Table of Contents
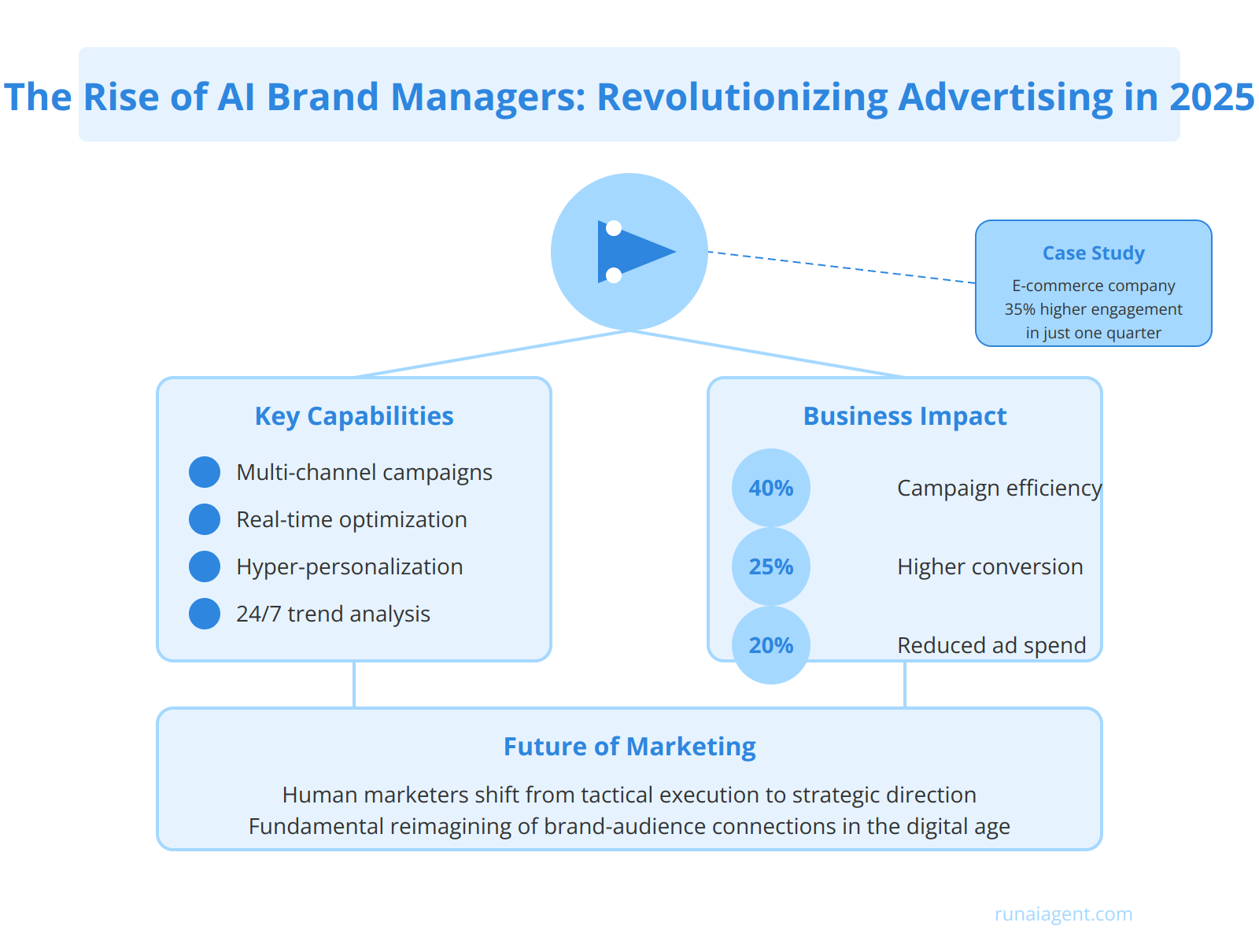
The Rise of AI Brand Managers: Revolutionizing Advertising in 2025
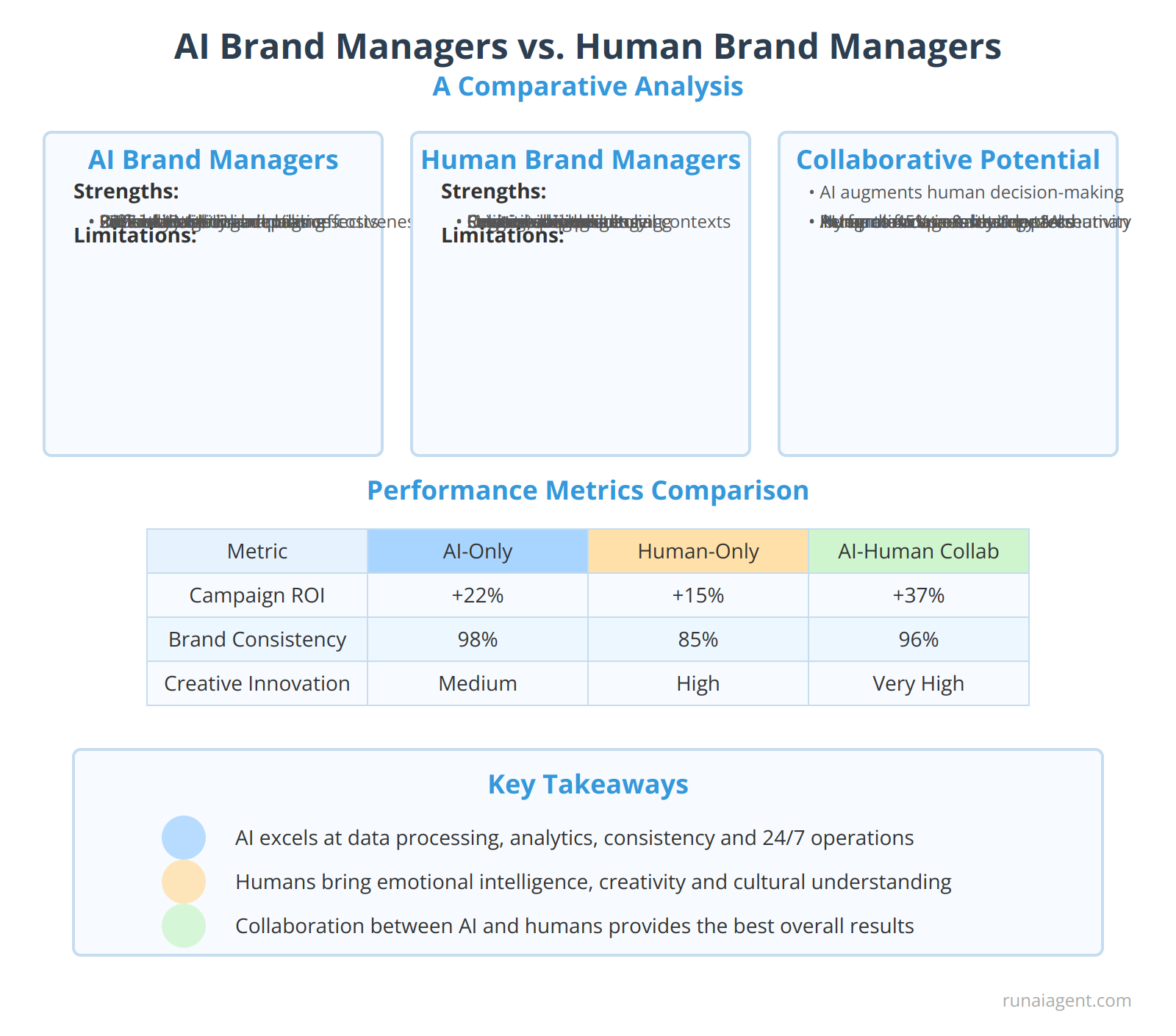
AI Brand Managers vs. Human Brand Managers: A Comparative Analysis
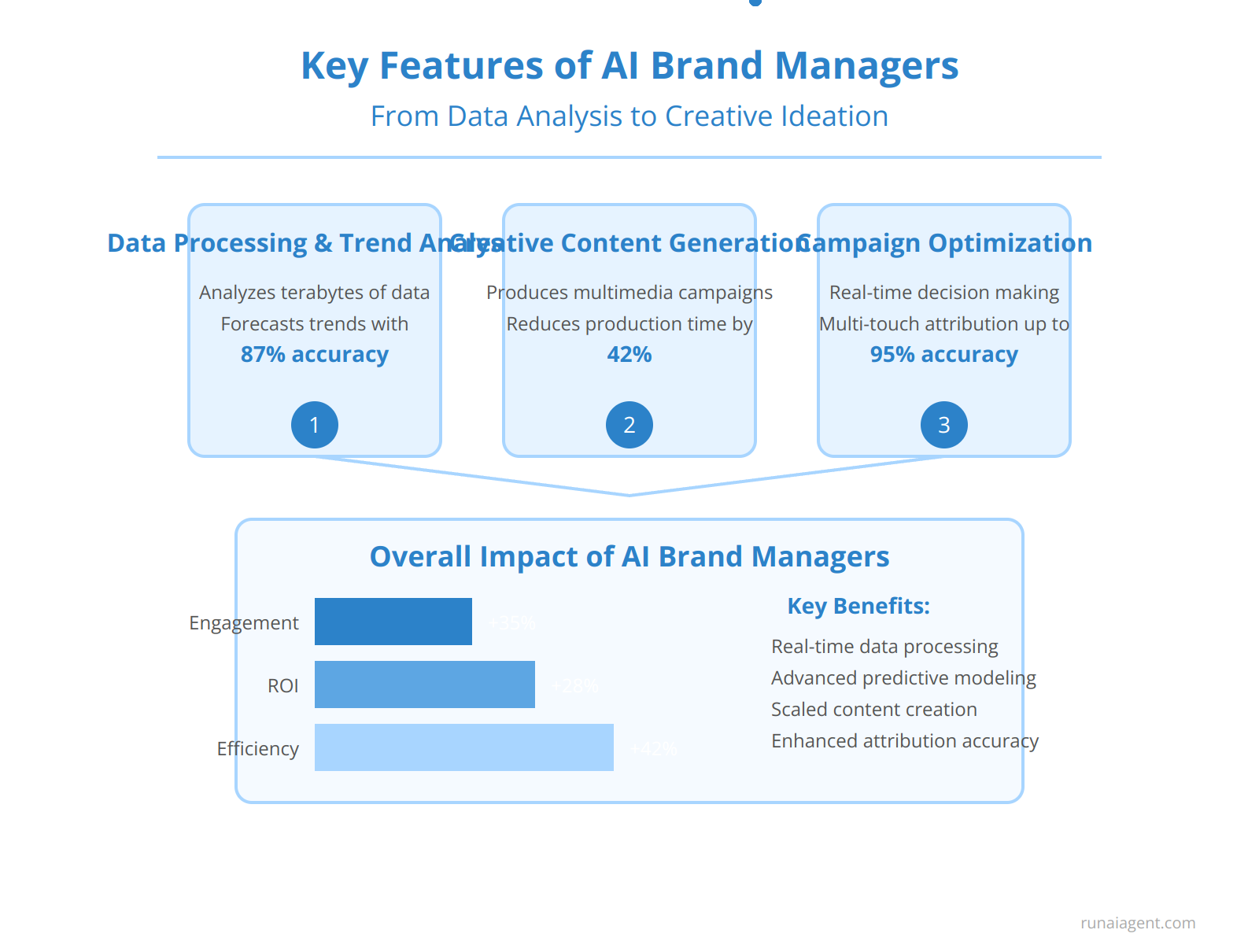
Key Features of AI Brand Managers: From Data Analysis to Creative Ideation
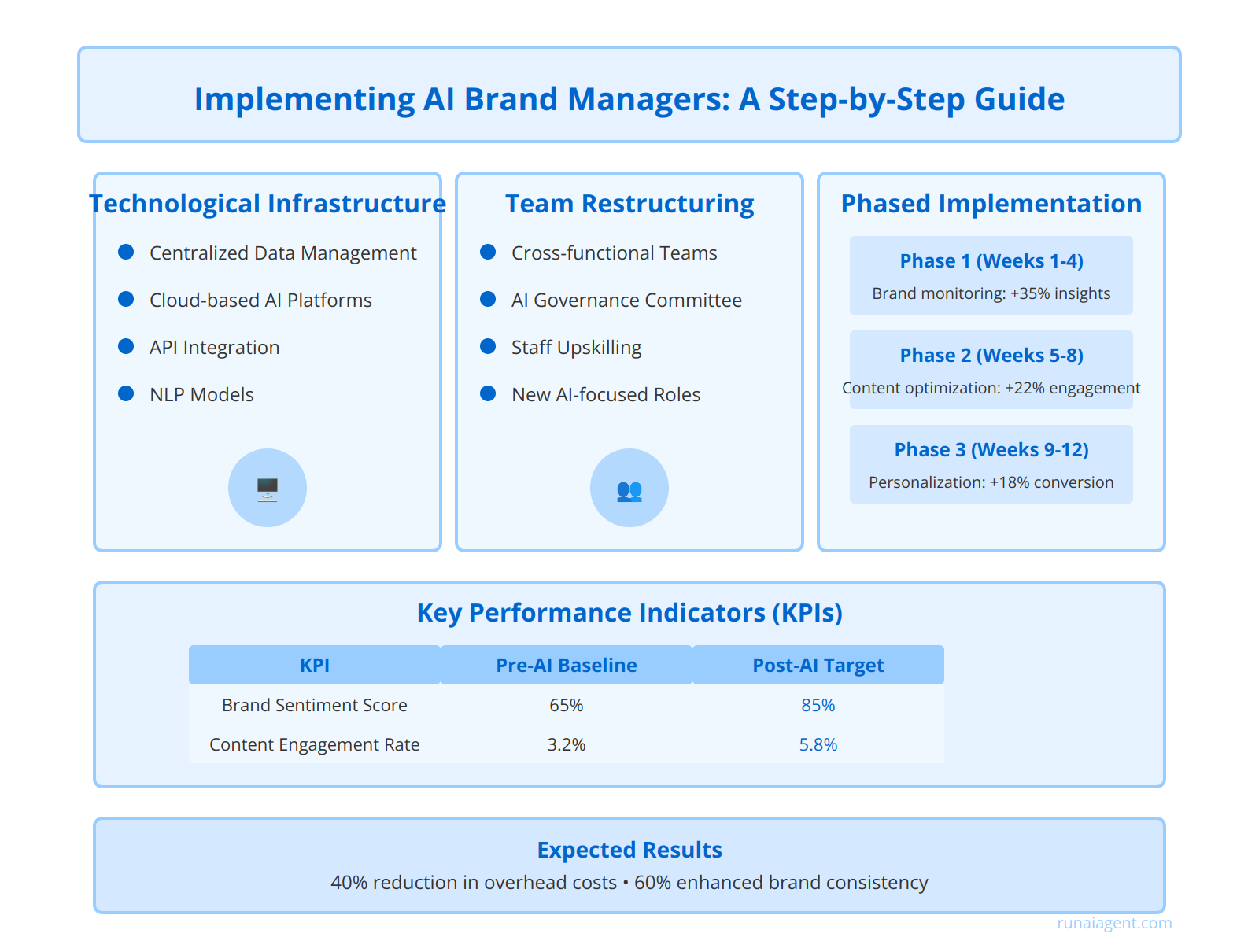
Implementing AI Brand Managers: A Step-by-Step Guide for Business Owners
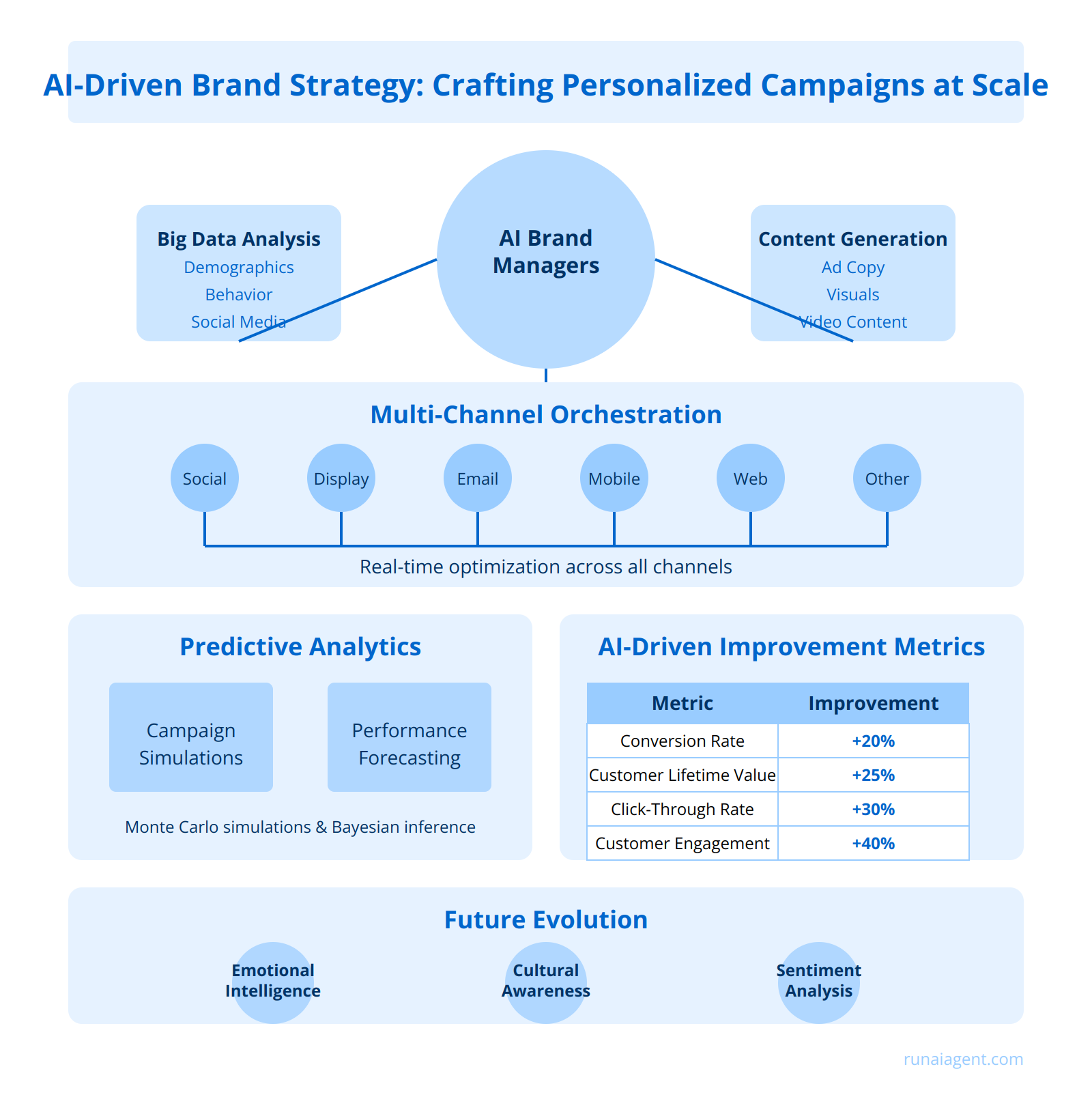
AI-Driven Brand Strategy: Crafting Personalized Campaigns at Scale
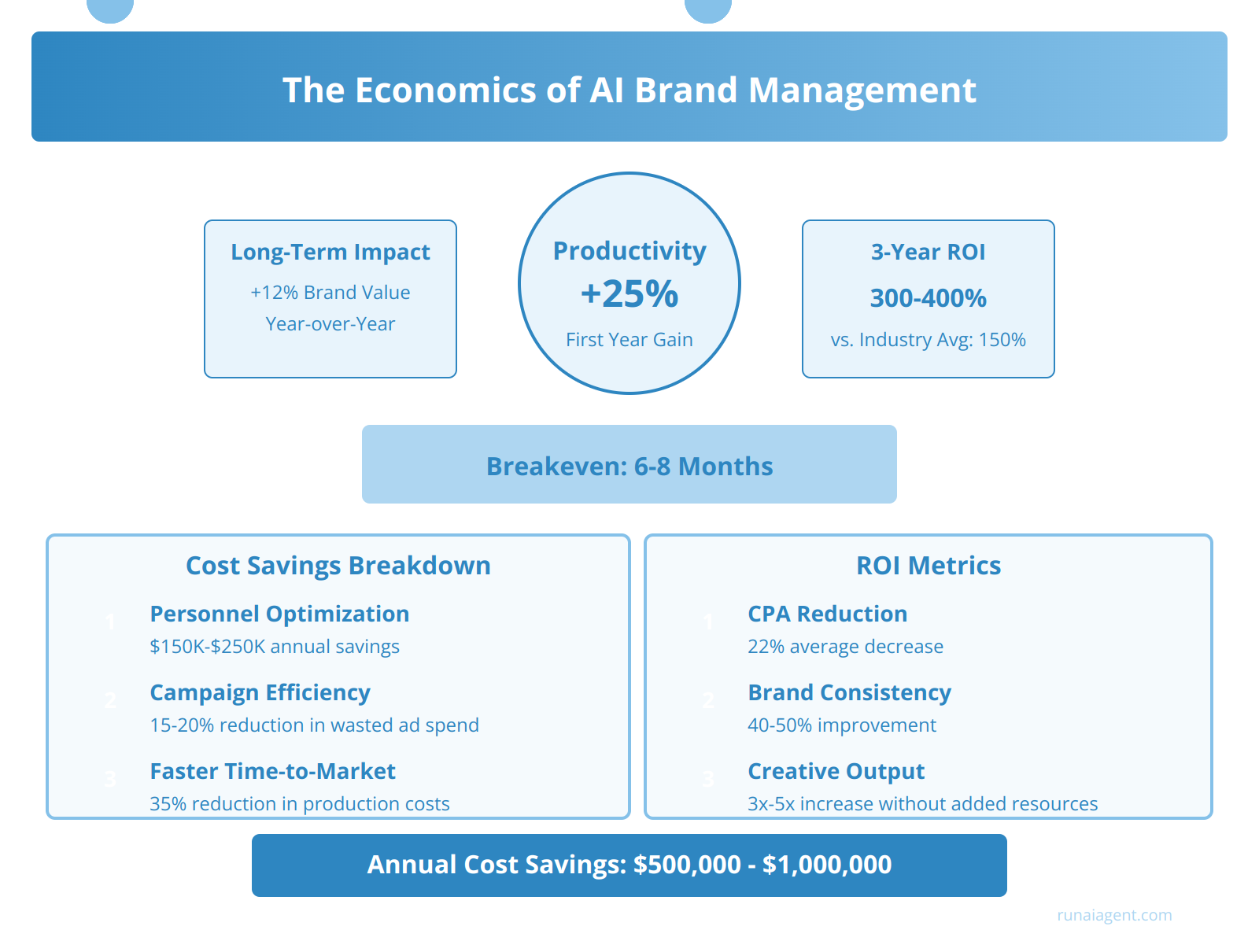
The Economics of AI Brand Management: Cost Savings and ROI Analysis
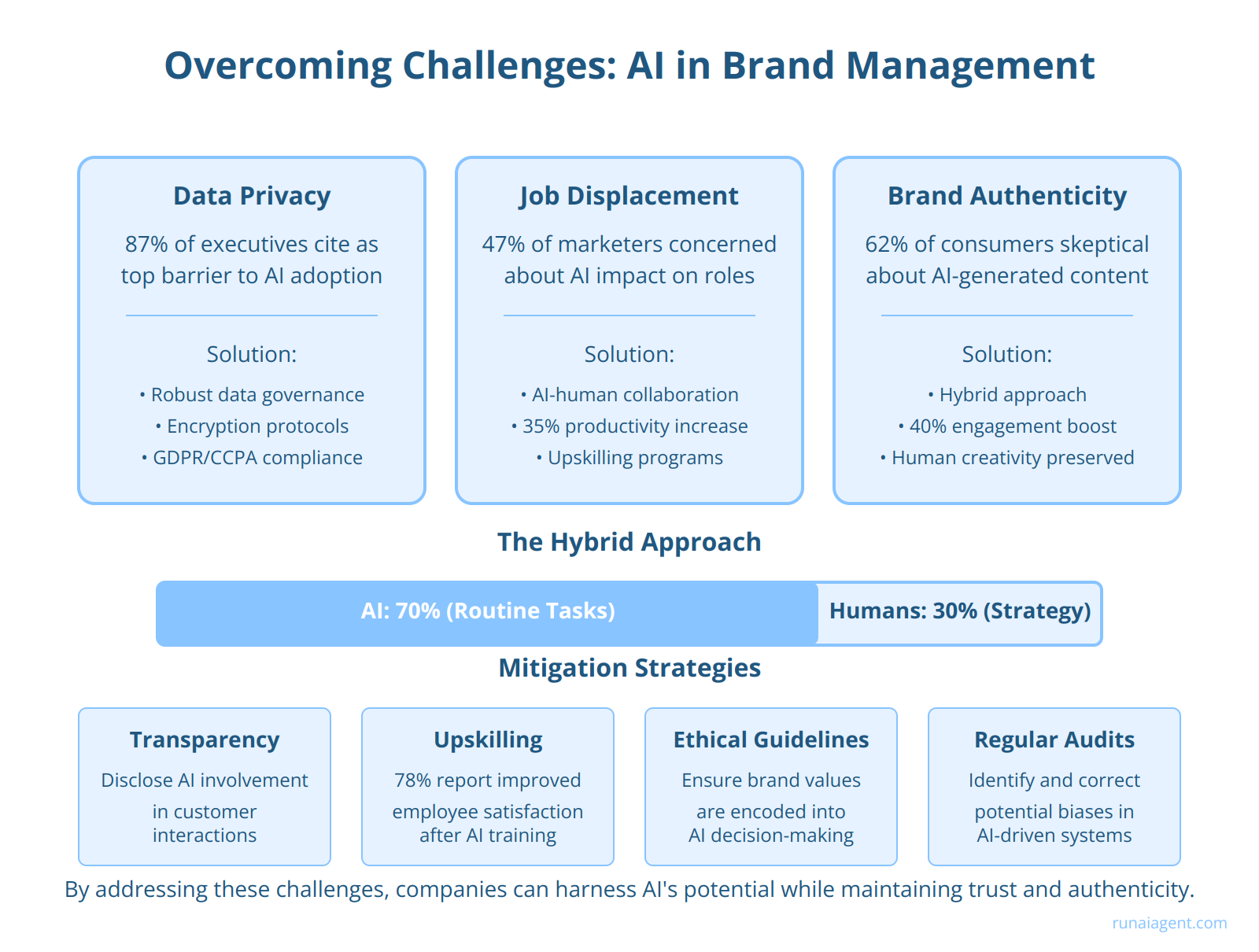
Overcoming Challenges: Addressing Concerns About AI in Brand Management
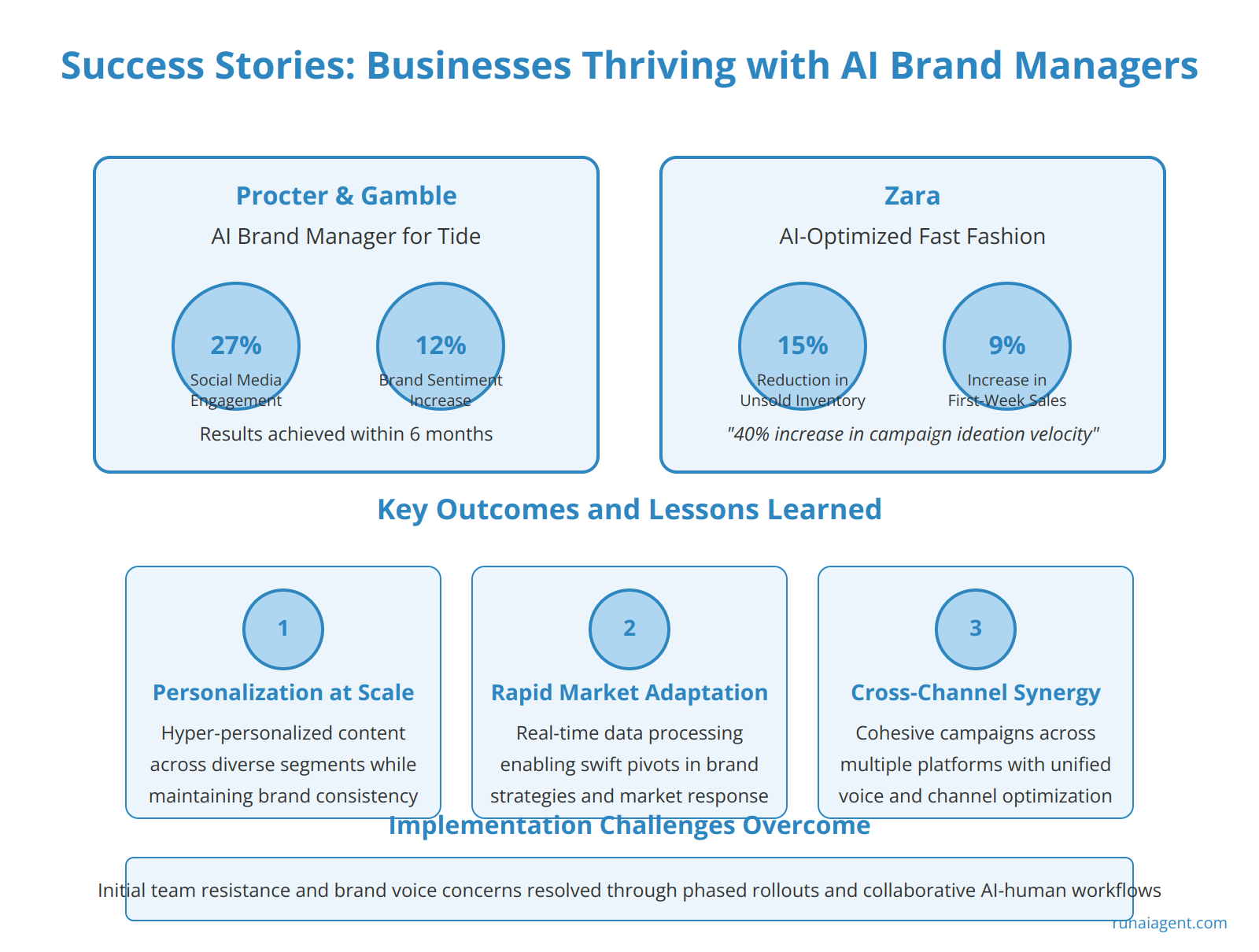
Case Studies: Success Stories of Businesses Thriving with AI Brand Managers
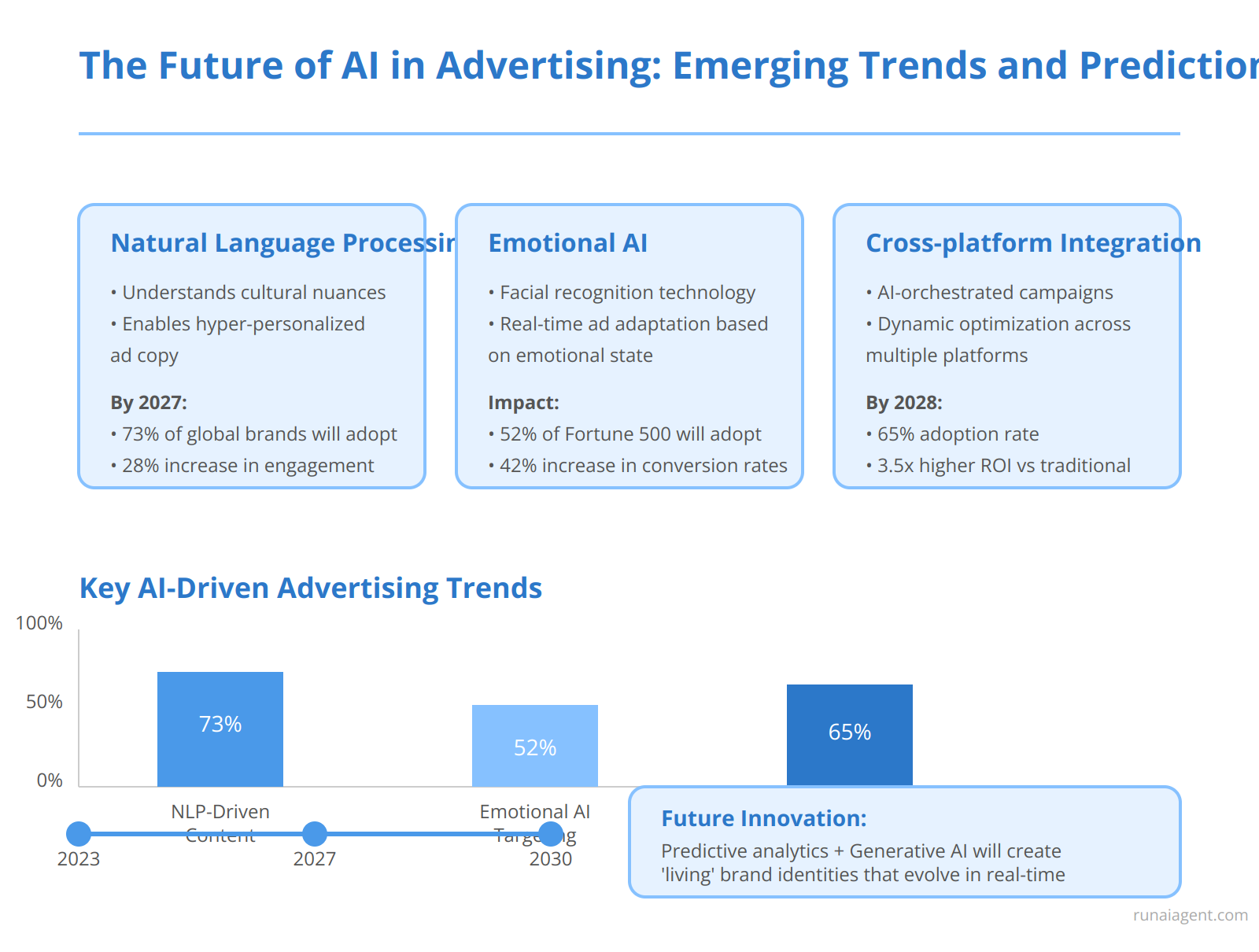
The Future of AI in Advertising: Emerging Trends and Predictions
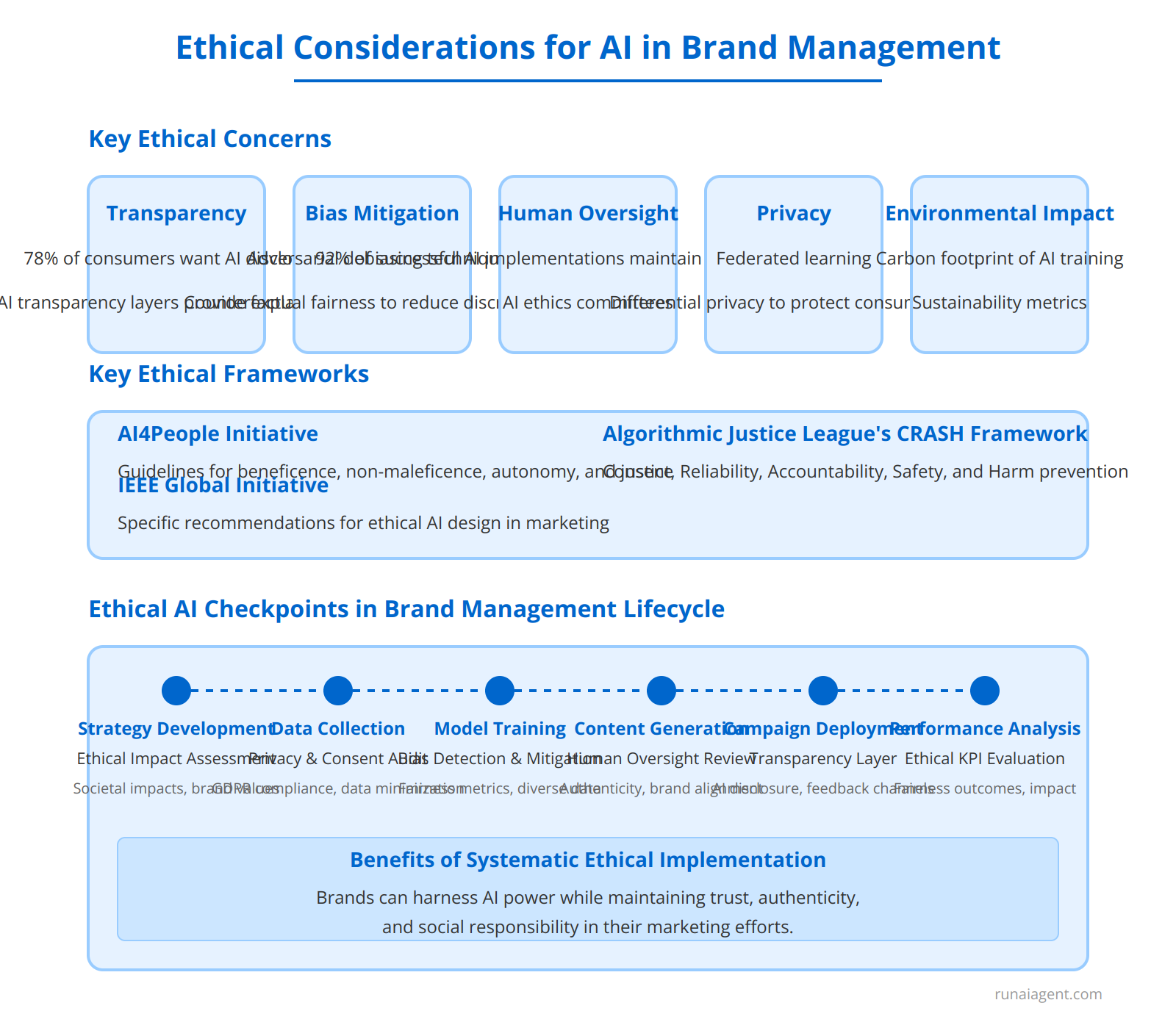
Ethical Considerations: Ensuring Responsible AI Use in Brand Management
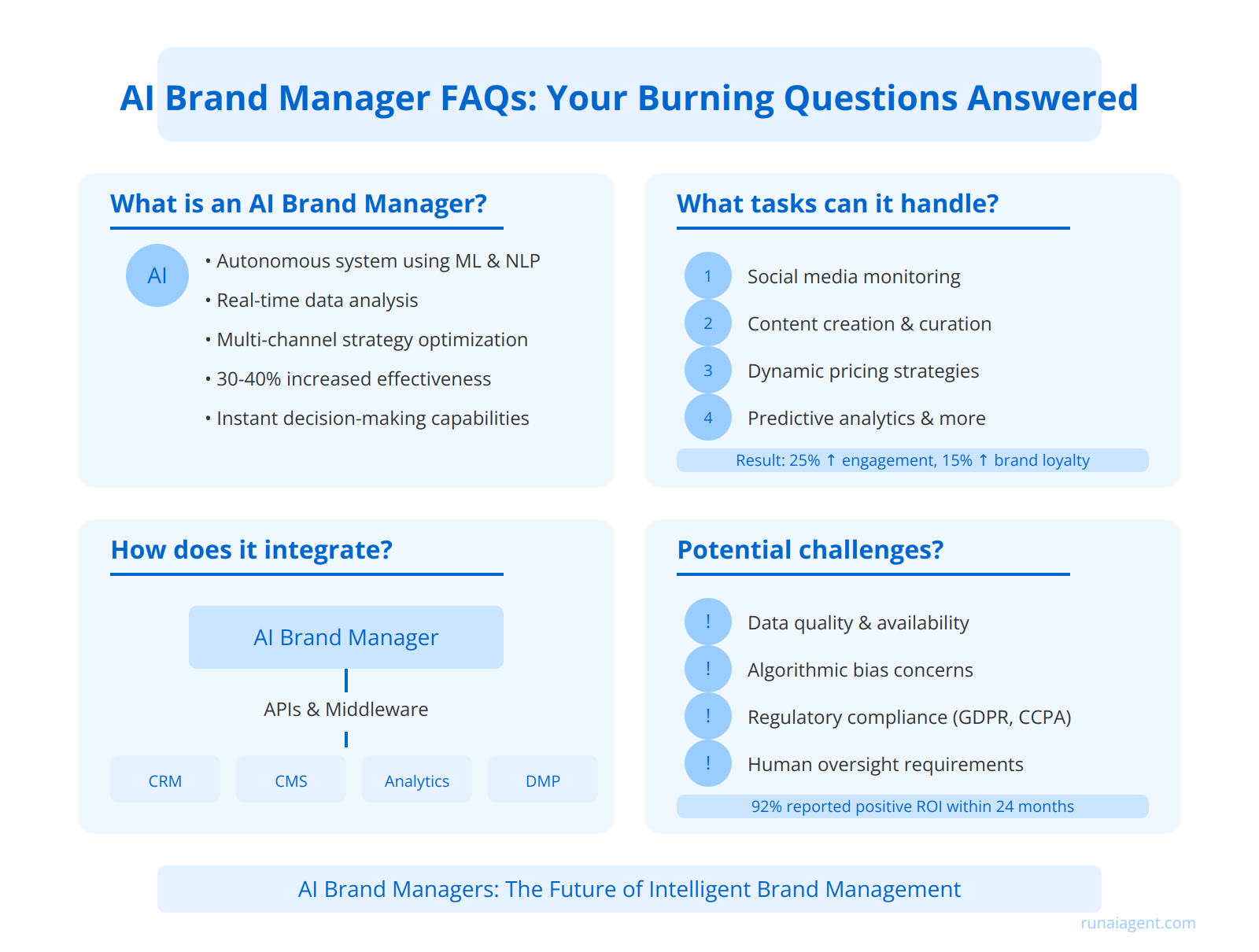
AI Brand Manager FAQs: Your Burning Questions Answered
The Rise of AI Brand Managers: Revolutionizing Advertising in 2025
