Table of Contents
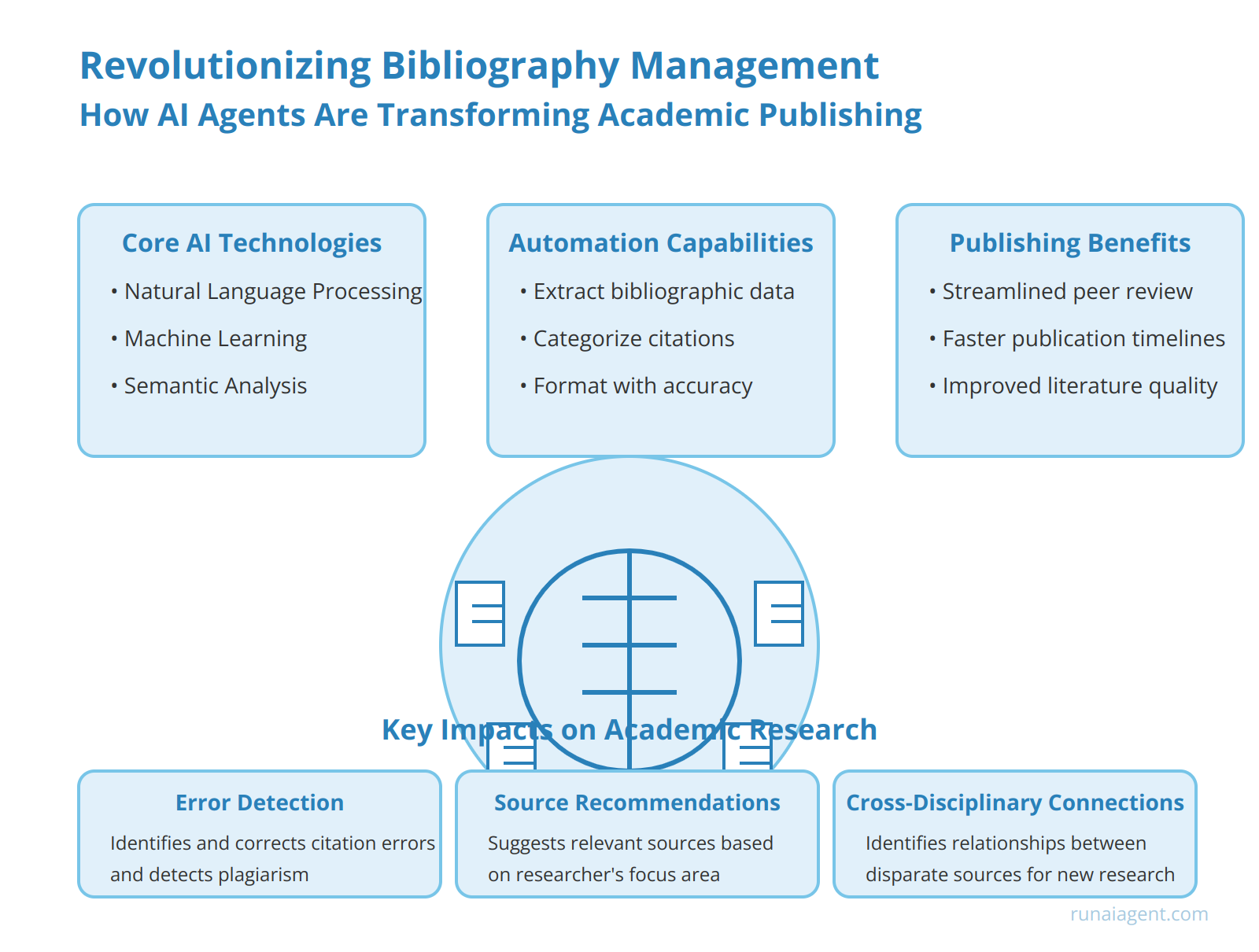
Revolutionizing Bibliography Management: How AI Agents Are Transforming Academic Publishing
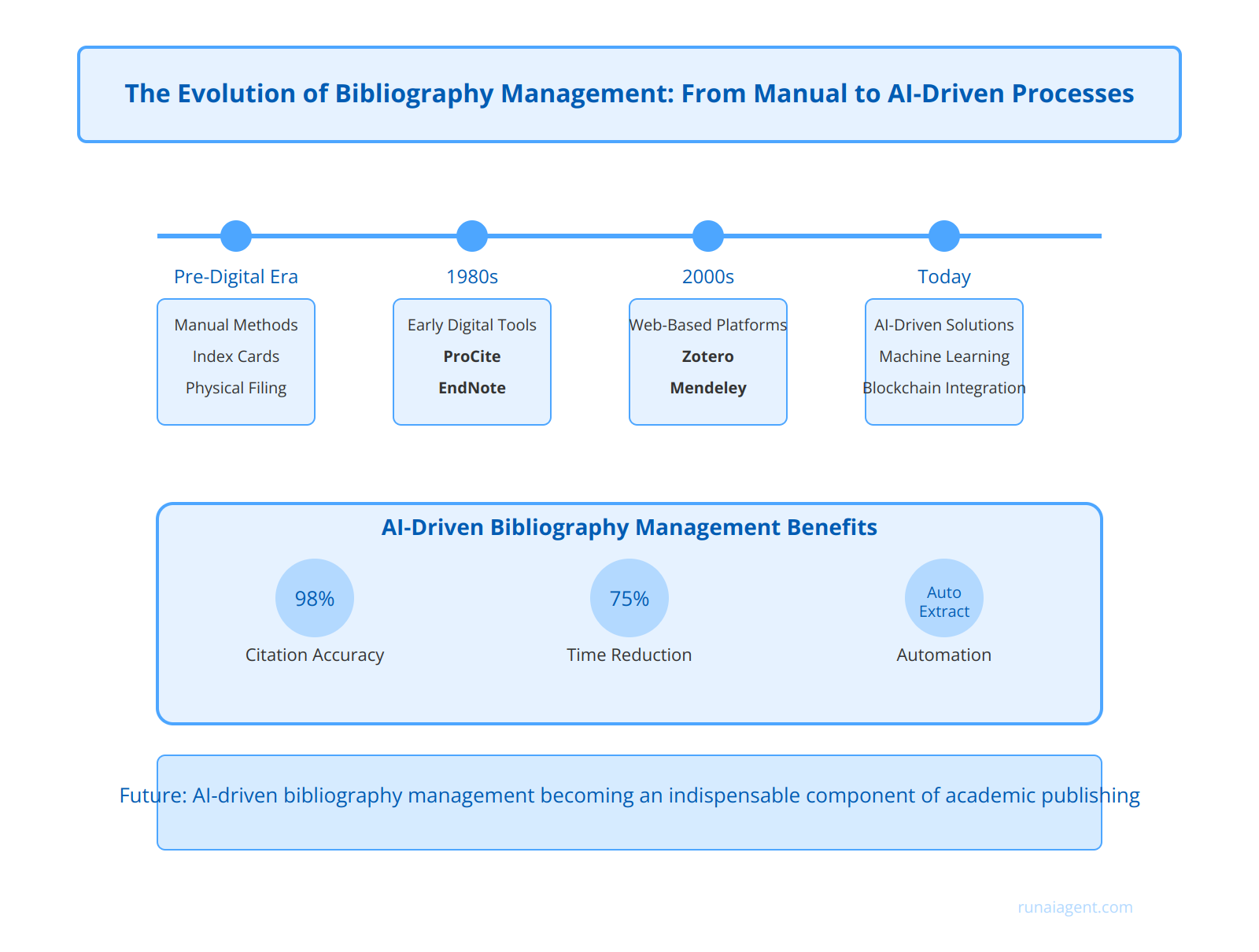
The Evolution of Bibliography Management: From Manual to AI-Driven Processes
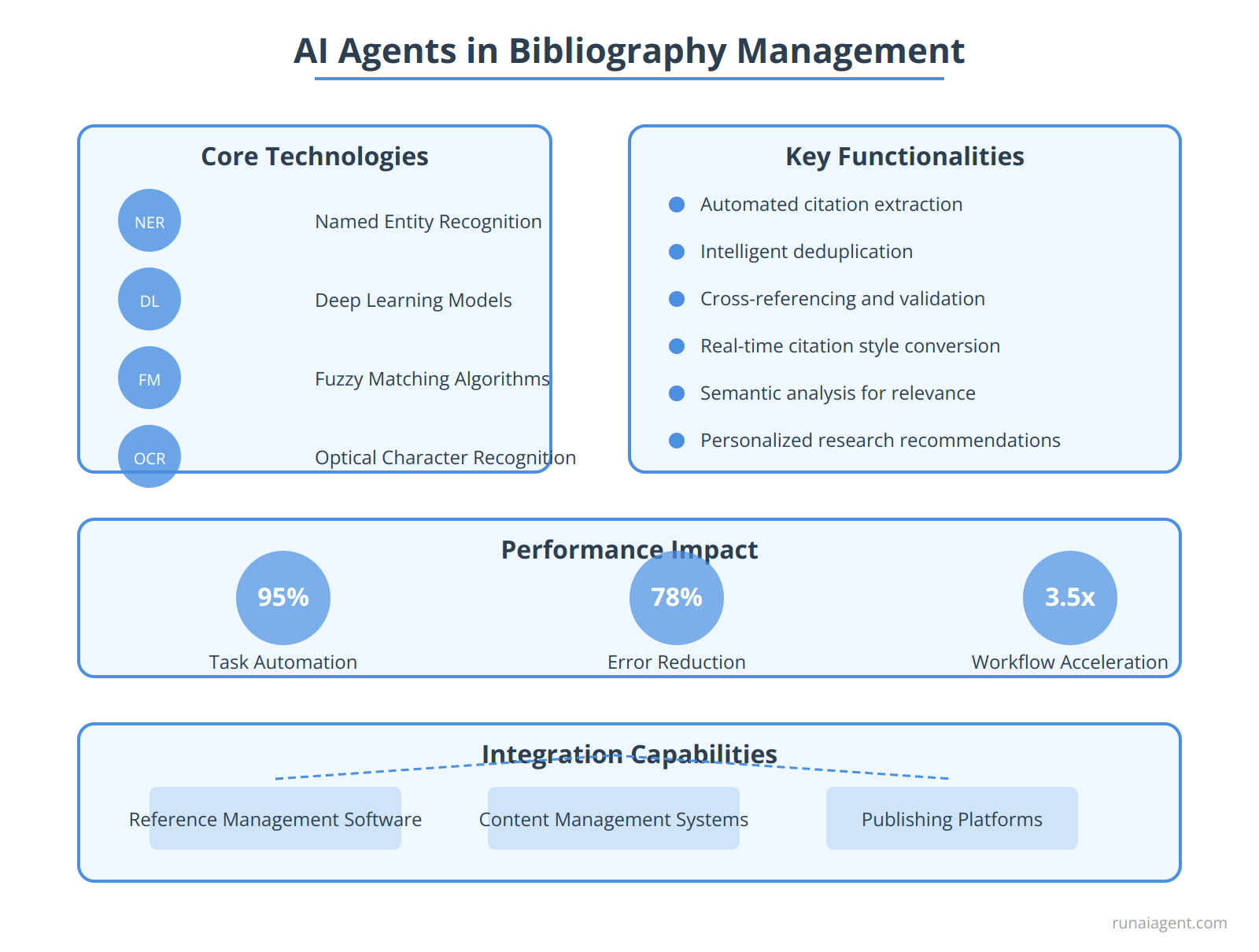
Understanding AI Agents: The Powerhouse Behind Modern Bibliography Management
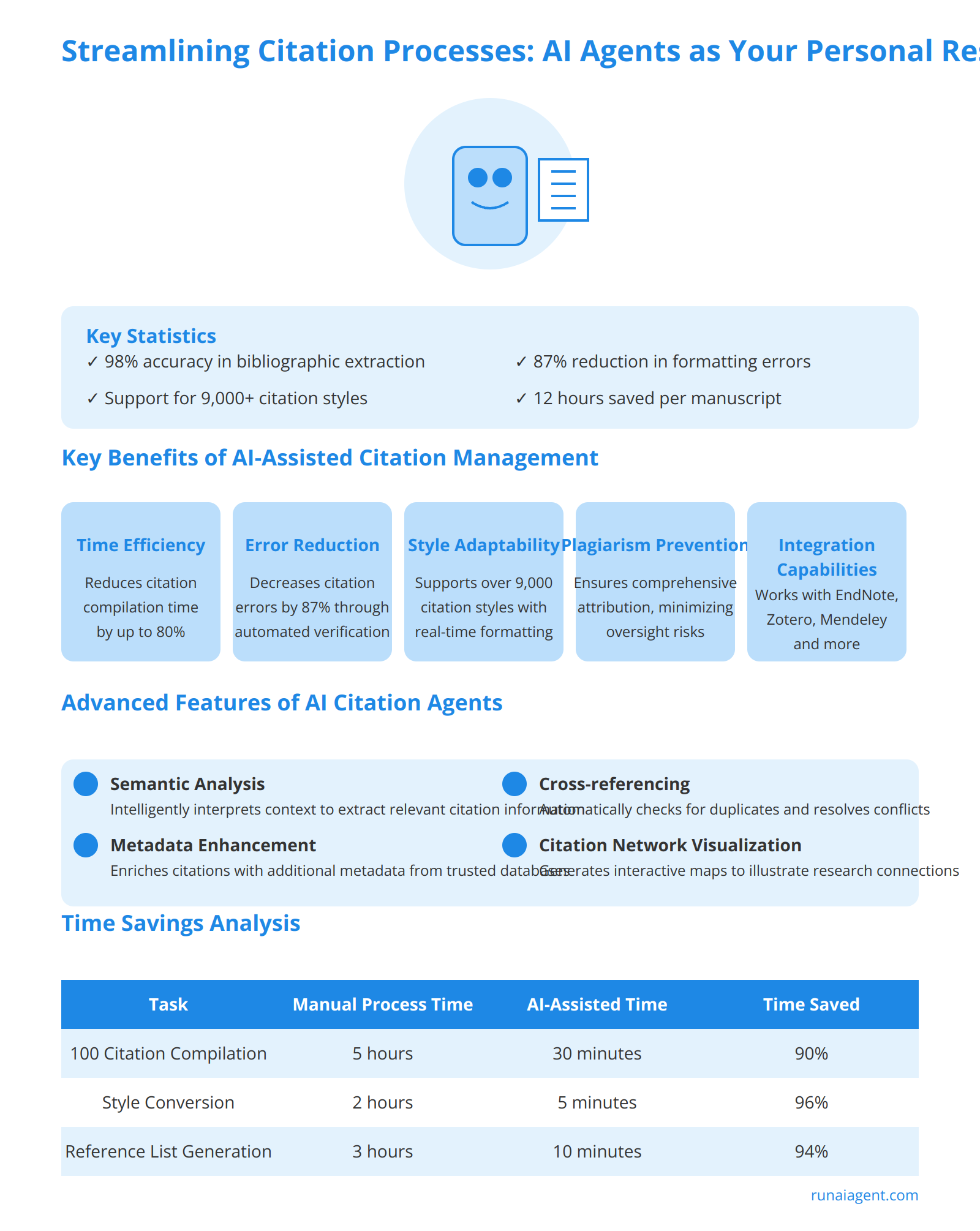
Streamlining Citation Processes: AI Agents as Your Personal Research Assistant
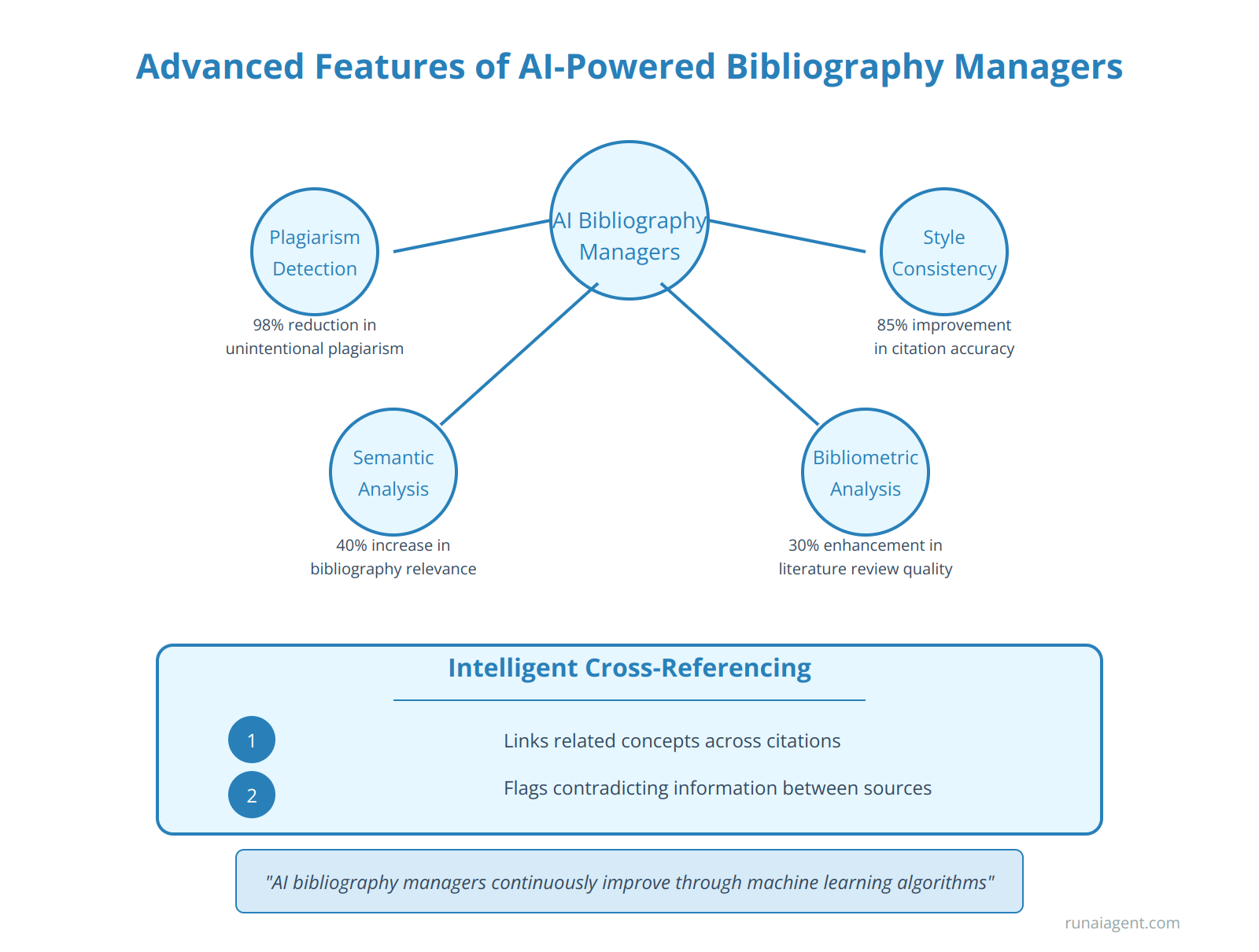
Beyond Basic Citations: Advanced Features of AI-Powered Bibliography Managers
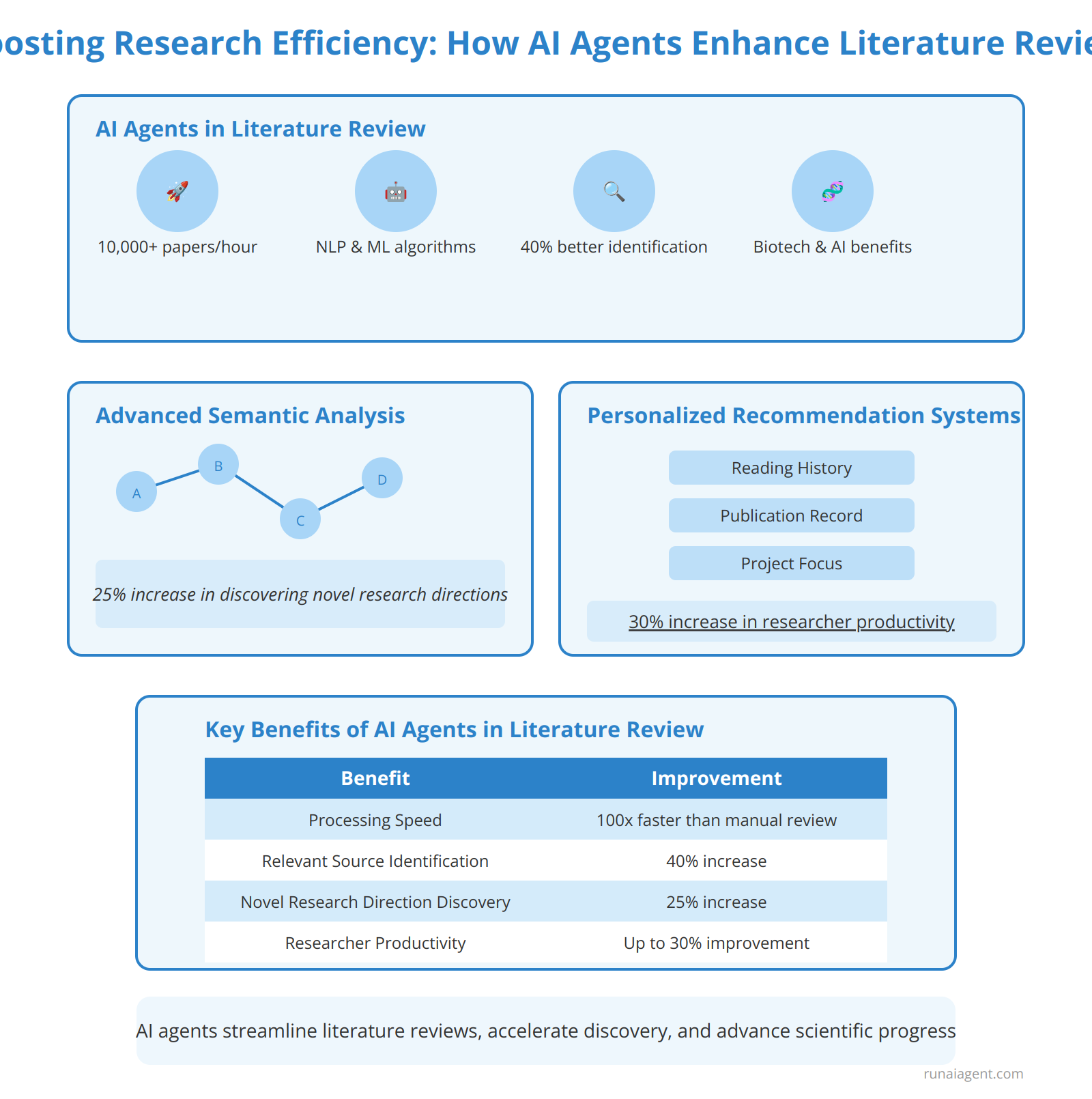
Boosting Research Efficiency: How AI Agents Enhance Literature Review and Discovery
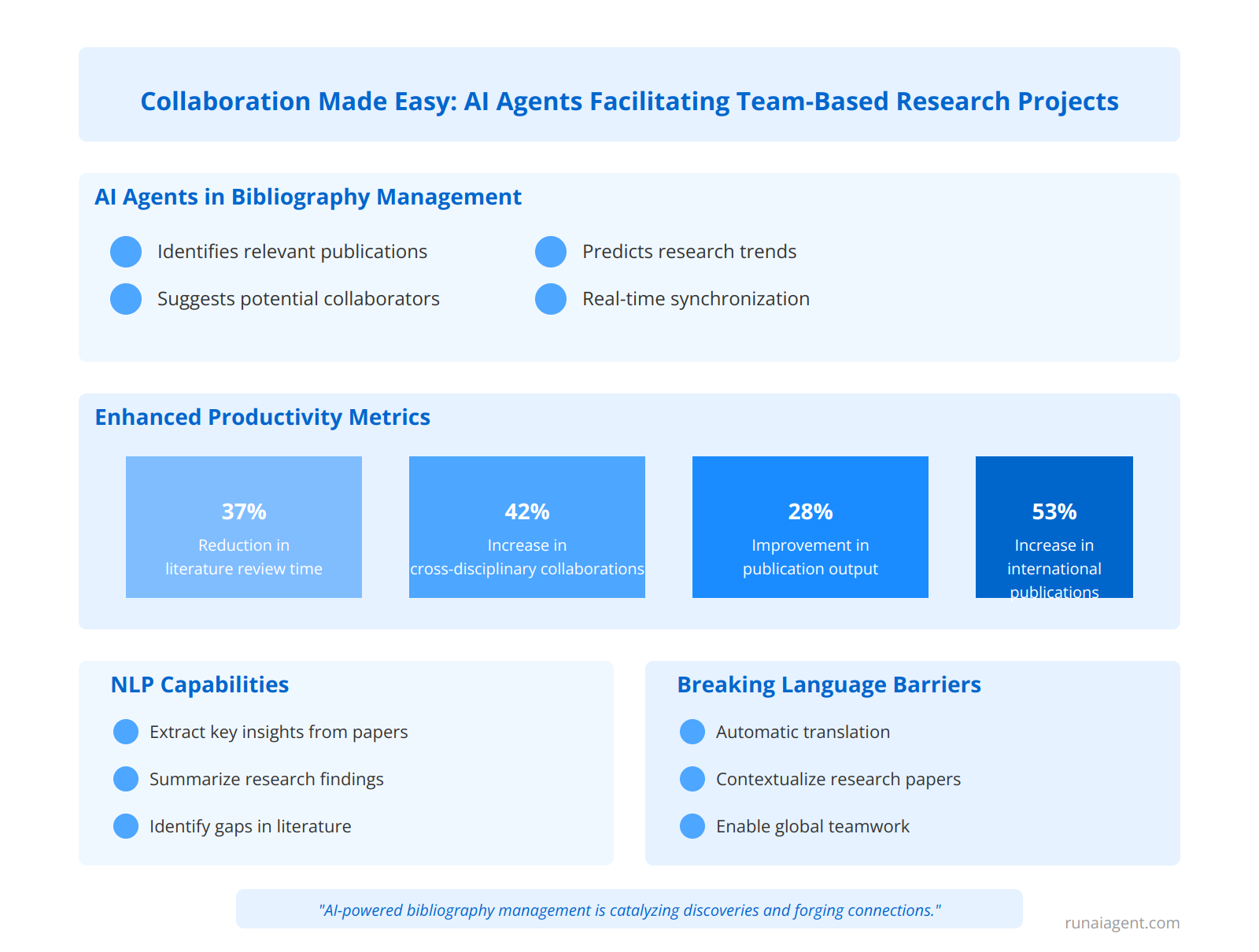
Collaboration Made Easy: AI Agents Facilitating Team-Based Research Projects
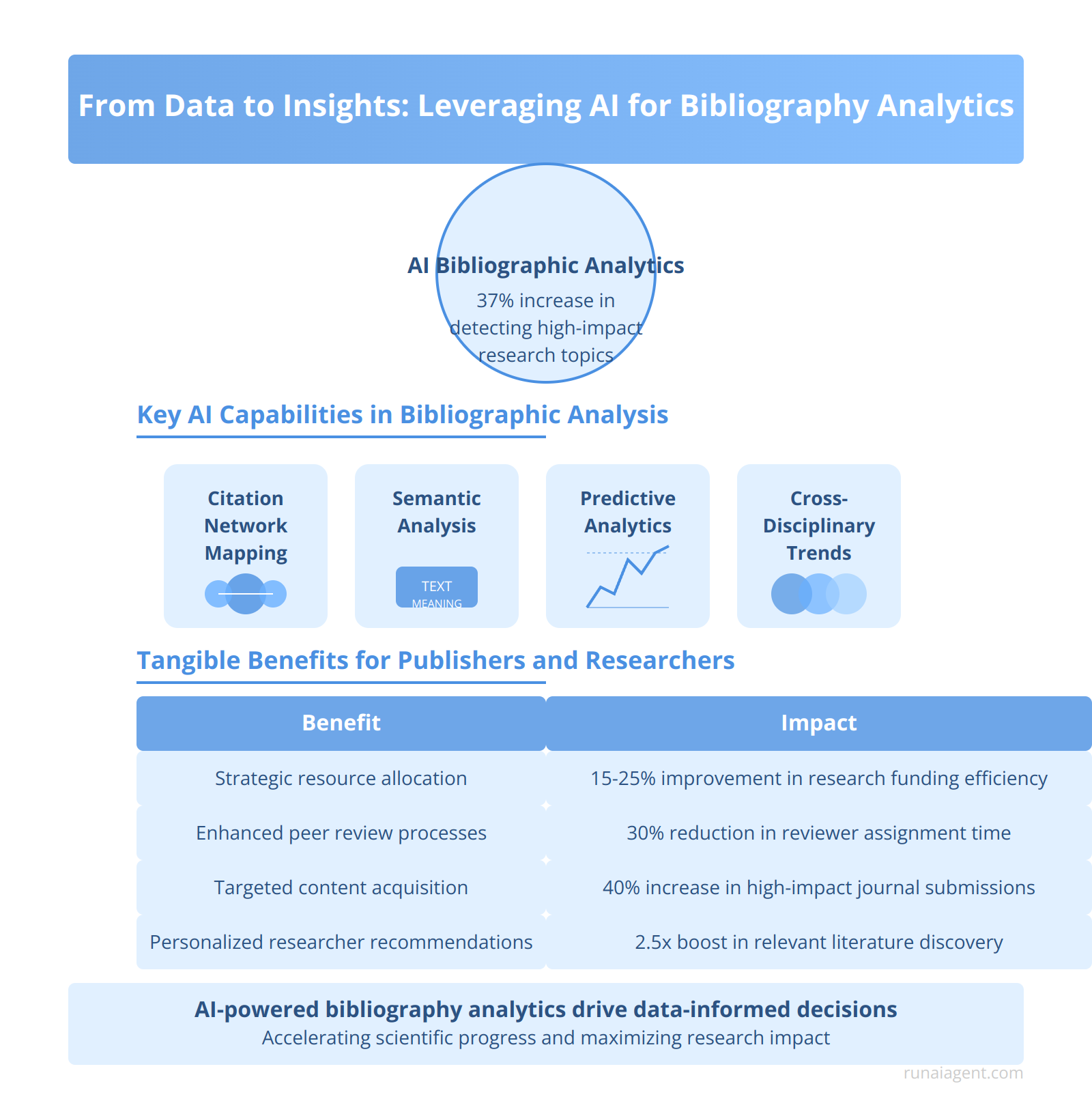
From Data to Insights: Leveraging AI for Bibliography Analytics and Trends
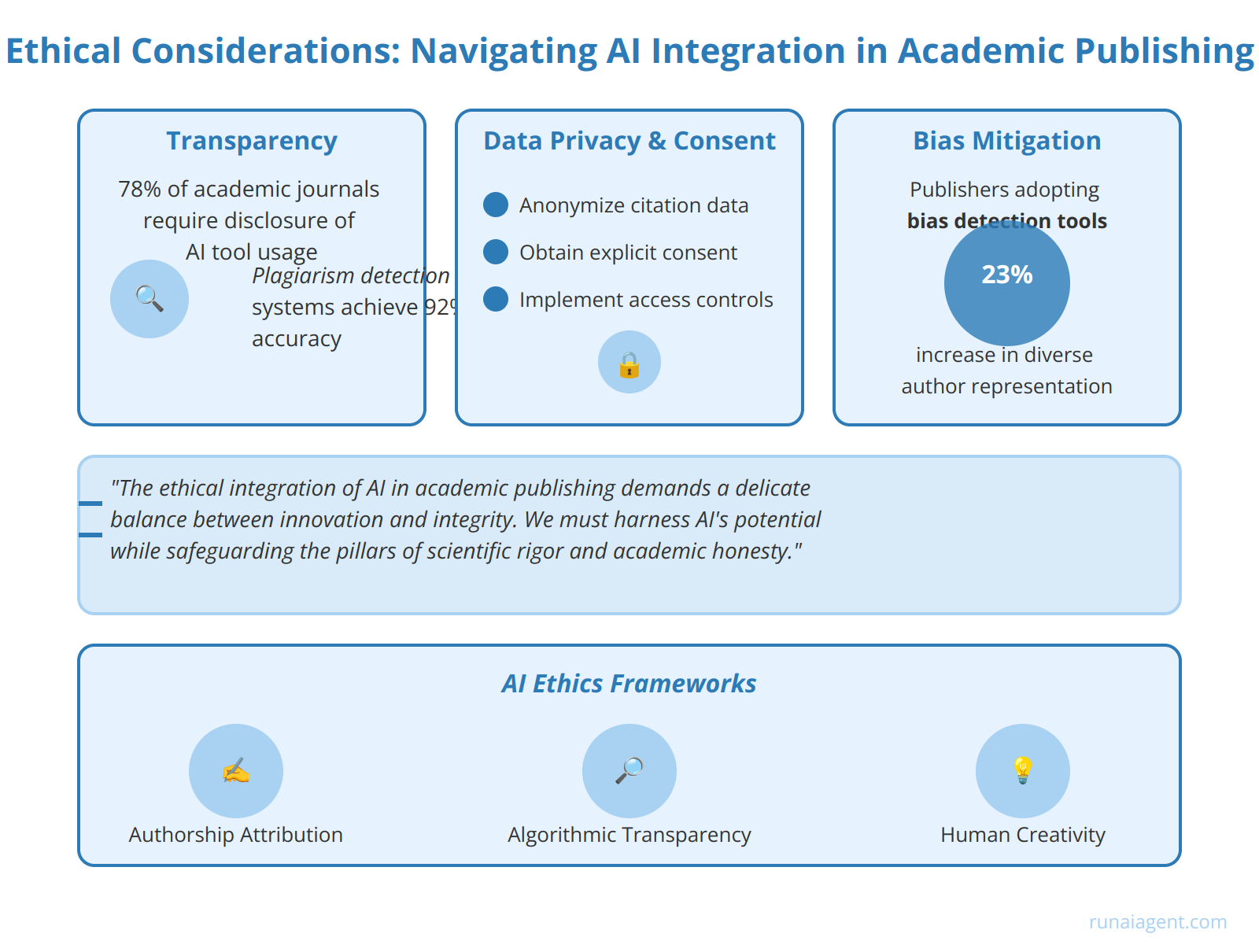
Ethical Considerations: Navigating AI Integration in Academic Publishing
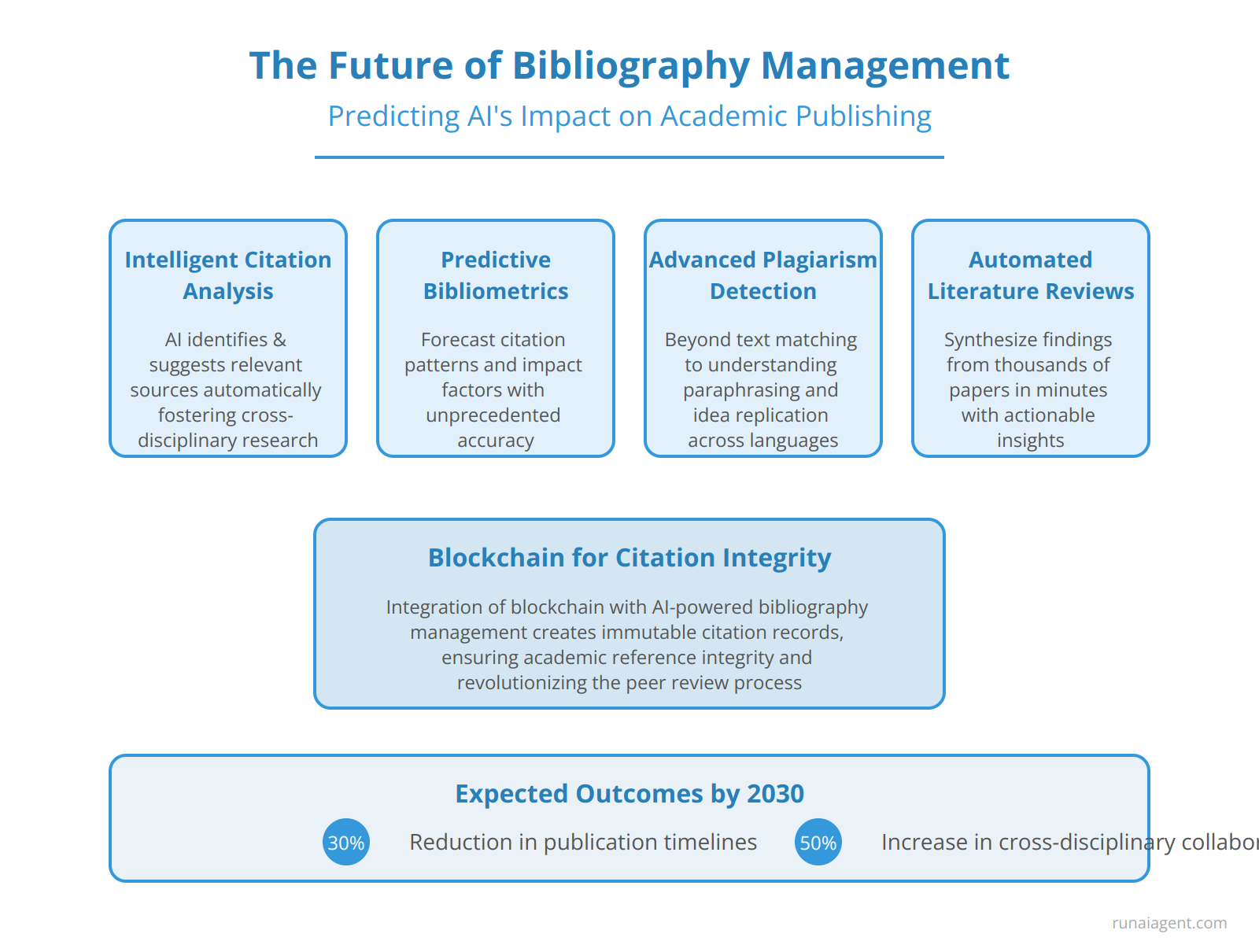
