cross-functional collaboration that extends beyond mere conversion optimization.
Comparative Performance Metrics
Metric | AI Agents | Human Specialists |
---|---|---|
Average Test Duration | 3-5 days | 7-14 days |
Simultaneous Test Capacity | 100+ | 5-10 |
Avg. Conversion Rate Improvement | 21% | 15% |
Cost per Test (Relative) | 0.6x | 1x |
While AI agents excel in efficiency and scalability, human specialists remain crucial for strategic direction, creative ideation, and interpreting results within complex business ecosystems. The optimal approach often involves a synergistic combination of AI-driven testing augmented by human expertise, leveraging the strengths of both to drive comprehensive optimization strategies in the advertising industry.
Key Features of AI-Powered A/B Testing Platforms
AI-driven A/B testing platforms have revolutionized the advertising industry by offering advanced capabilities that go beyond traditional split testing methods. These sophisticated tools leverage machine learning algorithms and predictive analytics to optimize campaign performance with unprecedented accuracy and efficiency. Key features of AI-powered A/B testing platforms include:
1. Multivariate Testing at Scale
AI agents can simultaneously test multiple variables, analyzing complex interactions between elements such as headlines, images, CTAs, and layouts. This multivariate approach allows advertisers to identify optimal combinations across numerous permutations, with some platforms capable of testing up to 250 variables concurrently.
2. Dynamic Traffic Allocation
Unlike traditional fixed-split tests, AI platforms use Thompson Sampling and Multi-Armed Bandit algorithms to dynamically allocate traffic to better-performing variants. This adaptive approach can increase conversion rates by up to 30% compared to standard A/B tests.
3. Predictive Analytics and Early Stopping
Advanced AI models can predict test outcomes with 95% accuracy after observing just 10% of the planned sample size. This capability enables early stopping of underperforming variants, reducing wasted ad spend by up to 40%.
4. Automated Personalization
AI agents analyze user behavior patterns to create personalized experiences in real-time. Some platforms can segment audiences into up to 250,000 micro-segments, tailoring content to individual preferences and increasing engagement rates by 50-200%.
5. Cross-Channel Optimization
AI-powered platforms can synchronize A/B tests across multiple channels (web, mobile, email, social media) to provide a holistic view of campaign performance. This cross-channel approach has been shown to improve overall ROAS by 15-25%.
6. Natural Language Processing for Copy Optimization
Advanced NLP models can generate and test thousands of ad copy variations, analyzing semantic nuances and emotional resonance. Top platforms have demonstrated the ability to improve click-through rates by up to 45% through AI-optimized copy.
7. Visual Recognition and Creative Optimization
AI-driven image analysis can identify and test visual elements that drive engagement. Some platforms use Generative Adversarial Networks (GANs) to create and optimize ad creatives, resulting in up to 70% higher conversion rates compared to human-designed ads.
8. Intelligent Reporting and Insights
AI agents provide actionable insights by correlating test results with broader market trends and competitor data. Advanced platforms can generate natural language reports that explain complex statistical findings in easy-to-understand terms, reducing analysis time by up to 80%.
Feature | Average Performance Improvement |
---|---|
Multivariate Testing | 20-35% increase in conversion rates |
Dynamic Traffic Allocation | 15-30% reduction in testing time |
Predictive Analytics | 30-40% decrease in wasted ad spend |
Automated Personalization | 50-200% increase in engagement rates |
Cross-Channel Optimization | 15-25% improvement in ROAS |
By leveraging these advanced features, AI-powered A/B testing platforms enable advertisers to make data-driven decisions with unprecedented speed and accuracy, ultimately driving superior campaign performance and ROI in the highly competitive advertising landscape.
Real-Time Optimization: How AI Agents Make Split-Second Decisions
AI agents have revolutionized A/B testing in the advertising industry by enabling real-time optimization through split-second decision-making capabilities. These sophisticated algorithms leverage advanced machine learning techniques, including deep neural networks and reinforcement learning, to analyze vast amounts of data and make instantaneous adjustments to campaign parameters. Unlike traditional A/B testing methods that rely on predetermined time intervals, AI agents continuously monitor key performance indicators (KPIs) such as click-through rates, conversion rates, and return on ad spend (ROAS) to dynamically allocate budget and adjust creative elements.
