Table of Contents
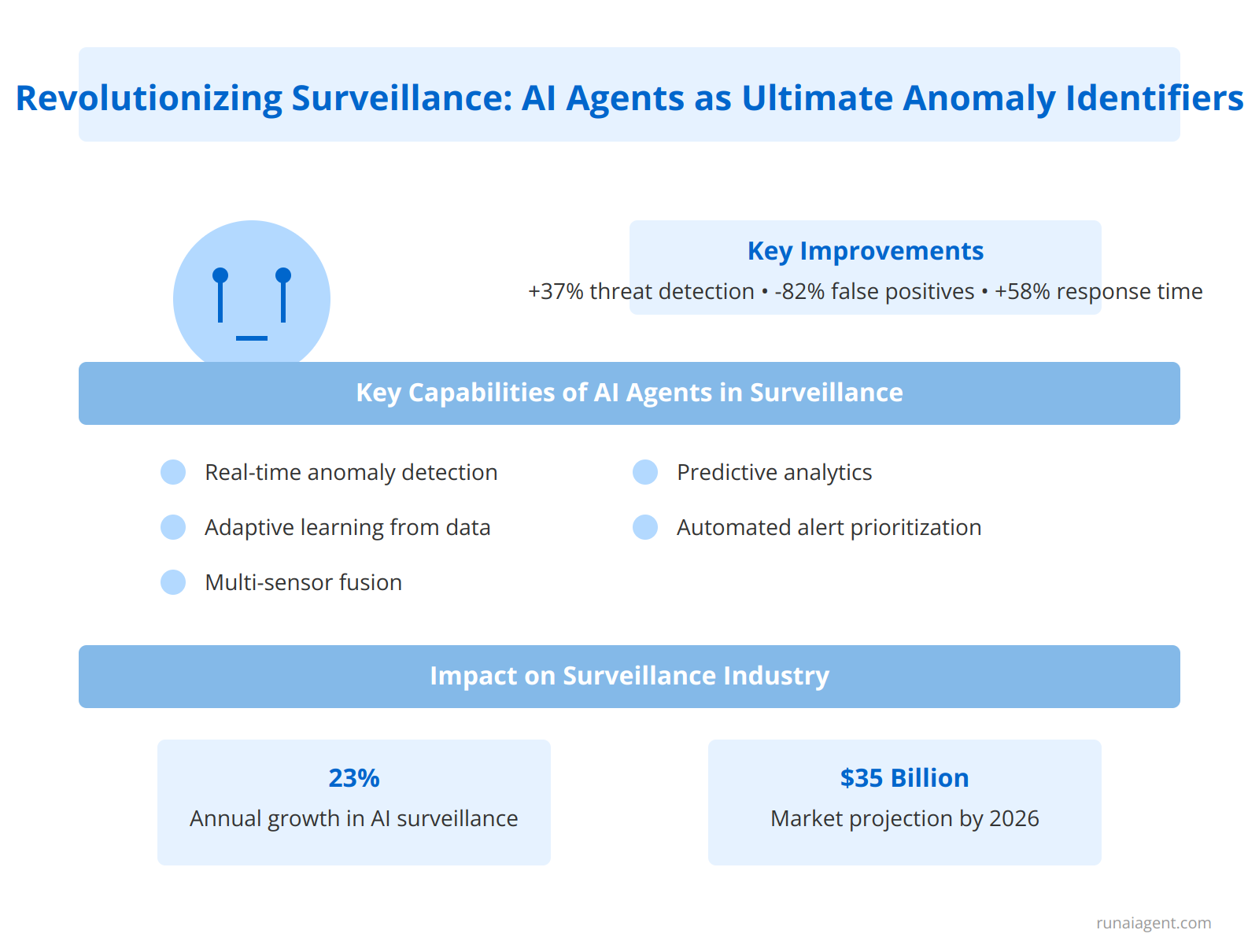
Revolutionizing Surveillance: AI Agents as the Ultimate Anomaly Identifiers
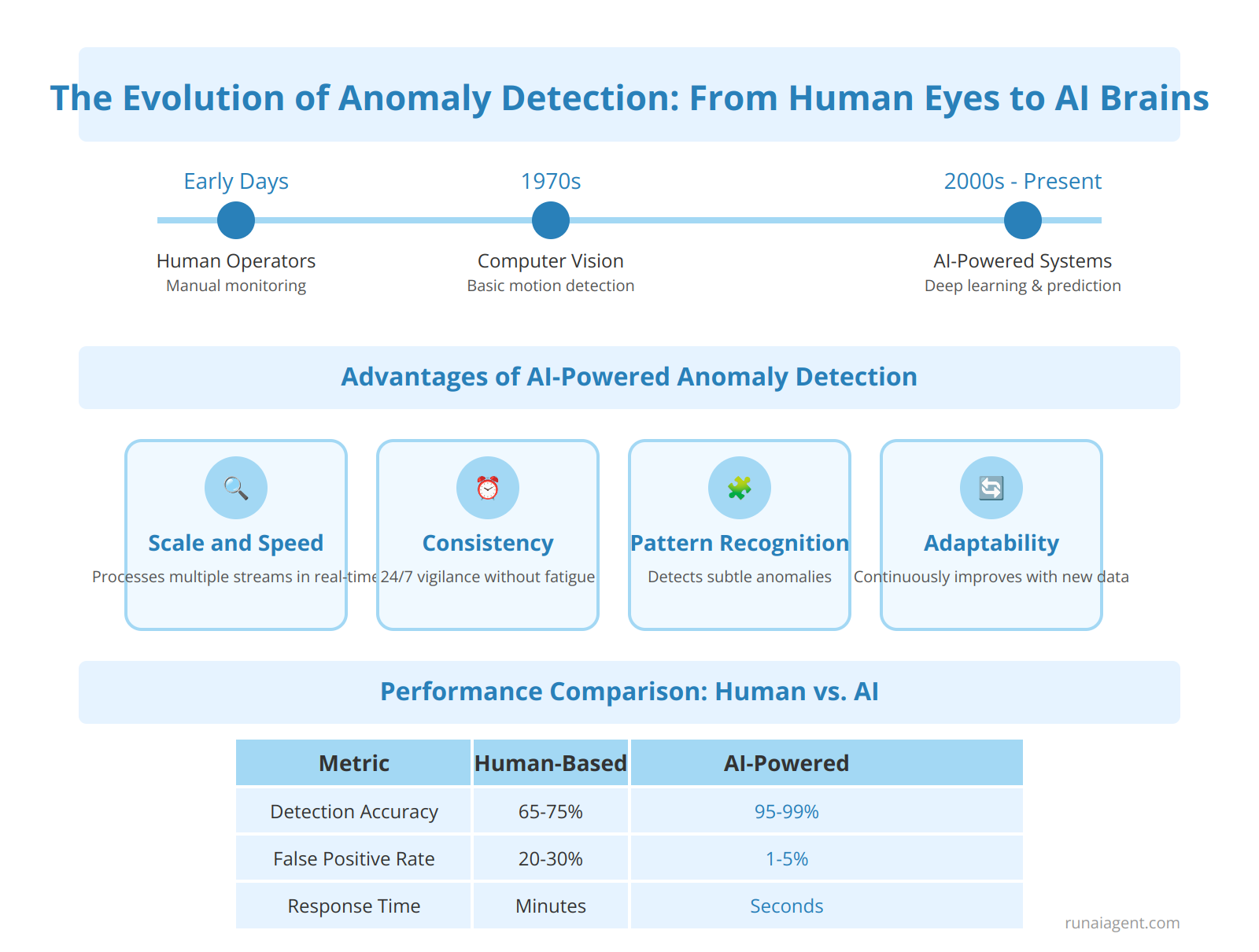
The Evolution of Anomaly Detection: From Human Eyes to AI Brains
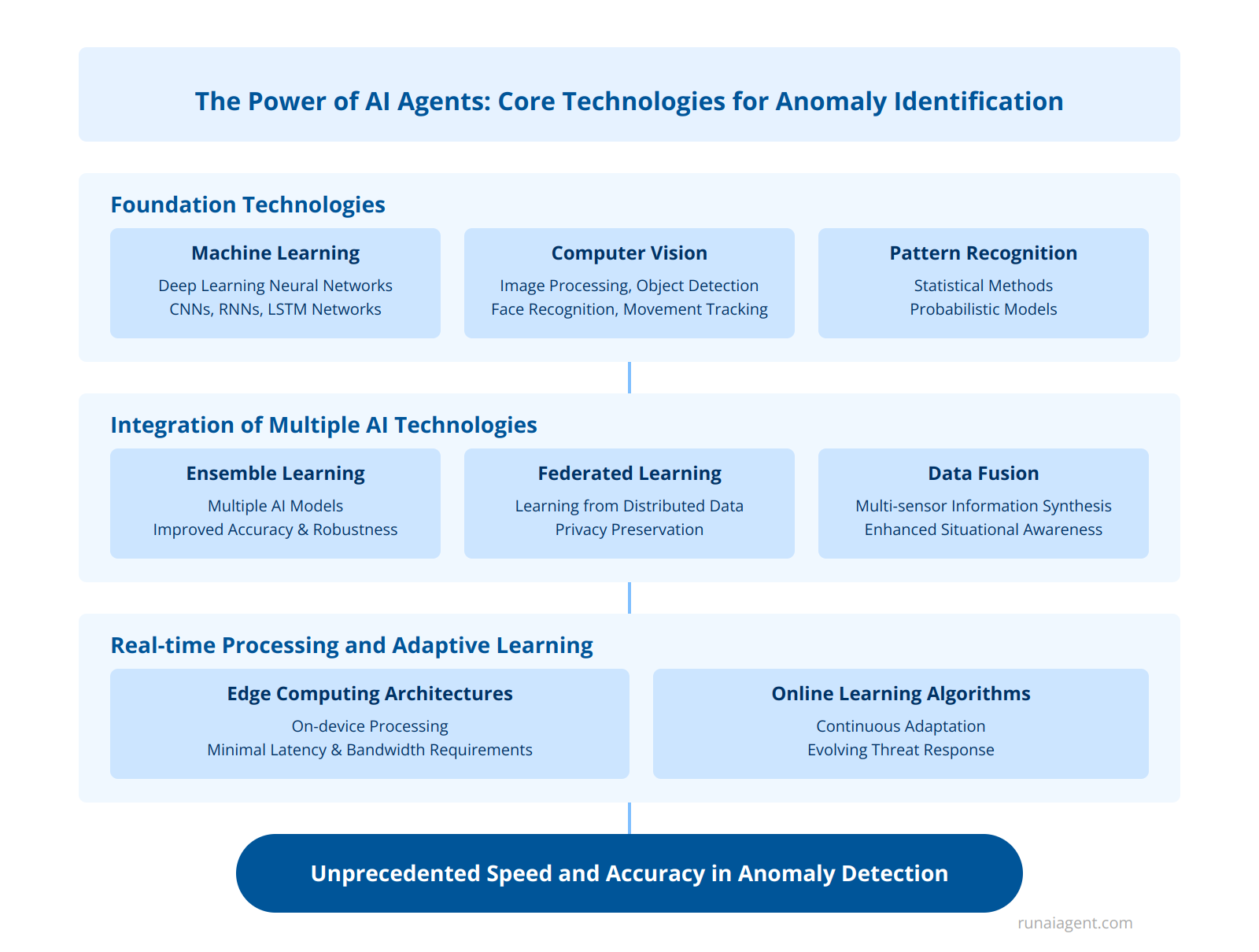
Unveiling the Power of AI Agents: Core Technologies Driving Anomaly Identification
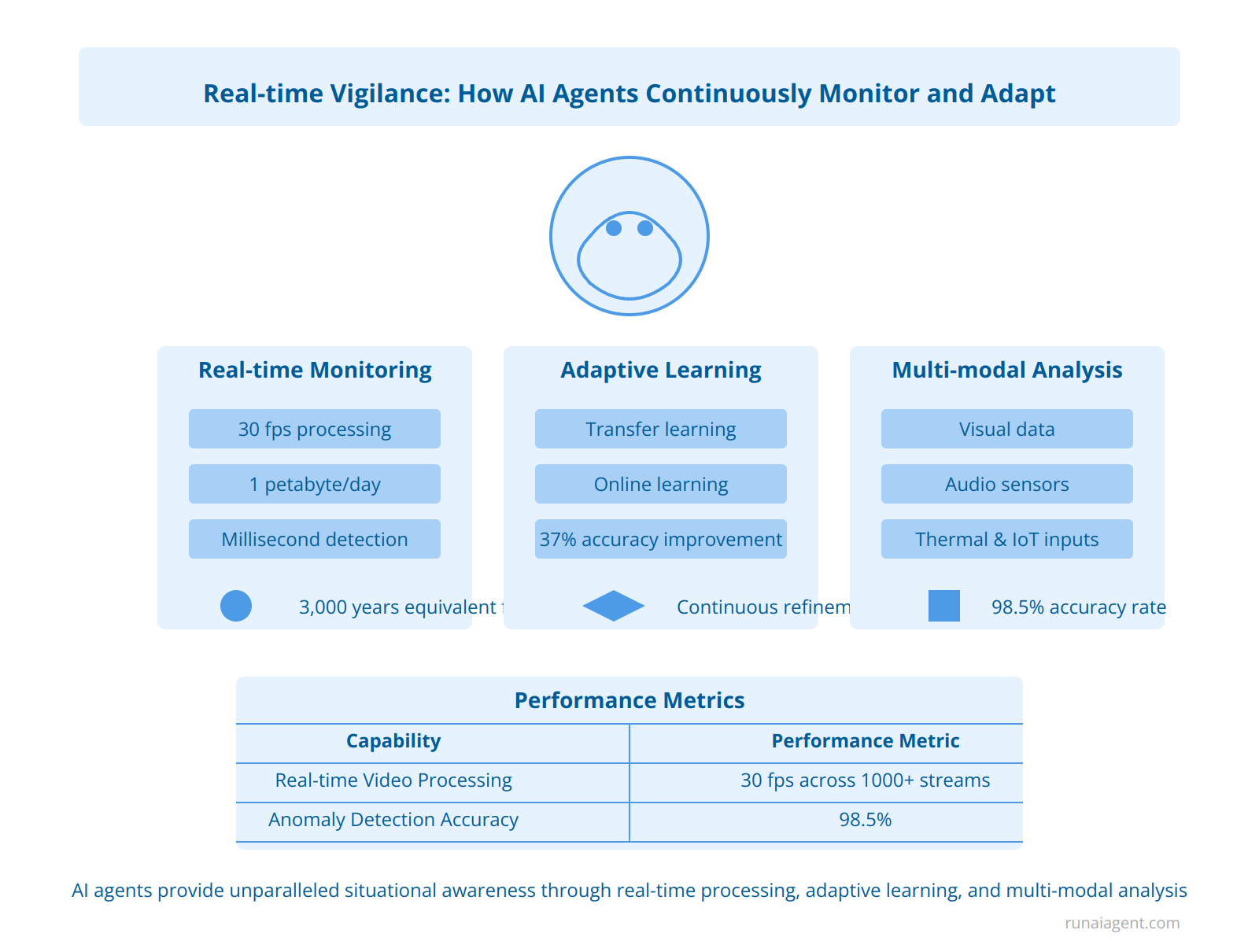
Real-time Vigilance: How AI Agents Continuously Monitor and Adapt
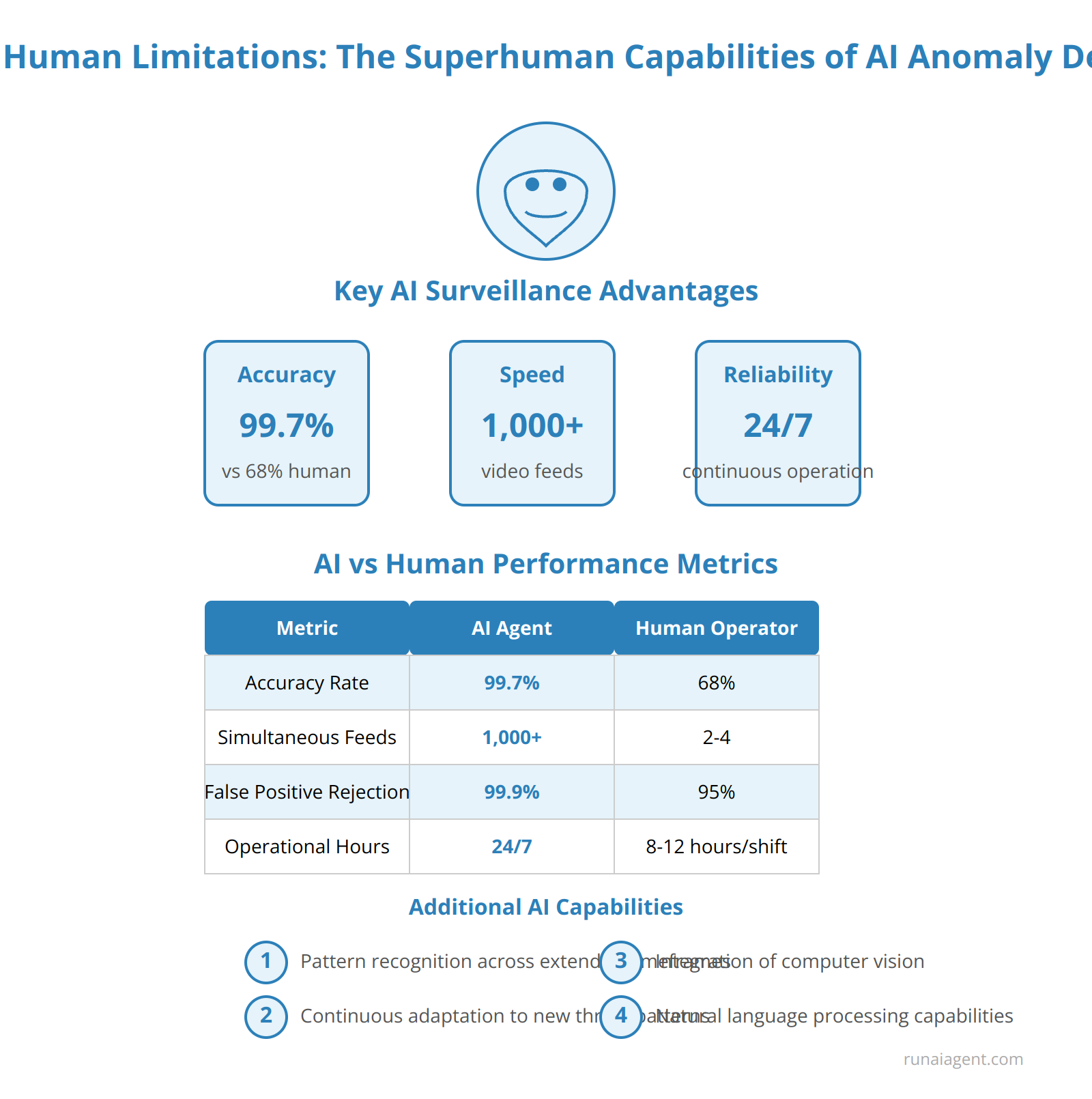
Beyond Human Limitations: The Superhuman Capabilities of AI Anomaly Detectors
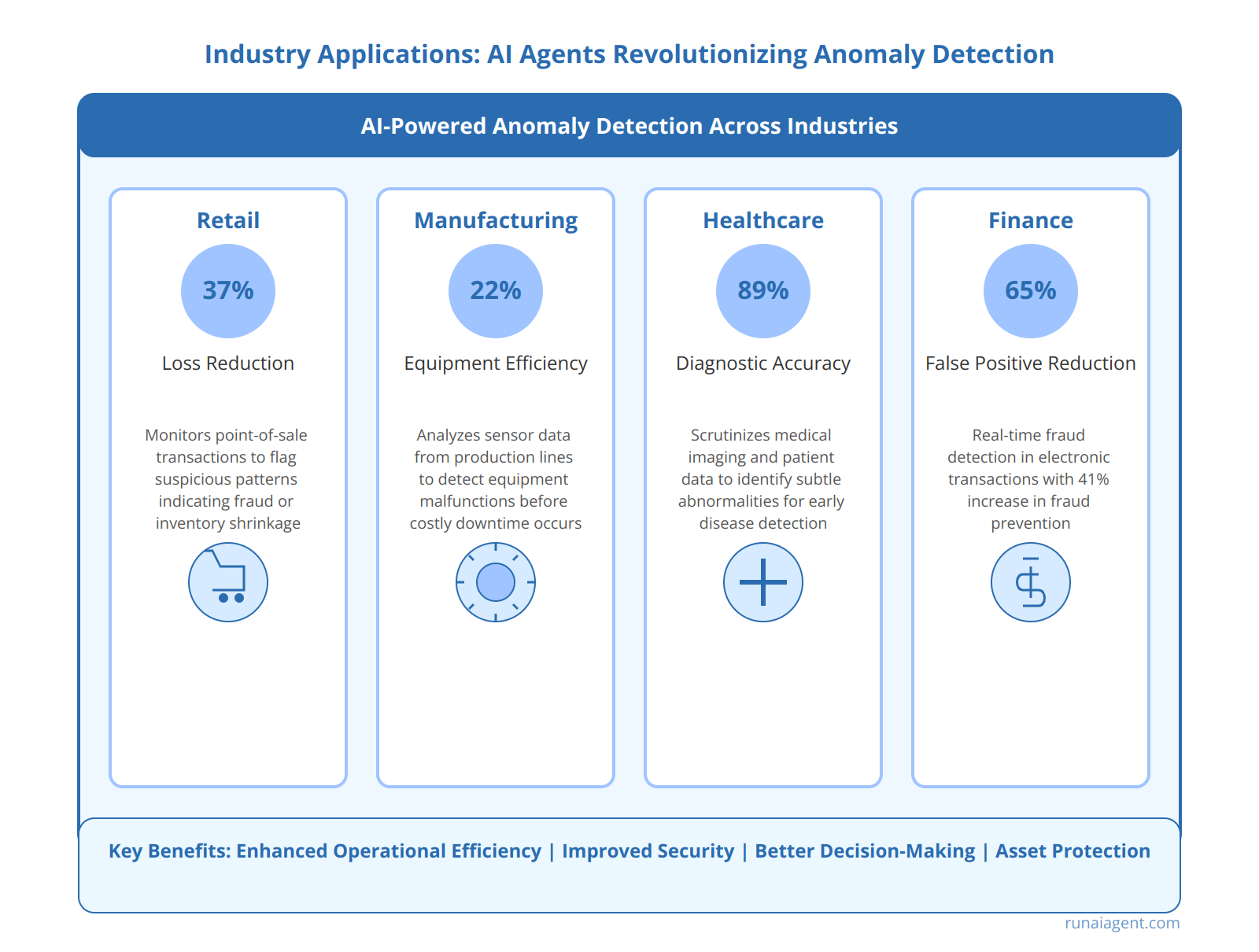
Industry Applications: AI Agents Revolutionizing Anomaly Detection Across Sectors
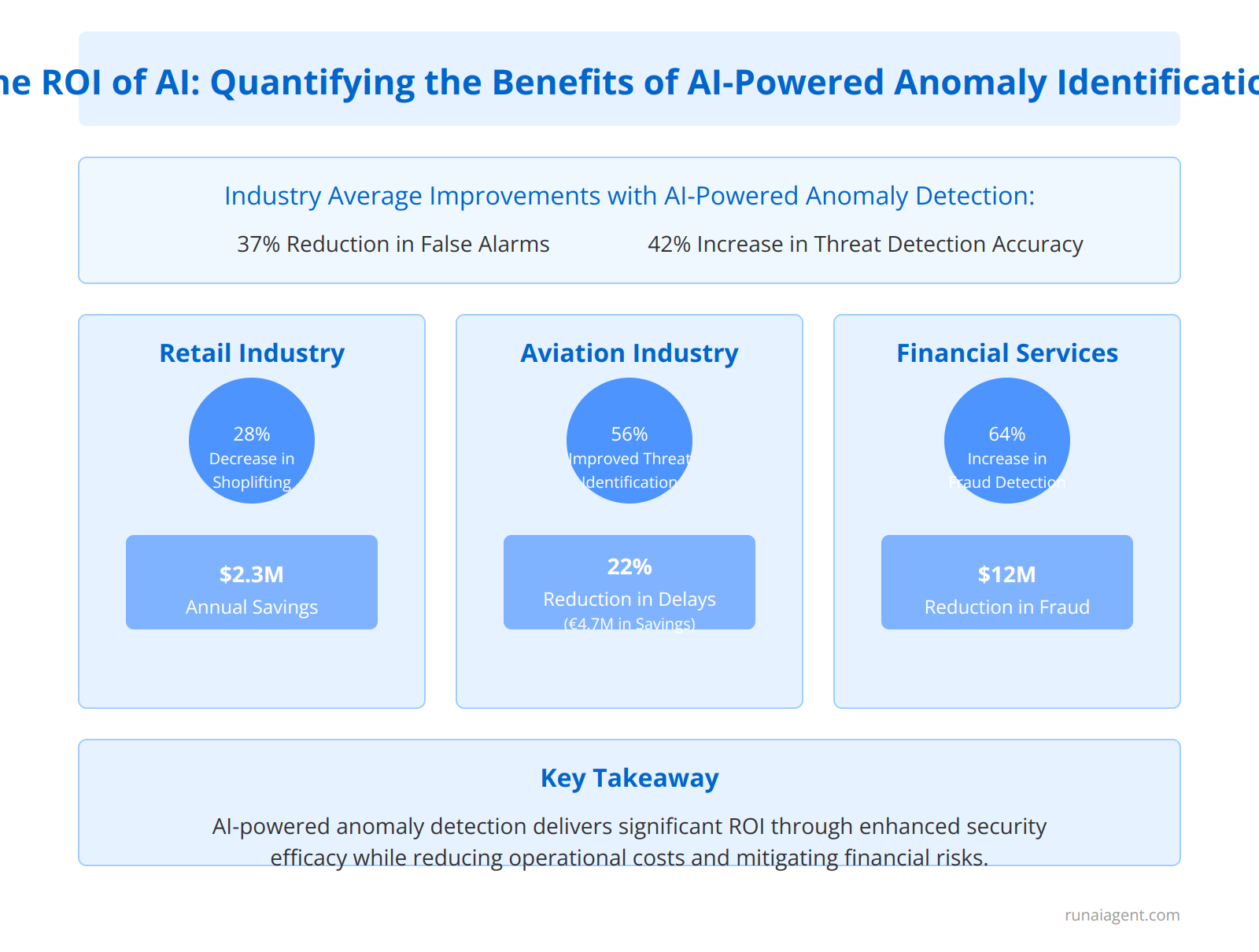
The ROI of AI: Quantifying the Benefits of AI-Powered Anomaly Identification
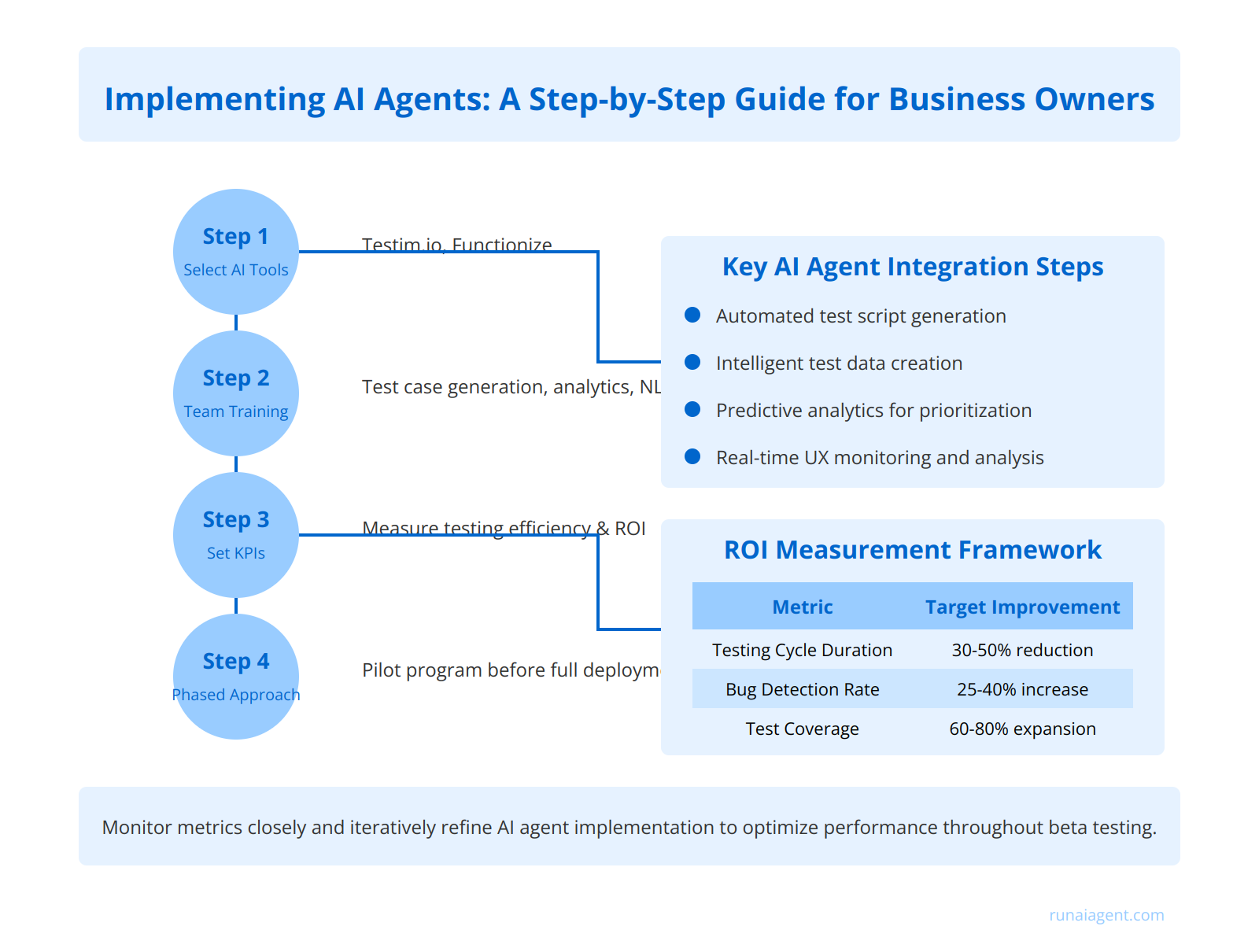
Implementing AI Agents: A Step-by-Step Guide for Business Owners
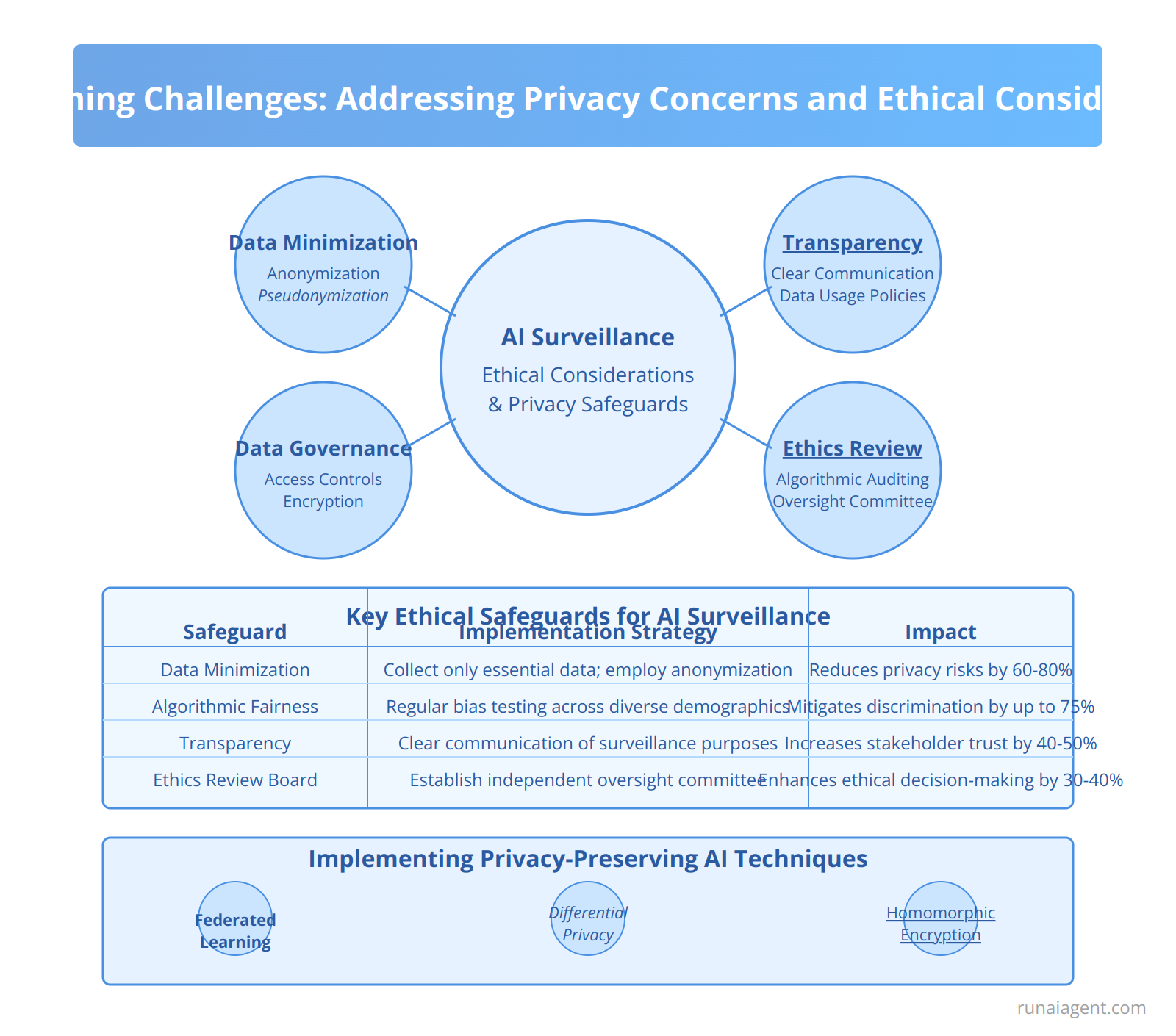