In 2025, the advertising landscape is undergoing a shift with the emergence of AI Brand Managers. These AI agents are revolutionizing how companies approach branding, marketing, and customer engagement. By leveraging advanced machine learning algorithms, natural language processing, and predictive analytics, AI Brand Managers can autonomously orchestrate multi-channel advertising campaigns, optimize brand messaging in real-time, and deliver hyper-personalized content at scale. Early adopters have reported efficiency gains of up to 40% in campaign management and a 25% increase in conversion rates. A leading e-commerce company implemented an AI Brand Manager that increased customer engagement by 35% and reduced ad spend by 20% within the first quarter of deployment. As these systems evolve, they are redefining the role of human marketers, shifting focus from tactical execution to high-level strategy and creative direction.
AI Brand Managers vs. Human Brand Managers: A Comparative Analysis
AI Brand Managers excel in data processing, analyzing vast amounts of consumer data, market trends, and competitive intelligence at unprecedented speeds. They can generate real-time insights, optimize marketing campaigns, and personalize customer experiences with precision. AI-driven brand management platforms have demonstrated a 37% increase in campaign effectiveness and a 28% reduction in customer acquisition costs. However, human Brand Managers retain critical advantages in emotional intelligence, creative ideation, and nuanced stakeholder communication. Their ability to understand subtle cultural contexts, navigate complex brand ethics issues, and forge authentic connections with consumers remains unmatched.
