Table of Contents
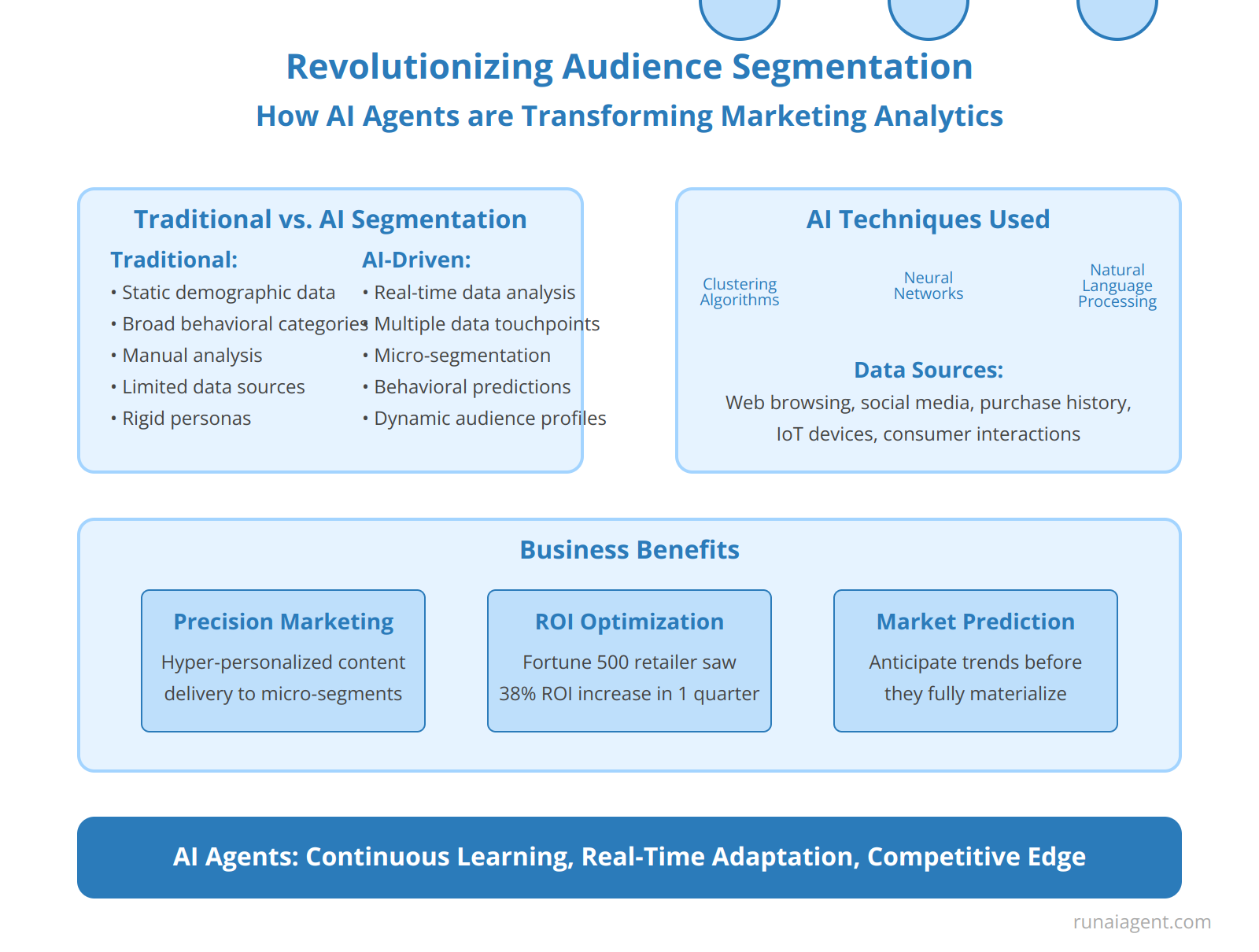
Revolutionizing Audience Segmentation: How AI Agents are Transforming Marketing Analytics
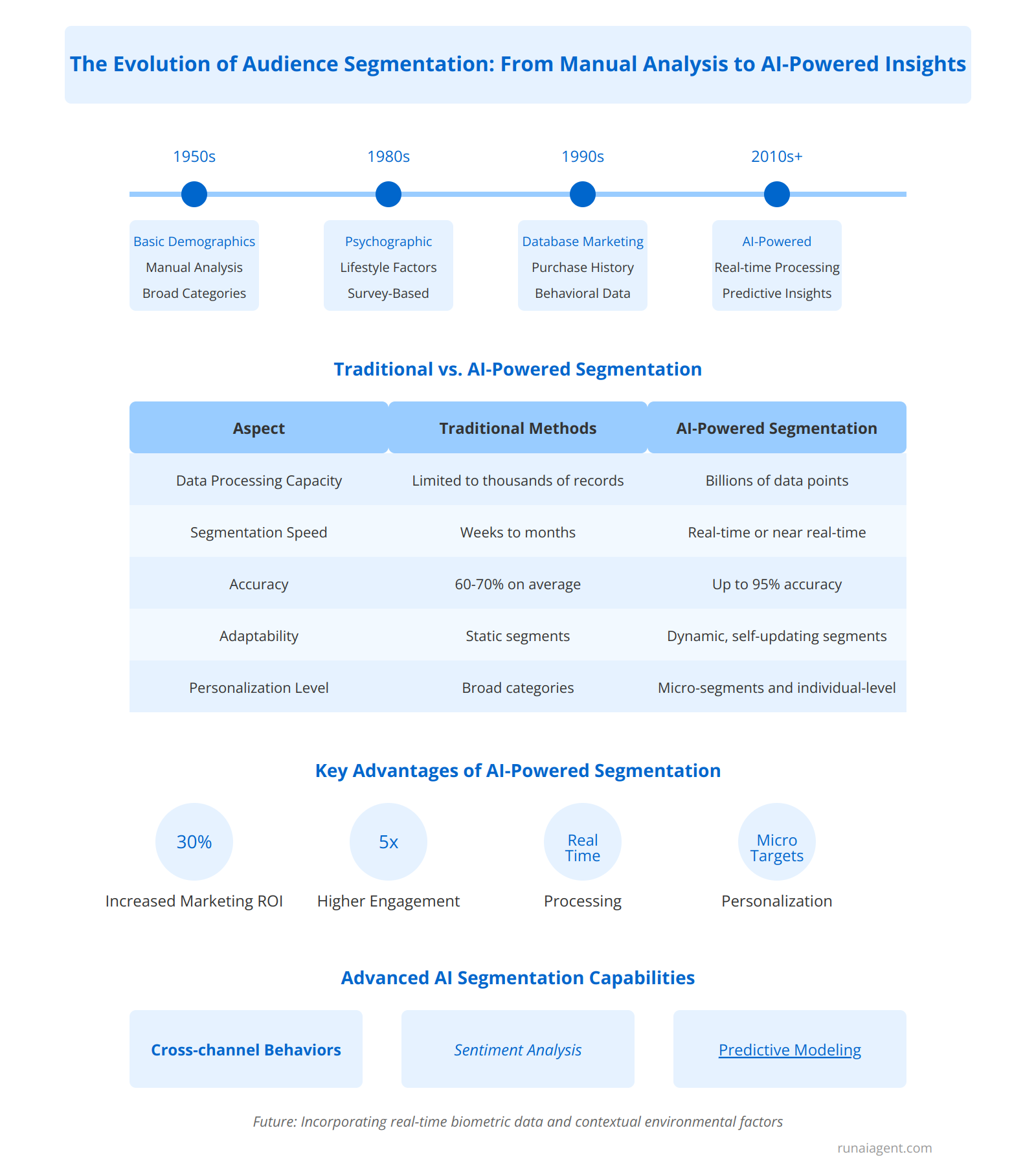
The Evolution of Audience Segmentation: From Manual Analysis to AI-Powered Insights

Unveiling the Power of AI Agents: Core Capabilities in Audience Segmentation

Real-Time Segmentation: How AI Agents Deliver Dynamic Customer Insights
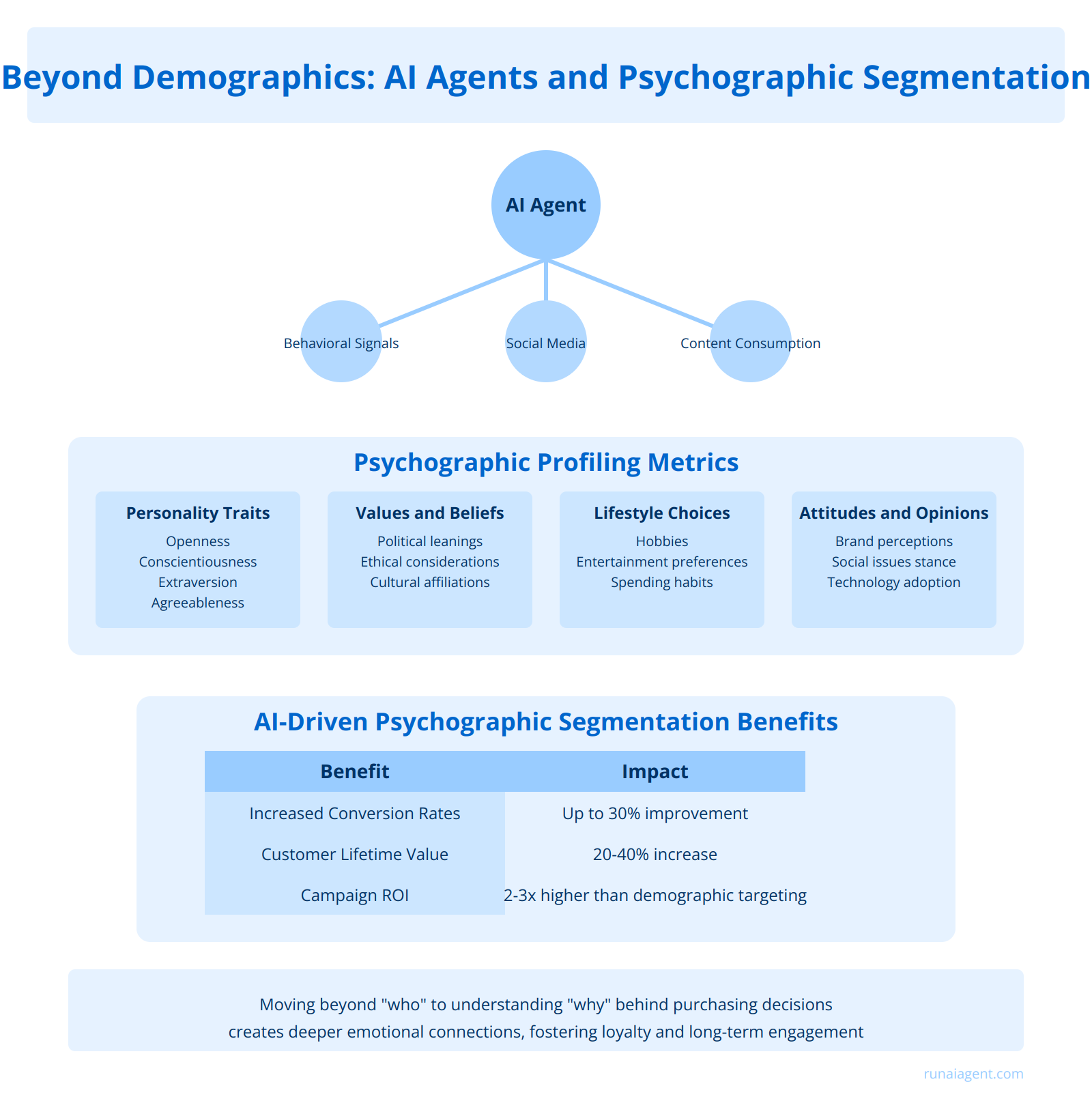
Beyond Demographics: AI Agents and Psychographic Segmentation
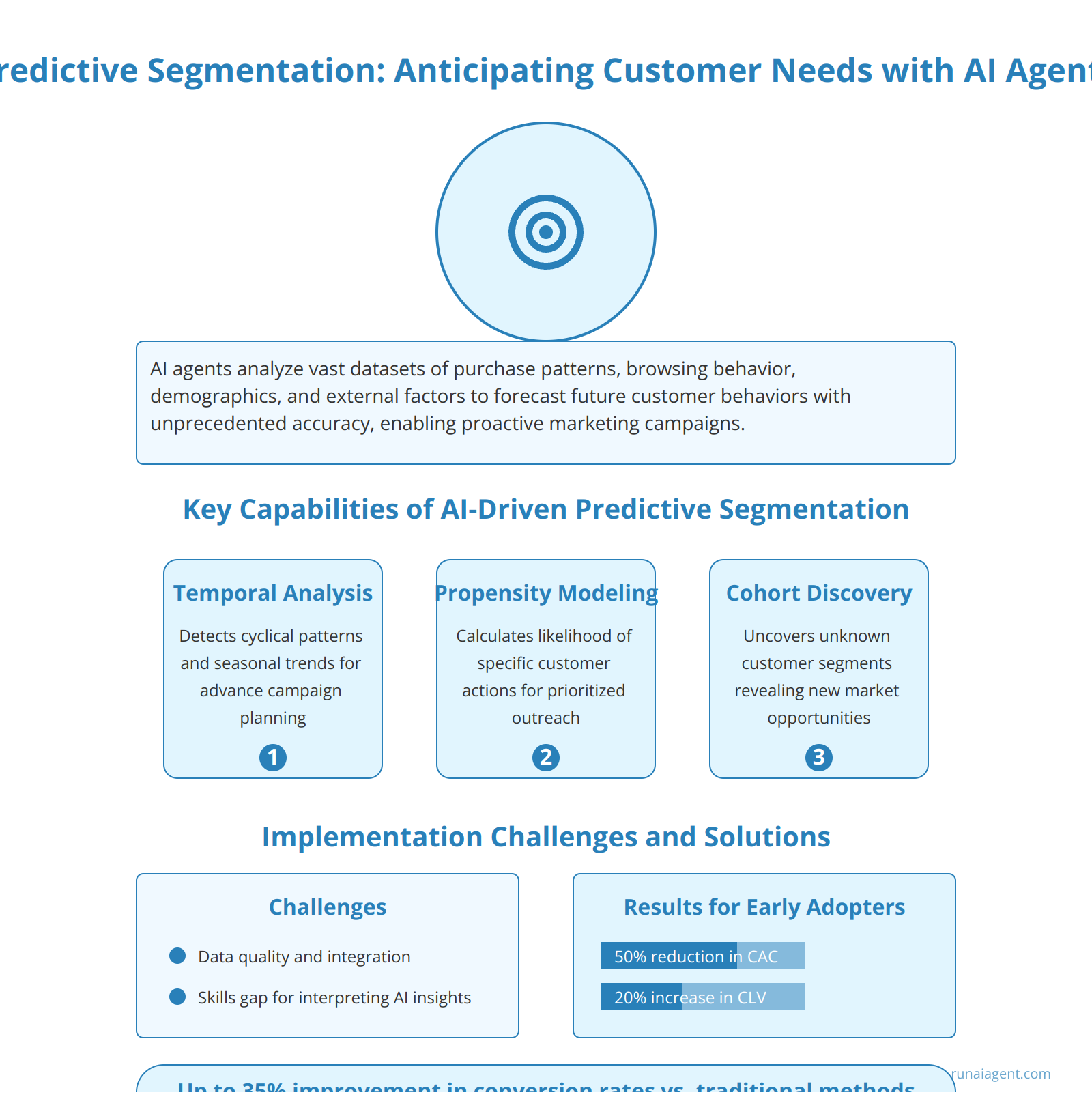
