Table of Contents
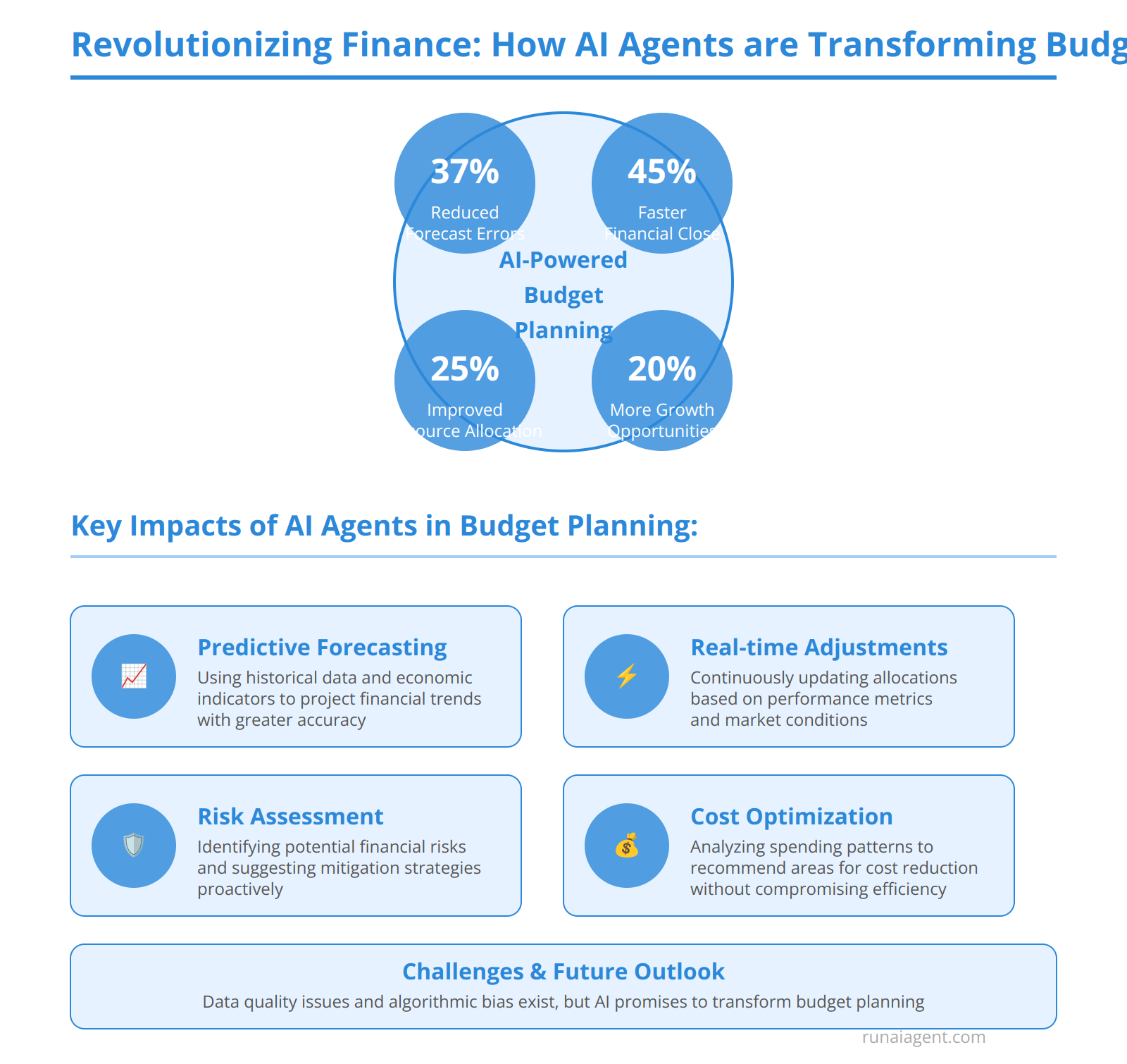
Revolutionizing Finance: How AI Agents are