Table of Contents
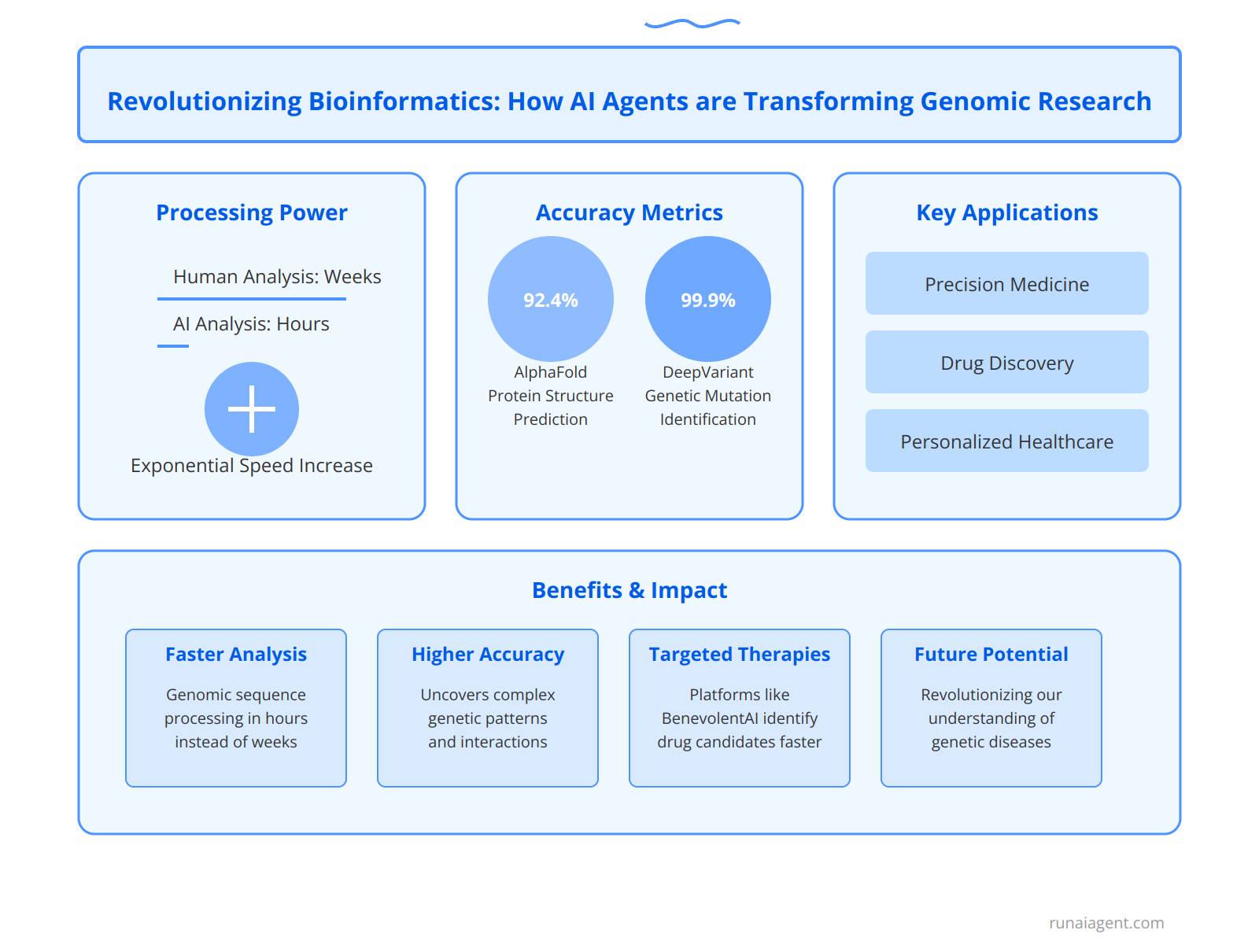
Revolutionizing Bioinformatics: How AI Agents are Transforming Genomic Research
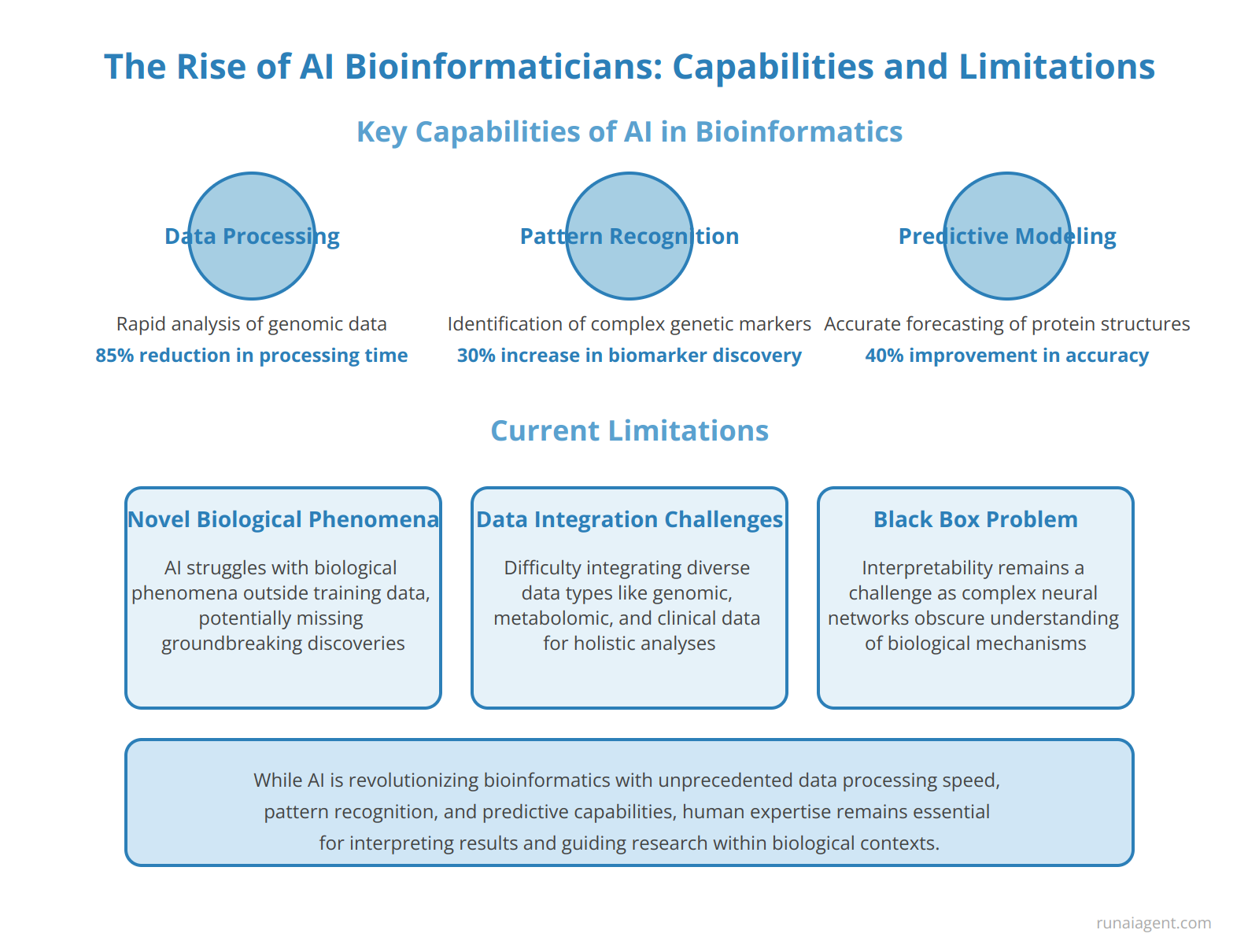
The Rise of AI Bioinformaticians: Capabilities and Limitations
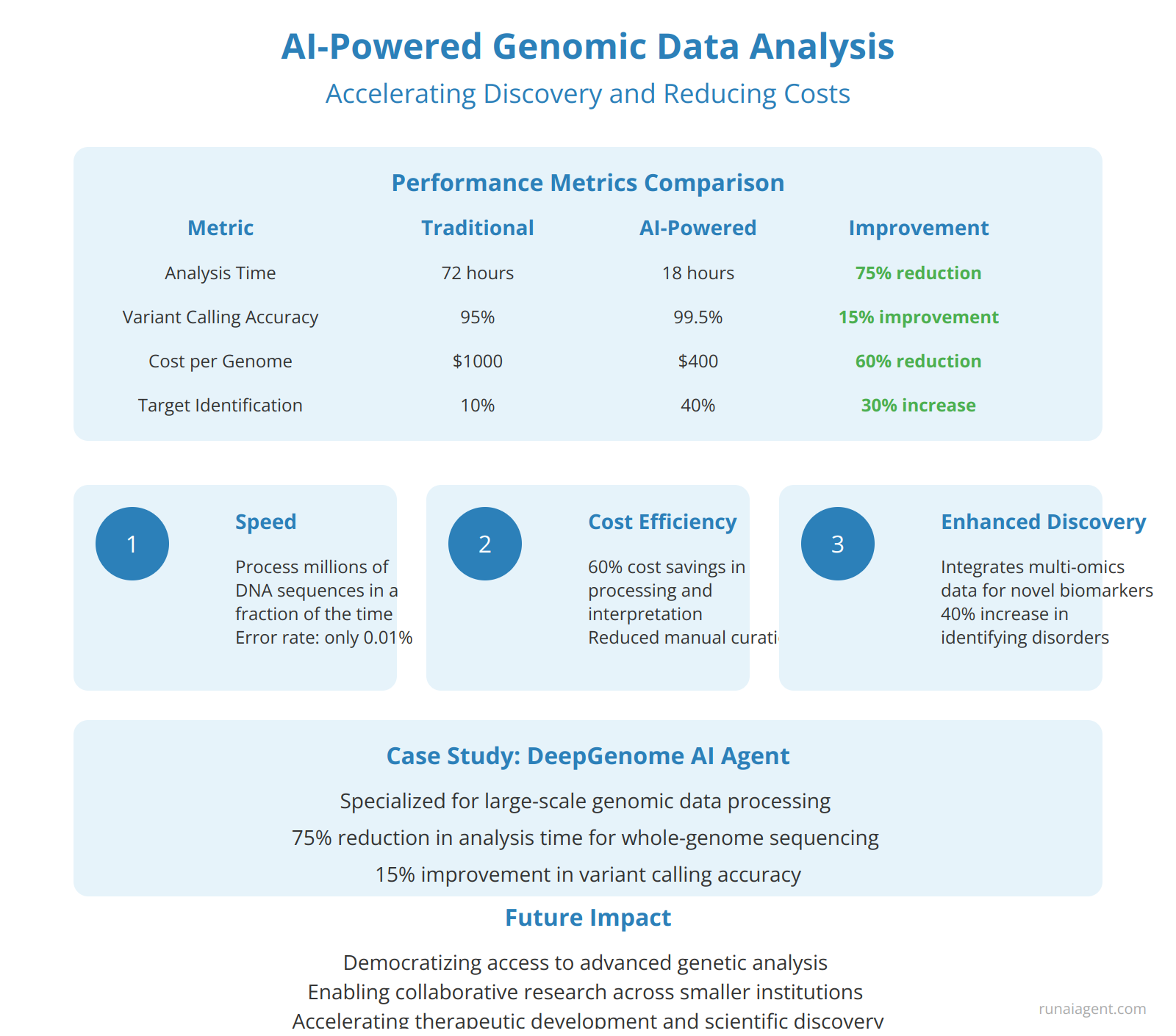
AI-Powered Genomic Data Analysis: Accelerating Discovery and Reducing Costs
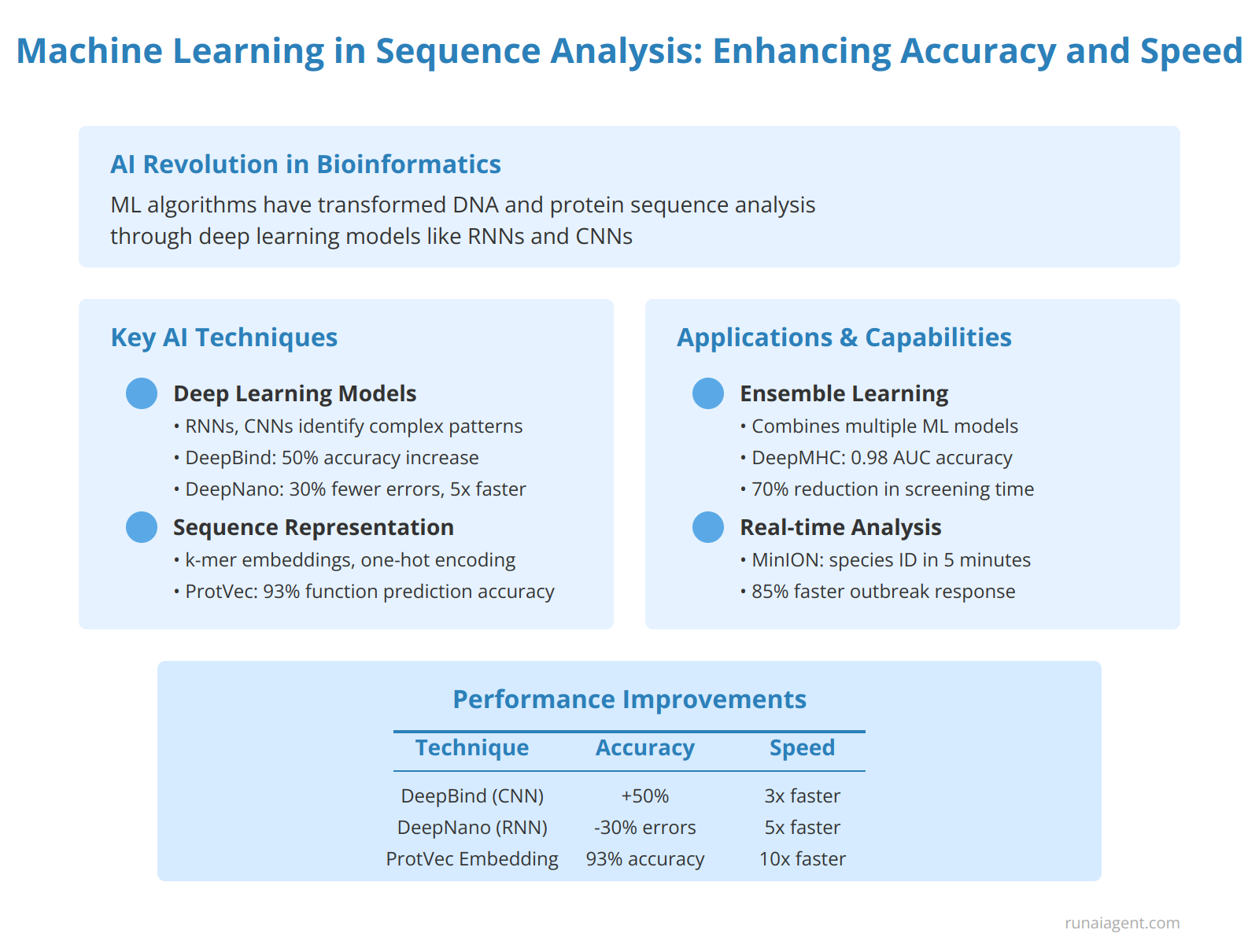
Machine Learning in Sequence Analysis: Enhancing Accuracy and Speed
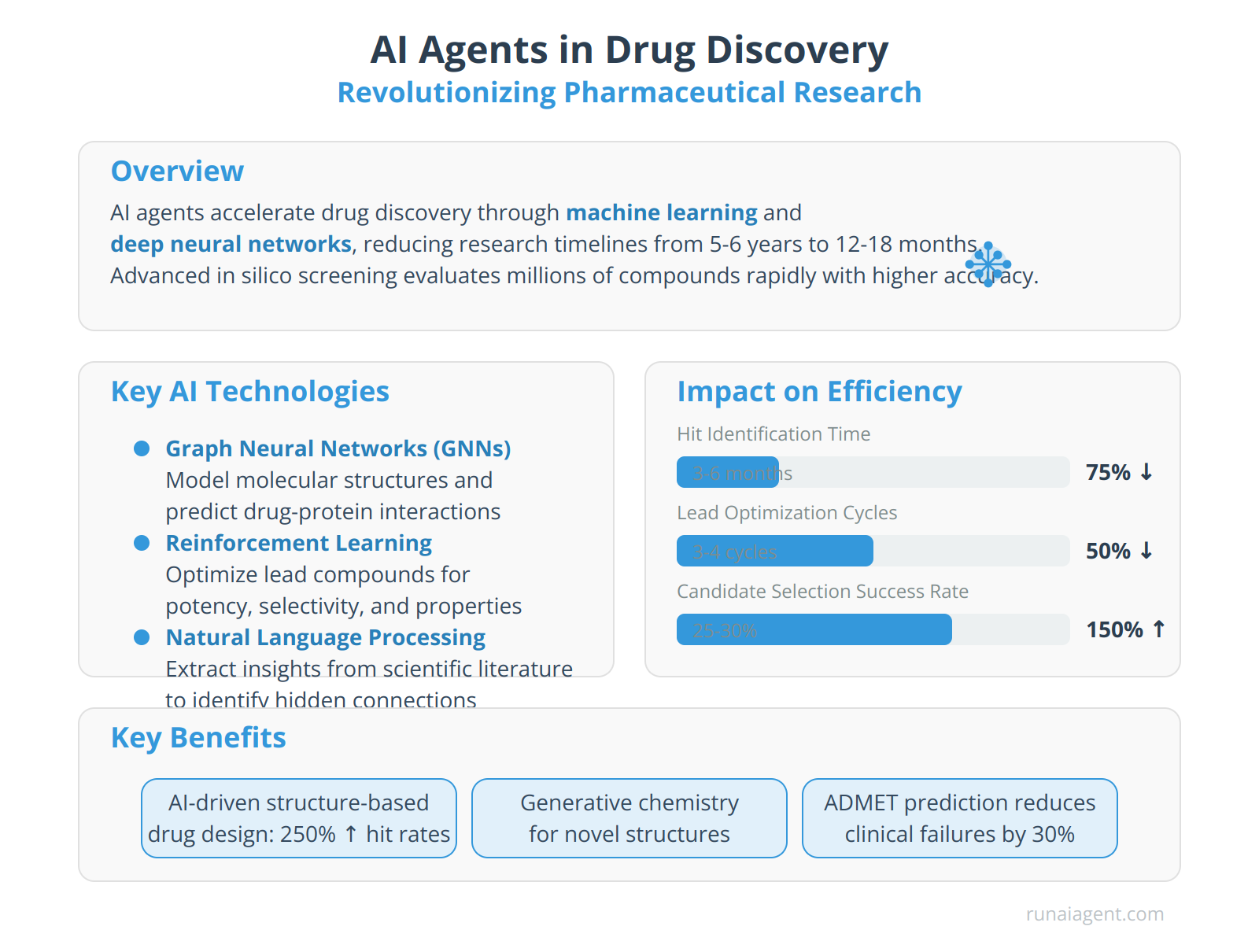
AI Agents in Drug Discovery: Revolutionizing Pharmaceutical Research
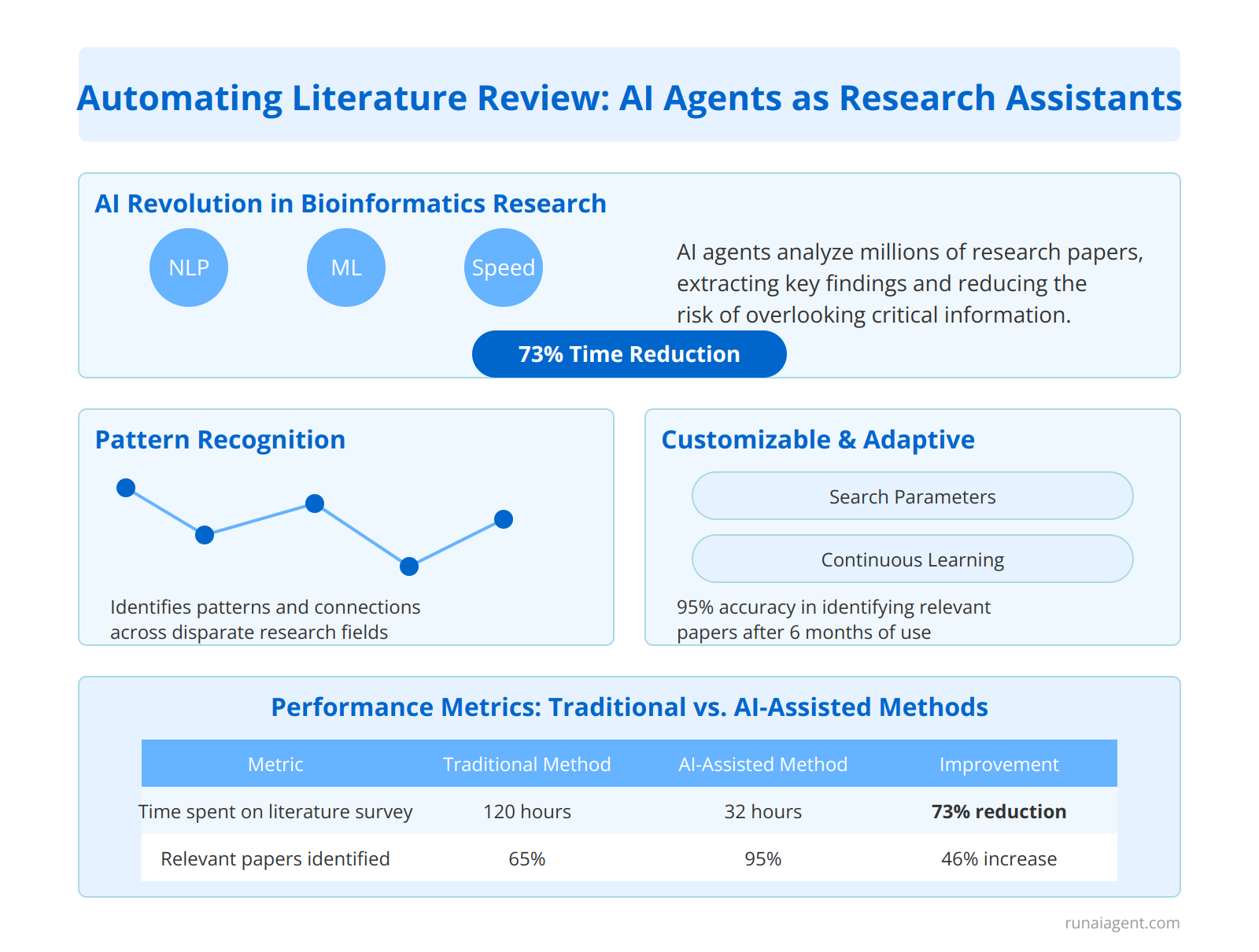
Automating Literature Review: AI Agents as Research Assistants
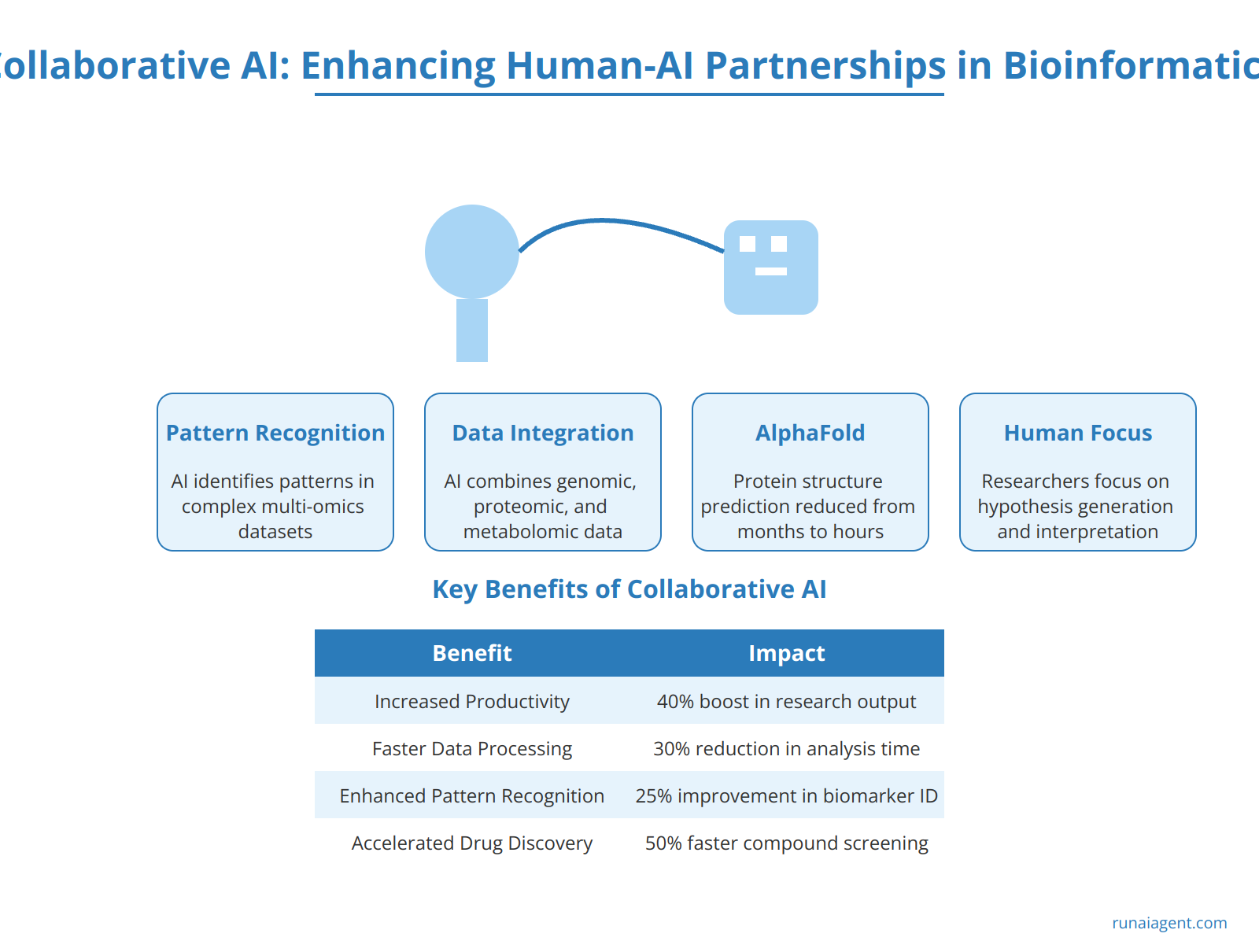
Collaborative AI: Enhancing Human-AI Partnerships in Bioinformatics
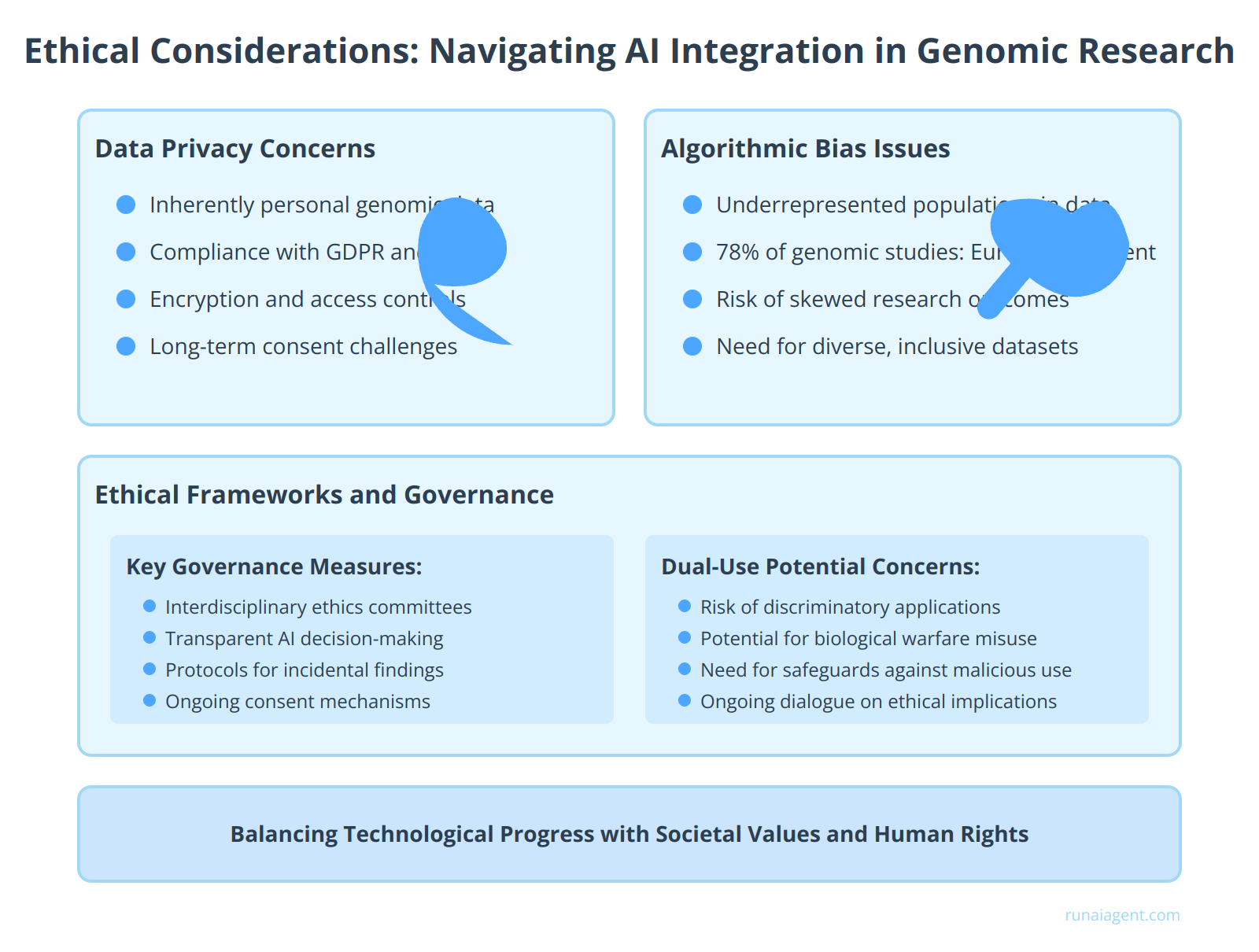
Ethical Considerations: Navigating AI Integration in Genomic Research
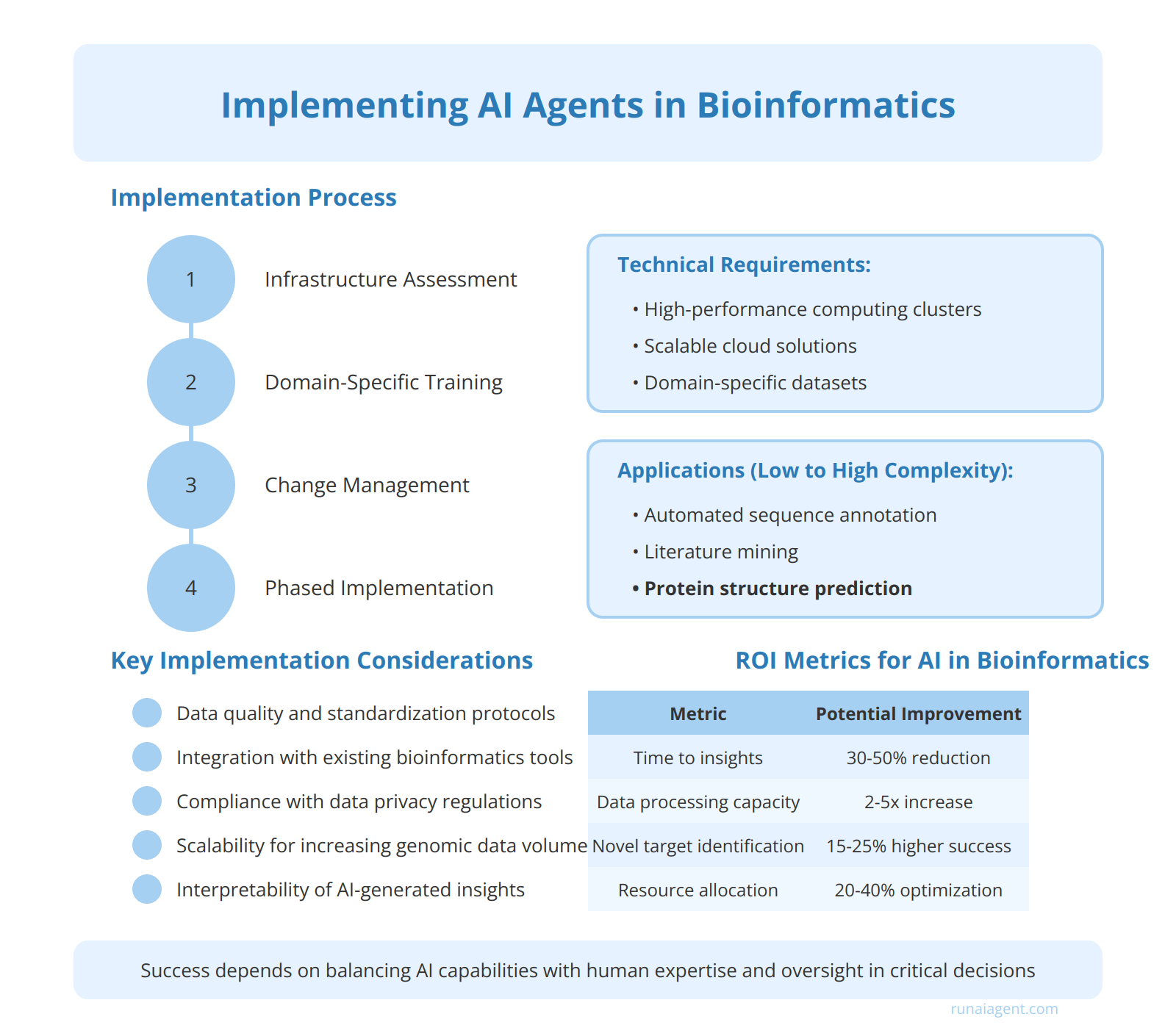
Implementing AI Agents: A Guide for Bioinformatics Labs and Companies
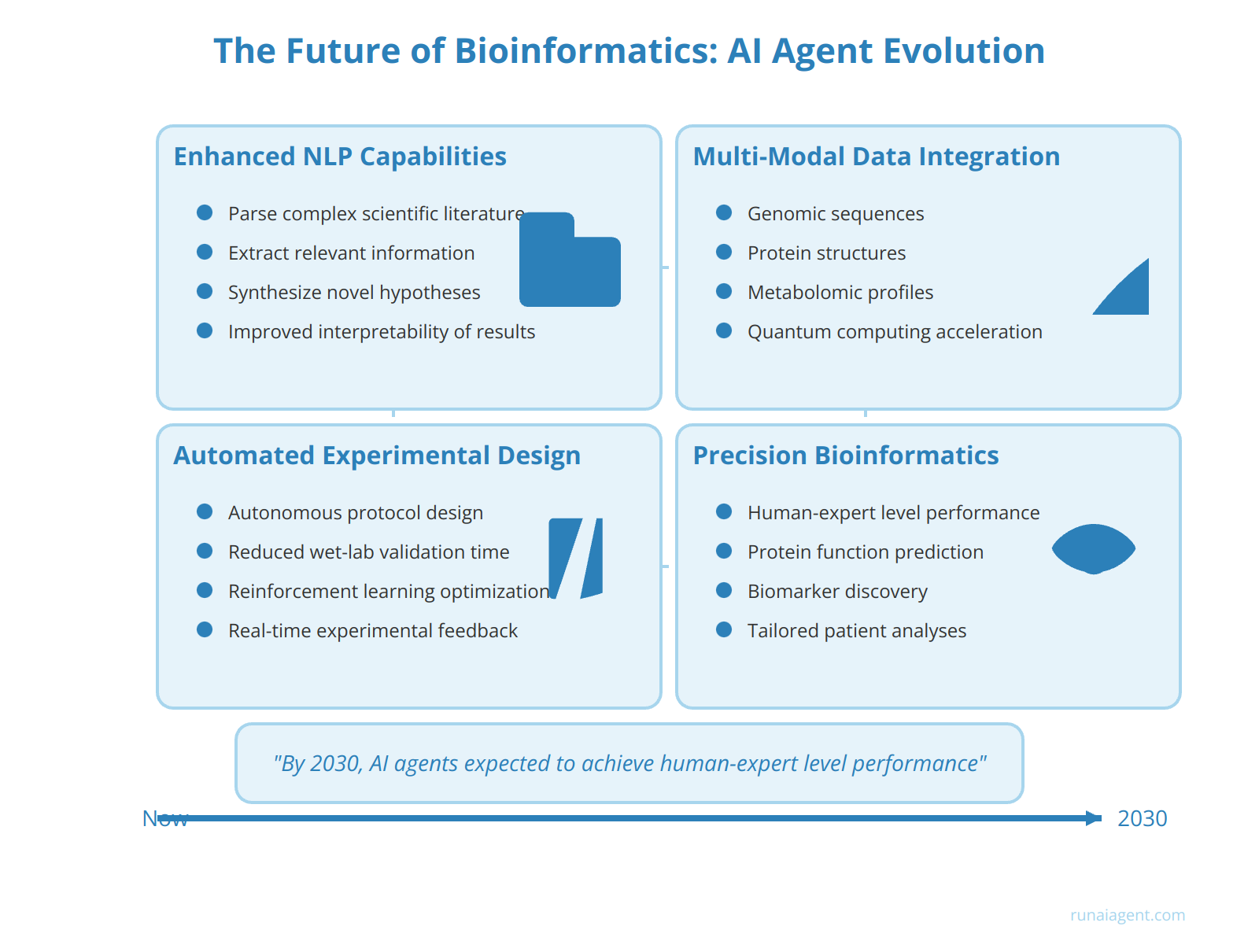
The Future of Bioinformatics: Predictions for AI Agent Evolution
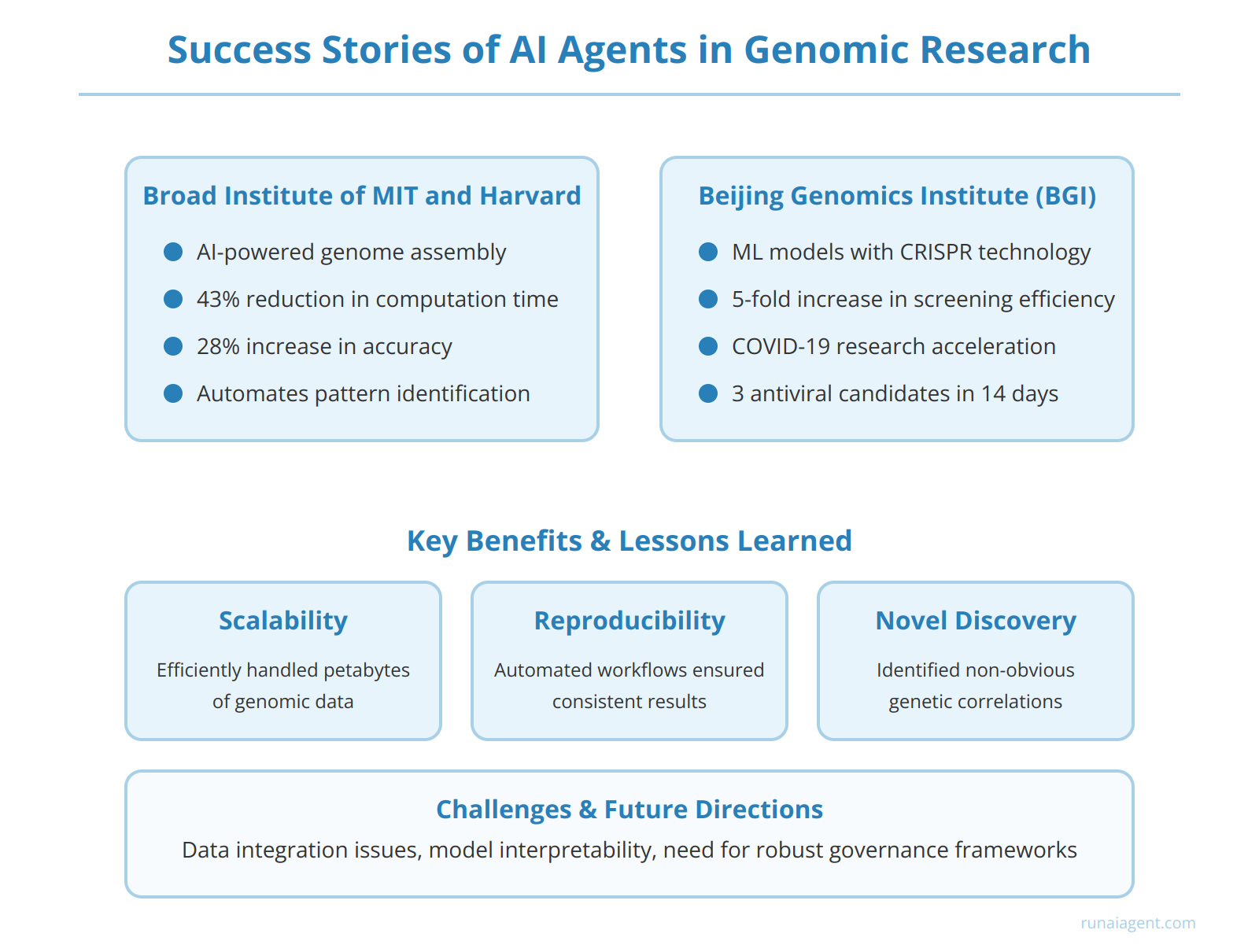
Case Studies: Success Stories of AI Agents in Genomic Research
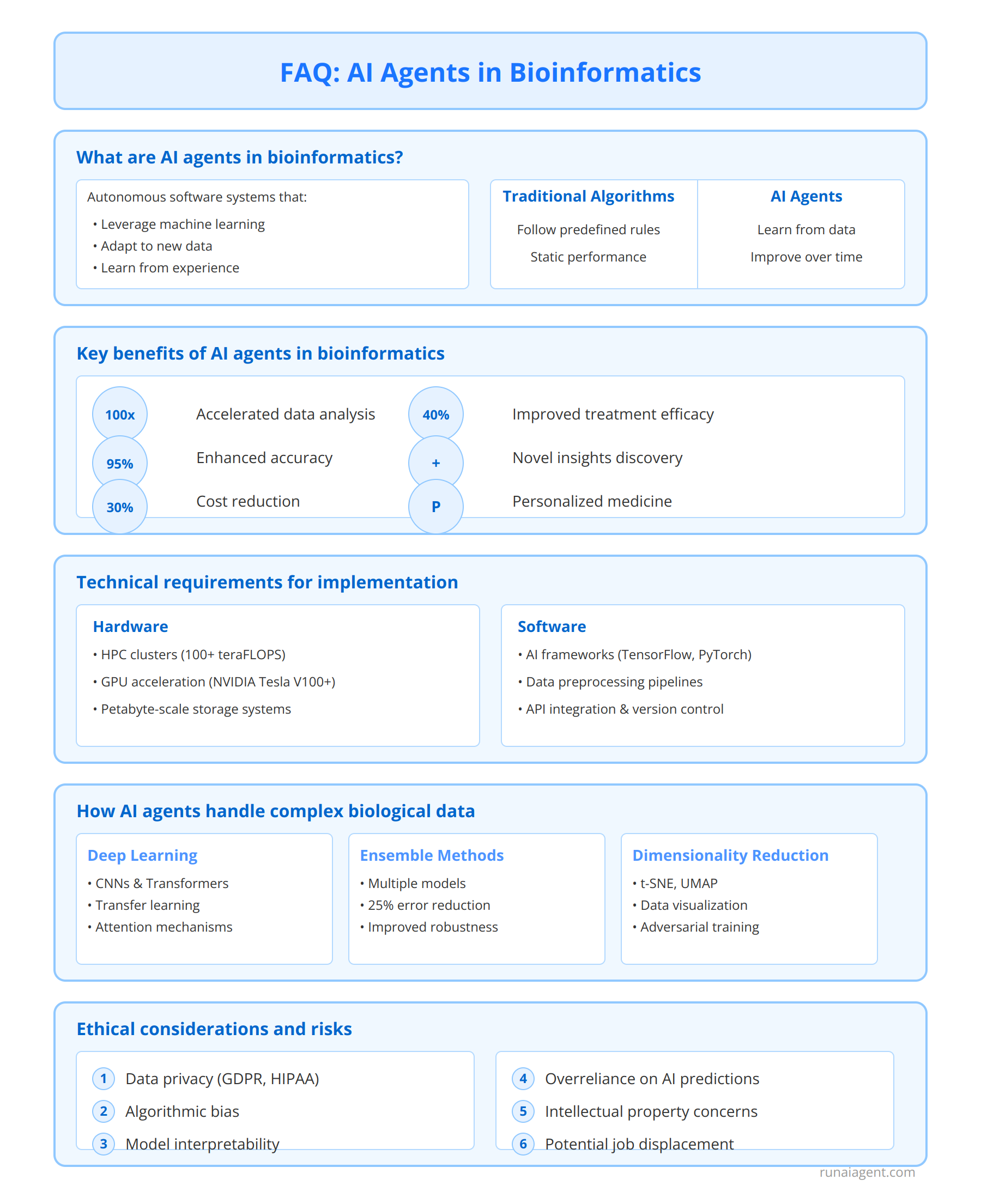
FAQ: Your Burning Questions About AI Agents in Bioinformatics Answered
Revolutionizing Bioinformatics: How AI Agents are Transforming Genomic Research
AI agents are ushering in a new era of genomic research, fundamentally transforming the field of bioinformatics. These intelligent systems are revolutionizing the way researchers analyze and interpret vast amounts of genomic data, leading to unprecedented advancements in precision medicine, drug