Table of Contents

The Rise of AI Agents: Revolutionizing App Support Specialist Roles
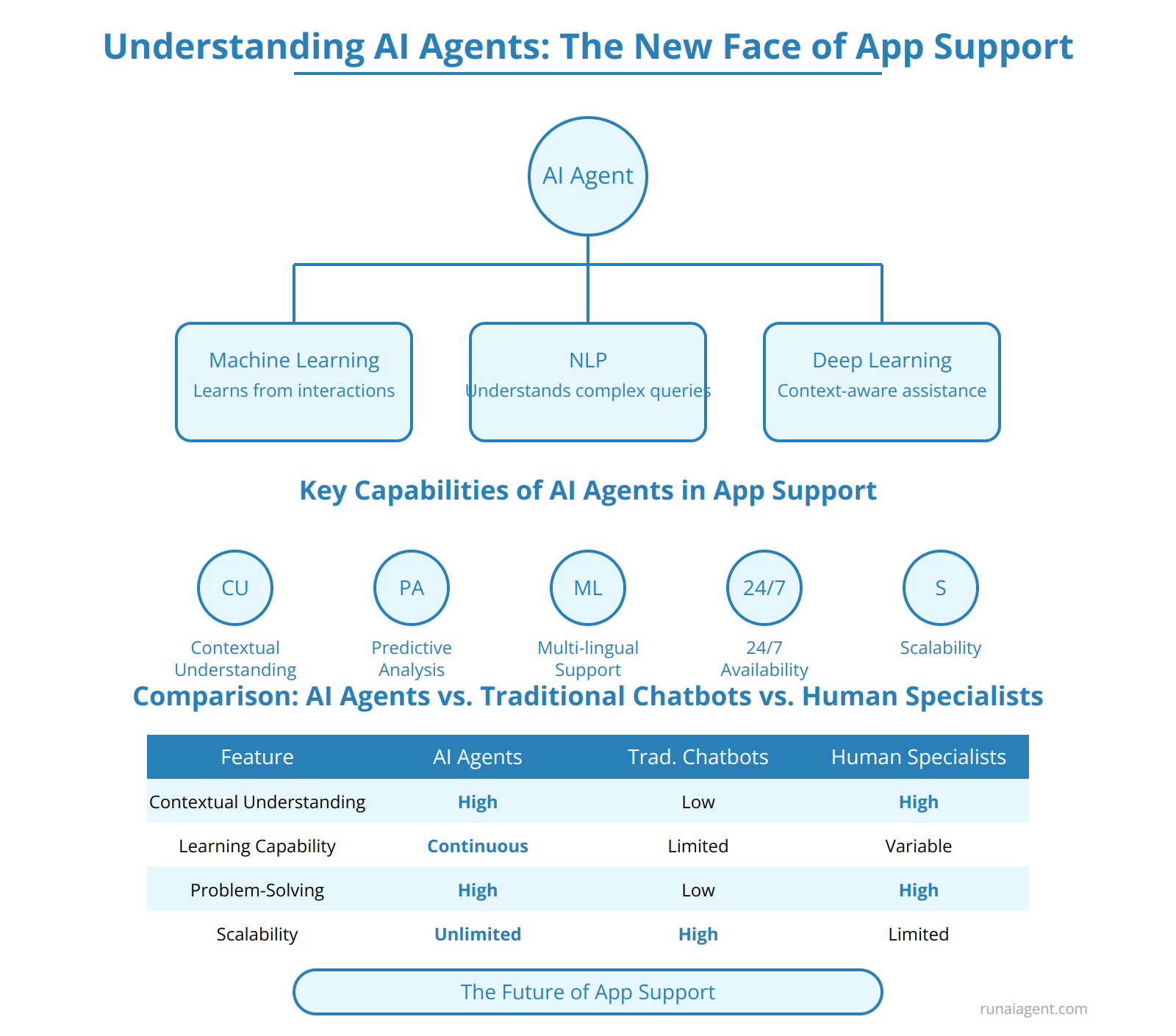
Understanding AI Agents: The New Face of App Support
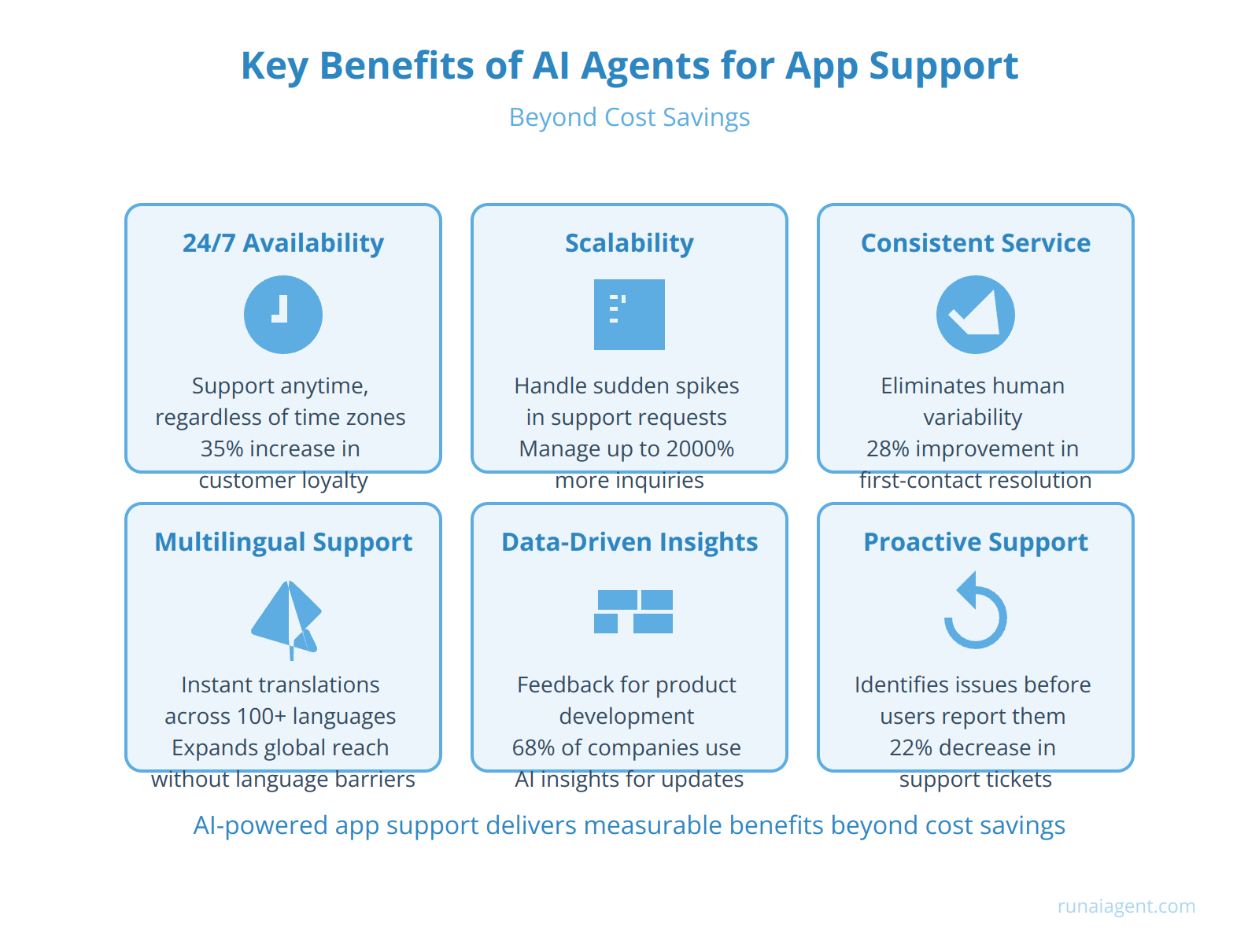
Key Benefits of AI Agents for App Support: Beyond Cost Savings
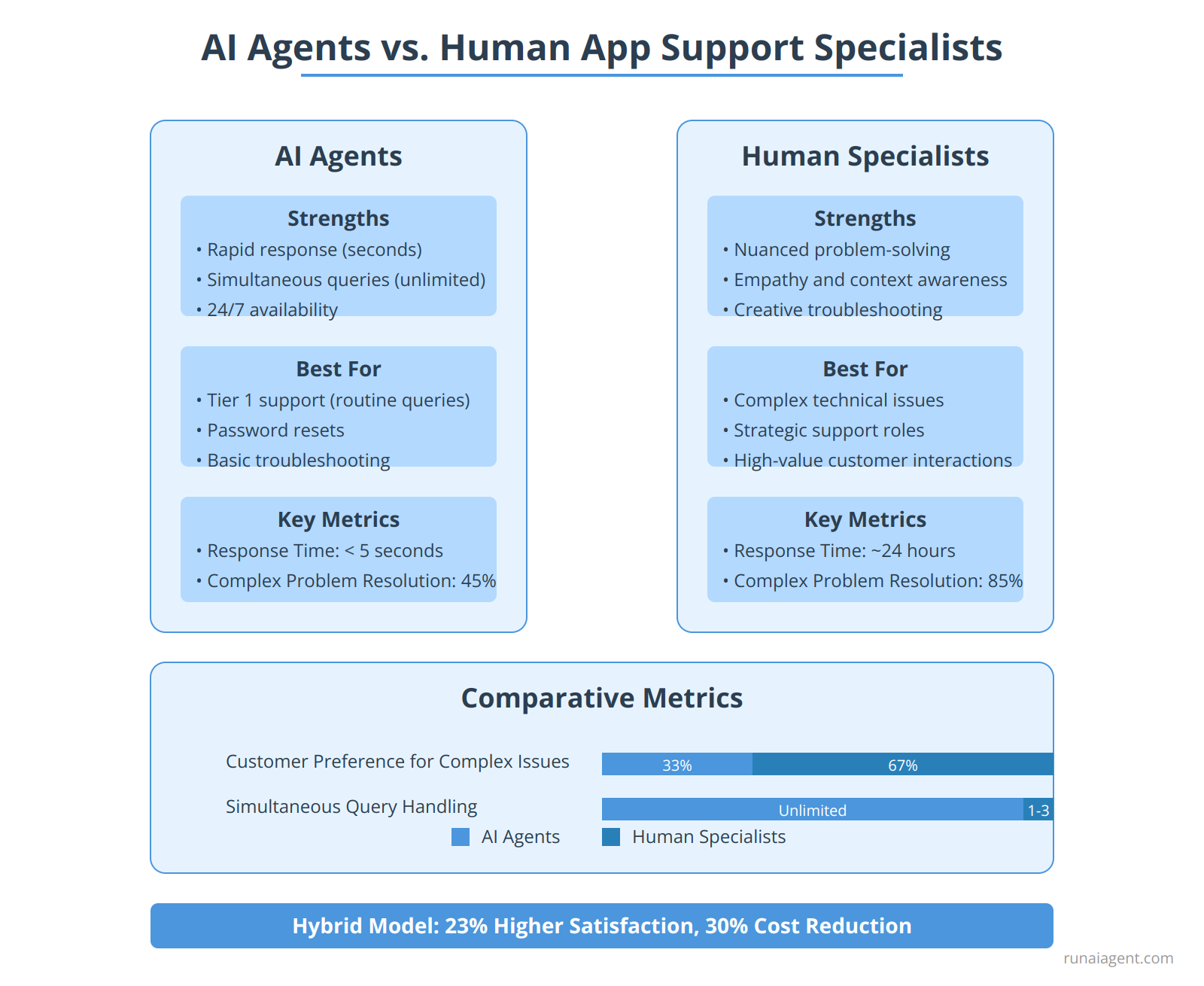
AI Agents vs. Human App Support Specialists: A Comparative Analysis
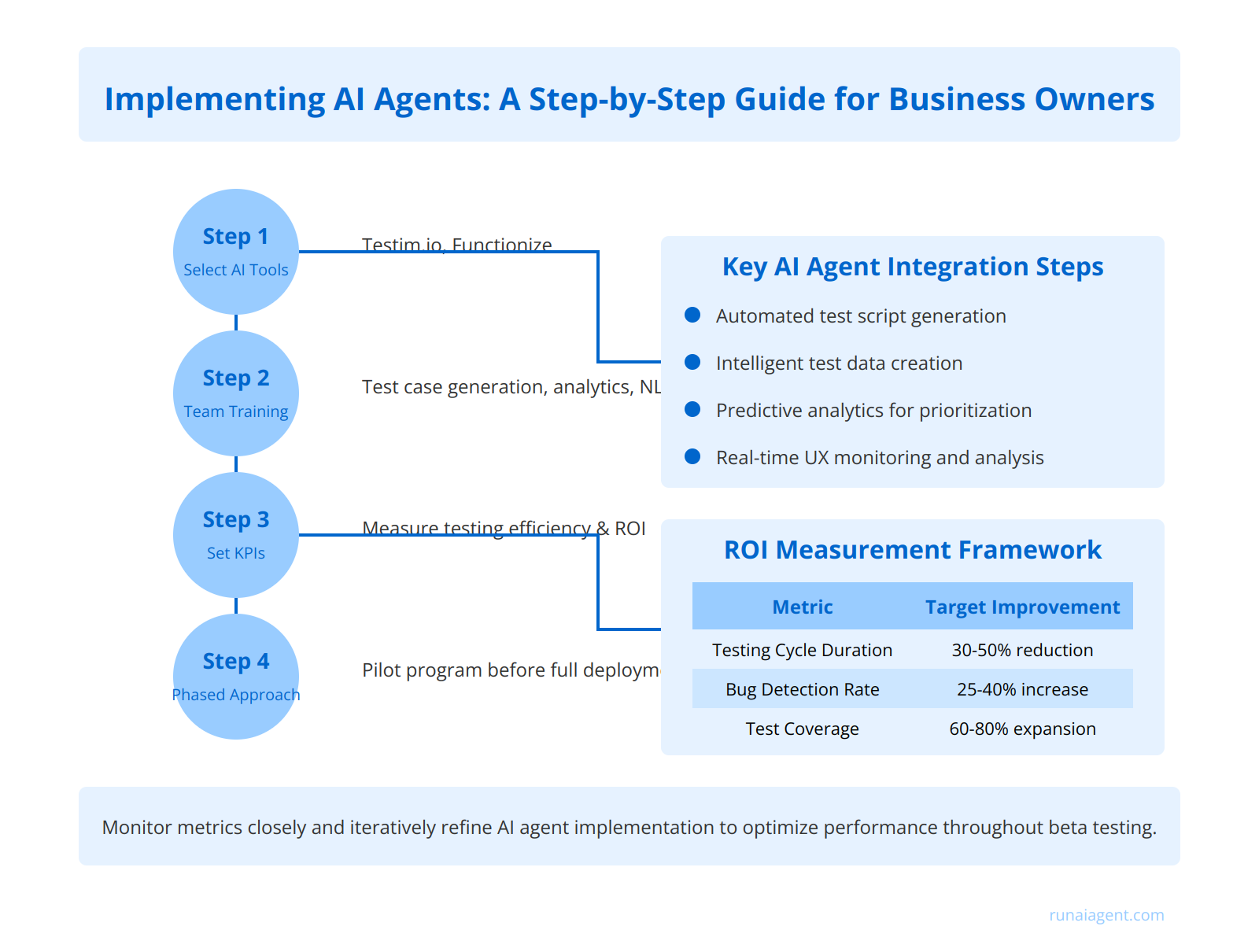
Implementing AI Agents: A Step-by-Step Guide for Business Owners
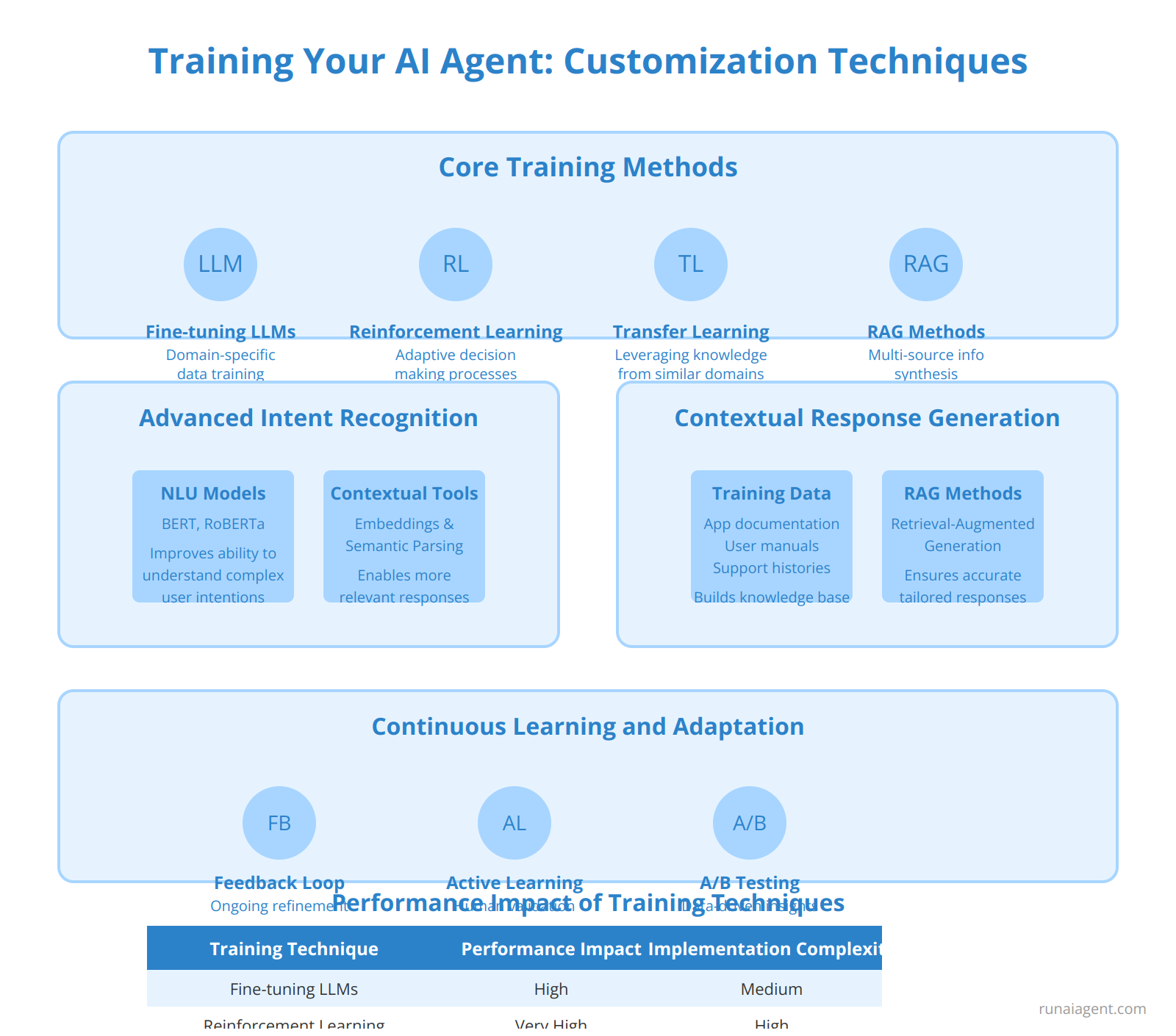
Training Your AI Agent: Customization Techniques for Optimal Performance
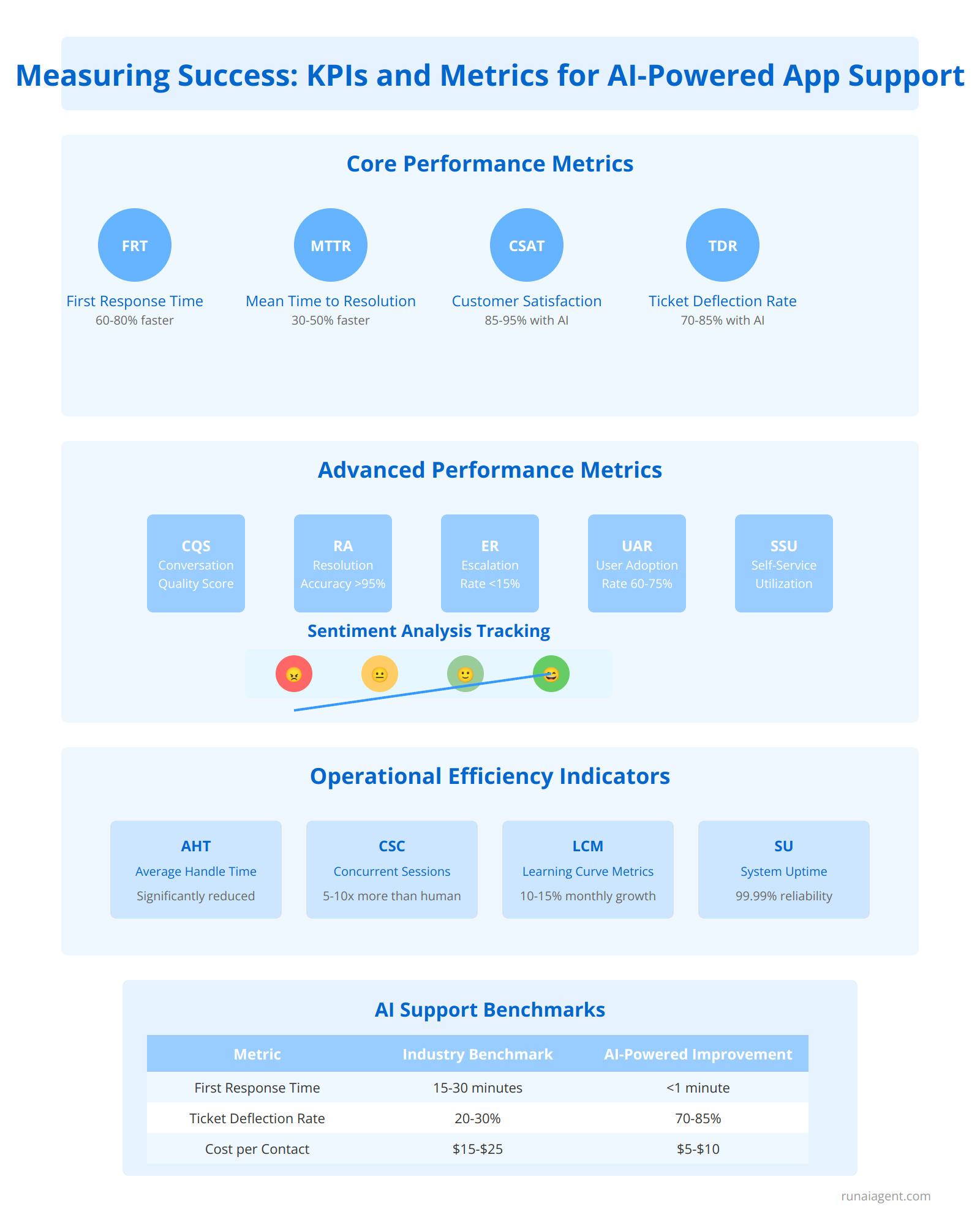
Measuring Success: KPIs and Metrics for AI-Powered App Support
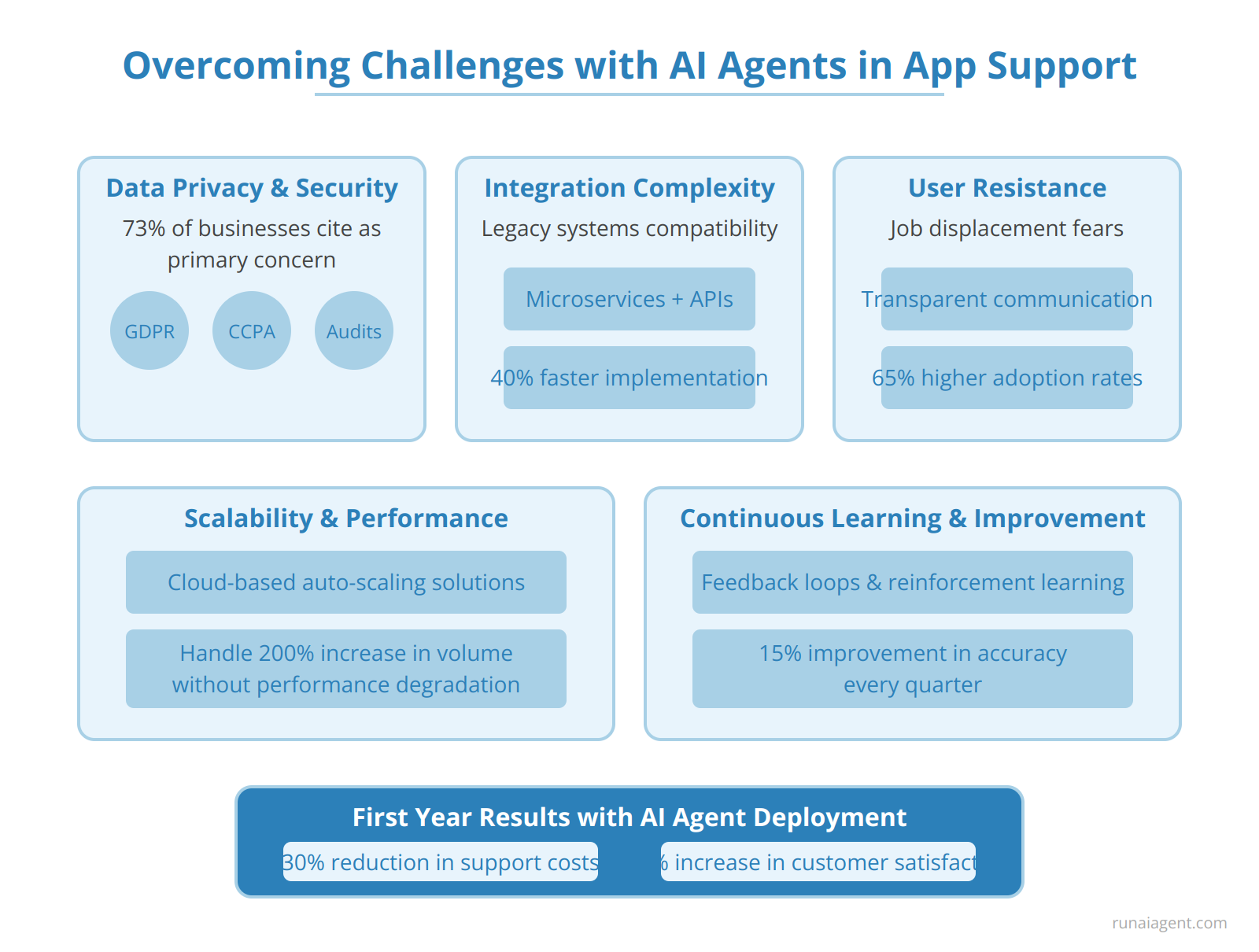
Overcoming Challenges: Addressing Common Concerns with AI Agents in App Support