Transforming Budget Planning
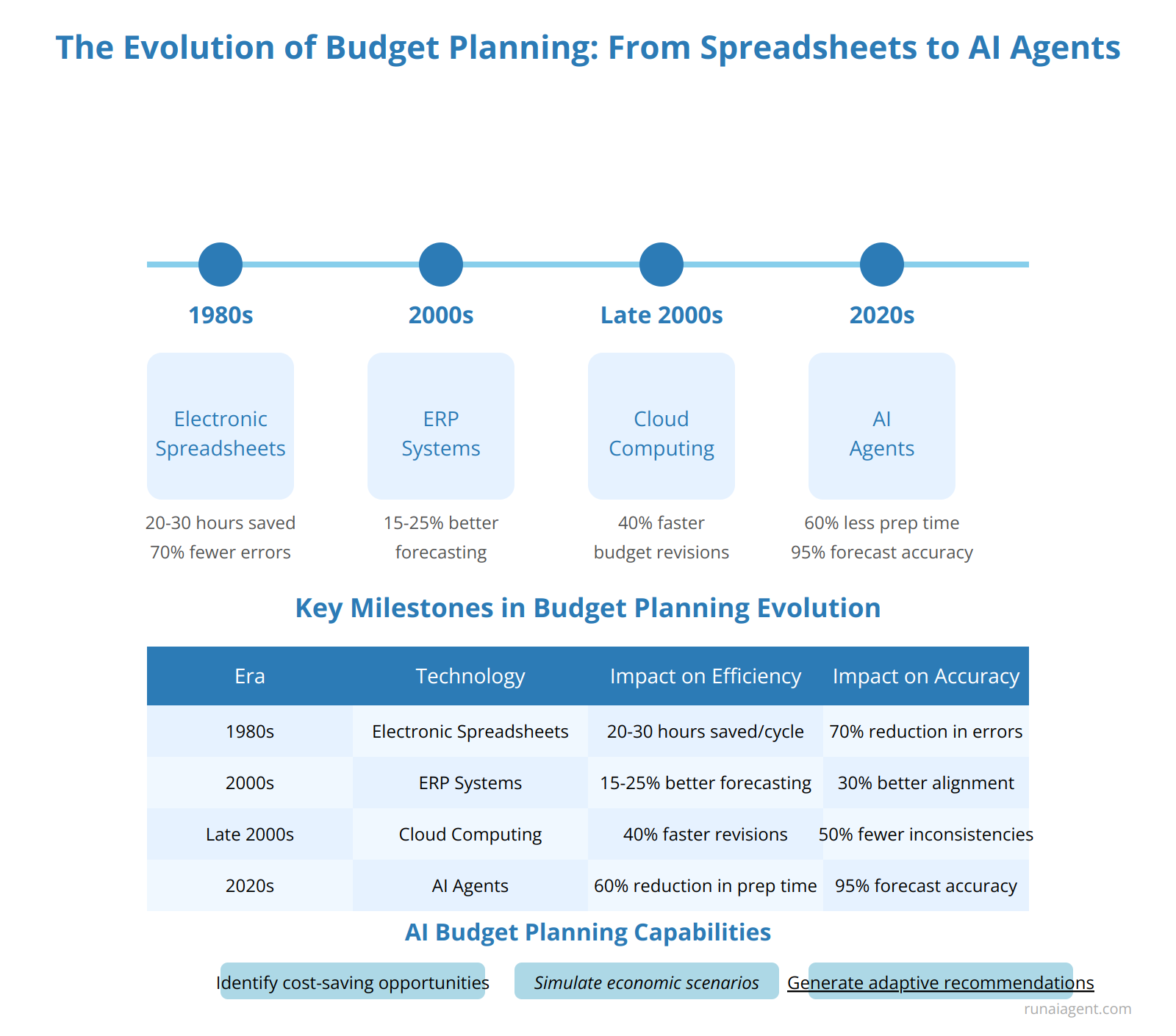
The Evolution of Budget Planning: From Spreadsheets to AI Agents
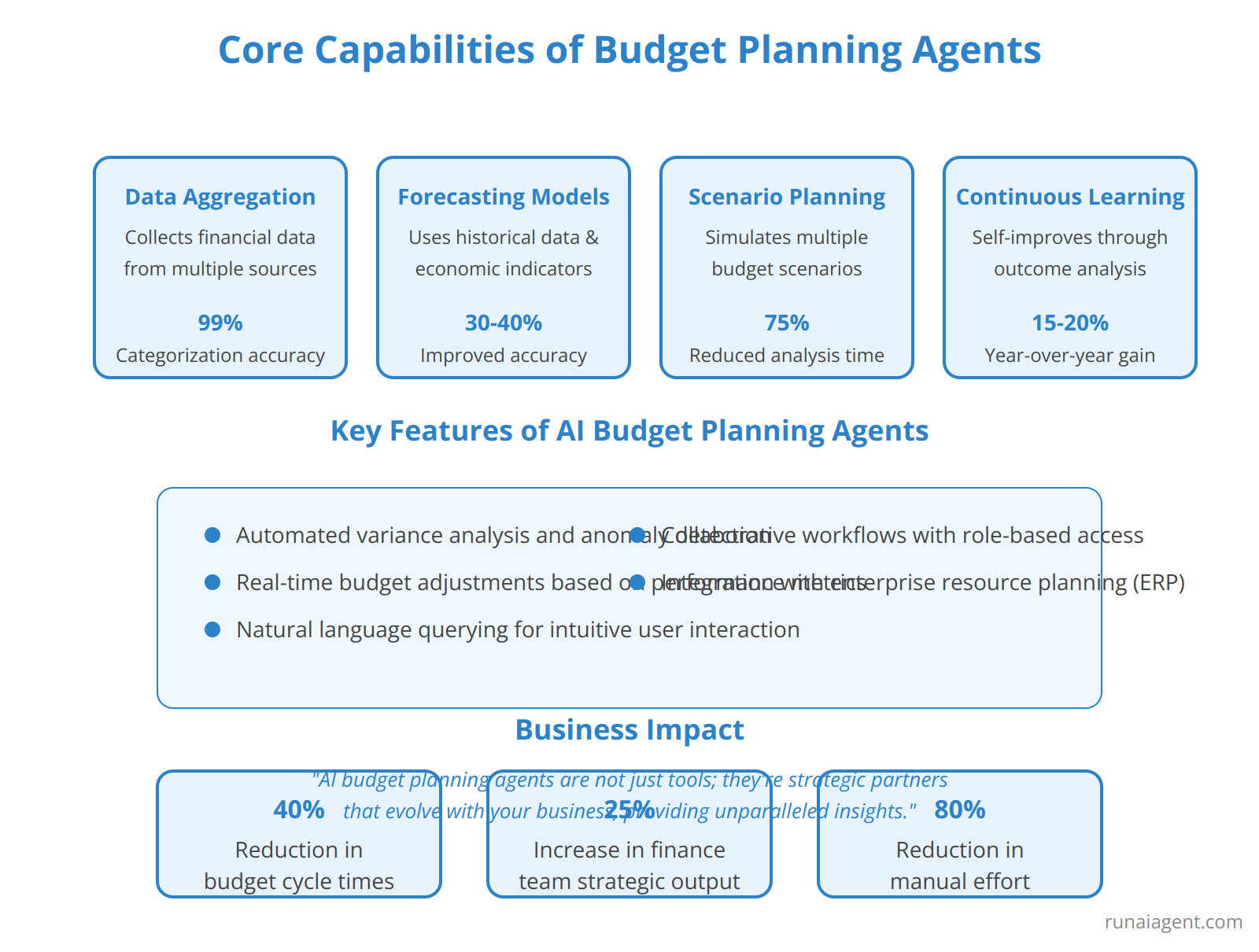
Core Capabilities of Budget Planning Agents