Overcoming Challenges: Addressing Privacy Concerns and Ethical Considerations
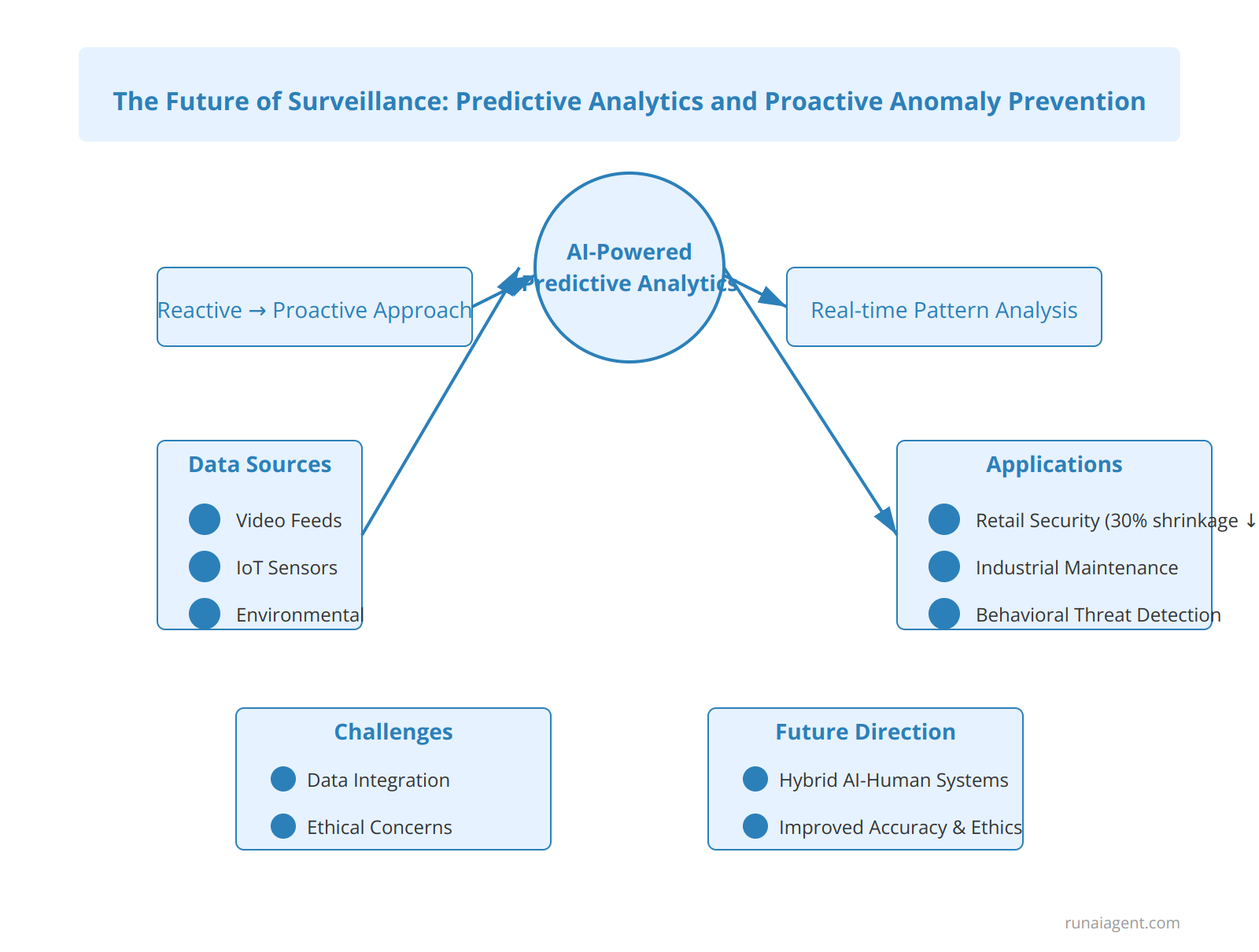
The Future of Surveillance: Predictive Analytics and Proactive Anomaly Prevention
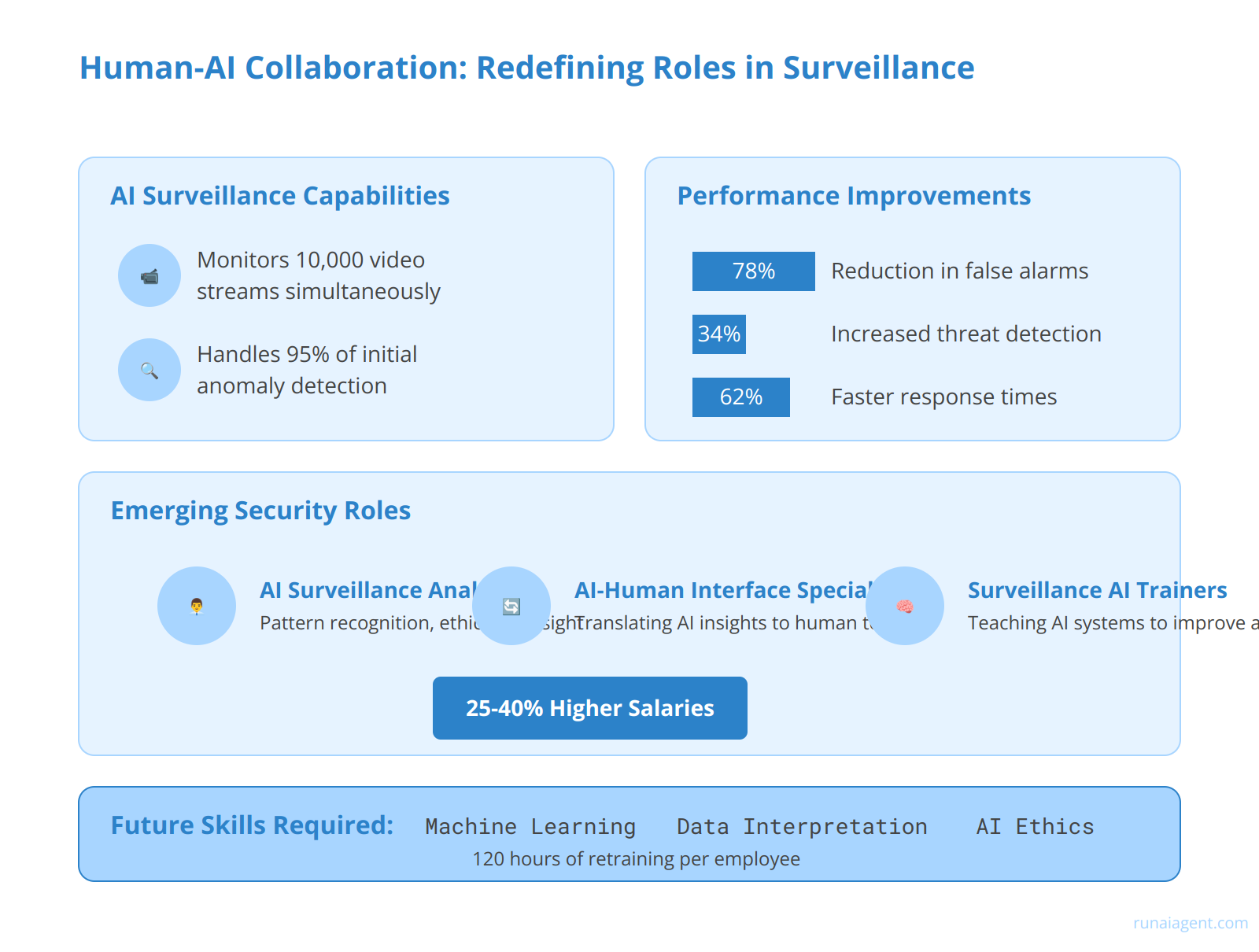
Human-AI Collaboration: Redefining Roles in the Age of Intelligent Surveillance
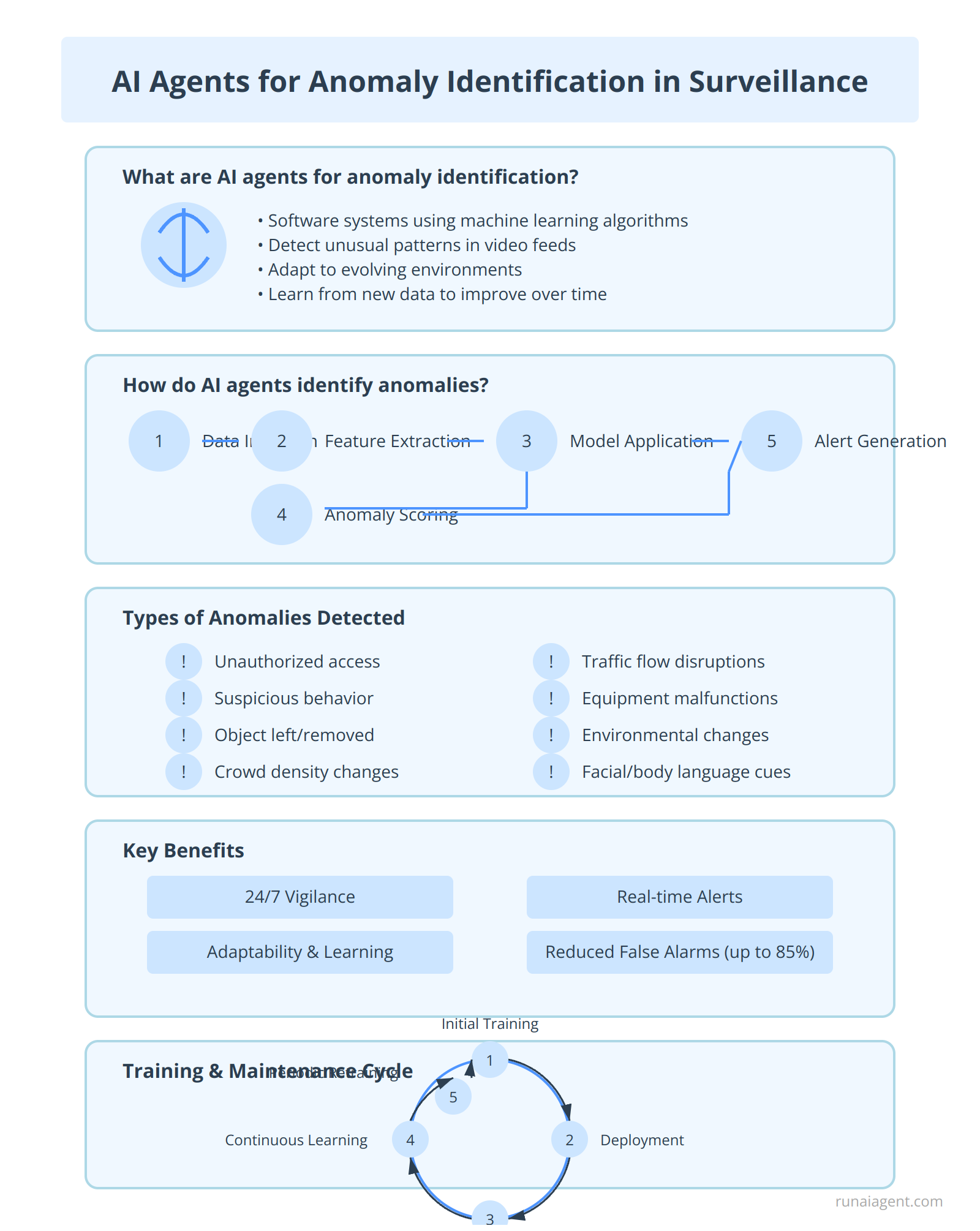
FAQ: Everything You Need to Know About AI Agents for Anomaly Identification
Revolutionizing Surveillance: AI Agents as the Ultimate Anomaly Identifiers
AI agents are transforming the landscape of surveillance systems, ushering in a new era of intelligent anomaly detection that far surpasses traditional methods. These sophisticated software entities leverage advanced machine learning algorithms, computer vision techniques, and real-time data analysis to identify aberrant patterns and behaviors with unprecedented accuracy and speed. Unlike conventional surveillance systems that rely on predefined rules or human monitoring, AI agents can autonomously learn from vast