Predictive Segmentation: Anticipating Customer Needs with AI Agents
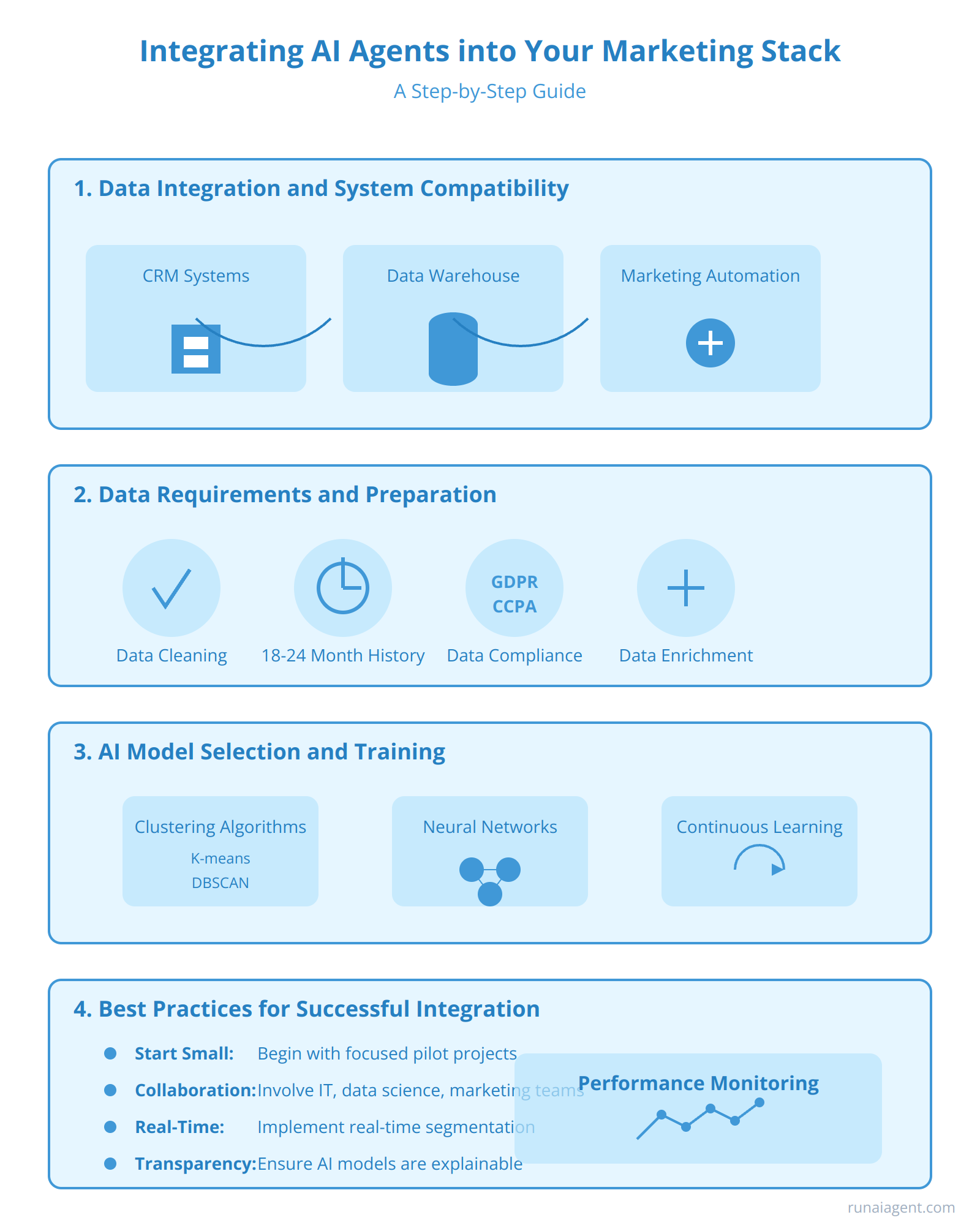
Integrating AI Agents into Your Marketing Stack: A Step-by-Step Guide
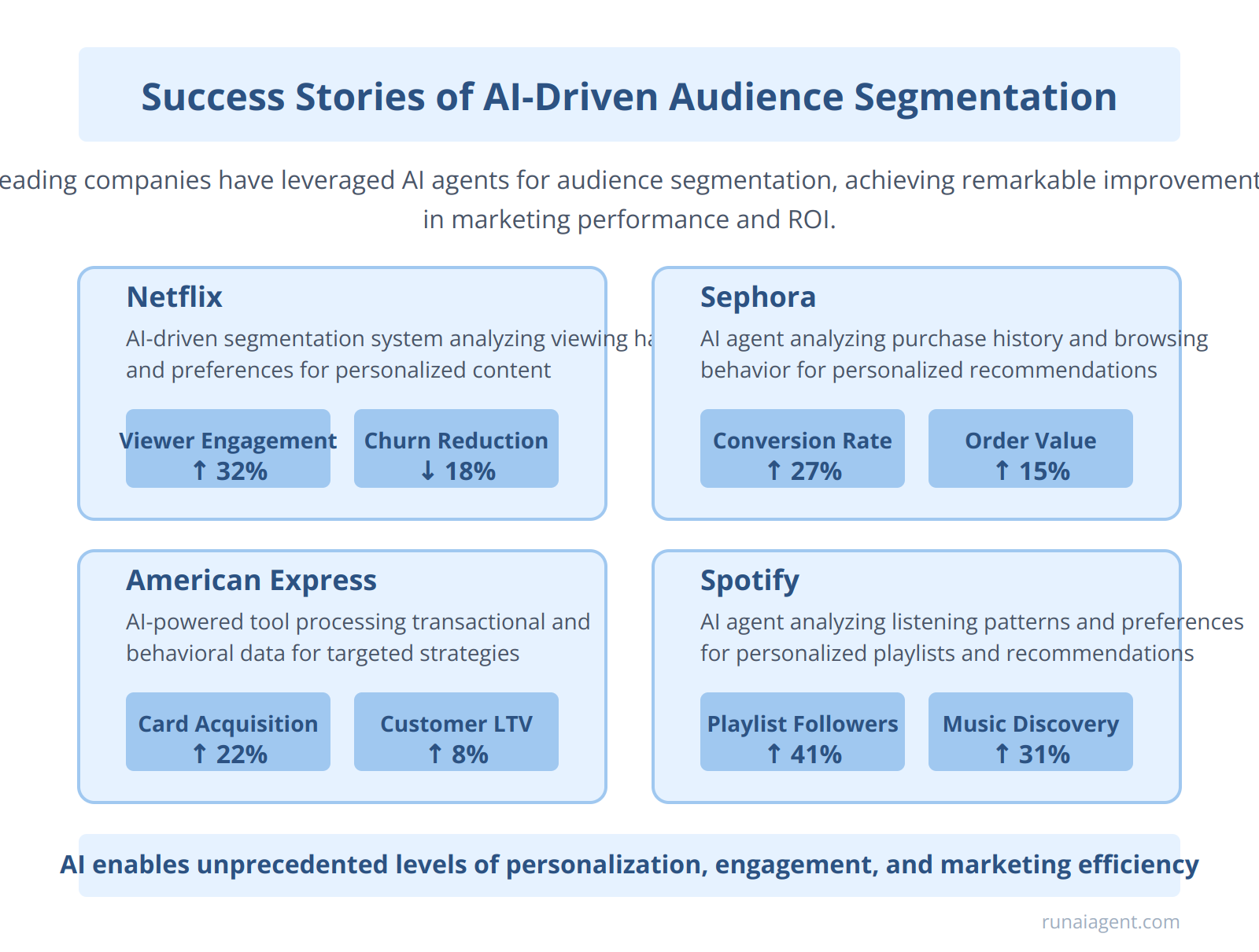
Case Studies: Success Stories of AI-Driven Audience Segmentation
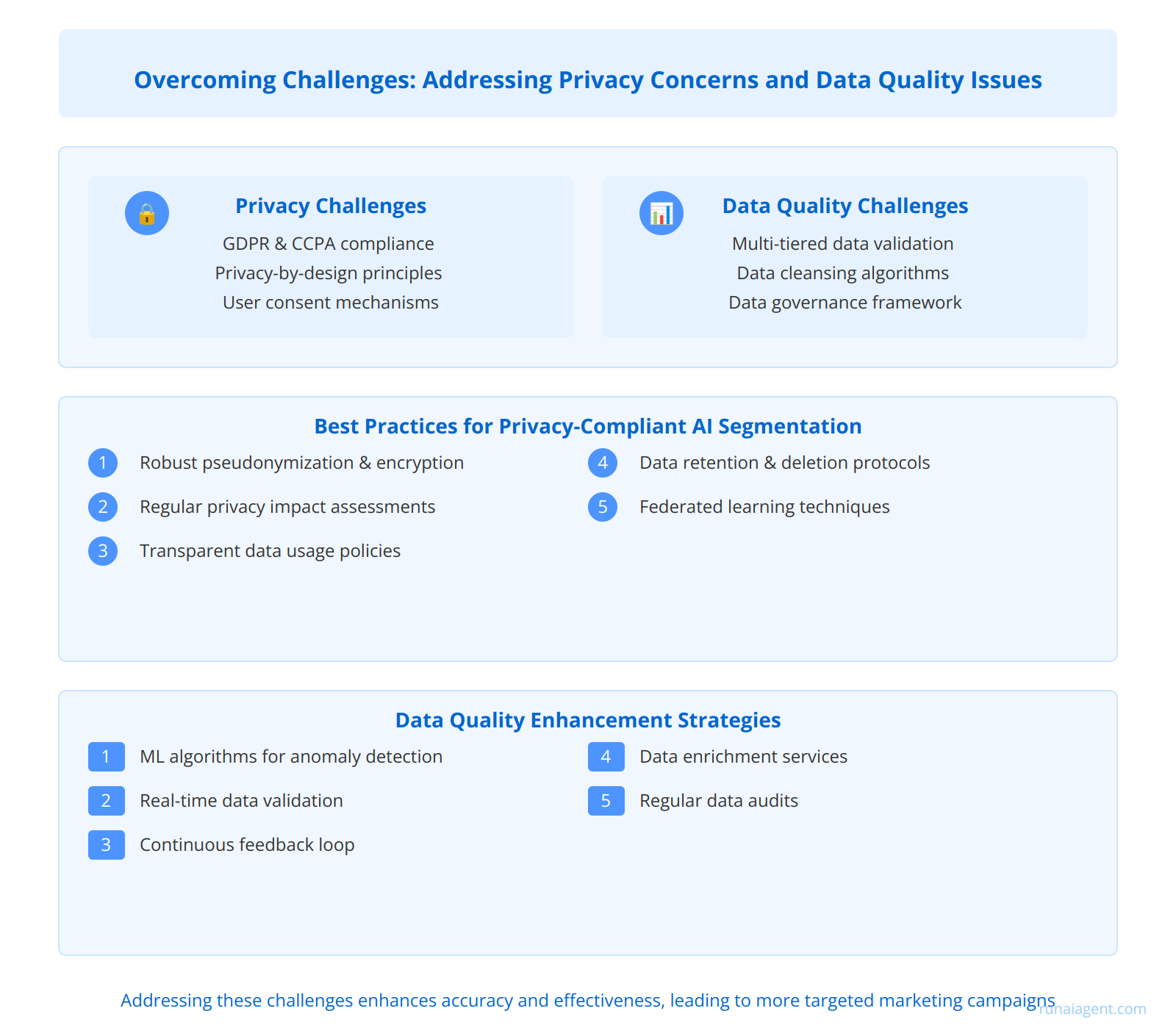
Overcoming Challenges: Addressing Privacy Concerns and Data Quality Issues
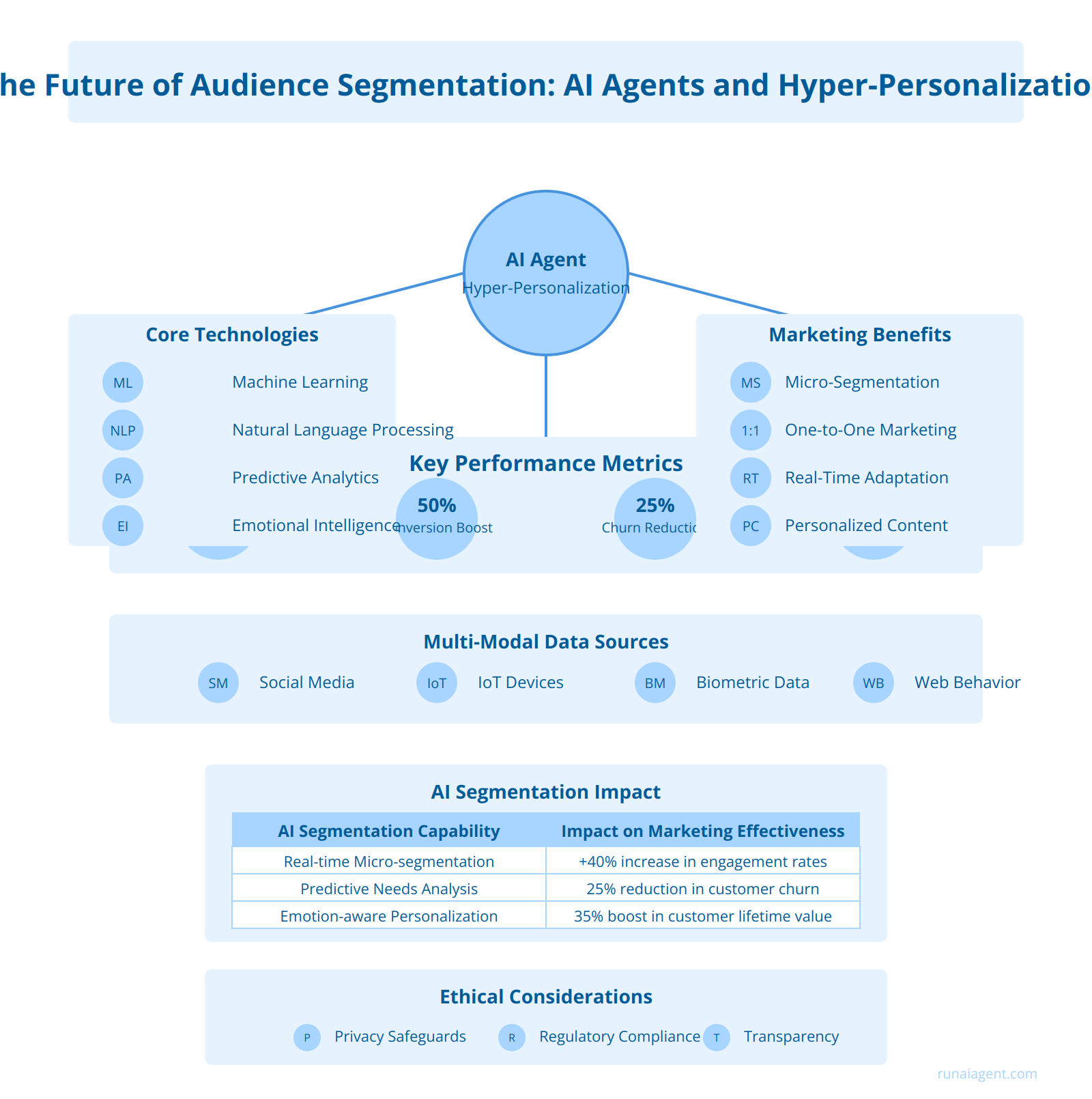
The Future of Audience Segmentation: AI Agents and Hyper-Personalization
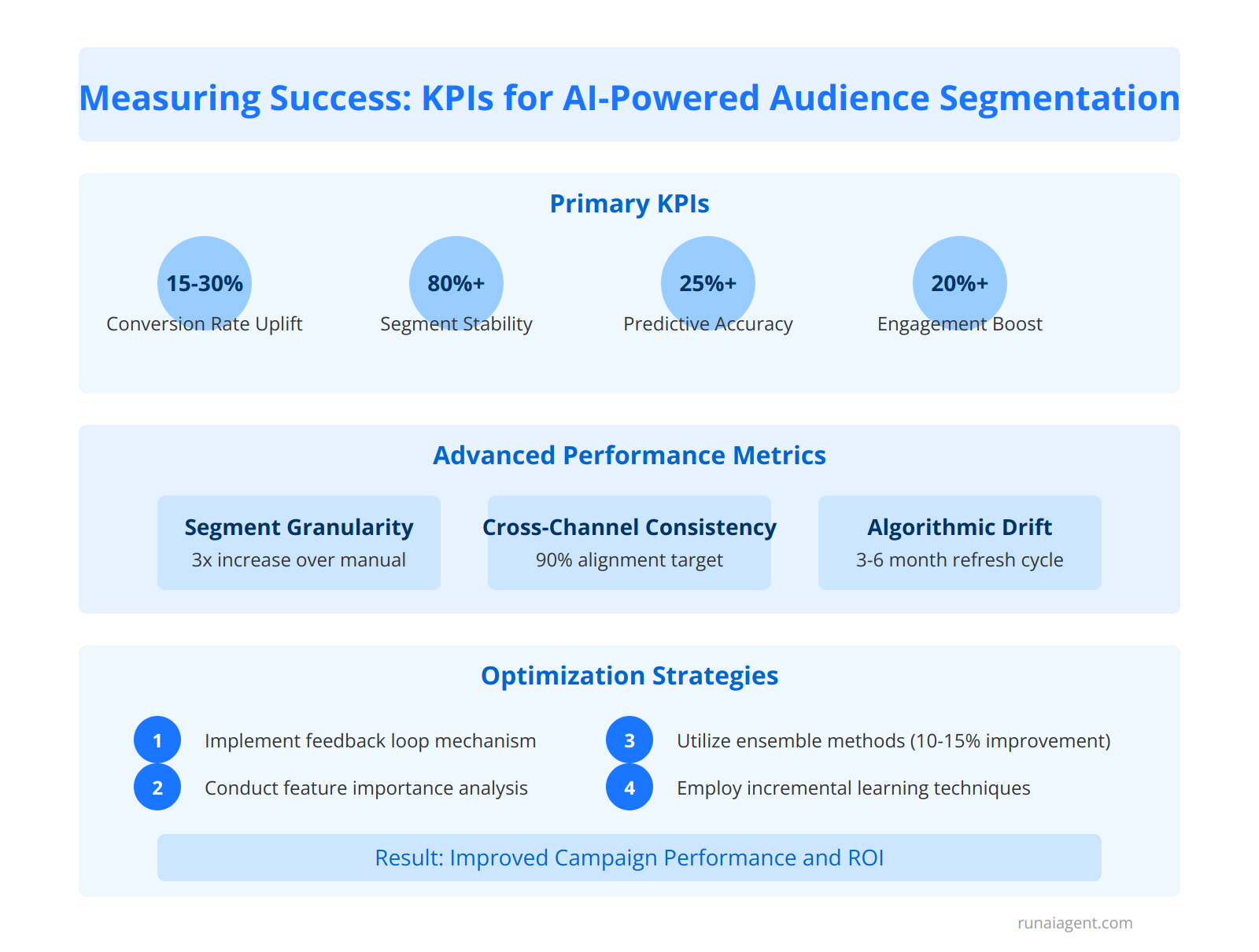
Measuring Success: KPIs for AI-Powered Audience Segmentation