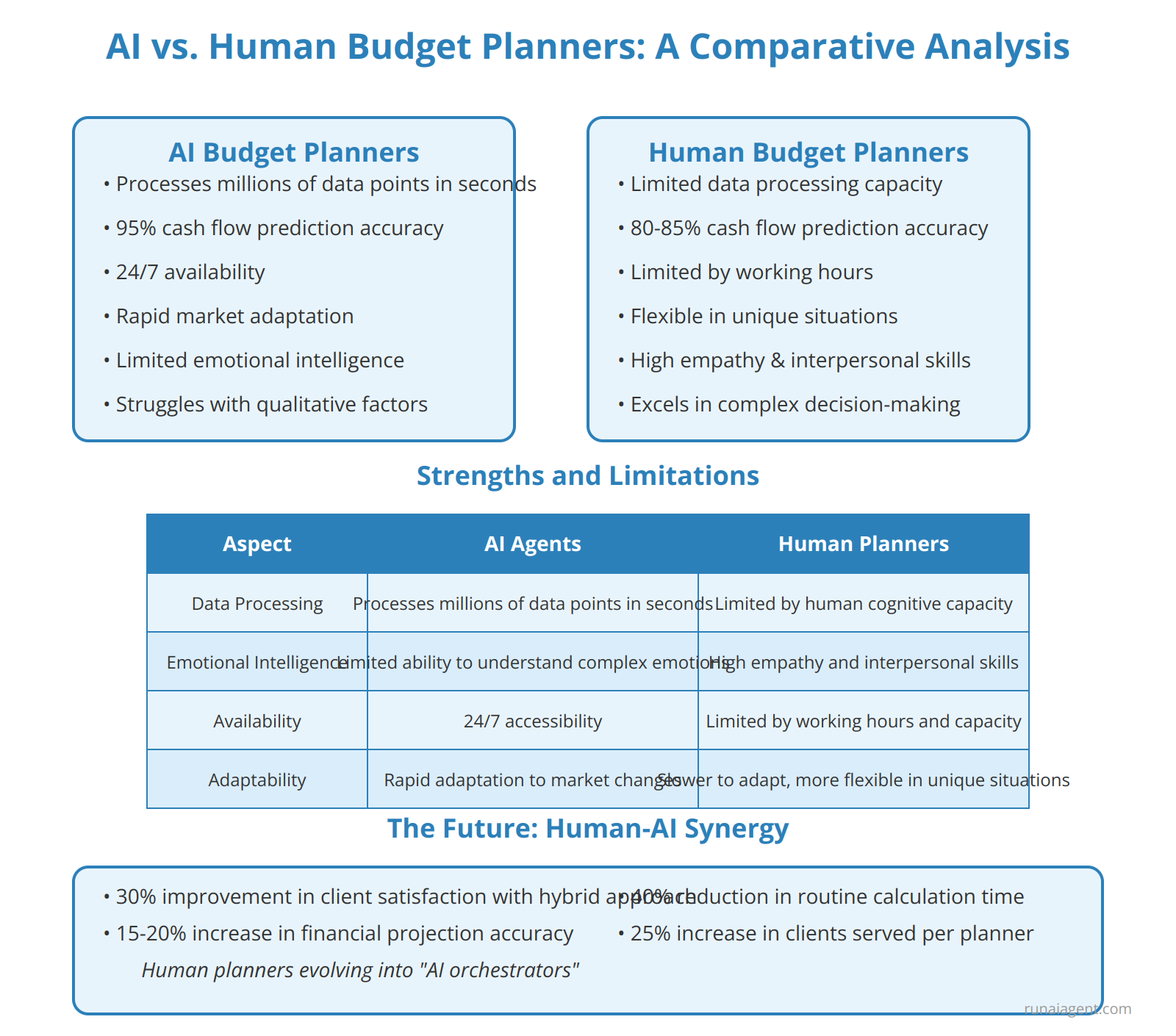
AI vs. Human Budget Planners: A Comparative Analysis