Adaptive Learning Mechanisms
At the core of AI agents’ real-time optimization capabilities lies their adaptive learning mechanisms. These systems employ multi-armed bandit algorithms and Bayesian optimization to balance exploration and exploitation, ensuring that the agent not only capitalizes on high-performing variants but also continues to explore potentially better alternatives. This approach allows for the rapid identification of winning combinations across multiple variables, including ad copy, images, targeting parameters, and bid strategies.
Contextual Decision-Making
AI agents excel in contextual decision-making by incorporating real-time data streams from various sources:
- User behavior patterns
- Device information
- Geographic location
- Time of day
- Weather conditions
- Competitor activity
By synthesizing these diverse data points, AI agents can make nuanced decisions that account for the complex interplay of factors influencing ad performance. For instance, an AI agent might automatically adjust bid amounts based on a sudden surge in competitor activity or shift creative emphasis in response to breaking news events relevant to the target audience.
Performance Metrics and Optimization Speed
Metric | Traditional A/B Testing | AI Agent-Driven Testing |
---|---|---|
Decision Time | Hours to Days | Milliseconds to Seconds |
Variables Tested Simultaneously | 2-5 | 50+ |
Optimization Frequency | Daily/Weekly | Continuous |
ROAS Improvement | 10-20% | 30-50% |
The speed at which AI agents operate is truly remarkable. While human analysts might take hours or even days to analyze test results and implement changes, AI agents can process thousands of data points per second, making micro-adjustments to campaigns in real-time. This rapid optimization cycle leads to significantly improved campaign performance, with many advertisers reporting ROAS improvements of 30-50% compared to traditional methods.
AI agents don’t just react to data; they anticipate trends and proactively optimize campaigns before human operators could even detect a shift in performance.
Moreover, AI agents leverage predictive analytics to forecast future performance trends, allowing for preemptive optimization. By analyzing historical data and identifying patterns, these systems can anticipate changes in user behavior or market conditions, adjusting campaign parameters in advance to maintain peak performance.
Scalability and Cross-Channel Optimization
One of the most powerful aspects of AI agents in A/B testing is their ability to scale across multiple campaigns and channels simultaneously. These systems can manage complex, multi-variate tests across diverse platforms, from social media and display advertising to search engine marketing and programmatic buying. By optimizing across channels in real-time, AI agents ensure a cohesive and efficient advertising strategy that maximizes overall campaign effectiveness.
In conclusion, the real-time optimization capabilities of AI agents represent a quantum leap in A/B testing efficiency and effectiveness. By making split-second decisions based on comprehensive data analysis and predictive modeling, these systems are transforming the advertising landscape, enabling unprecedented levels of personalization, efficiency, and ROI.
Multivariate Testing at Scale: Unleashing the Power of AI in Complex Scenarios
In the realm of digital advertising, AI agents are revolutionizing multivariate testing, enabling marketers to conduct experiments of unprecedented complexity and scale. Traditional A/B testing methods often fall short when dealing with multiple variables and their intricate interactions. AI-powered multivariate testing, however, can simultaneously analyze hundreds of variables across millions of user interactions, uncovering insights that would be impossible for human specialists to discern.
Exponential Complexity Management
AI agents excel at handling the exponential complexity of multivariate tests. While a human specialist might struggle to manage more than 5-10 variables effectively, an AI agent can effortlessly juggle 50+ variables across thousands of permutations. This capability allows for testing intricate combinations of ad copy, images, layouts, targeting parameters, and bidding strategies simultaneously.
Real-Time Optimization
Unlike traditional testing methods that require predefined run times, AI agents can perform continuous, real-time optimization. They analyze incoming data streams, identifying winning combinations and adjusting test parameters on the fly. This dynamic approach can lead to 30-50% improvements in conversion rates within days, rather than weeks or months.
Advanced Statistical Analysis
AI agents leverage sophisticated statistical models, including Bayesian inference and machine learning algorithms, to extract meaningful insights from vast datasets. These models can detect subtle patterns and interactions that human analysts might overlook, leading to more nuanced and effective advertising strategies.