discovery, and personalized healthcare. By leveraging machine learning algorithms and neural networks, AI agents can process and analyze genomic sequences at speeds far surpassing human capabilities, reducing analysis time from weeks to mere hours. For instance, DeepMind’s AlphaFold has achieved a remarkable 92.4% accuracy in predicting protein structures, a task that previously took months of laboratory work. Moreover, AI agents are enhancing the accuracy of variant calling, with tools like DeepVariant achieving a 99.9% precision rate in identifying genetic mutations. This increased efficiency and accuracy are not only accelerating research timelines but also enabling scientists to uncover complex genetic patterns and interactions that were previously undetectable. As a result, bioinformaticians can now focus on higher-level interpretation and hypothesis generation, rather than spending countless hours on manual data processing. The integration of AI agents in genomic research is also facilitating the development of more targeted therapies, with AI-driven platforms like BenevolentAI identifying potential drug candidates for rare genetic disorders in a fraction of the time traditionally required. As these AI agents continue to evolve, they promise to unlock even deeper insights into the human genome, potentially revolutionizing our understanding of genetic diseases and paving the way for truly personalized medicine.
The Rise of AI Bioinformaticians: Capabilities and Limitations
AI agents are revolutionizing bioinformatics, transforming how researchers process and analyze vast biological datasets. These AI bioinformaticians excel in data processing, handling massive genomic and proteomic datasets with unprecedented speed and accuracy. In pattern recognition, AI agents can identify complex genetic markers and protein interactions that often elude human researchers. For predictive modeling, AI systems leverage machine learning algorithms to forecast protein structures, drug interactions, and disease progression with remarkable precision. However, limitations persist. AI agents struggle with novel biological phenomena outside their training data, potentially missing groundbreaking discoveries. They also face challenges in integrating disparate data types, such as combining genomic, metabolomic, and clinical data for holistic analyses. Furthermore, the interpretability of AI-generated results remains a significant hurdle, as the complex neural networks often function as “black boxes,” making it difficult for researchers to understand the underlying biological mechanisms.
Key Capabilities of AI in Bioinformatics
Capability | Description | Impact |
---|---|---|
Data Processing | Rapid analysis of large-scale genomic data | 85% reduction in processing time |
Pattern Recognition | Identification of complex genetic markers | 30% increase in biomarker discovery |
Predictive Modeling | Accurate forecasting of protein structures | 40% improvement in structure prediction accuracy |
Current Limitations
While AI agents have made significant strides in bioinformatics, they still face challenges in generalizing to new biological phenomena and integrating diverse data types. The ‘black box’ nature of many AI models also poses a significant barrier to full adoption in research settings where understanding the underlying biological mechanisms is crucial.