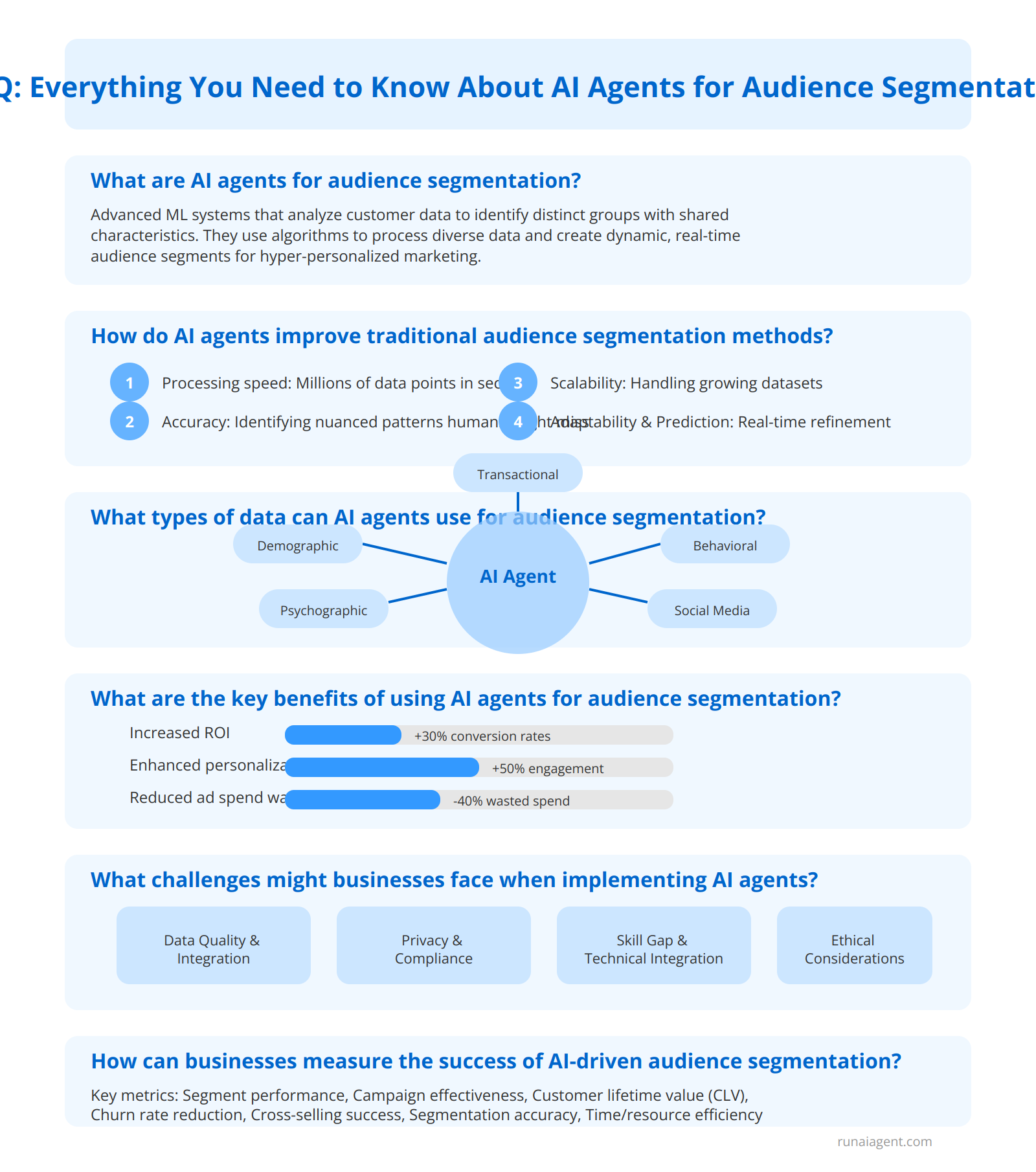
FAQ: Everything You Need to Know About AI Agents for Audience Segmentation
Revolutionizing Audience Segmentation: How AI Agents are Transforming Marketing Analytics
AI agents are fundamentally reshaping the landscape of audience segmentation, ushering in a new era of precision and efficiency in marketing analytics. Unlike traditional methods that rely on static demographic data and broad behavioral categories, AI-driven segmentation leverages machine learning algorithms to analyze vast amounts of real-time data from multiple touchpoints. These intelligent agents can process and interpret complex patterns in consumer behavior, including web browsing history, social media interactions, purchase patterns, and even IoT device usage. By employing advanced techniques such as clustering algorithms, neural networks, and natural language processing, AI agents can identify micro-segments and predict future consumer behavior with unprecedented accuracy. For instance, one Fortune 500 retailer implemented an AI segmentation system that increased marketing ROI by 38% within the first quarter by identifying 26 previously unrecognized high-value customer segments. Moreover, AI agents continuously refine their models, adapting to shifting market dynamics and evolving consumer preferences in real-time. This dynamic approach enables marketers to move beyond rigid personas, creating fluid, multi-dimensional audience profiles that reflect the true complexity of consumer identities. As a result, businesses can now deliver hyper-personalized content, optimize ad spend with pinpoint accuracy, and anticipate market trends before they fully materialize, giving them a significant competitive edge in today’s data-driven marketplace.