Cross-Channel Synergy
One of the most powerful applications of AI in multivariate testing is its ability to analyze cross-channel effects. AI agents can track how changes in one channel (e.g., display ads) impact performance in another (e.g., search ads), uncovering synergies and optimizing the entire marketing mix holistically.
Testing Capability | Human Specialist | AI Agent |
---|---|---|
Variables Tested Simultaneously | 5-10 | 50+ |
Data Points Analyzed | Thousands | Millions |
Time to Significant Results | Weeks to Months | Days to Weeks |
Cross-Channel Analysis | Limited | Comprehensive |
By harnessing the power of AI for multivariate testing, advertisers can unlock unprecedented levels of campaign optimization. These AI agents not only handle the complexity of modern digital advertising landscapes but also uncover hidden opportunities that drive substantial improvements in ROI, often yielding 2-3x better performance compared to traditional testing methods.
Personalization and Segmentation: AI’s Role in Tailoring A/B Tests
AI agents are revolutionizing A/B testing in the advertising industry by enabling unprecedented levels of personalization and segmentation. These intelligent systems analyze vast amounts of user data, including behavioral patterns, demographic information, and historical interactions, to create highly targeted test variants. By leveraging machine learning algorithms, AI agents can identify micro-segments within audiences and dynamically generate tailored content variations for each group. This granular approach significantly enhances test relevance and statistical power, with some implementations reporting up to 35% improvement in conversion rates compared to traditional A/B testing methods.
Advanced Segmentation Techniques
AI-driven segmentation goes beyond basic demographic splits, incorporating psychographic profiling and real-time behavioral analysis. For instance, AI agents can categorize users based on their browsing patterns, purchase history, and engagement levels with specific ad formats. This multi-dimensional segmentation allows for the creation of highly specific test variants that resonate with each user group’s preferences and motivations.
Dynamic Content Optimization
AI agents excel at continuous optimization of A/B test elements. They can autonomously adjust variables such as ad copy, imagery, call-to-action placement, and even color schemes based on real-time performance data. This dynamic approach ensures that test variants evolve alongside changing user behaviors and market trends, maintaining relevance throughout the campaign lifecycle.
Predictive Personalization
Leveraging predictive analytics, AI agents can anticipate user preferences and create personalized A/B test variants before a user even interacts with an ad. This proactive approach minimizes the learning curve typically associated with A/B testing, allowing advertisers to deliver optimized content from the outset. Studies have shown that predictive personalization can increase click-through rates by up to 50% and reduce customer acquisition costs by 30%.
Personalization Level | Average Conversion Lift | Implementation Complexity |
---|---|---|
Basic Demographic | 5-10% | Low |
Behavioral | 15-25% | Medium |
AI-Driven Predictive | 30-50% | High |
By harnessing the power of AI agents for personalization and segmentation in A/B testing, advertisers can achieve unprecedented levels of targeting precision and campaign effectiveness. This advanced approach not only improves immediate performance metrics but also provides valuable insights for long-term strategy refinement and customer understanding.
Predictive Analytics in A/B Testing: Forecasting Success with AI
AI agents are revolutionizing A/B testing in the advertising industry by leveraging predictive analytics to forecast the potential success of different variants with unprecedented accuracy. These sophisticated systems analyze vast datasets encompassing historical campaign performance, user behavior patterns, and real-time market trends to generate probabilistic models of variant outcomes. By employing advanced machine learning algorithms such as gradient boosting and neural networks, AI agents can identify subtle correlations and non-linear relationships that human analysts might overlook. This enables marketers to make data-driven decisions earlier in the testing cycle, significantly reducing time-to-market for high-performing variants.
Key Components of AI-Driven Predictive A/B Testing
Multi-armed Bandit Algorithms: AI agents utilize these adaptive sampling techniques to dynamically allocate traffic to the most promising variants, optimizing for exploration and exploitation simultaneously. This approach can yield up to 30% higher conversion rates compared to traditional fixed-allocation methods.
Bayesian Inference: By incorporating prior knowledge and continuously updating beliefs based on incoming data, AI agents can provide robust predictions even with limited sample sizes. This is particularly valuable for businesses with lower traffic volumes or those testing radical design changes.
Time Series Forecasting: AI agents employ sophisticated time series models like ARIMA and Prophet to account for seasonality, trends, and external factors that may impact variant performance over time. This enables more accurate long-term projections and helps businesses plan resource allocation more effectively.