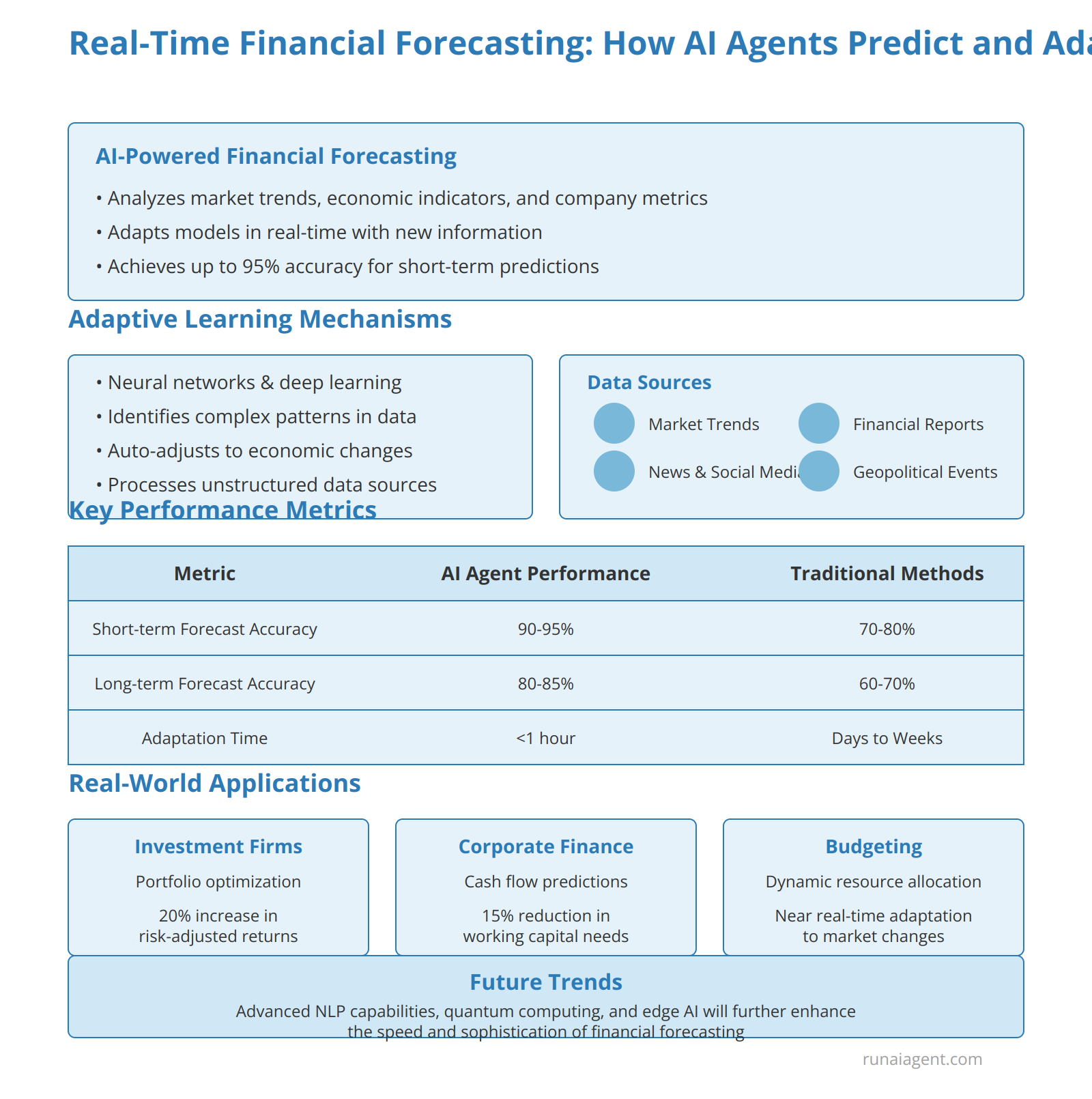
Real-Time Financial Forecasting: How AI Agents Predict and Adapt