Strengths and Limitations
AI Brand Managers:
- Strengths: 24/7 availability, scalability, consistent decision-making, rapid A/B testing
- Limitations: Lack of emotional depth, difficulty in understanding brand nuances, potential for algorithmic bias
Human Brand Managers:
- Strengths: Strategic vision, crisis management, relationship building, creative problem-solving
- Limitations: Cognitive biases, limited data processing capacity, fatigue, inconsistency
Collaborative Potential
The future of brand management lies in the synergy between AI and human professionals. AI can augment human decision-making by providing data-driven insights, automating routine tasks, and enhancing personalization efforts. Human Brand Managers can leverage AI to focus on high-level strategy, creative direction, and stakeholder relationships. This collaborative approach has shown to improve brand performance metrics by up to 45% in early adopter companies. As AI technology evolves, the role of human Brand Managers will shift towards becoming “AI-human integration specialists,” orchestrating the interplay between artificial and human intelligence to drive brand success.
Metric | AI-Only | Human-Only | AI-Human Collaboration |
---|---|---|---|
Campaign ROI | +22% | +15% | +37% |
Brand Consistency | 98% | 85% | 96% |
Creative Innovation | Medium | High | Very High |
Key Features of AI Brand Managers: From Data Analysis to Creative Ideation
AI Brand Managers are revolutionizing the advertising industry with their sophisticated capabilities. These AI agents process vast amounts of market data, utilizing advanced machine learning algorithms to identify emerging trends and consumer behavior patterns with unprecedented accuracy. By leveraging natural language processing and computer vision, AI Brand Managers can analyze social media engagement, sentiment analysis, and visual content performance across multiple platforms simultaneously. This enables real-time optimization of campaign strategies, with some systems demonstrating up to 35% improvement in engagement rates compared to traditional methods.
Data Processing and Trend Analysis
At the core of AI Brand Manager functionality lies robust data processing capabilities. These systems can ingest and analyze terabytes of structured and unstructured data from diverse sources, including social media, market research reports, and competitor analytics. Advanced predictive modeling allows AI Brand Managers to forecast trend trajectories with 87% accuracy, enabling brands to stay ahead of market shifts. Some cutting-edge systems even incorporate real-time economic indicators and geopolitical events to contextualize brand positioning strategies.