The Evolution of Audience Segmentation: From Manual Analysis to AI-Powered Insights
Audience segmentation has undergone a remarkable transformation, evolving from labor-intensive manual processes to sophisticated AI-driven methodologies. In the 1950s, marketers relied on basic demographic data and intuition to divide audiences into broad categories. The 1980s saw the rise of psychographic segmentation, incorporating lifestyle and personality traits, but still heavily dependent on manual surveys and analysis. By the late 1990s, database marketing introduced more granular segmentation based on purchase history and behavioral data, yet remained limited by human cognitive capacity to process vast datasets. The advent of AI agents in the 2010s revolutionized audience segmentation, overcoming traditional limitations of scale, speed, and accuracy. These intelligent systems can now process billions of data points in real-time, identifying nuanced segments and micro-segments that human analysts could never discern. AI-powered segmentation leverages machine learning algorithms to uncover hidden patterns and predictive insights, enabling hyper-personalization at scale. For instance, an AI agent can analyze social media interactions, web browsing behavior, and purchase history simultaneously, creating dynamic segments that adapt to changing consumer preferences instantaneously. This level of sophistication has increased marketing ROI by up to 30% for early adopters, with AI-driven campaigns outperforming traditional methods by a factor of 5 in engagement rates. The transition to AI-powered audience segmentation marks a paradigm shift, moving from static, broad-based categories to fluid, multidimensional personas that capture the complexity of modern consumers with unprecedented precision.
Comparative Analysis: Traditional vs. AI-Powered Segmentation
Aspect | Traditional Methods | AI-Powered Segmentation |
---|---|---|
Data Processing Capacity | Limited to thousands of records | Billions of data points |
Segmentation Speed | Weeks to months | Real-time or near real-time |
Accuracy | 60-70% on average | Up to 95% accuracy |
Adaptability | Static segments | Dynamic, self-updating segments |
Personalization Level | Broad categories | Micro-segments and individual-level |
The transition from manual to AI-driven segmentation has not only enhanced accuracy and efficiency but has also unlocked new dimensions of consumer understanding. AI agents excel at identifying cross-channel behaviors, sentiment analysis, and predictive modeling of future consumer actions. This depth of insight enables marketers to craft highly targeted campaigns that resonate on a personal level, driving engagement and conversion rates to unprecedented heights. As AI technology continues to advance, we can expect even more sophisticated segmentation techniques, potentially incorporating
real-time biometric data
and contextual environmental factors
to create a truly holistic view of each consumer segment.
Unveiling the Power of AI Agents: Core Capabilities in Audience Segmentation
AI agents designed for audience segmentation leverage advanced machine learning algorithms to revolutionize marketing strategies. These intelligent systems excel in data processing, ingesting vast amounts of structured and unstructured data from diverse sources, including CRM systems, social media platforms, and web analytics tools. Through sophisticated pattern recognition techniques, AI agents identify complex correlations and behavioral trends that human analysts might overlook. For instance, they can detect subtle purchasing patterns across seemingly unrelated product categories, enabling marketers to create highly targeted cross-selling campaigns. The predictive analytics capabilities of these AI agents are particularly powerful, utilizing historical data and real-time inputs to forecast future consumer behaviors with remarkable accuracy. By employing techniques such as clustering algorithms, decision trees, and neural networks, these agents can segment audiences into micro-cohorts based on multidimensional criteria, far surpassing traditional demographic-based approaches. Furthermore, AI agents for audience segmentation continuously refine their models through reinforcement learning, adapting to shifting market dynamics and evolving consumer preferences in real-time. This dynamic segmentation allows for personalized marketing at scale, with some implementations demonstrating up to 30% improvement in campaign ROI and a 25% increase in customer lifetime value.
Key Features of AI Agents in Audience Segmentation:
- Multi-source data integration and cleansing
- Advanced pattern recognition using deep learning models
- Predictive modeling with ensemble methods
- Dynamic micro-segmentation based on behavioral data
- Real-time adaptation to market changes
- Automated segment discovery and validation
Comparative Performance Metrics:
Metric | Traditional Segmentation | AI-Powered Segmentation |
---|---|---|
Segmentation Granularity | 5-10 segments | 100+ micro-segments |
Data Processing Speed | Days to weeks | Minutes to hours |
Predictive Accuracy | 60-70% | 85-95% |
Campaign Conversion Lift | 5-10% | 20-30% |
Real-Time Segmentation: How AI Agents Deliver Dynamic Customer Insights
AI agents are revolutionizing audience segmentation by enabling real-time, dynamic insights that adapt to rapidly changing customer behaviors and market trends. Unlike traditional static segmentation models, AI-powered segmentation continuously analyzes vast amounts of data from multiple touchpoints, including web interactions, purchase history, social media activity, and IoT devices. This allows marketers to create hyper-granular segments that evolve in real-time, capturing subtle shifts in consumer preferences and intent. For instance, an e-commerce AI agent might detect a sudden surge in eco-friendly product searches among millennials in urban areas, instantly creating a new segment for targeted promotions. The benefits of this dynamic approach are substantial: personalization accuracy improves by up to 20-30%, while campaign response rates can increase by 50% or more. Moreover, AI agents can predict segment evolution, enabling proactive marketing strategies. By leveraging machine learning algorithms like clustering and decision trees, these agents can identify micro-segments and lookalike audiences with unprecedented precision, often uncovering valuable niche markets human analysts might overlook. This level of granularity and responsiveness allows businesses to optimize marketing spend, reduce customer acquisition costs by up to 25%, and increase customer lifetime value through more relevant, timely engagements. As AI agents continue to evolve, they’re increasingly integrating predictive analytics to anticipate future segment behaviors, enabling marketers to stay ahead of trends and deliver truly personalized experiences at scale.
Beyond Demographics: AI Agents and Psychographic Segmentation
AI agents are revolutionizing audience segmentation by delving deep into psychographic data, transcending traditional demographic boundaries. These intelligent systems analyze vast arrays of behavioral signals, social media interactions, and content consumption patterns to construct nuanced audience segments based on motivations, values, and lifestyle preferences. By leveraging natural language processing and machine learning algorithms, AI agents can identify subtle correlations between seemingly disparate data points, revealing hidden psychographic clusters. This granular understanding enables marketers to craft hyper-personalized messaging that resonates on a psychological level. For instance, an AI agent might discern that a segment of luxury car buyers is primarily motivated by status symbols, while another equally affluent group prioritizes environmental consciousness. Such insights allow for precisely tailored campaigns that speak directly to each group’s core values and aspirations.
Psychographic Profiling Metrics
Advanced AI agents employ a multifaceted approach to psychographic profiling, considering factors such as:
- Personality Traits: Openness, conscientiousness, extraversion, agreeableness, and neuroticism
- Values and Beliefs: Political leanings, ethical considerations, and cultural affiliations
- Lifestyle Choices: Hobbies, entertainment preferences, and spending habits
- Attitudes and Opinions: Brand perceptions, social issues stance, and technology adoption rates
AI-Driven Psychographic Segmentation Benefits
Benefit | Impact |
---|---|
Increased Conversion Rates | Up to 30% improvement |
Customer Lifetime Value | 20-40% increase |
Campaign ROI | 2-3x higher than demographic targeting |
By harnessing the power of AI agents for psychographic segmentation, marketers can move beyond simplistic demographic categorizations and tap into the underlying motivations that drive consumer behavior. This shift towards understanding the why behind purchasing decisions, rather than just the who, enables brands to forge deeper emotional connections with their audience, fostering loyalty and driving long-term engagement. As AI technologies continue to evolve, the ability to predict and influence consumer preferences based on psychographic insights will become an indispensable competitive advantage in the marketing landscape.