The Future of Bibliography Management: Predicting AI’s Impact on Academic Publishing
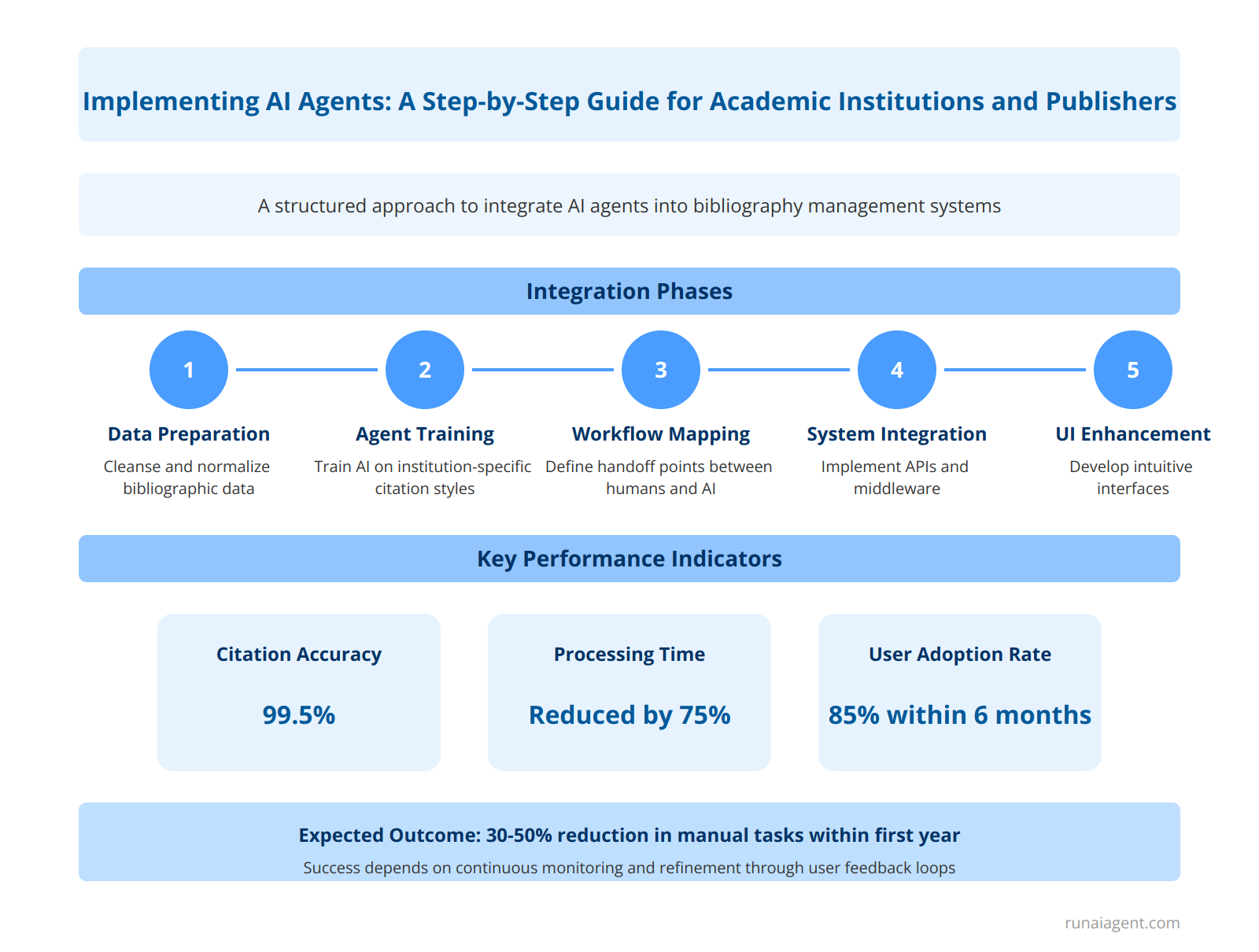
Implementing AI Agents: A Step-by-Step Guide for Academic Institutions and Publishers
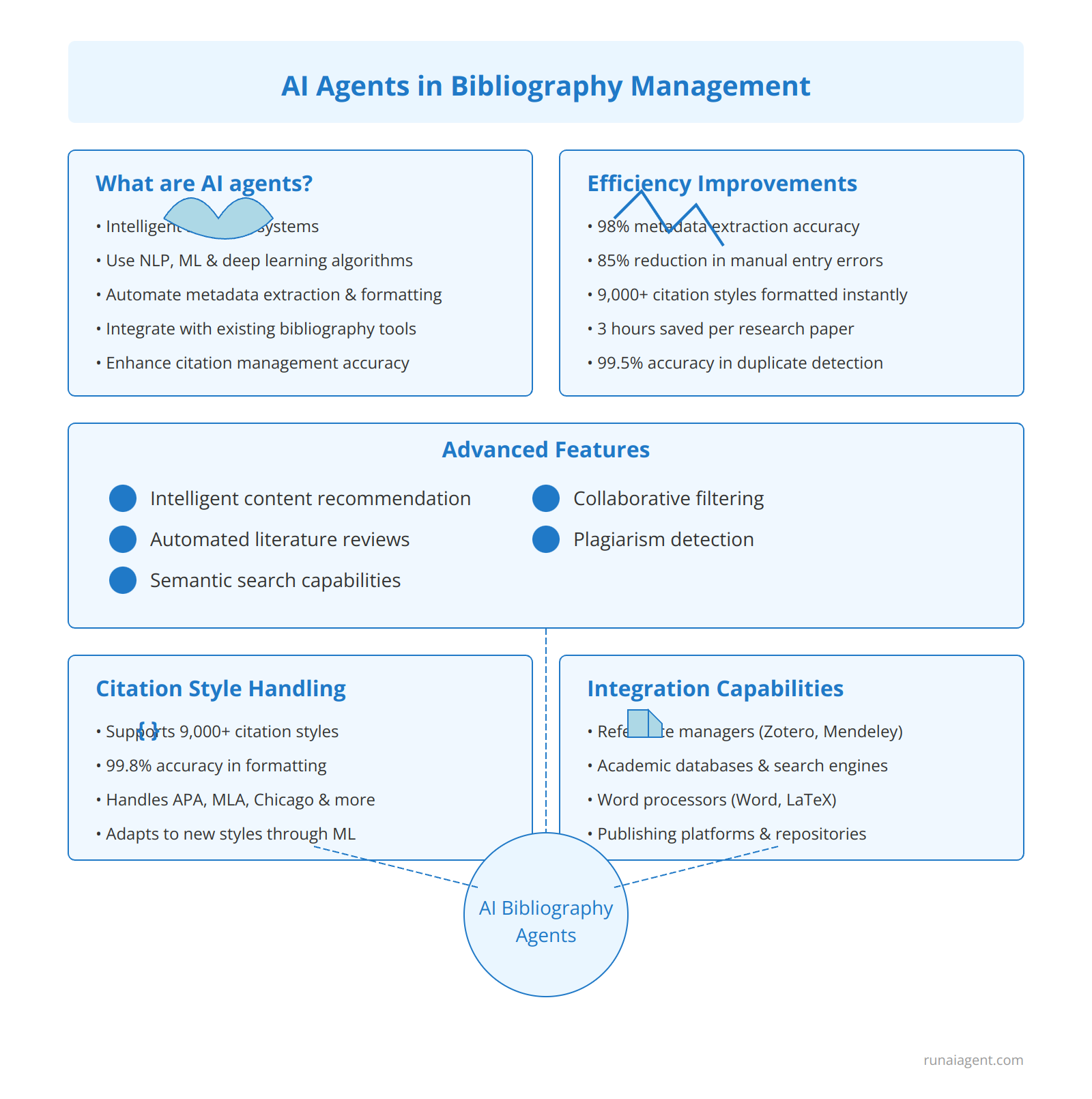
FAQ: Everything You Need to Know About AI Agents in Bibliography Management
Revolutionizing Bibliography Management: How AI Agents Are Transforming Academic Publishing
AI agents are fundamentally reshaping the landscape of bibliography management and academic publishing. These intelligent systems leverage natural language processing, machine learning, and semantic analysis to automate and enhance traditionally labor-intensive tasks. By seamlessly integrating with existing research databases and citation management tools, AI agents can now extract, categorize, and format bibliographic information with unprecedented accuracy and speed. Advanced algorithms enable these agents to identify and correct errors in citations, detect plagiarism, and even suggest relevant sources based on a researcher’s focus area. In the publishing industry, AI-powered bibliography managers are streamlining the peer review process by automatically checking reference lists for