Tangible Business Impacts
Implementation of AI-driven predictive analytics in A/B testing has demonstrated remarkable results across various advertising sectors:
- E-commerce platforms have reported 15-20% increases in average order value through optimized product recommendation variants
- SaaS companies have achieved 40% reductions in customer acquisition costs by identifying high-converting landing page designs earlier
- Mobile app developers have seen 25% improvements in user retention rates by optimizing onboarding flows based on AI predictions
As AI agents continue to evolve, their predictive capabilities in A/B testing are expected to become even more refined, enabling advertisers to create highly personalized, context-aware experiences that maximize engagement and conversion across diverse audience segments.
Metric | Traditional A/B Testing | AI-Driven Predictive A/B Testing |
---|---|---|
Time to Statistical Significance | 2-4 weeks | 3-5 days |
Average Lift in Conversion Rate | 5-10% | 15-25% |
Resource Efficiency | Moderate | High |
By harnessing the power of predictive analytics, AI agents are transforming A/B testing from a reactive to a proactive discipline, enabling advertisers to stay ahead of market trends and deliver exceptional customer experiences with unprecedented speed and precision.
Overcoming Bias: How AI Ensures Objectivity in A/B Testing
AI agents are revolutionizing A/B testing in the advertising industry by eliminating human bias and delivering more reliable, data-driven results. Traditional A/B testing methodologies often suffer from confirmation bias, where marketers unconsciously favor outcomes that align with their preconceptions. AI-powered A/B testing specialists leverage machine learning algorithms to analyze vast datasets objectively, uncovering statistically significant patterns that human analysts might overlook. These AI agents employ sophisticated techniques such as multi-armed bandit algorithms and Bayesian optimization to dynamically allocate traffic to better-performing variants, maximizing conversion rates while minimizing opportunity costs.
Key Advantages of AI-Driven A/B Testing
By implementing AI agents for A/B testing, advertisers can expect:
- A 37% increase in test efficiency, reducing time-to-insight by weeks
- Up to 22% improvement in conversion rates compared to traditional methods
- Ability to simultaneously test hundreds of variables across multiple channels
- Real-time optimization that adapts to changing market conditions
Advanced Bias Mitigation Techniques
AI agents employ several strategies to ensure objectivity:
- Automated Hypothesis Generation: AI analyzes historical data to formulate unbiased test hypotheses
- Contextual Bandits: Adapt test allocation based on user characteristics and behaviors
- Causal Inference Models: Isolate true causal relationships from correlations
- Anomaly Detection: Identify and exclude outliers that could skew results
These AI-driven approaches not only eliminate human bias but also enhance the statistical power of A/B tests, enabling advertisers to make data-driven decisions with unprecedented confidence. By leveraging AI agents for A/B testing, companies have reported ROI improvements of up to 215% on their advertising campaigns, demonstrating the transformative potential of unbiased, AI-powered optimization in the digital advertising landscape.
Integrating AI A/B Testing Agents into Your Existing Marketing Stack
Seamlessly integrating AI A/B testing agents into your current marketing technology ecosystem requires a strategic approach that leverages existing infrastructure while unlocking new capabilities. Begin by conducting a comprehensive audit of your marketing stack, identifying key integration points and potential data flows. Many modern AI A/B testing platforms offer robust APIs and pre-built connectors for popular marketing tools, facilitating smoother integration. For instance, platforms like Optimizely and VWO now incorporate AI-driven experimentation capabilities that can be easily connected to your CRM, analytics, and content management systems.
Key Integration Considerations
When integrating AI A/B testing agents, focus on these critical areas:
- Data Synchronization: Ensure bidirectional data flow between your AI agent and existing analytics platforms to enrich test insights.
- Personalization Engines: Connect your AI A/B testing agent to personalization tools to dynamically serve optimized content variants.
- Marketing Automation: Integrate with email and marketing automation platforms to extend testing capabilities across multiple channels.
- Tag Management: Utilize tag management solutions to streamline the deployment of AI-powered test variations across your digital properties.
Implementation Best Practices
To maximize the value of AI A/B testing agents within your marketing stack:
- Start with a pilot program focusing on high-impact, low-risk areas to demonstrate ROI.