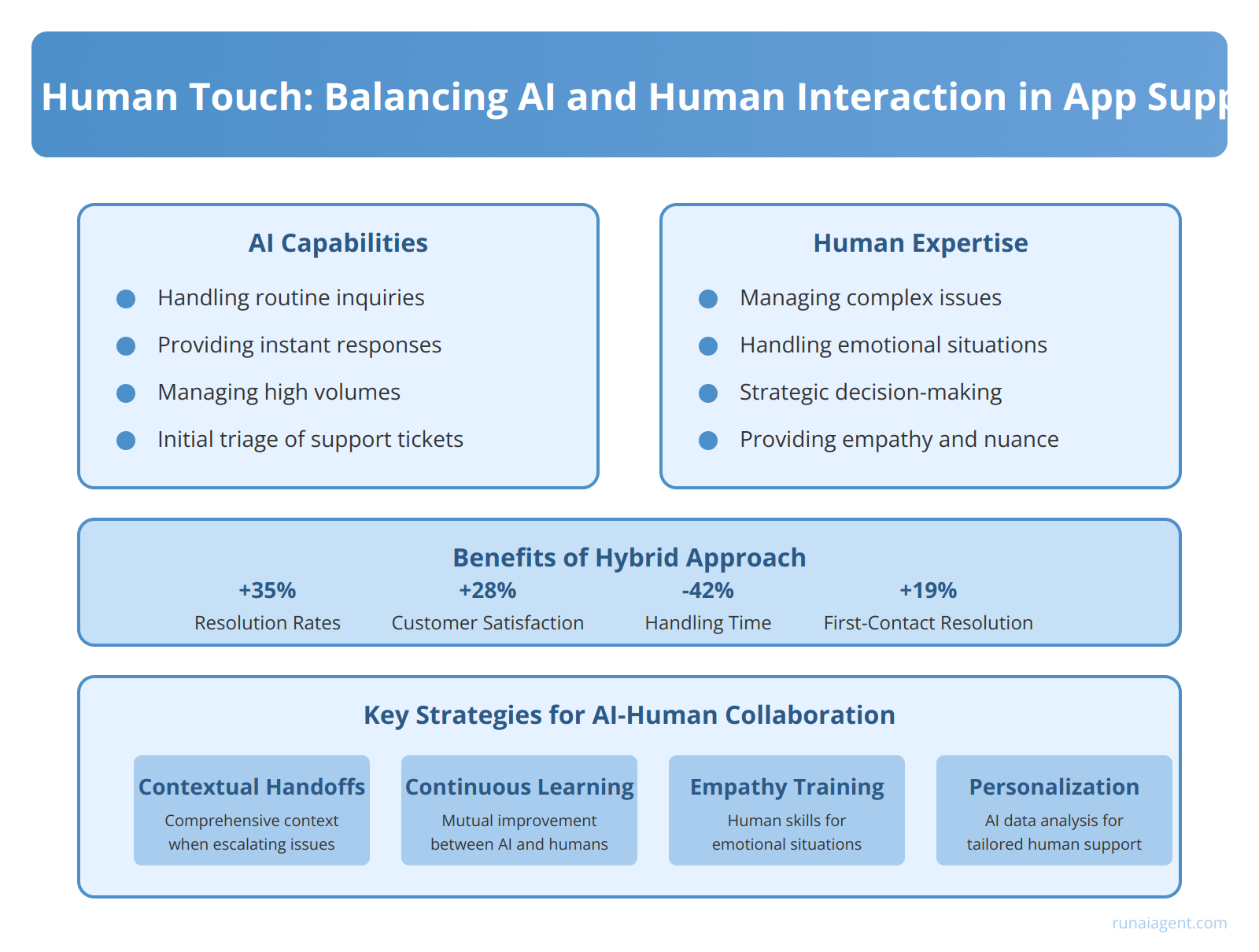
The Human Touch: Balancing AI and Human Interaction in App Support
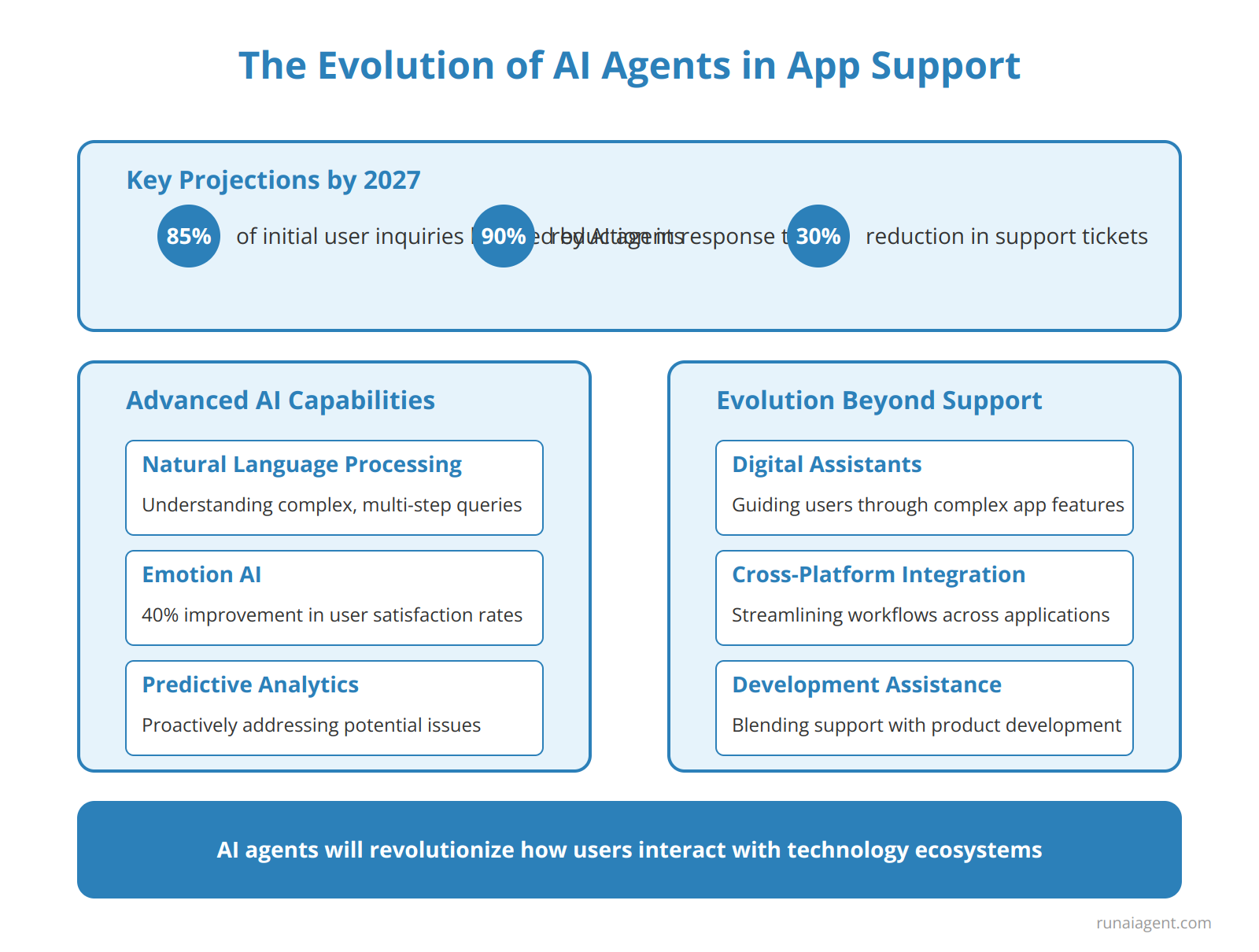
Future Trends: The Evolution of AI Agents in App Support and Beyond
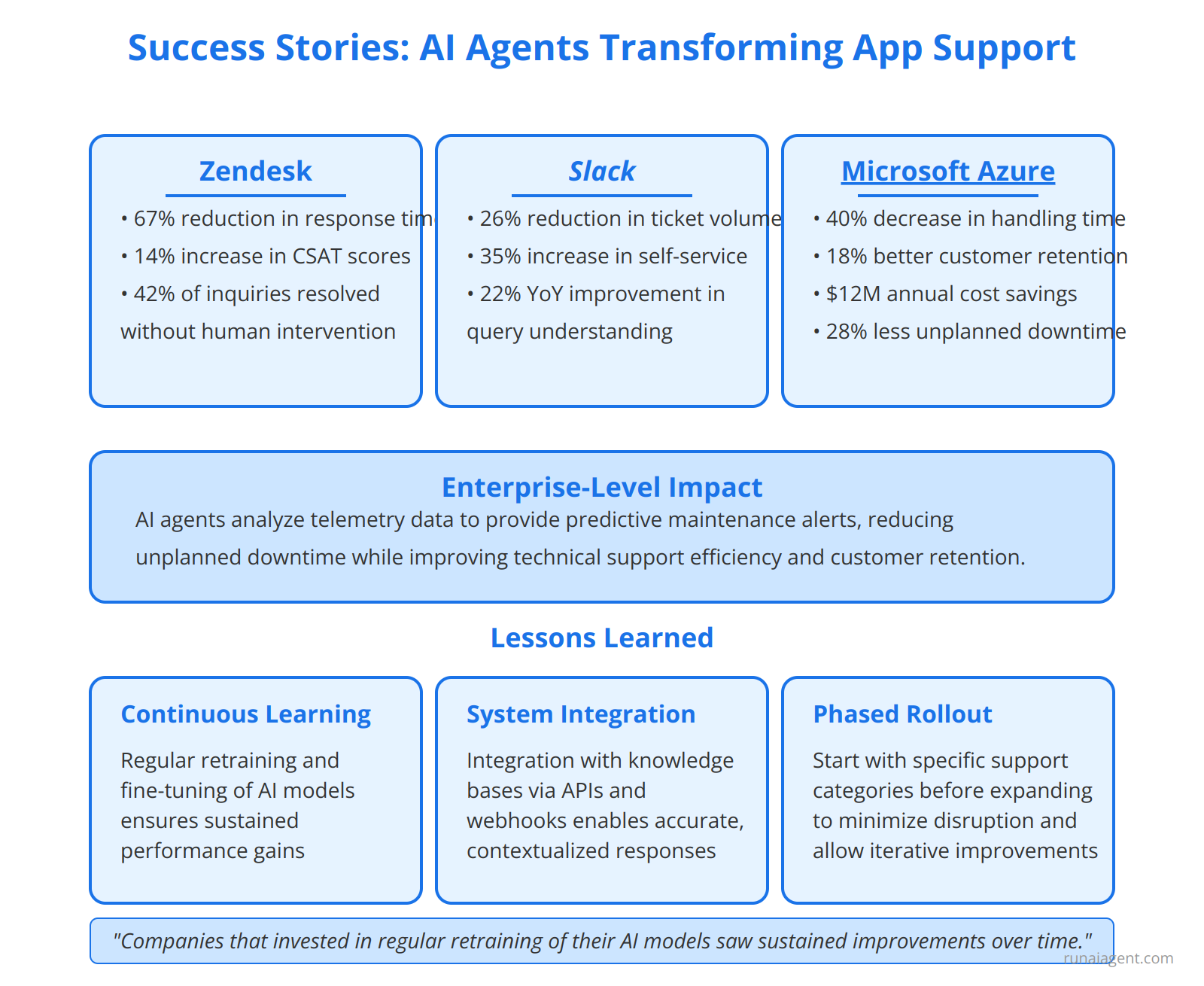
Case Studies: Success Stories of AI Agents Transforming App Support
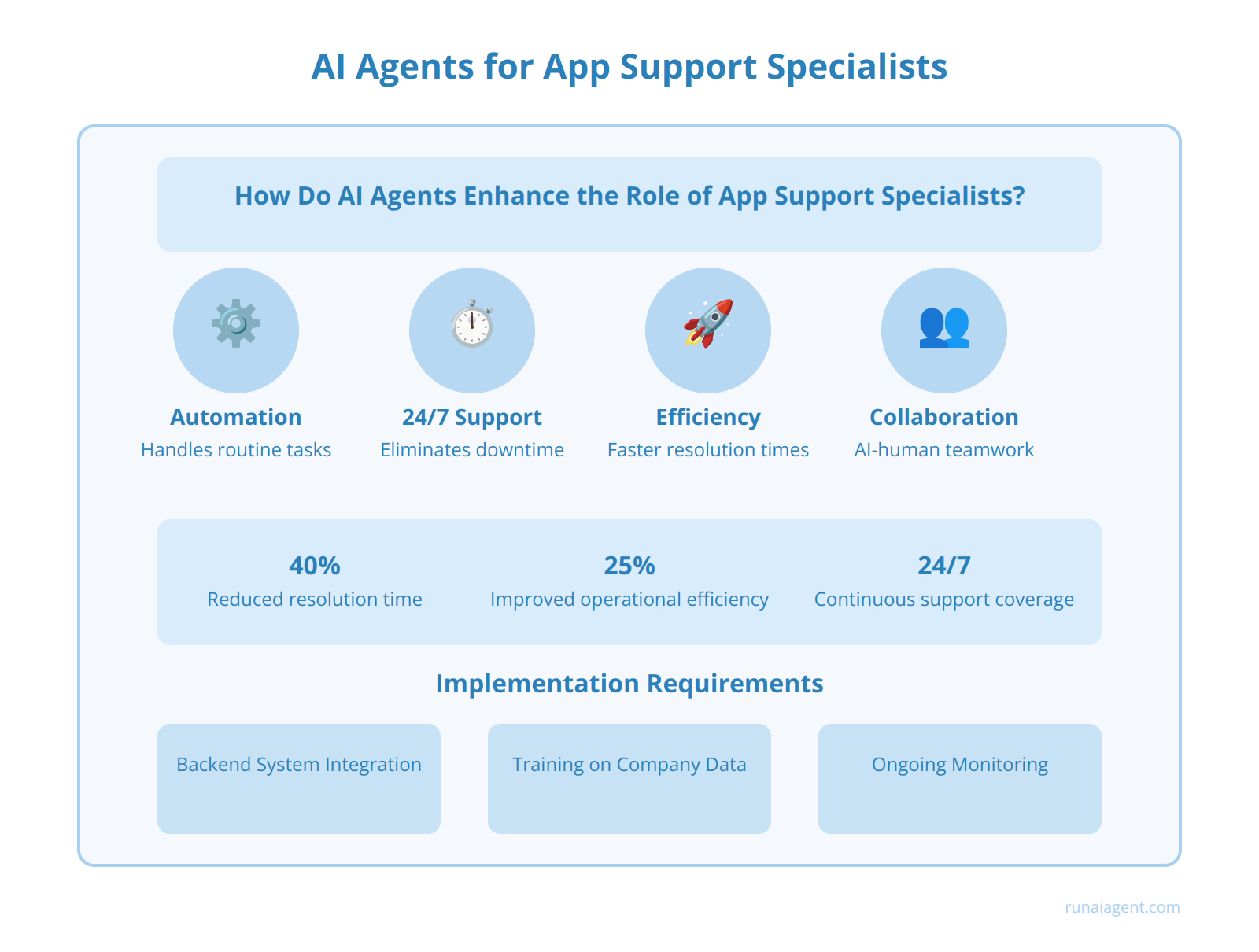
FAQ: Everything You Need to Know About AI Agents for App Support Specialists
The Rise of AI Agents: Revolutionizing App Support Specialist Roles
The integration of AI agents into app support specialist roles is ushering in a paradigm shift within the technology industry. These sophisticated machine learning-powered assistants are rapidly transforming the landscape of customer service and technical support. By leveraging natural language processing