Predictive Segmentation: Anticipating Customer Needs with AI Agents
AI agents are revolutionizing audience segmentation by leveraging predictive analytics to forecast future customer behaviors and preferences with unprecedented accuracy. These intelligent systems analyze vast datasets encompassing historical purchase patterns, browsing behaviors, demographic information, and external factors to construct dynamic customer profiles. By employing advanced machine learning algorithms such as gradient boosting and neural networks, AI agents can identify subtle correlations and emerging trends that human analysts might overlook. This enables marketers to move beyond reactive strategies and implement proactive campaigns tailored to anticipated customer needs. For instance, an AI agent might predict that a segment of customers is likely to churn within the next 30 days based on declining engagement metrics, triggering personalized retention offers before the customer even considers leaving. Similarly, predictive segmentation can forecast which customers are most likely to respond positively to cross-selling initiatives, with some implementations achieving up to 35% improvement in conversion rates compared to traditional segmentation methods.
Key Capabilities of AI-Driven Predictive Segmentation
Temporal Analysis: AI agents excel at detecting cyclical patterns and seasonal trends, allowing marketers to prepare targeted campaigns months in advance of predicted spikes in demand. Propensity Modeling: By calculating the likelihood of specific customer actions, AI agents can prioritize high-value prospects for personalized outreach. Cohort Discovery: Advanced clustering algorithms uncover previously unknown customer segments with shared characteristics and behaviors, revealing new market opportunities.
Implementation Challenges and Solutions
While the potential of AI-driven predictive segmentation is immense, successful implementation requires overcoming several hurdles:
Data quality and integration remain critical challenges, as AI agents require diverse, high-quality datasets to generate accurate predictions. Organizations must invest in robust data governance frameworks and ETL processes to ensure the integrity of input data.
Additionally, marketers must develop new skills to effectively interpret and act upon AI-generated insights. This often involves close collaboration between data scientists and marketing strategists to translate predictive models into actionable campaign tactics. Despite these challenges, companies that successfully implement AI-driven predictive segmentation can achieve a significant competitive advantage, with some early adopters reporting up to 50% reduction in customer acquisition costs and 20% increase in customer lifetime value.
Integrating AI Agents into Your Marketing Stack: A Step-by-Step Guide
Data Integration and System Compatibility
To successfully implement AI agents for audience segmentation, begin by assessing your existing marketing technology stack. Ensure your Customer Relationship Management (CRM) system, Data Management Platform (DMP), and Marketing Automation tools are compatible with AI integration. Most modern platforms offer APIs or native connectors for seamless data flow. Establish a centralized data lake or warehouse to consolidate customer data from various touchpoints, including website interactions, social media engagement, and purchase history. This unified data repository will serve as the foundation for AI-driven segmentation.
Data Requirements and Preparation
AI agents require high-quality, structured data to perform effectively. Conduct a thorough data audit to identify gaps and inconsistencies. Implement data cleansing processes to remove duplicates, standardize formats, and enrich profiles with third-party data when necessary. Aim for a minimum of 18-24 months of historical data to train your AI models effectively. Ensure compliance with data privacy regulations such as GDPR and CCPA by implementing robust data governance policies and obtaining necessary consent for AI-driven personalization.
AI Model Selection and Training
Choose AI models tailored for marketing segmentation, such as clustering algorithms (e.g., K-means, DBSCAN) or more advanced deep learning models like neural networks. Consider using pre-trained models from reputable AI service providers to accelerate implementation. Allocate sufficient computational resources for model training, which can take several weeks depending on data volume and complexity. Implement a continuous learning pipeline to retrain models periodically, ensuring they adapt to evolving customer behaviors and market trends.
Best Practices for Successful Integration
- Start Small: Begin with a pilot project focusing on a specific segment or campaign to demonstrate ROI before scaling.
- Cross-Functional Collaboration: Involve IT, data science, and marketing teams from the outset to align objectives and technical requirements.
- Real-Time Activation: Implement real-time segmentation capabilities to capitalize on immediate customer intent signals.
- Interpretability: Ensure AI models provide explainable outputs to maintain transparency and trust in decision-making processes.
- Performance Monitoring: Establish KPIs to measure the impact of AI-driven segmentation on marketing performance, customer engagement, and conversion rates.
By following this structured approach, marketers can effectively integrate AI agents into their audience segmentation strategies, unlocking deeper customer insights and driving more personalized, effective campaigns.
Case Studies: Success Stories of AI-Driven Audience Segmentation
Leading companies across industries have leveraged AI agents for audience segmentation, achieving remarkable improvements in marketing performance and ROI. Netflix, a pioneer in content personalization, implemented an AI-driven segmentation system that increased viewer engagement by 32% and reduced churn by 18% within six months. The system analyzes viewing habits, content preferences, and demographic data to create micro-segments, enabling hyper-targeted content recommendations and marketing campaigns. Sephora deployed an AI agent for customer segmentation that boosted conversion rates by 27% and increased average order value by 15%. The AI analyzes purchase history, browsing behavior, and beauty profiles to create dynamic segments, allowing for personalized product recommendations and tailored email campaigns. Financial services giant American Express implemented an AI-powered segmentation tool that improved credit card acquisition rates by 22% and increased customer lifetime value by 8%. The AI agent processes vast amounts of transactional and behavioral data to identify high-value prospects and create targeted acquisition strategies. Spotify‘s AI-driven audience segmentation resulted in a 41% increase in playlist followers and a 31% boost in music discovery, enhancing user engagement and retention. The AI agent analyzes listening patterns, genre preferences, and social connections to create highly personalized playlists and music recommendations for distinct user segments.
Company | Key Metric | Improvement |
---|---|---|
Netflix | Viewer Engagement | 32% increase |
Sephora | Conversion Rate | 27% increase |
American Express | Credit Card Acquisition | 22% increase |
Spotify | Playlist Followers | 41% increase |
These case studies demonstrate the transformative power of AI agents in audience segmentation, enabling businesses to achieve unprecedented levels of personalization, engagement, and marketing efficiency across diverse industries and use cases.
Overcoming Challenges: Addressing Privacy Concerns and Data Quality Issues
Implementing AI agents for audience segmentation in marketing presents significant challenges, particularly in the realms of data privacy and quality. The General Data Protection Regulation (GDPR) and California Consumer Privacy Act (CCPA) have fundamentally altered the landscape of data collection and usage, necessitating robust compliance measures. AI agents must be designed with privacy-by-design principles, incorporating data minimization, purpose limitation, and user consent mechanisms. To ensure data quality, organizations should implement multi-tiered data validation processes, including automated cleansing algorithms and human oversight. A critical step is the establishment of a data governance framework that defines data ownership, access controls, and quality standards across the organization.
Best Practices for Privacy-Compliant AI Segmentation
To navigate these challenges effectively, marketers should adopt the following best practices:
- Implement robust pseudonymization and encryption techniques for personal data
- Conduct regular privacy impact assessments (PIAs) on AI segmentation models
- Develop transparent data usage policies and obtain explicit consent for AI-driven segmentation
- Establish data retention and deletion protocols aligned with regulatory requirements
- Utilize federated learning techniques to minimize centralized data storage
Data Quality Enhancement Strategies
Ensuring high-quality data inputs is paramount for accurate AI-driven segmentation. Consider the following strategies:
- Deploy machine learning algorithms for anomaly detection and data cleansing
- Implement real-time data validation at collection points
- Establish a continuous feedback loop with data sources for ongoing quality improvement
- Utilize data enrichment services to supplement and verify first-party data
- Conduct regular data audits and quality assessments
By addressing these challenges head-on, marketers can harness the full potential of AI agents for audience segmentation while maintaining regulatory compliance and data integrity. This approach not only mitigates risks but also enhances the accuracy and effectiveness of segmentation efforts, leading to more targeted and successful marketing campaigns.