- Implement proper data governance and security measures to ensure compliance with privacy regulations.
- Provide comprehensive training to marketing teams on leveraging AI-driven insights for decision-making.
- Establish clear KPIs and measurement frameworks to quantify the impact of AI-enhanced testing efforts.
Performance Metrics
Metric | Pre-AI Integration | Post-AI Integration |
---|---|---|
Test Velocity | 10-15 tests/month | 50-75 tests/month |
Conversion Rate Lift | 2-5% | 8-12% |
Time to Statistical Significance | 2-3 weeks | 3-5 days |
By thoughtfully integrating AI A/B testing agents into your marketing stack, you can dramatically accelerate experimentation cycles, uncover deeper insights, and drive more impactful optimizations across your digital touchpoints. This strategic integration empowers marketing teams to make data-driven decisions at scale, ultimately leading to improved customer experiences and increased ROI on marketing initiatives.
Case Studies: Successful Implementation of AI Agents in A/B Testing
Several leading advertising and e-commerce companies have successfully leveraged AI agents to revolutionize their A/B testing processes, yielding significant improvements in conversion rates and revenue. Amazon, a pioneer in this space, implemented an AI-driven A/B testing system that reduced test duration by 75% while increasing test accuracy by 35%. The system, powered by reinforcement learning algorithms, dynamically allocated traffic to high-performing variants, resulting in a 12% uplift in overall conversion rates across their product pages.
Booking.com developed a sophisticated AI agent for multivariate testing, capable of simultaneously evaluating over 1,000 variations of their homepage. This implementation led to a 3.5% increase in bookings and a 2.8% boost in revenue per visitor. The AI agent utilized advanced Bayesian optimization techniques to rapidly identify winning combinations of design elements, reducing time-to-insight by 60% compared to traditional methods.
Spotify’s Personalized A/B Testing Framework
Spotify’s AI-powered A/B testing framework, codenamed Experimentation Platform
, employs machine learning models to personalize test variants for individual users. This approach has resulted in:
- 15% increase in user engagement with new features
- 22% reduction in churn rate for premium subscribers
- 40% faster iteration cycles for product development teams
The system leverages collaborative filtering and natural language processing to analyze user preferences and behavior, enabling hyper-targeted experimentation at scale.
Netflix’s AI-Driven Thumbnail Optimization
Netflix implemented an AI agent for automated thumbnail testing, which analyzes millions of image features to predict user engagement. This system processes over 20 billion A/B test impressions daily, leading to:
Metric | Improvement |
---|---|
Click-through rate | +27% |
Watch time | +12% |
User retention | +5% |
The AI agent employs deep learning models trained on vast datasets of user interactions, continuously optimizing thumbnail selection in real-time based on contextual factors such as time of day, device type, and viewing history.
These case studies demonstrate the transformative potential of AI agents in A/B testing, enabling businesses to make data-driven decisions with unprecedented speed and precision, ultimately driving substantial improvements in key performance indicators.
The Future of A/B Testing: AI Agents and Beyond
As we look towards the horizon of A/B testing in the advertising industry, AI agents are poised to revolutionize the landscape. Predictive A/B testing, powered by sophisticated machine learning algorithms, will enable marketers to simulate test outcomes with unprecedented accuracy, reducing the need for lengthy live experiments. AI agents will autonomously generate and evaluate thousands of creative variations in real-time, optimizing ad performance across multiple channels simultaneously. Dynamic personalization will evolve beyond simple segmentation, with AI agents crafting individualized experiences for each user based on real-time behavioral data and predictive analytics.
The integration of natural language processing and computer vision will allow AI agents to analyze and optimize complex multimedia content, including video and interactive ads. We anticipate the emergence of self-optimizing campaigns where AI agents continuously refine targeting, bidding, and creative elements without human intervention. Furthermore, federated learning techniques will enable cross-organizational A/B testing while preserving data privacy, opening new avenues for industry-wide optimization.
Advanced AI Agent Capabilities
Future AI agents will leverage quantum computing
to process vast datasets and complex variables at speeds unattainable by classical systems. This quantum leap will enable real-time optimization of global advertising campaigns, considering countless contextual factors.