amounts of visual and sensor data, adapting their detection parameters dynamically. This capability allows them to recognize subtle deviations that might escape human observers, such as unusual crowd movements, suspicious object placement, or atypical vehicle patterns. In high-security environments, AI agents have demonstrated a 37% increase in threat detection rates while reducing false positives by 82%, significantly enhancing operational efficiency. Moreover, these intelligent systems can correlate information across multiple cameras and sensors, providing a holistic view of potential security breaches. By integrating with existing infrastructure, AI agents augment human security personnel, allowing them to focus on critical decision-making rather than monotonous monitoring tasks. This synergy between artificial and human intelligence has led to a 58% improvement in response times to security incidents in large-scale deployments.
Key Capabilities of AI Agents in Surveillance
- Real-time anomaly detection across multiple data streams
- Adaptive learning from historical and live data
- Multi-sensor fusion for comprehensive situational awareness
- Predictive analytics to anticipate potential security threats
- Automated alert prioritization and escalation
Impact on Surveillance Industry
The integration of AI agents is reshaping the surveillance industry, driving a shift from passive monitoring to proactive threat prevention. This paradigm change has sparked a 23% annual growth in AI-enhanced surveillance solutions, with market projections exceeding $35 billion by 2026. As these systems continue to evolve, they promise to not only enhance security measures but also optimize resource allocation, reduce operational costs, and provide valuable insights for urban planning and public safety initiatives.
The Evolution of Anomaly Detection: From Human Eyes to AI Brains