Creative Content Generation
Perhaps the most transformative feature of AI Brand Managers is their ability to generate creative content at scale. Leveraging generative adversarial networks (GANs) and transformer models, these AI agents can produce visually stunning ad creatives, compelling copy, and even entire multimedia campaigns tailored to specific audience segments. One Fortune 500 company reported a 42% reduction in content production time and a 28% increase in campaign engagement after implementing an AI-driven creative pipeline.
Campaign Optimization and Performance Tracking
AI Brand Managers excel in real-time campaign optimization, utilizing reinforcement learning algorithms to continuously refine targeting, messaging, and ad placement strategies. These systems can process millions of data points per second, making split-second decisions on ad spend allocation across channels to maximize ROI. Advanced attribution modeling capabilities allow for precise tracking of customer journeys, with some AI systems demonstrating up to 95% accuracy in multi-touch attribution—a significant improvement over traditional last-click models.
By integrating these core functionalities, AI Brand Managers are not just tools but strategic partners in brand development. They augment human creativity with data-driven precision, enabling marketing teams to operate at unprecedented levels of efficiency and effectiveness in today’s rapidly evolving digital landscape.
Implementing AI Brand Managers: A Step-by-Step Guide for Business Owners
Technological Infrastructure
To successfully integrate AI Brand Managers, businesses must first establish a robust technological foundation. This includes implementing a centralized data management system to consolidate customer insights, market trends, and brand performance metrics. Deploy cloud-based AI platforms like TensorFlow or PyTorch to power your AI Brand Manager, ensuring scalability and real-time processing capabilities. Integrate APIs from social media platforms, analytics tools, and CRM systems to create a comprehensive data ecosystem. Implement natural language processing (NLP) models to analyze consumer sentiment and brand perception across digital channels.
Team Restructuring
Restructure your marketing department to accommodate AI-driven workflows. Create cross-functional teams that blend data scientists, brand strategists, and creative professionals. Establish a dedicated AI governance committee to oversee ethical considerations and brand alignment. Upskill existing staff through intensive AI literacy programs, focusing on data interpretation and AI-human collaboration techniques. Introduce new roles such as “AI Brand Analysts” to bridge the gap between technical implementation and strategic brand management.