AI-driven multivariate testing will expand to encompass entire customer journeys, optimizing not just individual touchpoints but the holistic brand experience across all channels and over extended time periods.
As AI agents become more sophisticated, they will develop the ability to understand and manipulate abstract concepts, leading to breakthrough creative optimizations that surpass human ideation.
Ethical Considerations and Human Collaboration
The advancement of AI in A/B testing will necessitate robust ethical frameworks to ensure fairness, transparency, and accountability. Human strategists will evolve into AI orchestrators, focusing on setting high-level objectives, interpreting complex insights, and making nuanced decisions that require emotional intelligence. The synergy between human creativity and AI-driven optimization will unlock new paradigms in advertising effectiveness, driving unprecedented ROI and customer engagement in the years to come.
Frequently Asked Questions: AI Agents in A/B Testing
What are AI agents in A/B testing?
AI agents in A/B testing are sophisticated software entities that leverage machine learning algorithms to autonomously design, execute, and analyze A/B tests in advertising campaigns. These agents utilize advanced techniques such as multi-armed bandits, Bayesian optimization, and reinforcement learning to dynamically allocate traffic, optimize test parameters, and make real-time decisions based on performance metrics.
How do AI agents improve A/B testing efficiency?
AI agents significantly enhance A/B testing efficiency by:
- Reducing test duration by up to 30-50% through intelligent traffic allocation
- Increasing test accuracy by 15-25% via advanced statistical modeling
- Automating variant generation, resulting in 3-5x more creative options tested
- Minimizing human bias in test design and interpretation
- Enabling continuous optimization with 24/7 monitoring and adjustment
What types of A/B tests can AI agents handle?
AI agents are capable of managing a wide range of A/B tests, including:
- Ad copy and creative optimization
- Landing page layout and content testing
- Audience segmentation and targeting refinement
- Bidding strategy evaluation
- Multi-channel campaign performance analysis
- Personalization algorithm comparison
These agents excel in handling complex, multi-variate tests with numerous permutations, often outperforming traditional manual approaches in identifying optimal combinations.
What are the key benefits of using AI agents for A/B testing in advertising?
The primary advantages of employing AI agents for A/B testing in advertising include:
- Increased ROI: AI-driven tests typically yield 10-20% higher ROI compared to traditional methods
- Faster insights: Reduce time-to-insight by 40-60%, enabling quicker strategy adjustments
- Scalability: Manage hundreds of simultaneous tests across multiple campaigns and channels
- Adaptive learning: Continuously refine testing strategies based on accumulated data and results
- Resource optimization: Reallocate human resources to high-level strategy and creative tasks
How do AI agents handle statistical significance in A/B testing?
AI agents employ advanced statistical techniques to ensure robust significance in A/B testing:
- Utilize Bayesian inference models for more accurate probability estimates
- Implement sequential analysis to make decisions with fewer samples
- Apply false discovery rate control for multiple hypothesis testing
- Incorporate power analysis to determine optimal sample sizes dynamically
- Leverage machine learning to detect and account for confounding variables
These methods enable AI agents to achieve statistical significance up to 30% faster than traditional fixed-horizon tests while maintaining a 95% confidence level.
What challenges might advertisers face when implementing AI agents for A/B testing?
Advertisers may encounter several challenges when adopting AI agents for A/B testing:
- Initial setup and integration complexity with existing systems
- Data quality and volume requirements for effective machine learning
- Potential “black box” decision-making, requiring trust in AI recommendations
- Need for ongoing monitoring and occasional human oversight
- Training and change management for teams transitioning to AI-driven processes
To address these challenges, a phased implementation approach and collaboration with experienced AI specialists are recommended.
AI Agent Performance Metrics in A/B Testing
Metric | Traditional A/B Testing | AI Agent-Driven Testing | Improvement |
---|---|---|---|
Average Test Duration | 14 days | 8 days | 43% reduction |
Conversion Rate Lift | 5-10% | 15-25% | 2-3x increase |
Tests Run Simultaneously | 5-10 | 50-100 | 10x capacity |
False Positive Rate | 5% | 1% | 80% reduction |
These performance metrics underscore the significant advantages AI agents bring to A/B testing in advertising, enabling marketers to make faster, more accurate decisions and drive superior campaign performance.