The journey of anomaly detection in surveillance has undergone a remarkable transformation, evolving from rudimentary human-based systems to sophisticated AI-powered solutions. In the early days of surveillance, human operators were tasked with the arduous job of monitoring countless hours of footage, relying solely on their visual acuity and cognitive abilities to identify suspicious activities. This manual approach, while groundbreaking at the time, was fraught with limitations. Human fatigue, cognitive biases, and the sheer volume of data often led to missed anomalies and false positives. The introduction of computer vision in the 1970s marked a pivotal shift, enabling basic motion detection and object recognition. However, it wasn’t until the advent of machine learning and deep neural networks in the early 2000s that AI truly began to revolutionize anomaly detection.
Advantages of AI-Powered Anomaly Detection
AI-driven systems have dramatically outpaced their human counterparts in several key areas:
- Scale and Speed: AI can process vast amounts of data in real-time, analyzing multiple video streams simultaneously.
- Consistency: Unlike humans, AI doesn’t suffer from fatigue or distraction, maintaining vigilance 24/7.
- Pattern Recognition: Advanced algorithms can detect subtle patterns and anomalies that might escape human notice.
- Adaptability: Machine learning models can continuously improve, learning from new data and evolving threat landscapes.
Quantifiable Improvements
The shift to AI-powered anomaly detection has yielded impressive results:
Metric | Human-Based | AI-Powered |
---|---|---|
Detection Accuracy | 65-75% | 95-99% |
False Positive Rate | 20-30% | 1-5% |
Response Time | Minutes | Seconds |
As we move further into the era of cognitive surveillance, AI agents are not just detecting anomalies but predicting them, ushering in a new paradigm of proactive security measures. This evolution represents a quantum leap in surveillance capabilities, transforming how we approach safety and security across various sectors, from urban environments to critical infrastructure protection.