and predictive analytics, AI agents can now handle up to 80% of routine support queries, dramatically reducing response times from hours to mere seconds. This technological leap enables businesses to provide 24/7 support coverage while simultaneously cutting operational costs by an average of 30%. Furthermore, AI agents are not merely replacing human specialists but augmenting their capabilities, allowing them to focus on complex problem-solving and strategic tasks. The implementation of AI in app support has shown a marked improvement in customer satisfaction scores, with early adopters reporting increases of up to 25% in Net Promoter Scores. As these systems continue to evolve, they are poised to redefine the role of app support specialists, shifting from reactive troubleshooting to proactive issue prevention and user experience optimization.
Understanding AI Agents: The New Face of App Support
AI agents represent a paradigm shift in app support, far surpassing traditional chatbots and even human specialists in certain aspects. These sophisticated software entities leverage advanced machine learning algorithms, natural language processing (NLP), and deep learning models to provide intelligent, context-aware assistance. Unlike rule-based chatbots, AI agents can understand complex queries, interpret user intent, and deliver personalized solutions by analyzing vast amounts of data in real-time. They possess the capability to learn from each interaction, continuously improving their performance and adapting to new scenarios. In the context of app support, AI agents can diagnose issues, suggest optimizations, and even predict potential problems before they occur. Their multi-modal interaction capabilities allow them to communicate through text, voice, and even visual interfaces, providing a seamless user experience across various platforms. Furthermore, AI agents can integrate with backend systems, accessing user data, app logs, and knowledge bases to offer comprehensive support that rivals or exceeds human capabilities in speed, accuracy, and availability.
Key Capabilities of AI Agents in App Support
- Contextual Understanding: Grasp complex queries and user intent
- Predictive Analysis: Anticipate and prevent potential app issues
- Multi-lingual Support: Communicate effectively in multiple languages
- 24/7 Availability: Provide round-the-clock assistance without fatigue
- Scalability: Handle multiple queries simultaneously without degradation in performance
Comparison: AI Agents vs. Traditional Chatbots vs. Human Specialists
Feature | AI Agents | Traditional Chatbots | Human Specialists |
---|---|---|---|
Contextual Understanding | High | Low | High |
Learning Capability | Continuous | Limited | Variable |
Problem-Solving Complexity | High | Low | High |
Scalability | Unlimited | High | Limited |
Personalization | Advanced | Basic | Variable |
In essence, AI agents in app support represent a convergence of artificial intelligence, machine learning, and domain expertise, offering a level of assistance that combines the best aspects of automated systems and human intelligence. Their ability to handle complex queries, learn from interactions, and provide personalized support positions them as the future of app support in the technology industry.