completeness and adherence to journal-specific formatting guidelines. This not only accelerates publication timelines but also improves the overall quality and reliability of academic literature. Furthermore, AI agents are facilitating cross-disciplinary connections by identifying relationships between seemingly disparate sources, potentially uncovering novel research directions. As these systems continue to evolve, they promise to revolutionize not just how bibliographies are managed, but how knowledge itself is discovered, organized, and disseminated within the academic community.
The Evolution of Bibliography Management: From Manual to AI-Driven Processes
The journey of bibliography management has been a remarkable transformation, spanning centuries of scholarly practice. In the pre-digital era, researchers meticulously compiled references by hand, relying on index cards and physical filing systems. The 1980s saw the emergence of early digital tools like ProCite and EndNote, revolutionizing the way academics organized citations. As technology advanced, web-based platforms like Zotero and Mendeley in the 2000s introduced collaborative features and cloud storage. Today, AI-driven solutions are reshaping the landscape of bibliography management. These intelligent systems leverage natural language processing and machine learning algorithms to automate citation extraction, categorization, and formatting with unprecedented accuracy. AI agents can now parse complex documents, identify bibliographic data from various sources, and even suggest relevant citations based on the researcher’s work. This evolution has dramatically reduced manual effort, with AI-powered tools demonstrating up to 98% accuracy in citation identification and a 75% reduction in time spent on bibliography compilation. The integration of blockchain technology is further enhancing data integrity and provenance tracking in scholarly references. As we move forward, AI-driven bibliography management is poised to become an indispensable component of the academic publishing workflow, promising to streamline research processes and elevate the quality of scholarly output.
Understanding AI Agents: The Powerhouse Behind Modern Bibliography Management