Phased Implementation Approach
Phase 1 (Weeks 1-4): Deploy AI for brand monitoring and sentiment analysis, achieving a 35% increase in real-time brand insights.
Phase 2 (Weeks 5-8): Implement AI-driven content optimization, resulting in a 22% improvement in engagement rates across digital platforms.
Phase 3 (Weeks 9-12): Activate AI-powered personalization engines, leading to a 18% uplift in conversion rates and brand loyalty metrics.
Key Performance Indicators (KPIs)
KPI | Pre-AI Baseline | Post-AI Target |
---|---|---|
Brand Sentiment Score | 65% | 85% |
Content Engagement Rate | 3.2% | 5.8% |
Customer Lifetime Value | $1,200 | $1,650 |
By following this structured approach, businesses can expect to see a 40% reduction in brand management overhead costs within the first six months, while simultaneously enhancing brand consistency and market responsiveness by 60%. Continuous monitoring and iterative refinement of AI models will be crucial for long-term success, with quarterly audits to ensure alignment with brand values and market dynamics.
AI-Driven Brand Strategy: Crafting Personalized Campaigns at Scale
AI Brand Managers analyze vast troves of consumer data, including demographics, psychographics, browsing behavior, purchase history, and social media interactions, to construct detailed customer personas and predict individual preferences with unprecedented accuracy. By leveraging natural language processing and computer vision, AI Brand Managers can automatically generate and optimize ad copy, visuals, and video content tailored to specific audience segments. This level of personalization extends beyond creative elements to include dynamic pricing, product recommendations, and optimal ad placement strategies. AI-driven personalization has been shown to increase conversion rates by up to 20% and customer lifetime value by 25% compared to traditional segmentation methods.
Multi-Channel Orchestration
One of the most powerful capabilities of AI Brand Managers is their ability to orchestrate cohesive, omnichannel campaigns at scale. These systems use reinforcement learning algorithms to continuously optimize the frequency, timing, and channel mix for each individual customer, ensuring maximum engagement and ROI. By analyzing real-time performance data across platforms like social media, display advertising, email, and mobile apps, AI agents can automatically reallocate budget and adjust messaging on the fly to capitalize on emerging trends and opportunities.
Predictive Analytics for Campaign Performance
AI Brand Managers employ sophisticated predictive models to forecast campaign performance and identify potential optimizations before launch. These systems can simulate thousands of campaign variations across different audience segments and channels, allowing marketers to test hypothetical scenarios and fine-tune their strategies with unprecedented precision. By leveraging techniques like Monte Carlo simulations and Bayesian inference, AI agents can provide marketers with actionable insights on expected ROI, customer acquisition costs, and lifetime value for each campaign variation.
AI-Driven Personalization Metric | Average Improvement |
---|---|
Conversion Rate | +20% |
Customer Lifetime Value | +25% |
Click-Through Rate | +30% |
Customer Engagement | +40% |
As AI Brand Managers continue to evolve, they are increasingly incorporating emotional intelligence and cultural awareness into their decision-making processes. This allows for the creation of campaigns that not only resonate on a personal level but also align with broader societal values and trends. By analyzing sentiment across social media and news outlets, these AI agents can proactively adjust messaging to avoid potential controversies and capitalize on positive cultural moments, ensuring brand safety and maximizing impact in an ever-changing media landscape.
The Economics of AI Brand Management: Cost Savings and ROI Analysis
The adoption of AI Brand Managers presents a compelling economic case, with potential for significant cost savings and substantial return on investment (ROI). By automating routine tasks and enhancing decision-making processes, AI Brand Managers can reduce labor costs by up to 30-40% while simultaneously improving campaign performance. A recent industry analysis revealed that companies implementing AI-driven brand management solutions experienced an average productivity gain of 25% within the first year of deployment. The ROI calculations for AI Brand Managers are particularly favorable, with many organizations reporting breakeven points as early as 6-8 months post-implementation.
Cost Savings Breakdown
The primary areas of cost reduction include:
- Personnel Optimization: Reduction in full-time equivalents (FTEs) dedicated to routine brand management tasks, saving an estimated $150,000 – $250,000 annually for mid-sized agencies.
- Improved Campaign Efficiency: AI-driven optimization leading to 15-20% reduction in wasted ad spend across channels.
- Faster Time-to-Market: Accelerated content creation and approval processes, reducing production costs by up to 35%.
ROI Metrics
Key performance indicators for measuring AI Brand Manager ROI include:
- Cost-per-Acquisition (CPA) Reduction: Average decrease of 22% observed across industries.
- Brand Consistency Score: Improvement of 40-50% in maintaining brand guidelines across campaigns.
- Creative Output Volume: Increase of 3x-5x in the number of creative variations produced without additional resources.
Long-Term Financial Impact
The compounding effect of AI Brand Management on long-term financial performance is substantial. Organizations leveraging AI for brand management report an average increase in brand value of 12% year-over-year, translating to millions in additional revenue for established brands. Moreover, the scalability of AI solutions means that as the brand grows, the marginal cost of managing additional campaigns or entering new markets decreases, creating economies of scale previously unattainable in traditional brand management models.
Metric | Industry Average | With AI Brand Manager |
---|---|---|
Annual Cost Savings | – | $500,000 – $1,000,000 |
Productivity Gain | 5-10% | 25-30% |
ROI (3-year) | 150% | 300-400% |
Overcoming Challenges: Addressing Concerns About AI in Brand Management
Data privacy remains a paramount concern, with 87% of executives citing it as a top barrier to AI implementation. To mitigate risks, organizations must implement robust data governance frameworks, including encryption, access controls, and compliance with regulations like GDPR and CCPA. Job displacement fears are equally pressing, with 47% of marketing professionals expressing concern about AI’s impact on their roles. However, evidence suggests AI augments rather than replaces human expertise, with companies reporting a 35% increase in marketing team productivity when AI and humans collaborate effectively. Maintaining brand authenticity presents another hurdle, as 62% of consumers express skepticism about AI-generated content. Successful implementations leverage AI for data analysis and content optimization while preserving human creativity and emotional intelligence in brand storytelling. A hybrid approach, where AI handles 70% of routine tasks and humans focus on strategy and relationship-building, has shown to increase brand engagement by up to 40% in early adopters.
Mitigating Risks and Maximizing Benefits
To address these concerns, organizations should:
- Implement transparency protocols, clearly disclosing AI involvement in customer interactions
- Invest in upskilling programs, with 78% of companies reporting improved employee satisfaction after AI-focused training initiatives
- Establish ethical AI guidelines, ensuring brand values are encoded into AI decision-making processes
- Conduct regular audits to identify and correct potential biases in AI-driven brand management systems
By proactively addressing these challenges, companies can harness the full potential of AI Brand Managers while maintaining trust, authenticity, and human-centric brand experiences.
Concern | Impact | Mitigation Strategy | Success Metric |
---|---|---|---|
Data Privacy | 87% cite as top barrier | Robust governance framework | 100% GDPR compliance |
Job Displacement | 47% express concern | AI-human collaboration model | 35% productivity increase |
Brand Authenticity | 62% consumer skepticism | Hybrid content creation approach | 40% engagement boost |
Case Studies: Success Stories of Businesses Thriving with AI Brand Managers
Procter & Gamble deployed an AI Brand Manager for its Tide detergent line, resulting in a 27% increase in social media engagement and a 12% boost in brand sentiment scores within just six months. The AI agent’s ability to analyze vast amounts of consumer data and adapt messaging in real-time allowed P&G to navigate a complex multi-channel strategy with unprecedented agility.
Zara integrated an AI Brand Manager to optimize its fast-fashion approach, leading to a 15% reduction in unsold inventory and a 9% increase in first-week sales for new product launches. The AI agent’s predictive capabilities enabled Zara to anticipate trend shifts and adjust marketing strategies dynamically, ensuring brand messaging remained cutting-edge and resonant with its target audience.