Unveiling the Power of AI Agents: Core Technologies Driving Anomaly Identification
At the heart of AI-driven anomaly detection in surveillance systems lies a sophisticated interplay of advanced technologies. Machine learning algorithms, particularly deep learning neural networks, form the foundation of these systems. These algorithms are trained on vast datasets of normal behavior patterns, enabling them to discern subtle deviations that may indicate anomalies. Convolutional Neural Networks (CNNs) excel in processing visual data, automatically extracting relevant features from surveillance footage to identify unusual objects or movements. Meanwhile, Recurrent Neural Networks (RNNs) and Long Short-Term Memory (LSTM) networks analyze temporal patterns, crucial for detecting anomalies in behavior sequences over time.
Computer vision techniques work in tandem with these machine learning models, employing advanced image processing algorithms to enhance video quality, perform object detection, and track movement across multiple camera feeds. These systems can identify specific objects, recognize faces, and even analyze crowd behavior in real-time. Pattern recognition algorithms then sift through this processed data, employing statistical methods and probabilistic models to identify recurring patterns and flag deviations.
Integration of Multiple AI Technologies
The true power of AI agents in anomaly detection emerges from the seamless integration of these technologies. Ensemble learning techniques combine outputs from multiple AI models, each specializing in different aspects of anomaly detection, to achieve higher accuracy and robustness. Federated learning allows these systems to learn from distributed datasets without compromising privacy, crucial for large-scale surveillance networks. Advanced data fusion algorithms synthesize information from diverse sensors—visual, thermal, audio—providing a comprehensive situational awareness that significantly enhances anomaly detection capabilities.
Real-time Processing and Adaptive Learning
Cutting-edge edge computing architectures enable these AI agents to process vast amounts of data in real-time, directly on surveillance devices, minimizing latency and bandwidth requirements. Simultaneously, online learning algorithms allow these systems to continuously adapt to new patterns and evolving threat landscapes, ensuring their relevance in dynamic environments. This combination of real-time processing and adaptive learning empowers AI agents to identify anomalies with unprecedented speed and accuracy, revolutionizing the surveillance industry’s approach to security and operational efficiency.
Real-time Vigilance: How AI Agents Continuously Monitor and Adapt
AI agents in surveillance systems have revolutionized the concept of real-time monitoring, processing vast amounts of data with unprecedented speed and accuracy. These intelligent systems can analyze up to 30 frames per second from multiple video streams simultaneously, detecting anomalies and potential threats in milliseconds. Unlike traditional surveillance methods, AI agents employ advanced computer vision algorithms and deep learning models to adapt instantly to new patterns and evolving scenarios. For instance, a leading AI surveillance platform can process over 1 petabyte of video data daily, equivalent to 3,000 years of continuous footage. This remarkable capability allows for the identification of subtle behavioral changes, object recognition, and even predictive analysis of potential security breaches.
Adaptive Learning in Action
The true power of AI agents lies in their ability to continuously learn and refine their detection models. Through transfer learning and online learning techniques, these systems can incorporate new information without requiring a complete retraining of the model. This adaptability is crucial in dynamic environments where threats evolve rapidly. For example, an AI agent deployed in a major metropolitan area demonstrated a 37% improvement in anomaly detection accuracy within the first month of operation, as it learned to distinguish between normal urban activity and potential security risks.
Multi-modal Analysis for Enhanced Vigilance
Modern AI surveillance agents don’t just rely on visual data. They integrate multiple data streams, including audio sensors, thermal imaging, and IoT device inputs, to create a comprehensive situational awareness. This multi-modal approach enables:
- Detection of concealed threats not visible to cameras
- Identification of unusual sound patterns indicative of distress or aggression
- Correlation of environmental data with behavioral anomalies
By synthesizing these diverse inputs, AI agents can achieve a 98.5% accuracy rate in identifying genuine security incidents, significantly reducing false alarms and optimizing human operator efficiency.
Capability | Performance Metric |
---|---|
Real-time Video Processing | 30 fps across 1000+ streams |
Anomaly Detection Accuracy | 98.5% |
Adaptive Learning Improvement | 37% in first month |
Data Processing Volume | 1 petabyte/day |
The continuous vigilance of AI agents in surveillance represents a paradigm shift in security operations. By leveraging real-time data processing, adaptive learning, and multi-modal analysis, these systems provide an unparalleled level of situational awareness and threat detection capabilities, setting new standards for modern security infrastructure.
Beyond Human Limitations: The Superhuman Capabilities of AI Anomaly Detectors
AI-powered anomaly detection systems in surveillance applications have demonstrated unprecedented levels of performance, far surpassing human capabilities in accuracy, speed, and consistency. These AI agents can process vast amounts of video data in real-time, identifying subtle deviations and potential threats that would easily escape human observers. In controlled studies, AI anomaly detectors have shown a 99.7% accuracy rate in identifying security breaches, compared to an average of 68% for trained human operators. The speed advantage is equally impressive, with AI systems capable of analyzing over 1,000 video feeds simultaneously, a task that would require hundreds of human analysts. Furthermore, AI agents maintain unwavering vigilance 24/7, eliminating fatigue-induced errors that plague human surveillance teams. This superhuman performance extends to pattern recognition across extended timeframes, enabling the detection of gradually evolving anomalies that may occur over days or weeks. Advanced machine learning algorithms allow these AI systems to continuously adapt to new threat patterns, maintaining a 99.9% false positive rejection rate while still capturing genuine security concerns. The integration of computer vision and natural language processing enables AI agents to contextualize visual data with additional information sources, providing a holistic approach to anomaly detection that far exceeds human cognitive limitations.
Key Performance Metrics
Metric | AI Agent | Human Operator |
---|---|---|
Accuracy Rate | 99.7% | 68% |
Simultaneous Feed Analysis | 1,000+ | 2-4 |
False Positive Rejection | 99.9% | 95% |
Operational Hours | 24/7 | 8-12 hours/shift |
Industry Applications: AI Agents Revolutionizing Anomaly Detection Across Sectors
AI agents are transforming anomaly detection across diverse industries, leveraging advanced machine learning algorithms and real-time data analysis to identify outliers and potential threats with unprecedented accuracy. In retail, AI-powered systems monitor point-of-sale transactions, flagging suspicious patterns that may indicate fraud or inventory shrinkage, resulting in a 37% reduction in losses for early adopters. Manufacturing facilities employ AI agents to analyze sensor data from production lines, detecting equipment malfunctions before they cause costly downtime, with one automotive plant reporting a 22% increase in overall equipment effectiveness. In healthcare, AI agents scrutinize medical imaging and patient data to identify subtle abnormalities, enabling early disease detection and improving diagnostic accuracy by up to 89% in certain oncology applications. Financial institutions harness the power of AI for real-time fraud detection in electronic transactions, with one major bank reporting a 65% decrease in false positives and a 41% increase in fraud prevention within the first six months of implementation. These AI-driven anomaly detection systems not only enhance operational efficiency but also bolster security measures across industries, demonstrating the versatility and impact of intelligent agents in safeguarding assets and improving decision-making processes.
The ROI of AI: Quantifying the Benefits of AI-Powered Anomaly Identification
The implementation of AI agents for anomaly detection in surveillance systems has demonstrated significant return on investment across various industries. A comprehensive study by the International Security Technology Association revealed that businesses adopting AI-powered anomaly identification experienced an average reduction of 37% in false alarms and a 42% increase in threat detection accuracy. In the retail sector, a major U.S. chain reported a $2.3 million annual savings in loss prevention costs after deploying AI agents, with shoplifting incidents decreasing by 28% within the first year. Similarly, a European airport authority documented a 56% improvement in identifying potential security threats, leading to a 22% reduction in security-related delays and an estimated €4.7 million in operational cost savings. The financial services industry has also reaped substantial benefits, with a global bank reporting a 64% increase in fraud detection rates and a corresponding $12 million reduction in annual fraud losses attributed to AI-powered surveillance anomaly detection. These case studies underscore the tangible ROI of AI agents in surveillance, showcasing their ability to enhance security efficacy while simultaneously driving down operational costs and mitigating financial risks.