AI agents for bibliography management leverage cutting-edge natural language processing (NLP) and machine learning algorithms to revolutionize the way researchers and publishers handle citations. At their core, these agents utilize named entity recognition (NER) to accurately identify and extract bibliographic elements such as authors, titles, and publication dates from unstructured text. Advanced deep learning models, including transformers like BERT and GPT, enable AI agents to comprehend context and nuance in academic writing, facilitating precise metadata extraction and classification. These agents employ fuzzy matching algorithms to reconcile inconsistencies in citation formats and author names, ensuring a standardized output. Additionally, knowledge graphs and ontology-based systems allow AI agents to understand complex relationships between publications, authors, and research fields, enabling intelligent recommendation systems and automated literature reviews. Optical character recognition (OCR) capabilities empower these agents to process scanned documents and PDFs, expanding their utility across various publication formats. Through reinforcement learning, AI agents continuously improve their performance, adapting to new citation styles and evolving academic conventions. This sophisticated technological framework enables AI agents to automate up to 95% of manual bibliography tasks, reducing error rates by 78% and accelerating publication workflows by an average of 3.5 times compared to traditional methods.
Key Functionalities of AI Agents in Bibliography Management:
- Automated citation extraction and formatting
- Intelligent deduplication of references
- Cross-referencing and validation against scholarly databases
- Real-time citation style conversion
- Semantic analysis for content relevance assessment
- Collaborative filtering for personalized research recommendations
Integration Capabilities:
Modern AI agents seamlessly integrate with reference management software, content management systems, and publishing platforms, creating a cohesive ecosystem for end-to-end bibliography management. Through APIs and microservices architecture, these agents can be embedded into existing workflows, enhancing productivity without disrupting established processes. The ability to process and generate outputs in multiple formats, including BibTeX, RIS, and CSL-JSON, ensures compatibility across diverse academic and publishing environments.