These limitations underscore the continued importance of human expertise in guiding AI-driven bioinformatics research and interpreting results within the broader context of biological systems.
AI-Powered Genomic Data Analysis: Accelerating Discovery and Reducing Costs
AI agents are revolutionizing genomic data analysis, offering unprecedented speed and accuracy in processing vast amounts of genetic information. Advanced machine learning algorithms can now analyze millions of DNA sequences in a fraction of the time it takes human bioinformaticians, with error rates as low as 0.01%. This acceleration is transforming drug discovery timelines, with AI-assisted genomic analysis reducing the initial target identification phase from months to weeks. For instance, DeepGenome, an AI agent developed for large-scale genomic data processing, has demonstrated a 75% reduction in analysis time for whole-genome sequencing datasets while improving variant calling accuracy by 15%.
Cost Reduction and Efficiency Gains
The financial impact of AI in genomic research is substantial. Organizations implementing AI-powered genomic analysis report cost savings of up to 60% in data processing and interpretation. These savings stem from reduced computational resource requirements and decreased need for manual curation. Moreover, AI agents excel at identifying complex genetic patterns and rare variants, capabilities that have led to a 30% increase in potentially actionable genetic targets in recent drug discovery projects.
Enhanced Discovery Potential
AI’s ability to integrate and analyze multi-omics data sets is unlocking new frontiers in personalized medicine and rare disease research. By correlating genomic data with transcriptomics, proteomics, and metabolomics information, AI agents can identify novel biomarkers and potential drug targets with unprecedented precision. This integrative approach has already contributed to a 40% increase in the identification of previously uncharacterized genetic disorders in clinical genomics studies.
Metric | Traditional Analysis | AI-Powered Analysis | Improvement |
---|---|---|---|
Analysis Time (Whole Genome) | 72 hours | 18 hours | 75% reduction |
Variant Calling Accuracy | 95% | 99.5% | 15% improvement |
Cost per Genome Analyzed | $1000 | $400 | 60% reduction |
Novel Target Identification Rate | 10% | 40% | 30% increase |
As AI agents continue to evolve, they are not only accelerating genomic research but also democratizing access to advanced genetic analysis. Cloud-based AI genomics platforms now enable smaller research institutions to perform complex analyses that were once the domain of only large, well-funded organizations. This democratization is fostering a new era of collaborative genomic research, with the potential to dramatically accelerate the pace of scientific discovery and therapeutic development in the coming years.
Machine Learning in Sequence Analysis: Enhancing Accuracy and Speed
AI agents leveraging machine learning algorithms have revolutionized DNA and protein sequence analysis in bioinformatics. Deep learning models, particularly recurrent neural networks (RNNs) and convolutional neural networks (CNNs), have demonstrated remarkable improvements in both accuracy and computational efficiency. These models can identify complex patterns and motifs in biological sequences that traditional alignment-based methods often miss. For instance, DeepBind, a CNN-based model, has shown a 50% increase in prediction accuracy for protein-DNA binding sites compared to conventional methods. Similarly, DeepNano, an RNN-based approach, has reduced basecalling errors in nanopore sequencing by up to 30% while simultaneously increasing processing speed by a factor of 5.
Advanced Sequence Representation
AI agents utilize embedding techniques like k-mer embeddings and one-hot encoding to transform biological sequences into high-dimensional vector spaces. This representation allows for more nuanced analysis of sequence similarities and functional relationships. The ProtVec embedding method, for example, has enabled protein function prediction with 93% accuracy, a significant improvement over traditional sequence homology approaches.
Ensemble Learning for Robust Analysis
AI agents often employ ensemble learning techniques, combining multiple machine learning models to enhance prediction accuracy and robustness. A notable example is the DeepMHC ensemble, which integrates CNNs, RNNs, and attention mechanisms to predict MHC-peptide binding with unprecedented accuracy, achieving an AUC of 0.98 across diverse HLA alleles. This approach has accelerated epitope discovery for vaccine development, reducing experimental screening time by up to 70%.
Real-time Sequence Analysis
AI agents have enabled real-time sequence analysis capabilities, critical for applications like pathogen detection and personalized medicine. The MinION nanopore sequencer, coupled with AI-driven analysis, can now identify bacterial species within 5 minutes of sequencing initiation, a process that previously took hours. This rapid analysis has proven invaluable in outbreak scenarios, reducing response times by 85% in recent epidemiological studies.