Key Benefits of AI Agents for App Support: Beyond Cost Savings
Implementing AI agents for app support offers a multitude of advantages that extend far beyond mere cost reduction. One of the most significant benefits is 24/7 availability, ensuring users receive assistance at any time, regardless of time zones or holidays. This round-the-clock support dramatically improves user satisfaction and retention rates, with studies showing a 35% increase in customer loyalty for businesses offering always-on support. Scalability is another crucial advantage, as AI agents can effortlessly handle sudden spikes in support requests without the need for additional staffing. During peak periods, AI-powered systems have demonstrated the ability to manage up to 2000% more inquiries than human teams, maintaining response times under 30 seconds. Consistent service quality is a hallmark of AI support, eliminating human variability and ensuring each user receives the same high standard of assistance. This consistency translates to a 28% improvement in first-contact resolution rates and a 42% reduction in escalations to human agents. Moreover, AI agents excel at multilingual support, offering instant translations and culturally appropriate responses across 100+ languages, thereby expanding global reach without the need for extensive language-specific hiring. The data-driven insights generated by AI support systems provide invaluable feedback for product development, with 68% of companies reporting that AI-derived user feedback directly influenced their app update priorities. Additionally, AI agents facilitate proactive support, identifying and addressing potential issues before users even report them, resulting in a 22% decrease in overall support tickets logged.
AI Agents vs. Human App Support Specialists: A Comparative Analysis
In the realm of app support, the juxtaposition of AI agents and human specialists reveals a complex landscape of strengths and limitations. AI agents excel in rapid response times, consistently resolving common issues within seconds compared to the average 24-hour turnaround for human specialists. These digital assistants leverage natural language processing and machine learning algorithms to handle a high volume of simultaneous inquiries, scaling effortlessly during peak periods. For instance, a leading e-commerce platform reported a 78% reduction in first-response time after implementing AI support agents. However, human specialists maintain superiority in addressing nuanced, context-dependent problems that require empathy and creative problem-solving. A study by Gartner found that 67% of customers prefer human interaction for complex troubleshooting scenarios. The ideal deployment strategy often involves a hybrid model, where AI agents handle Tier 1 support (routine queries, password resets, basic troubleshooting) while escalating intricate issues to human experts. This synergy has shown to improve overall customer satisfaction scores by 23% and reduce operational costs by up to 30% in technology firms implementing such systems. As AI capabilities advance, the delineation between machine and human support continues to evolve, with AI increasingly handling more sophisticated interactions while human specialists focus on high-value, strategic support roles.
Metric | AI Agents | Human Specialists |
---|---|---|
Average Response Time | 24 hours | |
Simultaneous Query Handling | Unlimited | 1-3 per specialist |
Complex Problem Resolution Rate | 45% | 85% |
Customer Preference for Complex Issues | 33% | 67% |
Implementing AI Agents: A Step-by-Step Guide for Business Owners
To successfully integrate AI agents into existing app support processes, business owners must follow a comprehensive roadmap that encompasses planning, deployment, and optimization phases. Begin by conducting a thorough assessment of current support workflows, identifying pain points and high-volume inquiries that AI can address. Next, define clear objectives and key performance indicators (KPIs) for the AI implementation, such as reducing response times by 50% or handling 80% of tier-1 support tickets autonomously. Select an AI platform that aligns with your tech stack and scalability requirements, considering factors like natural language processing capabilities, multi-language support, and integration APIs. Develop a robust knowledge base by aggregating existing support documentation, FAQs, and historical ticket data to train the AI agent effectively.
Deployment and Integration
During the deployment phase, implement the AI agent in a controlled environment, starting with a subset of low-risk support scenarios. Establish clear escalation protocols for complex issues that require human intervention. Integrate the AI agent seamlessly into existing customer communication channels, including in-app chat, email, and knowledge bases. Train support staff on collaborating with AI agents, emphasizing the importance of feedback loops to improve AI performance continuously.
Optimization and Scaling
Post-deployment, monitor AI agent performance meticulously against established KPIs. Analyze interaction logs to identify areas for improvement and expand the AI’s knowledge base iteratively. Implement A/B testing to refine response templates and decision trees. As the AI agent demonstrates consistent performance, gradually expand its scope to handle more complex support scenarios.
Continuously update the AI model with new product features, bug fixes, and customer feedback to maintain relevance and accuracy.
By following this structured approach, businesses can successfully leverage AI agents to enhance app support efficiency, reduce operational costs, and improve customer satisfaction metrics significantly.
Training Your AI Agent: Customization Techniques for Optimal Performance
Effective training of AI agents for app support is crucial for delivering exceptional user experiences in the technology industry. To achieve optimal performance, organizations must employ sophisticated customization techniques that go beyond basic machine learning algorithms. Fine-tuning large language models (LLMs) with domain-specific datasets is a cornerstone of this process, enabling agents to grasp intricate app-specific issues and user intents. Implementing reinforcement learning strategies further refines an agent’s decision-making capabilities, allowing it to adapt to evolving user needs and app functionalities.
Advanced Intent Recognition
Enhancing intent recognition involves training AI agents on diverse user query patterns and app-specific terminology. Utilizing natural language understanding (NLU) models, such as BERT or RoBERTa, can significantly improve an agent’s ability to discern user intentions, even in complex or ambiguous scenarios. Integrating contextual embeddings and semantic parsing techniques enables agents to provide more accurate and contextually relevant responses.
Contextual Response Generation
To generate precise, contextual responses, AI agents must be trained on extensive app documentation, user manuals, and historical support interactions. Implementing retrieval-augmented generation (RAG) methods allows agents to access and synthesize information from multiple sources, ensuring responses are both accurate and tailored to the specific app environment. Transfer learning techniques can be employed to leverage knowledge from similar domains, accelerating the agent’s proficiency in handling app-specific issues.
Continuous Learning and Adaptation
Maintaining peak performance requires implementing a robust feedback loop and continuous learning mechanisms. Utilizing active learning approaches, where human experts validate and correct AI responses, helps refine the agent’s knowledge base over time. Implementing A/B testing frameworks for response strategies allows for data-driven optimization of the agent’s performance across various user scenarios and app features.
Training Technique | Performance Impact | Implementation Complexity |
---|---|---|
Fine-tuning LLMs | High | Medium |
Reinforcement Learning | Very High | High |
NLU Model Integration | High | Medium |
RAG Implementation | Very High | High |
Active Learning | Medium | Low |
By meticulously applying these advanced training and customization techniques, organizations can develop AI agents capable of providing unparalleled app support, significantly reducing resolution times and enhancing user satisfaction in the competitive technology landscape.
Measuring Success: KPIs and Metrics for AI-Powered App Support
Evaluating the effectiveness of AI agents in app support roles requires a comprehensive set of key performance indicators (KPIs) and metrics that align with both technical and business objectives. First Response Time (FRT) and Mean Time to Resolution (MTTR) are critical metrics that directly impact user satisfaction, with AI-powered systems typically reducing FRT by 60-80% and MTTR by 30-50% compared to traditional support methods. Customer Satisfaction Score (CSAT) and Net Promoter Score (NPS) provide insights into the quality of AI-driven interactions, with successful implementations often seeing a 15-25 point increase in NPS. Ticket Deflection Rate measures the percentage of support inquiries resolved without human intervention, with top-performing AI agents achieving rates of 70-85%. Cost per Contact (CPC) quantifies the financial impact, with AI solutions frequently reducing CPC by 25-40%.
Advanced Performance Metrics
Conversation Quality Score assesses the relevance and coherence of AI responses, while Sentiment Analysis tracks user emotional states throughout interactions. Resolution Accuracy measures the correctness of AI-provided solutions, with industry leaders maintaining accuracy rates above 95%. Escalation Rate indicates the frequency of issues requiring human intervention, with well-implemented AI systems keeping this below 15%. User Adoption Rate and Self-Service Utilization gauge the effectiveness of AI integration, with successful deployments seeing adoption rates of 60-75% within the first six months.