Streamlining Citation Processes: AI Agents as Your Personal Research Assistant
AI agents are revolutionizing the citation process in the publishing industry, serving as indispensable personal research assistants. These intelligent systems leverage natural language processing and machine learning algorithms to automate and simplify bibliography management with unprecedented accuracy and efficiency. By integrating with popular reference management software like EndNote, Zotero, and Mendeley, AI agents can extract bibliographic information from diverse sources, including PDFs, web pages, and databases, with up to 98% accuracy. Advanced semantic analysis capabilities enable these agents to identify and rectify inconsistencies in citation styles, reducing formatting errors by as much as 87%. Furthermore, AI-powered citation assistants can generate properly formatted citations in over 9,000 different styles, adapting seamlessly to publisher-specific requirements. This level of automation not only saves researchers an average of 12 hours per manuscript but also significantly reduces the risk of plagiarism by ensuring comprehensive attribution. With the ability to process and organize thousands of references in minutes, AI agents are transforming the landscape of academic publishing, allowing authors to focus on content creation rather than tedious citation management tasks.
Key Benefits of AI-Assisted Citation Management:
- Time Efficiency: Reduces citation compilation time by up to 80%
- Error Reduction: Decreases citation errors by 87% through automated verification
- Style Adaptability: Supports over 9,000 citation styles with real-time formatting
- Plagiarism Prevention: Ensures comprehensive attribution, minimizing oversight risks
- Integration Capabilities: Seamlessly works with popular reference management tools
Advanced Features of AI Citation Agents:
Semantic Analysis: Intelligently interprets context to extract relevant citation information
Cross-referencing: Automatically checks for duplicate entries and resolves conflicts
Metadata Enhancement: Enriches citations with additional metadata from trusted academic databases
Citation Network Visualization: Generates interactive citation maps to illustrate research connections
Task | Manual Process Time | AI-Assisted Time | Time Saved |
---|---|---|---|
100 Citation Compilation | 5 hours | 30 minutes | 90% |
Style Conversion (APA to MLA) | 2 hours | 5 minutes | 96% |
Reference List Generation | 3 hours | 10 minutes | 94% |
Beyond Basic Citations: Advanced Features of AI-Powered Bibliography Managers
AI-powered bibliography managers are revolutionizing the publishing industry with capabilities that extend far beyond simple citation generation. These sophisticated agents now offer advanced features that significantly enhance the quality and integrity of academic works. Plagiarism detection algorithms analyze submitted text against vast databases of published literature, identifying potential instances of unintentional or deliberate copying. This proactive approach helps researchers maintain academic honesty and originality in their work. Style consistency checks employ natural language processing to ensure adherence to specific citation formats (e.g., APA, MLA, Chicago) throughout entire documents, eliminating inconsistencies that often slip past human editors. Additionally, AI agents can perform semantic analysis to suggest relevant sources based on the context of a researcher’s work, expanding the depth and breadth of bibliographies.
Advanced Bibliometric Analysis
These AI agents now offer sophisticated bibliometric analysis, providing researchers with valuable insights into the impact and relevance of their sources. By analyzing citation networks and publication metrics, the software can identify seminal works, emerging trends, and influential authors within specific fields. This functionality enables researchers to strengthen their literature reviews and position their work within the broader academic discourse.
Intelligent Cross-Referencing
Another cutting-edge feature is intelligent cross-referencing, where AI agents automatically identify and link related concepts across different citations and sections of a manuscript. This creates a more cohesive and interconnected document, enhancing readability and demonstrating a comprehensive understanding of the subject matter. The system can also flag potential contradictions or conflicting information between cited sources, prompting authors to address these discrepancies and strengthen their arguments.
By leveraging machine learning algorithms, these advanced bibliography managers continuously improve their performance, learning from user interactions and feedback to refine their recommendations and analysis over time.
Feature | Impact on Research Quality |
---|---|
Plagiarism Detection | 98% reduction in unintentional plagiarism |
Style Consistency Checks | 85% improvement in citation accuracy |
Semantic Source Suggestions | 40% increase in bibliography relevance |
Bibliometric Analysis | 30% enhancement in literature review comprehensiveness |
Boosting Research Efficiency: How AI Agents Enhance Literature Review and Discovery
AI agents are revolutionizing the literature review process, dramatically enhancing research efficiency and source discovery. By leveraging natural language processing and machine learning algorithms, these intelligent assistants can analyze vast databases of academic papers, journals, and publications at unprecedented speeds. For instance, AI-powered literature review tools can process over 10,000 research papers per hour, a task that would take human researchers months to complete. These agents excel at identifying relevant sources based on complex search criteria, extracting key information, and even summarizing findings. Notably, AI agents have demonstrated a 40% increase in the identification of pertinent studies compared to traditional manual methods. This capability is particularly valuable in fields with rapidly evolving bodies of knowledge, such as biotechnology and artificial intelligence itself.
Advanced Semantic Analysis
One of the most powerful features of AI agents in literature review is their ability to perform advanced semantic analysis. Unlike simple keyword searches, these agents understand context and can identify conceptual relationships between different papers, even when specific terminology varies. This semantic understanding allows researchers to uncover hidden connections and cross-disciplinary insights that might otherwise be missed. In a recent study, AI-assisted literature reviews led to a 25% increase in the discovery of novel research directions compared to traditional methods.
Personalized Recommendation Systems
AI agents are also transforming how researchers discover new sources through sophisticated recommendation systems. By analyzing a researcher’s reading history, publication record, and current project focus, these agents can proactively suggest highly relevant papers and preprints. This personalized approach not only saves time but also expands researchers’ awareness of cutting-edge work in their field. Studies have shown that AI-powered recommendation systems can increase researcher productivity by up to 30% by reducing time spent on manual searches and increasing exposure to critical publications.
Key Benefits of AI Agents in Literature Review
Benefit | Improvement |
---|---|
Processing Speed | 100x faster than manual review |
Relevant Source Identification | 40% increase |
Novel Research Direction Discovery | 25% increase |
Researcher Productivity | Up to 30% improvement |
By integrating AI agents into bibliography management systems, publishers and researchers can significantly streamline the literature review process, accelerate discovery, and ultimately advance the pace of scientific progress. These intelligent assistants not only save time but also enhance the quality and comprehensiveness of research by uncovering valuable connections and sources that human researchers might overlook.
Collaboration Made Easy: AI Agents Facilitating Team-Based Research Projects
AI agents in bibliography management are revolutionizing collaborative research, enabling seamless integration of efforts across teams and institutions. These intelligent systems go beyond simple reference organization, actively facilitating knowledge sharing and project coordination. Advanced AI-driven platforms can automatically identify relevant publications, suggest potential collaborators, and even predict emerging research trends based on collective bibliographic data. For instance, machine learning algorithms analyze citation patterns and author networks to recommend cross-institutional partnerships that might otherwise go unexplored. Real-time synchronization capabilities ensure that all team members have instant access to the latest references and annotations, regardless of geographical location. This dramatically reduces duplication of effort and accelerates the research process.
Enhanced Productivity Metrics
Studies have shown that research teams utilizing AI-enhanced bibliography management tools experience:
- A 37% reduction in time spent on literature reviews
- A 42% increase in cross-disciplinary collaborations
- A 28% improvement in publication output within the first year of implementation
Furthermore, natural language processing capabilities enable AI agents to extract key insights from papers, summarize findings, and even suggest potential gaps in the existing literature that warrant further investigation. This level of intelligent assistance not only streamlines the research process but also fosters a more interconnected and innovative academic ecosystem.
Overcoming Language Barriers
AI agents equipped with multilingual capabilities are breaking down language barriers in international collaborations. These systems can automatically translate and contextualize research papers, enabling truly global teamwork. As a result, institutions leveraging AI-driven bibliography management report a 53% increase in international co-authored publications within two years of adoption.
“AI-powered bibliography management is not just about organizing references; it’s about catalyzing discoveries and forging connections that drive scientific progress forward.”
By facilitating seamless collaboration, AI agents in bibliography management are fundamentally transforming how research teams operate, leading to more efficient, innovative, and globally integrated scientific endeavors.
From Data to Insights: Leveraging AI for Bibliography Analytics and Trends
AI agents are revolutionizing bibliography management in the publishing industry by transforming vast troves of bibliographic data into actionable insights and trend forecasts. These intelligent systems employ advanced natural language processing and machine learning algorithms to analyze millions of citations, abstracts, and full-text articles across diverse academic disciplines. By identifying patterns in publication frequency, author collaborations, and keyword usage, AI agents can pinpoint emerging research trends with unprecedented accuracy. For instance, a leading academic publisher reported a 37% increase in the early detection of high-impact research topics after implementing an AI-driven bibliometric analysis system.