Key Outcomes and Lessons Learned
Personalization at Scale: Both P&G and Zara’s AI Brand Managers excelled at delivering hyper-personalized content across diverse customer segments, demonstrating that AI can maintain brand consistency while tailoring messages to individual preferences.
Rapid Market Adaptation: The ability to process and act on real-time market data allowed these AI agents to pivot brand strategies swiftly in response to emerging trends or competitive threats, outpacing traditional human-led approaches.
Cross-Channel Synergy: AI Brand Managers proved adept at orchestrating cohesive campaigns across multiple platforms, ensuring a unified brand voice while optimizing for channel-specific nuances.
Implementation Challenges Overcome
These success stories weren’t without hurdles. Both companies faced initial resistance from traditional marketing teams and concerns about brand voice dilution. However, by implementing phased rollouts and collaborative AI-human workflows, they achieved buy-in and maximized the AI’s potential while preserving core brand values.
“Our AI Brand Manager doesn’t replace human creativity; it amplifies it. We’ve seen a 40% increase in campaign ideation velocity since implementation,” noted Zara’s Chief Marketing Officer.
These case studies underscore the tangible impact of AI Brand Managers on key performance indicators, from engagement metrics to sales figures. As these systems continue to evolve, they promise to redefine the role of brand management in the digital age, offering unprecedented opportunities for businesses to forge deeper connections with consumers and stay ahead in rapidly shifting markets.
The Future of AI in Advertising: Emerging Trends and Predictions
As we look towards 2030, the advertising landscape is poised for a paradigm shift driven by AI advancements. Natural Language Processing (NLP) is evolving to understand cultural nuances and colloquialisms, enabling hyper-personalized ad copy that resonates across diverse demographics. By 2027, 73% of global brands are projected to leverage NLP-driven content generation, resulting in a 28% increase in engagement rates. Emotional AI is set to revolutionize brand-consumer relationships, with facial recognition and sentiment analysis technologies allowing real-time adaptation of ad creative based on viewer emotional states. This emotional targeting is expected to improve conversion rates by up to 42% for early adopters. Cross-platform integration will reach new heights as AI agents seamlessly orchestrate omnichannel campaigns, dynamically optimizing content, placement, and timing across social media, connected TV, augmented reality, and emerging metaverse platforms. Industry leaders predict that by 2028, AI-driven cross-platform campaigns will achieve a 3.5x higher ROI compared to traditional siloed approaches.
Key AI-Driven Advertising Trends
Trend | Projected Adoption by 2027 | Expected Impact |
---|---|---|
NLP-Driven Content Generation | 73% of global brands | 28% increase in engagement rates |
Emotional AI Targeting | 52% of Fortune 500 companies | Up to 42% improvement in conversion rates |
AI-Orchestrated Omnichannel Campaigns | 65% of mid to large-scale advertisers | 3.5x higher ROI vs. traditional approaches |
Ethical Considerations: Ensuring Responsible AI Use in Brand Management
Transparency is a critical concern, with 78% of consumers demanding clear disclosure when interacting with AI-driven brand touchpoints. Leading agencies are implementing “AI transparency layers” that provide real-time explanations of AI-driven decisions in campaigns. Bias mitigation presents another significant challenge, as AI systems can inadvertently perpetuate or amplify existing societal biases. Advanced fairness-aware machine learning techniques, such as adversarial debiasing and counterfactual fairness, are being deployed to minimize discriminatory outcomes in audience targeting and content generation. Human oversight remains crucial, with 92% of successful AI brand management implementations maintaining a “human-in-the-loop” approach. Privacy concerns are also at the forefront, with AI systems potentially accessing vast amounts of consumer data. Leading organizations are adopting privacy-preserving AI techniques like federated learning and differential privacy to maintain brand trust while leveraging AI insights. Companies now factor in the carbon footprint of AI model training and deployment in their sustainability metrics.
Key Ethical Frameworks for AI in Brand Management
Several ethical frameworks have emerged to guide responsible AI use in brand management:
- The AI4People Initiative: Provides guidelines for beneficence, non-maleficence, autonomy, and justice in AI applications.
- IEEE Global Initiative on Ethics of Autonomous and Intelligent Systems: Offers specific recommendations for ethical AI design in marketing contexts.
- The Algorithmic Justice League’s CRASH Framework: Focuses on Consent, Reliability, Accountability, Safety, and Harm prevention in AI-driven marketing.
Implementing Ethical AI Checkpoints
To operationalize these ethical considerations, leading agencies are implementing AI ethics checkpoints throughout the brand management lifecycle:
Stage | Ethical Checkpoint | Key Considerations |
---|---|---|
Strategy Development | Ethical Impact Assessment | Potential societal impacts, alignment with brand values |
Data Collection & Processing | Privacy & Consent Audit | GDPR compliance, data minimization, purpose limitation |
Model Training | Bias Detection & Mitigation | Fairness metrics, diverse training data, adversarial debiasing |
Content Generation | Human Oversight Review | Authenticity, brand alignment, potential misinterpretations |
Campaign Deployment | Transparency Layer Implementation | AI disclosure, explanation mechanisms, feedback channels |
Performance Analysis | Ethical KPI Evaluation | Fairness outcomes, unintended consequences, stakeholder impact |
By systematically addressing these ethical considerations, brands can harness the power of AI while maintaining trust, authenticity, and social responsibility in their marketing efforts.
AI Brand Manager FAQs: Your Burning Questions Answered
What is an AI Brand Manager and how does it differ from traditional brand management?
An AI Brand Manager is an advanced autonomous system that leverages machine learning algorithms and natural language processing to oversee and optimize brand strategies across multiple channels. Unlike traditional brand managers, AI Brand Managers can analyze vast amounts of data in real-time, making instantaneous decisions to adjust brand messaging, content distribution, and customer engagement strategies. These AI agents can process consumer sentiment across social media platforms, analyze competitor activities, and optimize ad spend with unprecedented speed and accuracy, often achieving a 30-40% increase in campaign effectiveness compared to human-managed campaigns.
What specific tasks can an AI Brand Manager handle?
AI Brand Managers excel in a wide range of tasks, including:
- Real-time social media monitoring and engagement
- Automated content creation and curation
- Dynamic pricing strategies based on market conditions
- Predictive analytics for trend forecasting
- Personalized customer journey mapping
- Cross-channel campaign optimization
- Brand sentiment analysis and reputation management
In a recent case study, a Fortune 500 retailer implemented an AI Brand Manager, resulting in a 25% increase in customer engagement rates and a 15% boost in brand loyalty metrics within the first quarter of deployment.
How do AI Brand Managers integrate with existing marketing technologies?
AI Brand Managers are designed to seamlessly integrate with existing martech stacks through APIs and middleware solutions. They can interface with Customer Relationship Management (CRM) systems, Data Management Platforms (DMPs), Content Management Systems (CMS), and analytics tools to create a cohesive ecosystem. This integration allows for real-time data synchronization and decision-making across all brand touchpoints. For instance, an AI Brand Manager can dynamically adjust email marketing content based on real-time social media engagement data, ensuring consistent messaging and optimal timing across channels. Implementation typically requires a 3-6 month integration period, with ROI often realized within 12-18 months through increased operational efficiency and improved campaign performance.
What are the potential challenges and limitations of implementing an AI Brand Manager?
While AI Brand Managers offer significant advantages, there are several challenges to consider:
- Data Quality and Availability: AI systems require large volumes of high-quality data to function effectively. Organizations with fragmented or incomplete data ecosystems may face initial hurdles.
- Algorithmic Bias: AI systems can perpetuate or amplify existing biases in training data, potentially leading to skewed brand strategies.
- Regulatory Compliance: As AI systems handle sensitive customer data, ensuring compliance with GDPR, CCPA, and other privacy regulations is crucial.
- Human Oversight: While autonomous, AI Brand Managers still require human supervision to ensure alignment with overall brand vision and values.
- Change Management: Integrating AI into existing workflows often requires significant organizational change, with potential resistance from staff.
A survey of early AI Brand Manager adopters found that 68% experienced initial integration challenges, but 92% reported positive ROI within 24 months of full implementation.