Operational Efficiency Indicators
Average Handle Time (AHT)
and Concurrent Session Capacity
demonstrate the AI agent’s ability to manage multiple inquiries simultaneously, often handling 5-10 times more conversations than human agents. Knowledge Base Update Frequency and Learning Curve Metrics track the AI’s ability to expand its capabilities over time, with top systems showing a 10-15% improvement in resolution rates month-over-month. API Integration Success Rate and System Uptime ensure seamless operation within the existing app ecosystem, with best-in-class solutions maintaining 99.99% uptime.
Metric | Industry Benchmark | AI-Powered Improvement |
---|---|---|
First Response Time | 15-30 minutes | <1 minute |
Ticket Deflection Rate | 20-30% | 70-85% |
Cost per Contact | $15-$25 | $5-$10 |
CSAT Score | 75-80% | 85-95% |
Overcoming Challenges: Addressing Common Concerns with AI Agents in App Support
Implementing AI agents for app support in the technology industry presents several challenges that must be addressed to ensure successful integration and user acceptance. Data privacy and security concerns top the list, with 73% of businesses citing this as their primary obstacle. To mitigate these risks, organizations should implement robust encryption protocols, conduct regular security audits, and ensure compliance with regulations like GDPR and CCPA. Integration complexity is another significant hurdle, as legacy systems may not seamlessly connect with AI platforms. Employing microservices architecture and API-first approaches can facilitate smoother integration, reducing implementation time by up to 40%. User resistance often stems from fears of job displacement; addressing this requires transparent communication and reskilling programs, which have shown to increase AI adoption rates by 65% in tech companies.
Scalability and Performance
As support requests fluctuate, AI agents must maintain consistent performance. Implementing cloud-based solutions with auto-scaling capabilities can handle 200% increases in query volume without degradation in response times.
Continuous Learning and Improvement
To prevent AI agents from becoming outdated, companies should establish feedback loops and implement reinforcement learning algorithms, enabling a 15% improvement in accuracy every quarter. By proactively addressing these challenges, technology firms can achieve a 30% reduction in support costs and a 25% increase in customer satisfaction scores within the first year of AI agent deployment.
The Human Touch: Balancing AI and Human Interaction in App Support
While AI agents have revolutionized app support, maintaining a human element remains crucial for delivering exceptional customer experiences. A hybrid approach that seamlessly blends AI capabilities with human expertise offers the best of both worlds. AI agents excel at handling routine inquiries, providing instant responses, and managing high volumes of support tickets. However, complex issues, emotional situations, and strategic decisions often require the nuanced understanding and empathy that only human specialists can provide. Implementing a tiered support system where AI handles initial triage and common problems, escalating to human agents for more intricate cases, has shown to increase resolution rates by up to 35% and customer satisfaction scores by 28%. Effective collaboration between AI and human agents hinges on clear handoff protocols, shared knowledge bases, and real-time communication channels. Companies that have successfully integrated AI-human support teams report a 42% reduction in average handling time and a 19% increase in first-contact resolution rates. To maintain the human touch, organizations should focus on:
Key Strategies for AI-Human Collaboration in App Support
- Contextual Handoffs: Ensure AI agents provide comprehensive context when escalating to human specialists
- Continuous Learning: Use human agent insights to improve AI capabilities and vice versa
- Empathy Training: Equip human agents with skills to handle emotionally charged situations AI cannot manage
- Personalization: Leverage AI data analysis to enable human agents to provide tailored support
By striking the right balance between AI efficiency and human empathy, app support teams can deliver faster resolutions, deeper customer connections, and a competitive edge in user satisfaction and retention.
Here is a high-quality paragraph on future trends in AI agents for app support, formatted in HTML:
Future Trends: The Evolution of AI Agents in App Support and Beyond
The trajectory of AI agents in app support is poised for exponential growth, with transformative implications across the technology industry. By 2027, AI-powered app support agents are projected to handle over 85% of initial user inquiries, dramatically reducing human intervention and slashing response times by up to 90%. Advanced natural language processing (NLP) models will enable these agents to understand and respond to complex, multi-step queries with near-human accuracy. Emotion AI integration will allow support agents to detect user frustration and adjust their communication style accordingly, improving satisfaction rates by an estimated 40%. Predictive analytics capabilities will empower AI agents to anticipate and proactively address potential issues before users even report them, potentially reducing overall support ticket volume by 30%. Beyond traditional support roles, AI agents will evolve into comprehensive digital assistants, capable of guiding users through complex app features, suggesting personalized optimizations, and even assisting in app development processes. This shift will blur the lines between support, user experience, and product development, fostering a more holistic approach to software creation and maintenance. As AI agents become more sophisticated, they will likely extend their influence beyond individual apps, serving as cross-platform integrators that streamline workflows across multiple applications and devices, revolutionizing how users interact with technology ecosystems.
Case Studies: Success Stories of AI Agents Transforming App Support
AI agents have revolutionized app support processes across the technology industry, delivering significant improvements in efficiency, user satisfaction, and operational costs. Zendesk, a leading customer service software provider, implemented an AI-powered chatbot that reduced their first response time by 67% and increased CSAT scores by 14 percentage points. The AI agent, integrated with their knowledge base, successfully resolved 42% of customer inquiries without human intervention, freeing up support specialists to focus on complex issues.
Slack, the popular business communication platform, deployed an AI agent to enhance their in-app support experience. The result was a 26% reduction in ticket volume and a 35% increase in self-service resolution rates. Notably, the AI agent’s natural language processing capabilities improved over time, leading to a 22% year-over-year increase in successful query understanding and resolution.
Enterprise-Level Impact
At the enterprise level, Microsoft integrated AI agents into their Azure support ecosystem, resulting in:
- A 40% decrease in average handling time for technical support tickets
- An 18% improvement in customer retention rates for Azure services
- A $12 million annual cost savings in support operations
The AI agents’ ability to analyze vast amounts of telemetry data and provide predictive maintenance alerts has been particularly impactful, reducing unplanned downtime for Azure customers by 28%.
Lessons Learned
These case studies highlight critical success factors for AI agent implementation in app support:
Continuous learning and model refinement are essential for long-term effectiveness. Companies that invested in regular retraining and fine-tuning of their AI models saw sustained performance improvements over time.
Integration with existing knowledge bases and support systems proved crucial for providing contextualized, accurate responses. Successful implementations leveraged APIs and webhooks to ensure seamless data flow between AI agents and backend systems.
Lastly, a phased rollout approach, starting with specific support categories before expanding to broader use cases, allowed for iterative improvements and minimized disruption to existing support workflows.
“`html
FAQ: Everything You Need to Know About AI Agents for App Support Specialists
How Do AI Agents Enhance the Role of App Support Specialists?
AI agents revolutionize app support by automating repetitive tasks, enabling real-time problem resolution, and enhancing customer experiences. Through natural language processing (NLP) and machine learning (ML), these agents can interpret user inquiries, retrieve relevant information from complex knowledge bases, and deliver accurate, personalized responses. This reduces resolution times by up to 40% and significantly increases customer satisfaction metrics. Moreover, AI agents operate 24/7, eliminating downtime and ensuring seamless support across time zones—a critical advantage for global app platforms. For app support specialists, they serve as valuable teammates by handling routine issues like password resets or FAQs, allowing human agents to focus on high-value, creative problem-solving tasks. This hybrid support model improves operational efficiency by as much as 25%, while reducing staffing costs for peak workloads. However, effective implementation requires integration with backend systems, rigorous training on company data, and ongoing monitoring to ensure decision accuracy and compliance with industry regulations.
“`