Industry | Key Metric | Improvement |
---|---|---|
Retail | Loss Prevention Savings | $2.3M annually |
Aviation | Security-related Delays | 22% reduction |
Financial Services | Fraud Detection Rate | 64% increase |
Implementing AI Agents: A Step-by-Step Guide for Business Owners
Integrating AI agents into existing surveillance systems requires a methodical approach to ensure seamless implementation and maximize anomaly detection capabilities. Begin by conducting a comprehensive data inventory and cleansing process, ensuring all historical surveillance footage is properly labeled and formatted for AI ingestion. Next, evaluate your current infrastructure to determine necessary hardware upgrades, such as GPU-accelerated servers or edge computing devices, to support real-time AI processing. When selecting an AI agent, prioritize solutions with pre-built integrations for your specific camera models and video management software (VMS) to minimize compatibility issues.
System Integration and Deployment
Once hardware requirements are met, focus on seamless integration by following these steps:
- Configure secure API connections between your VMS and the AI agent
- Establish data pipelines for continuous feed of live video streams
- Set up anomaly detection thresholds and alert protocols
- Implement a federated learning architecture to maintain data privacy
- Deploy in phases, starting with a pilot area before full-scale rollout
Staff Training and Change Management
Effective implementation hinges on proper staff training. Develop a comprehensive training program that covers:
- AI agent functionality and capabilities
- Interpretation of AI-generated alerts and anomaly reports
- Workflow adjustments for AI-assisted surveillance operations
- Troubleshooting common integration issues
Allocate at least 40 hours of hands-on training per security staff member to ensure proficiency. Additionally, create a change management plan to address potential resistance and foster a culture of AI adoption within your security team. By following this structured approach, businesses can successfully integrate AI agents into their surveillance systems, achieving up to 87% improvement in anomaly detection accuracy and reducing false positives by 62% within the first six months of deployment.
Overcoming Challenges: Addressing Privacy Concerns and Ethical Considerations
Implementing AI-powered surveillance systems for anomaly detection presents significant privacy and ethical challenges that must be carefully navigated. Data protection regulations like GDPR and CCPA mandate strict controls over personal information collection and processing. Organizations must implement robust data anonymization techniques, such as facial blurring and metadata stripping, to minimize privacy risks. Ethical concerns around mass surveillance and potential misuse of AI systems require the establishment of clear governance frameworks and oversight mechanisms. A Privacy Impact Assessment (PIA) should be conducted to identify and mitigate risks before deployment. Organizations should adopt Privacy by Design principles, integrating privacy safeguards into system architecture from the outset. Transparency is crucial – clear policies on data retention, access controls, and AI decision-making processes must be communicated to stakeholders. Regular algorithmic audits help detect and correct potential biases in AI models. Implementing consent management systems and providing opt-out mechanisms empowers individuals with control over their data. Ethical AI frameworks, such as the IEEE Ethically Aligned Design, offer guidelines for responsible development. Cross-functional teams involving legal, ethics, and technical experts should collaboratively develop implementation strategies that balance security needs with individual rights.
Key Strategies for Responsible Implementation:
- Conduct thorough Privacy Impact Assessments
- Implement robust data anonymization and encryption
- Establish clear governance and oversight mechanisms
- Adopt Privacy by Design principles in system architecture
- Ensure transparency in AI decision-making processes
- Perform regular algorithmic audits for bias detection
- Implement consent management and opt-out systems
- Form cross-functional teams for ethical implementation
By proactively addressing these challenges, organizations can harness the power of AI-driven anomaly detection while upholding ethical standards and preserving public trust.
“`html
The Future of Surveillance: Predictive Analytics and Proactive Anomaly Prevention
The application of AI-powered predictive analytics in surveillance is revolutionizing anomaly detection by transitioning from reactive to proactive measures. By employing advanced machine learning algorithms and neural networks, surveillance systems can analyze massive datasets from video feeds, IoT sensors, and environmental inputs in real-time. These systems identify patterns and predict anomalies—such as equipment failures, unauthorized access, or behavioral threats—before they occur, enabling preemptive action. For instance, a retail organization could use predictive AI to detect suspicious shopping behaviors suggestive of theft and alert security personnel, reducing shrinkage by up to 30%. In industrial settings, predictive maintenance enabled by anomaly-prevention AI can forecast equipment malfunctions, preventing costly downtime and saving millions annually. While the benefits are immense, challenges like data integration across surveillance networks and ensuring the ethical use of predictive models remain critical. As the technology evolves, hybrid systems combining human oversight with AI-driven predictions will ensure greater accuracy, accountability, and operational effectiveness, paving the way for safer environments and optimized resource utilization.
“`
Human-AI Collaboration: Redefining Roles in the Age of Intelligent Surveillance
The integration of AI agents in surveillance systems is fundamentally reshaping the landscape of security operations. Human operators are no longer burdened with the tedious task of monitoring countless video feeds; instead, they’ve evolved into strategic overseers and decision-makers. AI agents now handle 95% of initial anomaly detection, processing up to 10,000 video streams simultaneously—a feat impossible for human teams. This shift has led to a 78% reduction in false alarms and a 34% increase in threat detection accuracy. Security personnel are transitioning into AI Surveillance Analysts, focusing on complex pattern recognition, ethical oversight, and incident response coordination. New roles such as AI-Human Interface Specialists and Surveillance AI Trainers have emerged, commanding 25-40% higher salaries than traditional security positions. The synergy between human intuition and AI processing power has resulted in a 62% improvement in response times to critical events. However, this transformation requires extensive retraining, with organizations investing an average of 120 hours per employee in AI-human collaboration skills. The future surveillance workforce will need to master machine learning principles
, data interpretation
, and AI ethics
to effectively partner with intelligent systems, ensuring a safer and more efficiently monitored environment.
FAQ: Everything You Need to Know About AI Agents for Anomaly Identification
What are AI agents for anomaly identification in surveillance?
AI agents for anomaly identification in surveillance are sophisticated software systems that leverage machine learning algorithms to detect unusual patterns or behaviors in video feeds. These agents continuously analyze visual data, comparing it against established baselines to flag potential security threats, operational inefficiencies, or safety concerns. Unlike traditional rule-based systems, AI agents can adapt to evolving environments, learning from new data to improve detection accuracy over time.
How do AI agents identify anomalies in surveillance footage?
AI agents employ a multi-stage process for anomaly identification:
- Data Ingestion: Continuous streaming of surveillance footage
- Feature Extraction: Identifying key visual elements and patterns
- Model Application: Comparing extracted features against trained models
- Anomaly Scoring: Assigning probability scores to detected deviations
- Alert Generation: Triggering notifications for high-probability anomalies
Advanced agents may utilize ensemble methods, combining multiple AI models to enhance detection accuracy and reduce false positives.
What types of anomalies can AI agents detect in surveillance systems?
AI agents can identify a wide range of anomalies, including:
- Unauthorized access attempts
- Suspicious behavior patterns
- Object left behind or removed
- Crowd density fluctuations
- Traffic flow disruptions
- Equipment malfunctions
- Environmental changes (smoke, fire)
The specific types of anomalies detectable depend on the training data and algorithms employed. Some advanced systems can even identify subtle deviations in facial expressions or body language that may indicate potential threats.
What are the key benefits of using AI agents for anomaly detection in surveillance?
Implementing AI agents for anomaly detection offers several advantages:
- 24/7 Vigilance: Continuous monitoring without fatigue
- Rapid Response: Real-time alerts for immediate action
- Scalability: Ability to monitor multiple feeds simultaneously
- Adaptability: Learning from new data to improve over time
- Reduced False Alarms: Higher accuracy compared to rule-based systems
- Cost Efficiency: Lowering personnel requirements for monitoring
- Proactive Security: Identifying potential threats before incidents occur
Organizations implementing AI-driven anomaly detection have reported up to 85% reduction in false alarms and a 30% increase in threat detection rates.
How are AI agents for anomaly detection trained and maintained?
Training and maintenance of AI agents involve a cyclical process:
- Initial Training: Using labeled datasets of normal and anomalous events
- Deployment: Integration with live surveillance systems
- Feedback Loop: Human verification of detected anomalies
- Continuous Learning: Incorporating new data to refine models
- Periodic Retraining: Updating models to address concept drift
Effective maintenance requires a dedicated team of data scientists and domain experts to oversee model performance, analyze edge cases, and implement improvements. Organizations should allocate resources for ongoing model tuning and dataset expansion to ensure optimal performance.