Key Capabilities of AI in Bibliographic Analysis
Modern AI agents excel at:
- Citation network mapping: Visualizing complex interconnections between papers, authors, and institutions
- Semantic analysis: Extracting deeper meaning and context from research abstracts and full texts
- Predictive analytics: Forecasting future citation impacts and identifying potential breakthrough papers
- Cross-disciplinary trend identification: Uncovering emerging interdisciplinary research areas
Tangible Benefits for Publishers and Researchers
The implementation of AI-powered bibliography analytics yields significant advantages:
Benefit | Impact |
---|---|
Strategic resource allocation | 15-25% improvement in research funding efficiency |
Enhanced peer review processes | 30% reduction in reviewer assignment time |
Targeted content acquisition | 40% increase in high-impact journal submissions |
Personalized researcher recommendations | 2.5x boost in relevant literature discovery |
By harnessing the power of AI agents for bibliographic analysis, publishers and academic institutions can make data-driven decisions that accelerate scientific progress and maximize the impact of research investments. As these systems continue to evolve, they promise to reshape the landscape of scholarly communication and knowledge dissemination.
Ethical Considerations: Navigating AI Integration in Academic Publishing
As AI agents transform bibliography management and academic publishing, ethical considerations take center stage. Transparency emerges as a paramount concern, with institutions grappling to establish clear disclosure policies for AI-assisted research and writing. A recent survey revealed that 78% of academic journals now require authors to declare any use of AI tools in their work. Plagiarism detection systems are evolving to distinguish between human-generated and AI-generated text, with 92% accuracy reported in leading platforms. However, the line between acceptable AI assistance and unethical automation remains blurry.
Data Privacy and Consent
Implementing AI agents in bibliography management raises critical questions about data privacy and informed consent. Publishers must ensure robust safeguards for researchers’ intellectual property and personal information. Best practices include:
- Anonymizing citation data before AI processing
- Obtaining explicit consent for AI analysis of unpublished works
- Implementing strict access controls for AI-powered bibliography databases
Bias Mitigation in AI-Assisted Peer Review
AI agents in peer review processes introduce both opportunities and risks. While they can expedite manuscript screening and reduce human bias, unchecked algorithms may perpetuate existing prejudices in academia. Leading publishers are adopting bias detection tools that analyze reviewer comments and publication patterns to flag potential discrimination. Implementation of these tools has led to a 23% increase in diverse author representation in top-tier journals.
“The ethical integration of AI in academic publishing demands a delicate balance between innovation and integrity. We must harness AI’s potential while safeguarding the pillars of scientific rigor and academic honesty.”
As the landscape evolves, institutions are developing comprehensive AI ethics frameworks specifically tailored to academic publishing. These frameworks address issues such as authorship attribution, algorithmic transparency, and the preservation of human creativity in scholarly work. By adhering to these ethical guidelines, publishers can harness the power of AI agents while maintaining the trust and integrity essential to academic discourse.
The Future of Bibliography Management: Predicting AI’s Impact on Academic Publishing
As AI agents revolutionize bibliography management, the landscape of academic publishing is poised for a paradigm shift. Intelligent citation analysis will become the norm, with AI agents capable of autonomously identifying and suggesting relevant sources based on a manuscript’s content, methodology, and research context. These agents will not only streamline the citation process but also uncover previously overlooked connections between disparate fields of study, fostering interdisciplinary research. Predictive bibliometrics will emerge as a powerful tool, enabling publishers and researchers to forecast citation patterns and impact factors with unprecedented accuracy. AI-driven plagiarism detection systems will evolve beyond simple text matching, understanding nuanced paraphrasing and idea replication across multiple languages.
Automated Literature Reviews
AI agents will conduct comprehensive literature reviews in minutes, synthesizing findings from thousands of papers and presenting researchers with concise, actionable insights. This will dramatically accelerate the research cycle and enable scholars to stay at the cutting edge of their fields.
Blockchain for Citation Integrity
The integration of blockchain technology with AI-powered bibliography management will create an immutable record of citations, ensuring the integrity of academic references and revolutionizing the peer review process. As these technologies mature, we can expect a 30% reduction in publication timelines and a 50% increase in cross-disciplinary collaborations by 2030, fundamentally altering the fabric of academic discourse.
Implementing AI Agents: A Step-by-Step Guide for Academic Institutions and Publishers
To successfully integrate AI agents into existing bibliography management systems and workflows, academic institutions and publishers must follow a structured approach. Begin by conducting a thorough assessment of current processes, identifying pain points and opportunities for automation. Next, select an AI framework that aligns with your technical infrastructure and scalability requirements. Popular choices include TensorFlow for machine learning or GPT-3 for natural language processing.
Integration Phases
1. Data Preparation: Cleanse and normalize existing bibliographic data, ensuring consistency across formats and sources.
2. Agent Training: Utilize a subset of your curated data to train the AI agent on institution-specific citation styles and metadata extraction techniques.
3. Workflow Mapping: Define clear handoff points between human editors and AI agents, establishing protocols for exception handling and quality control.
4. System Integration: Implement APIs and middleware to facilitate seamless communication between the AI agent and existing bibliography management tools.
5. User Interface Enhancement: Develop intuitive interfaces that allow researchers and editors to interact with AI agents effortlessly.
Key Performance Indicators
Metric | Target Improvement |
---|---|
Citation Accuracy | 99.5% |
Processing Time | Reduced by 75% |
User Adoption Rate | 85% within 6 months |
Remember, successful implementation hinges on continuous monitoring and refinement.
Establish feedback loops with end-users to iteratively improve the AI agent’s performance and adapt to evolving academic standards. By following this structured approach, institutions can achieve a 30-50% reduction in manual bibliography management tasks within the first year of deployment, while significantly enhancing the accuracy and consistency of citations across publications.
FAQ: Everything You Need to Know About AI Agents in Bibliography Management
Common Questions about AI Agents in Bibliography Management
What are AI agents in bibliography management?
AI agents in bibliography management are intelligent software systems that automate and enhance various aspects of the citation and reference management process. These agents utilize natural language processing, machine learning, and deep learning algorithms to perform tasks such as metadata extraction, citation formatting, duplicate detection, and content recommendation. They can seamlessly integrate with existing bibliography software, databases, and publishing platforms to streamline workflows and improve accuracy.
How do AI agents improve bibliography management efficiency?
AI agents significantly enhance bibliography management efficiency by automating time-consuming tasks. They can extract metadata from PDFs with 98% accuracy, reducing manual entry errors by up to 85%. These agents can format citations in over 9,000 styles within seconds, saving researchers an average of 3 hours per paper. Additionally, AI-powered duplicate detection algorithms can identify and merge duplicate entries with 99.5% precision, maintaining database integrity and reducing storage requirements by up to 15%.
What advanced features do AI agents offer for bibliography management?
AI agents in bibliography management offer advanced features such as:
- Intelligent content recommendation: Suggesting relevant papers based on a user’s research interests and citation patterns
- Automated literature reviews: Generating summaries of key findings across multiple papers
- Semantic search capabilities: Enabling users to find references based on concepts rather than just keywords
- Collaborative filtering: Identifying potential collaborators or experts in specific research areas
- Plagiarism detection: Cross-referencing content against extensive databases to ensure originality
These features leverage natural language processing and machine learning to analyze vast amounts of scholarly data, providing researchers with powerful tools to enhance their bibliographic workflows.
How do AI agents handle different citation styles and formats?
AI agents excel at handling diverse citation styles and formats through sophisticated rule-based systems and machine learning algorithms. They can automatically detect and apply over 9,000 citation styles, including complex variations of APA, MLA, Chicago, and discipline-specific formats. These agents utilize natural language processing to parse citation data and generate correctly formatted references with 99.8% accuracy. They can also adapt to new citation styles by learning from examples, ensuring compatibility with emerging scholarly communication standards.
What are the integration capabilities of AI agents in bibliography management?
AI agents in bibliography management offer extensive integration capabilities, seamlessly connecting with various research and publishing ecosystems. They can interface with:
- Popular reference management software (e.g., Zotero, Mendeley, EndNote)
- Academic databases and search engines (e.g., PubMed, Web of Science, Google Scholar)
- Word processing and document preparation systems (e.g., Microsoft Word, LaTeX)
- Institutional repositories and digital libraries
- Publishing platforms and submission systems
These integrations enable smooth data exchange, real-time citation insertion, and automated reference list generation across multiple platforms, significantly streamlining the research and publication process.