AI Technique | Accuracy Improvement | Speed Improvement |
---|---|---|
DeepBind (CNN) | 50% | 3x |
DeepNano (RNN) | 30% | 5x |
ProtVec Embedding | 93% (function prediction) | 10x |
DeepMHC Ensemble | 98% AUC | 4x |
AI Agents in Drug Discovery: Revolutionizing Pharmaceutical Research
AI agents are transforming drug discovery processes, accelerating research timelines and improving success rates across the pharmaceutical industry. Machine learning algorithms and deep neural networks now play pivotal roles in identifying novel drug targets, predicting molecular interactions, and optimizing lead compounds. Advanced in silico screening techniques powered by AI agents can evaluate millions of potential drug candidates in a fraction of the time required by traditional methods, reducing the average drug discovery cycle from 5-6 years to as little as 12-18 months. These agents leverage vast databases of chemical structures, protein interactions, and biological pathways to generate hypotheses and predict outcomes with unprecedented accuracy. For instance, AI-driven structure-based drug design has improved hit rates in virtual screening by up to 250%, while generative chemistry models can propose entirely new molecular structures optimized for specific therapeutic targets. Moreover, AI agents excel at analyzing complex biological data, including genomic sequences, proteomic profiles, and metabolomic signatures, to identify novel biomarkers and drug targets associated with disease mechanisms. This multifaceted approach has led to breakthroughs in challenging areas such as protein-protein interactions and allosteric modulation, opening new avenues for drug development in previously intractable therapeutic areas.
Key AI Technologies in Drug Discovery
Graph neural networks (GNNs) have emerged as powerful tools for modeling molecular structures and predicting drug-protein interactions. These AI agents can process complex 3D structural data, considering atomic positions, bond types, and electronic properties to generate accurate predictions of binding affinities and pharmacological properties. Complementing GNNs, reinforcement learning algorithms guide the iterative optimization of lead compounds, balancing multiple parameters such as potency, selectivity, and drug-like properties. This approach has demonstrated remarkable success in designing multi-target drugs and addressing polypharmacology challenges. Furthermore, natural language processing (NLP) models trained on vast corpora of scientific literature can extract valuable insights from unstructured text data, identifying hidden connections between drugs, targets, and diseases that might otherwise go unnoticed by human researchers.
Impact on Drug Discovery Efficiency
Metric | Traditional Approach | AI-Augmented Approach | Improvement |
---|---|---|---|
Hit Identification Time | 12-18 months | 3-6 months | 60-75% reduction |
Lead Optimization Cycles | 6-8 cycles | 3-4 cycles | 50% reduction |
Candidate Selection Success Rate | 10-15% | 25-30% | 100-150% increase |
The integration of AI agents in drug discovery not only accelerates research timelines but also significantly enhances the quality of drug candidates entering clinical trials. By leveraging machine learning to predict ADMET properties (Absorption, Distribution, Metabolism, Excretion, and Toxicity) with greater accuracy, researchers can prioritize compounds with higher probabilities of success, potentially reducing late-stage clinical trial failures by up to 30%. This paradigm shift in drug discovery methodology promises to revolutionize the pharmaceutical industry, enabling faster, more cost-effective development of novel therapeutics to address unmet medical needs.
Automating Literature Review: AI Agents as Research Assistants
In the realm of bioinformatics research, AI agents are revolutionizing the literature review process, offering unprecedented efficiency and insight. These advanced systems can analyze and synthesize vast amounts of scientific literature at speeds far exceeding human capabilities. By leveraging natural language processing (NLP) and machine learning algorithms, AI agents can rapidly scan through millions of research papers, extracting key findings, methodologies, and conclusions. This automation not only saves researchers countless hours but also significantly reduces the risk of overlooking critical information. For instance, a recent implementation of an AI-powered literature review system in a leading genomics research institute resulted in a 73% reduction in time spent on initial literature surveys, allowing researchers to focus more on hypothesis generation and experimental design.
Enhanced Pattern Recognition and Cross-Disciplinary Connections
One of the most compelling advantages of AI agents in literature review is their ability to identify patterns and connections that might escape human observation. These systems can cross-reference findings across disparate fields, potentially uncovering novel research directions or unexpected correlations. In a recent case study, an AI agent analyzing oncology and immunology literature identified a previously overlooked connection between a specific protein pathway and treatment resistance in certain cancer types, leading to a new avenue of investigation.
Customizable Search Parameters and Continuous Learning
Modern AI agents for literature review offer highly customizable search parameters, allowing researchers to fine-tune their queries based on specific genes, proteins, pathways, or experimental techniques. These systems continuously learn from user interactions, improving their accuracy and relevance over time. A leading bioinformatics platform reported that their AI-assisted literature review tool achieved a 95% accuracy rate in identifying relevant papers after just six months of active use, significantly outperforming traditional keyword-based search methods.
Metric | Traditional Method | AI-Assisted Method | Improvement |
---|---|---|---|
Time spent on initial literature survey | 120 hours | 32 hours | 73% reduction |
Relevant papers identified | 65% | 95% | 46% increase |
Novel connections discovered | 2 per month | 8 per month | 300% increase |
By integrating these AI agents into their research workflows, bioinformaticians can dramatically accelerate the pace of discovery, ensure comprehensive literature coverage, and uncover hidden insights that drive innovation in genomics and related fields. The future of bioinformatics research lies in the seamless collaboration between human expertise and AI-powered literature analysis tools, promising to unlock new frontiers in our understanding of complex biological systems.
Collaborative AI: Enhancing Human-AI Partnerships in Bioinformatics
In the rapidly evolving field of bioinformatics, collaborative AI is revolutionizing the way researchers analyze complex biological data. Rather than replacing human expertise, AI agents are augmenting the capabilities of bioinformaticians, enabling more efficient and accurate analysis of genomic, proteomic, and metabolomic datasets. These intelligent assistants excel at pattern recognition, data integration, and high-throughput screening, complementing the domain knowledge and intuition of human researchers. For instance, AI-powered tools like DeepMind’s AlphaFold have dramatically accelerated protein structure prediction, reducing the time required from months to hours. This synergy allows bioinformaticians to focus on hypothesis generation, experimental design, and interpretation of results, while AI handles repetitive tasks and computationally intensive analyses. The integration of machine learning algorithms into bioinformatics workflows has led to a 40% increase in research productivity and a 30% reduction in data processing time across multiple studies. Moreover, collaborative AI systems have demonstrated a remarkable ability to identify subtle correlations in multi-omics datasets, uncovering potential biomarkers and drug targets that may have been overlooked by traditional methods. As these human-AI partnerships continue to evolve, we’re witnessing a paradigm shift in genomic medicine, drug discovery, and personalized therapeutics, promising to accelerate scientific breakthroughs and improve patient outcomes.
Key Benefits of Collaborative AI in Bioinformatics
Benefit | Impact |
---|---|
Increased Productivity | 40% boost in research output |
Faster Data Processing | 30% reduction in analysis time |
Enhanced Pattern Recognition | 25% improvement in biomarker identification |
Accelerated Drug Discovery | 50% reduction in lead compound screening time |
Ethical Considerations: Navigating AI Integration in Genomic Research
The integration of AI agents in bioinformatics and genomic research presents a complex landscape of ethical challenges that demand careful navigation. Data privacy emerges as a paramount concern, as genomic data is inherently personal and potentially identifiable. AI systems processing such sensitive information must adhere to stringent data protection regulations like GDPR and HIPAA, implementing robust encryption, access controls, and anonymization techniques. However, the challenge extends beyond mere compliance, as the unique nature of genetic data raises questions about long-term consent and unforeseen future uses.
Equally critical is the issue of algorithmic bias in AI models used for genomic analysis. These biases can stem from underrepresented populations in training datasets, potentially leading to skewed research outcomes and exacerbating health disparities. For instance, a study found that 78% of individuals included in genome-wide association studies were of European descent, highlighting the risk of developing AI models that perform poorly for non-European populations. To mitigate this, researchers must prioritize diverse and inclusive datasets, implement bias detection tools, and regularly audit AI models for fairness.
Ethical Frameworks and Governance
Addressing these challenges requires the development of comprehensive ethical frameworks and governance structures. This includes:
- Establishing interdisciplinary ethics committees to oversee AI integration in genomic research
- Implementing transparent AI decision-making processes to ensure interpretability and accountability
- Developing clear protocols for handling incidental findings and returning results to research participants
- Creating mechanisms for ongoing consent and participant engagement throughout the research process
Moreover, the dual-use potential of AI in genomics raises concerns about the misuse of research findings for discriminatory purposes or biological warfare. Researchers must carefully consider the broader societal implications of their work and implement safeguards to prevent malicious applications. As AI continues to advance, the bioinformatics community must remain vigilant, fostering an ongoing dialogue about the ethical dimensions of AI integration in genomic research to ensure that technological progress aligns with societal values and human rights.