The Future of Audience Segmentation: AI Agents and Hyper-Personalization
AI agents are revolutionizing audience segmentation, ushering in an era of hyper-personalization that promises to transform marketing strategies. These intelligent systems leverage advanced machine learning algorithms, natural language processing, and real-time data analysis to create incredibly granular customer segments. By 2025, AI-driven segmentation is projected to increase marketing ROI by 30% across industries. Predictive analytics enable AI agents to anticipate customer needs with uncanny accuracy, allowing for proactive engagement strategies that boost conversion rates by up to 50%. The integration of multi-modal data sources—including social media interactions, IoT device usage, and biometric responses—enables the creation of dynamic micro-segments that evolve in real-time. This level of precision facilitates true one-to-one marketing at scale, with AI agents autonomously crafting and delivering personalized content, offers, and experiences to millions of individual customers simultaneously.
Emerging Capabilities of AI Segmentation Agents
Next-generation AI agents are incorporating sentiment analysis and emotional intelligence to gauge customer mood and tailor interactions accordingly. These systems can detect subtle shifts in consumer behavior patterns and automatically adjust segmentation models, ensuring marketing efforts remain relevant in rapidly changing markets. Advanced natural language generation capabilities allow AI agents to produce personalized marketing copy and product recommendations that resonate deeply with each customer’s unique preferences and lifecycle stage.
Ethical Considerations and Privacy Safeguards
As AI-driven hyper-personalization becomes more prevalent, marketers must navigate complex ethical considerations. Implementing robust privacy safeguards and transparent data usage policies is crucial to maintaining consumer trust. AI agents are being designed with built-in compliance checks to ensure personalization efforts adhere to regulations like GDPR and CCPA, automatically adjusting strategies based on individual privacy preferences and consent levels.
AI Segmentation Capability | Impact on Marketing Effectiveness |
---|---|
Real-time Micro-segmentation | +40% increase in engagement rates |
Predictive Needs Analysis | 25% reduction in customer churn |
Emotion-aware Personalization | 35% boost in customer lifetime value |
The future of audience segmentation lies in the seamless integration of AI agents across the entire marketing ecosystem. By 2027, it’s estimated that 80% of Fortune 500 companies will employ AI-driven hyper-personalization strategies, creating a new standard for customer engagement that blends
data-driven precision with human-like intuition
. As these technologies mature, marketers who master the art of AI-augmented segmentation will gain a significant competitive edge in the battle for customer attention and loyalty.
Measuring Success: KPIs for AI-Powered Audience Segmentation
To effectively evaluate the performance of AI agents in audience segmentation, marketers must track specific key performance indicators (KPIs) that align with business objectives. Conversion rate uplift serves as a primary metric, typically aiming for a 15-30% improvement over traditional segmentation methods. Segment stability, measured by the percentage of audience members remaining in their assigned segment over time, should exceed 80% for optimal performance. Predictive accuracy, gauged through A/B testing against control groups, should demonstrate at least a 25% increase in targeting precision. Engagement metrics such as click-through rates (CTR) and time-on-site for segmented campaigns should show a minimum 20% boost compared to non-AI methods.
Advanced Performance Metrics
Beyond basic KPIs, sophisticated AI-driven segmentation demands more nuanced evaluation:
- Segment granularity index: Measure the number and specificity of identified micro-segments, aiming for a 3x increase over manual methods.
- Cross-channel consistency score: Evaluate the uniformity of segment behavior across various marketing channels, targeting 90% alignment.
- Algorithmic drift detection: Monitor the rate at which AI models require retraining, with an ideal refresh cycle of 3-6 months.
Optimization Strategies
To continuously enhance AI agent performance:
- Implement a feedback loop mechanism, integrating real-time campaign results to refine segmentation models.
- Conduct regular feature importance analysis to identify and prioritize the most influential audience attributes.
- Utilize ensemble methods, combining multiple AI models to improve overall segmentation accuracy by 10-15%.
- Employ incremental learning techniques to adapt to evolving customer behaviors without full model retraining.
By rigorously tracking these KPIs and implementing data-driven optimization strategies, marketers can maximize the effectiveness of AI-powered audience segmentation, driving substantial improvements in campaign performance and ROI.
FAQ: Everything You Need to Know About AI Agents for Audience Segmentation
What are AI agents for audience segmentation?
AI agents for audience segmentation are advanced machine learning systems that autonomously analyze vast amounts of customer data to identify distinct groups with shared characteristics, behaviors, and preferences. These agents leverage sophisticated algorithms, including clustering techniques, decision trees, and neural networks, to process diverse data sources such as demographic information, purchase history, browsing behavior, and social media interactions. By continuously learning and adapting to new data patterns, AI agents can create highly granular and dynamic audience segments that evolve in real-time, enabling marketers to deliver hyper-personalized content and experiences.
How do AI agents improve traditional audience segmentation methods?
AI agents significantly enhance traditional segmentation approaches by:
- Processing speed: Analyzing millions of data points in seconds, compared to manual methods that can take weeks.
- Accuracy: Identifying nuanced patterns and correlations that human analysts might miss, resulting in more precise segments.
- Scalability: Handling exponentially growing datasets without compromising performance.
- Adaptability: Continuously refining segments based on new data, ensuring relevance in rapidly changing markets.
- Predictive capabilities: Forecasting future customer behaviors and preferences to inform proactive marketing strategies.
What types of data can AI agents use for audience segmentation?
AI agents can process a wide array of structured and unstructured data, including:
- Demographic information (age, gender, location, income)
- Psychographic data (interests, values, lifestyle)
- Behavioral data (purchase history, product usage, website interactions)
- Transactional data (frequency, recency, monetary value)
- Social media activity and sentiment
- Customer service interactions and feedback
- Device usage and technological preferences
- Contextual data (time, location, weather)
- Third-party data from data management platforms (DMPs)
What are the key benefits of using AI agents for audience segmentation?
Implementing AI agents for audience segmentation offers numerous advantages:
- Increased ROI: More targeted campaigns resulting in up to 30% higher conversion rates.
- Enhanced personalization: Tailored messaging that resonates with specific audience segments, improving engagement by up to 50%.
- Improved customer retention: Better understanding of customer needs leading to a 25% increase in customer lifetime value.
- Efficient resource allocation: Focusing marketing efforts on high-value segments, reducing wasted ad spend by up to 40%.
- Real-time optimization: Continuous segment refinement enabling agile marketing strategies.
- Cross-channel consistency: Unified customer profiles across all touchpoints for seamless omnichannel experiences.
- Predictive insights: Anticipating future trends and customer behaviors to inform product development and marketing strategies.
What challenges might businesses face when implementing AI agents for audience segmentation?
While AI agents offer significant benefits, organizations may encounter several challenges:
- Data quality and integration: Ensuring clean, consistent data from disparate sources.
- Privacy and compliance: Adhering to regulations like GDPR and CCPA when collecting and processing customer data.
- Interpretability: Understanding and explaining AI-driven segmentation decisions to stakeholders.
- Skill gap: Acquiring or training talent to manage and interpret AI-driven insights.
- Technology integration: Incorporating AI agents into existing martech stacks and workflows.
- Overreliance on automation: Balancing AI-driven insights with human intuition and creativity.
- Ethical considerations: Ensuring fair and unbiased segmentation practices.
How can businesses measure the success of AI-driven audience segmentation?
To evaluate the effectiveness of AI agents in audience segmentation, businesses should track key performance indicators (KPIs) such as:
- Segment performance: Conversion rates, engagement metrics, and revenue per segment
- Campaign effectiveness: Click-through rates, cost per acquisition, and return on ad spend
- Customer lifetime value (CLV): Increase in average CLV across segments
- Churn rate reduction: Decrease in customer attrition within targeted segments
- Cross-selling and upselling success: Increase in average order value and product adoption rates
- Segmentation accuracy: Precision and recall metrics for predictive models
- Time and resource efficiency: Reduction in manual segmentation efforts and faster time-to-market for campaigns