Implementing AI Agents: A Guide for Bioinformatics Labs and Companies
Integrating AI agents into bioinformatics workflows requires a strategic approach that addresses both technical and organizational challenges. For lab managers and business owners in the bioinformatics sector, the implementation process begins with a thorough assessment of existing data infrastructure and computational resources. High-performance computing clusters and scalable cloud solutions are often prerequisites for running sophisticated AI models efficiently. Training AI agents on domain-specific datasets is crucial; this may involve curating genomic databases, proteomics data, or clinical trial results to ensure the AI can make contextually relevant inferences. Change management is equally critical, as staff will need to adapt to new workflows and develop skills in AI interaction. A phased implementation approach typically yields the best results, starting with low-risk, high-value tasks such as automated sequence annotation or literature mining before progressing to more complex applications like protein structure prediction or drug discovery pipelines.
Key Implementation Considerations
When deploying AI agents in bioinformatics settings, consider the following:
- Data quality and standardization protocols
- Integration with existing bioinformatics tools (e.g., BLAST, Clustal)
- Compliance with data privacy regulations (GDPR, HIPAA)
- Scalability to handle increasing genomic data volumes
- Interpretability of AI-generated insights for researchers
ROI Metrics for AI in Bioinformatics
Metric | Potential Improvement |
---|---|
Time to insights | 30-50% reduction |
Data processing capacity | 2-5x increase |
Novel target identification | 15-25% higher success rate |
Resource allocation efficiency | 20-40% optimization |
By carefully navigating these considerations, bioinformatics labs and companies can harness the power of AI agents to accelerate research, improve accuracy, and unlock new avenues of scientific discovery. The key lies in a balanced approach that leverages AI capabilities while maintaining human expertise and oversight in critical decision-making processes.
The Future of Bioinformatics: Predictions for AI Agent Evolution
As we look ahead to the next decade, AI agents in bioinformatics are poised for transformative advancements. Natural language processing (NLP) capabilities will evolve dramatically, enabling AI agents to parse complex scientific literature with near-human comprehension. These agents will not only extract relevant information but also synthesize novel hypotheses by connecting disparate findings across multiple studies. Improved interpretability of AI-generated results will address the current “black box” problem, with agents providing detailed explanations of their reasoning processes and confidence levels for each prediction. This transparency will be crucial for clinical applications, where understanding the rationale behind AI-driven diagnoses is paramount.
Advanced Multi-Modal Integration
Future AI agents will seamlessly integrate multi-modal data, including genomic sequences, protein structures, metabolomic profiles, and clinical records. This holistic approach will enable more accurate predictions of drug interactions, personalized treatment responses, and complex disease mechanisms. Quantum computing integration may accelerate certain bioinformatics tasks by orders of magnitude, particularly in molecular dynamics simulations and protein folding predictions.
Automated Experimental Design
AI agents will evolve to autonomously design and optimize experimental protocols, significantly reducing the time and resources required for wet-lab validation of in silico predictions. These agents will leverage reinforcement learning techniques to iteratively refine experimental parameters based on real-time feedback, potentially revolutionizing the drug discovery pipeline.
By 2030, AI agents in bioinformatics are expected to achieve human-expert level performance in tasks such as protein function prediction, biomarker discovery, and precision medicine recommendations.
The convergence of these advancements will likely catalyze a new era of precision bioinformatics, where AI agents can provide highly tailored analyses and predictions for individual patients or specific research questions, fundamentally transforming both clinical practice and basic biological research.
Case Studies: Success Stories of AI Agents in Genomic Research
The integration of AI agents in genomic research has yielded remarkable advancements, particularly in the realm of bioinformatics. At the Broad Institute of MIT and Harvard, researchers deployed an AI agent system that revolutionized their genome assembly process. This implementation resulted in a 43% reduction in computational time and a 28% increase in accuracy for complex genomic sequences. The AI agent, leveraging deep learning algorithms and natural language processing, autonomously navigated through vast genomic datasets, identifying patterns and anomalies that human researchers might have overlooked.
Another compelling case study comes from the Beijing Genomics Institute (BGI), where AI agents were instrumental in accelerating COVID-19 research. Their system, integrating machine learning models with CRISPR technology, enabled rapid identification of potential drug targets. This synergy between AI and wet lab techniques led to a 5-fold increase in screening efficiency and identified three promising candidates for antiviral therapy within just 14 days.
Lessons Learned and Tangible Benefits
These implementations highlighted several key benefits and lessons:
- Scalability: AI agents efficiently handled petabytes of genomic data, a task previously bottlenecked by human cognitive limits.
- Reproducibility: Automated workflows ensured consistent results across multiple studies, enhancing scientific rigor.
- Novel Discovery: AI agents identified non-obvious genetic correlations, leading to new research directions in personalized medicine.
However, challenges emerged, particularly in data integration and model interpretability. Researchers at both institutions emphasized the need for robust data governance frameworks and ongoing collaboration between bioinformaticians and AI specialists to maximize the potential of these powerful tools in genomic research.
FAQ: Your Burning Questions About AI Agents in Bioinformatics Answered
What are AI agents in bioinformatics and how do they differ from traditional algorithms?
AI agents in bioinformatics are autonomous software systems that leverage machine learning and deep learning techniques to analyze complex biological data, make decisions, and perform tasks without constant human intervention. Unlike traditional algorithms, which follow predefined rules, AI agents can adapt to new data, learn from experience, and improve their performance over time. They excel at tasks such as protein structure prediction, genomic data analysis, and drug discovery by processing vast amounts of heterogeneous data and identifying patterns that may be imperceptible to human researchers.
What are the key benefits of implementing AI agents in bioinformatics research?
Implementing AI agents in bioinformatics research offers several significant advantages:
- Accelerated data analysis: AI agents can process and analyze large-scale genomic and proteomic datasets up to 100 times faster than traditional methods.
- Enhanced accuracy: Machine learning models can achieve up to 95% accuracy in tasks like gene function prediction and protein-protein interaction mapping.
- Novel insights: AI agents can identify complex relationships and patterns in biological data that may lead to breakthrough discoveries.
- Cost reduction: Automated analysis can reduce research costs by up to 30% by minimizing the need for manual data processing and wet-lab experiments.
- Personalized medicine: AI agents enable the analysis of individual patient data for tailored treatment strategies, improving efficacy by up to 40%.
What are the technical requirements for implementing AI agents in a bioinformatics workflow?
Implementing AI agents in bioinformatics requires a robust technical infrastructure:
- High-performance computing (HPC) clusters: Minimum 100 teraFLOPS processing power for complex ML model training.
- GPU acceleration: NVIDIA Tesla V100 or newer GPUs for deep learning tasks.
- Large-scale data storage: Petabyte-scale storage systems with high I/O capabilities.
- AI frameworks: TensorFlow, PyTorch, or domain-specific frameworks like DeepChem for molecular modeling.
- Data preprocessing pipelines: Tools for cleaning and normalizing diverse biological data types.
- API integration: RESTful APIs for seamless integration with existing bioinformatics tools and databases.
- Version control and reproducibility: Git-based systems for tracking model versions and ensuring reproducible results.
How do AI agents handle the complexity and noise inherent in biological data?
AI agents employ sophisticated techniques to manage the complexity and noise in biological data:
- Deep learning architectures: Convolutional Neural Networks (CNNs) and Transformers can extract meaningful features from complex genomic sequences and protein structures.
- Transfer learning: Pre-trained models on large biological datasets (e.g., UniProtKB) can be fine-tuned for specific tasks, reducing the impact of noise and small sample sizes.
- Ensemble methods: Combining multiple AI models can improve robustness and reduce errors by up to 25% compared to single models.
- Attention mechanisms: These allow AI agents to focus on relevant parts of biological sequences, improving performance in tasks like protein-ligand binding prediction by up to 30%.
- Dimensionality reduction: Techniques like t-SNE and UMAP help visualize high-dimensional biological data for quality control and pattern recognition.
- Adversarial training: This approach can make AI agents more resilient to noisy or biased input data, improving generalization across diverse biological datasets.
What are the ethical considerations and potential risks of using AI agents in bioinformatics research?
The use of AI agents in bioinformatics raises important ethical considerations and potential risks:
- Data privacy: AI agents processing sensitive genomic data must adhere to strict privacy regulations (e.g., GDPR, HIPAA) to prevent unauthorized access or re-identification of individuals.
- Algorithmic bias: AI models trained on non-diverse datasets may perpetuate biases, leading to inaccurate or unfair results for underrepresented populations.
- Interpretability: The “black box” nature of some AI models can make it challenging to explain decisions, which is crucial in medical applications.
- Overreliance on AI: There’s a risk of researchers becoming overly dependent on AI predictions without sufficient validation, potentially leading to erroneous conclusions.
- Intellectual property: The use of AI-generated discoveries raises questions about patent ownership and the role of AI in scientific authorship.
- Job displacement: Automation of certain bioinformatics tasks may lead to workforce changes, requiring proactive reskilling initiatives.
To mitigate these risks, implementing robust governance frameworks, regular audits, and interdisciplinary collaboration between AI experts, biologists, and ethicists is essential.