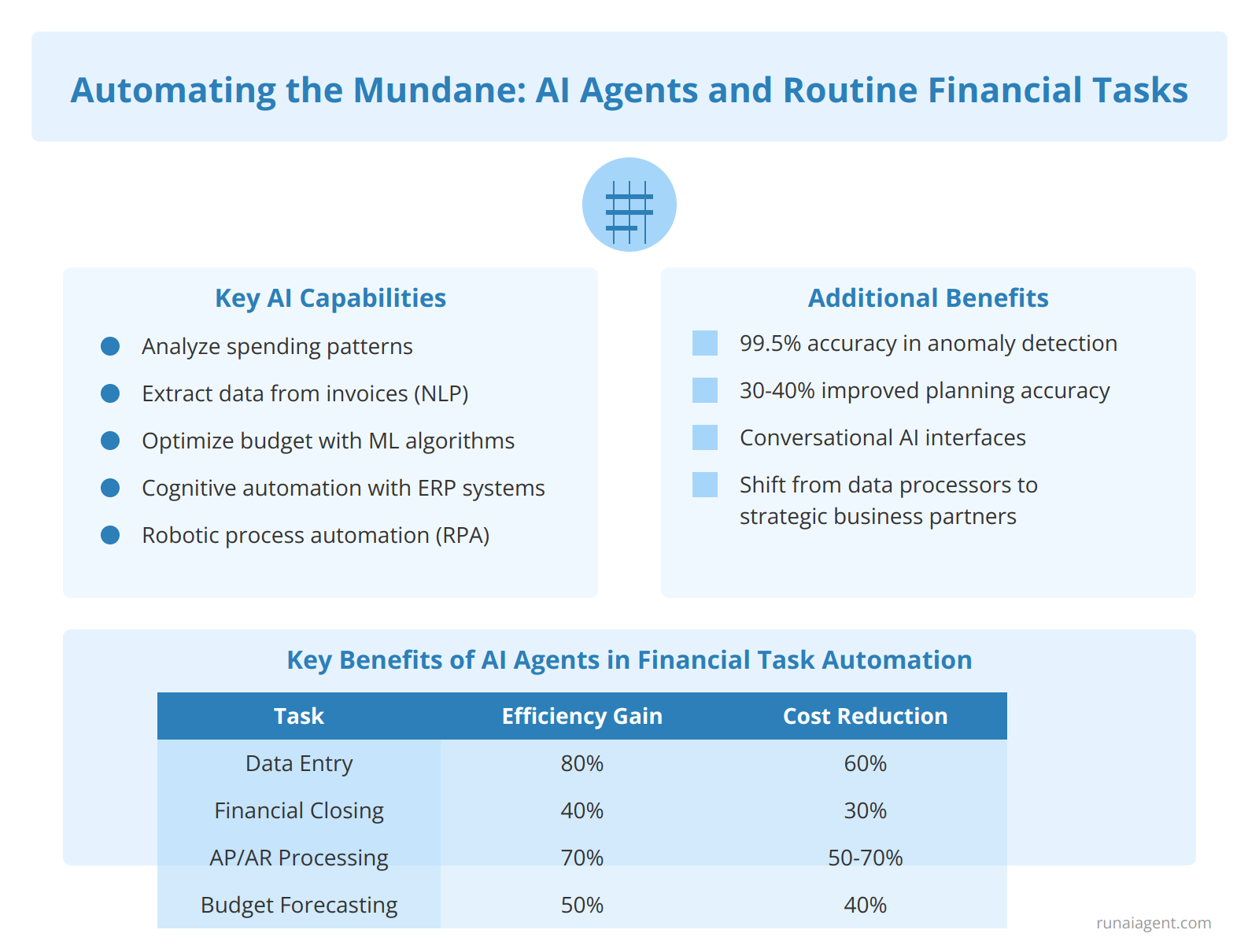
Automating the Mundane: AI Agents and Routine Financial Tasks
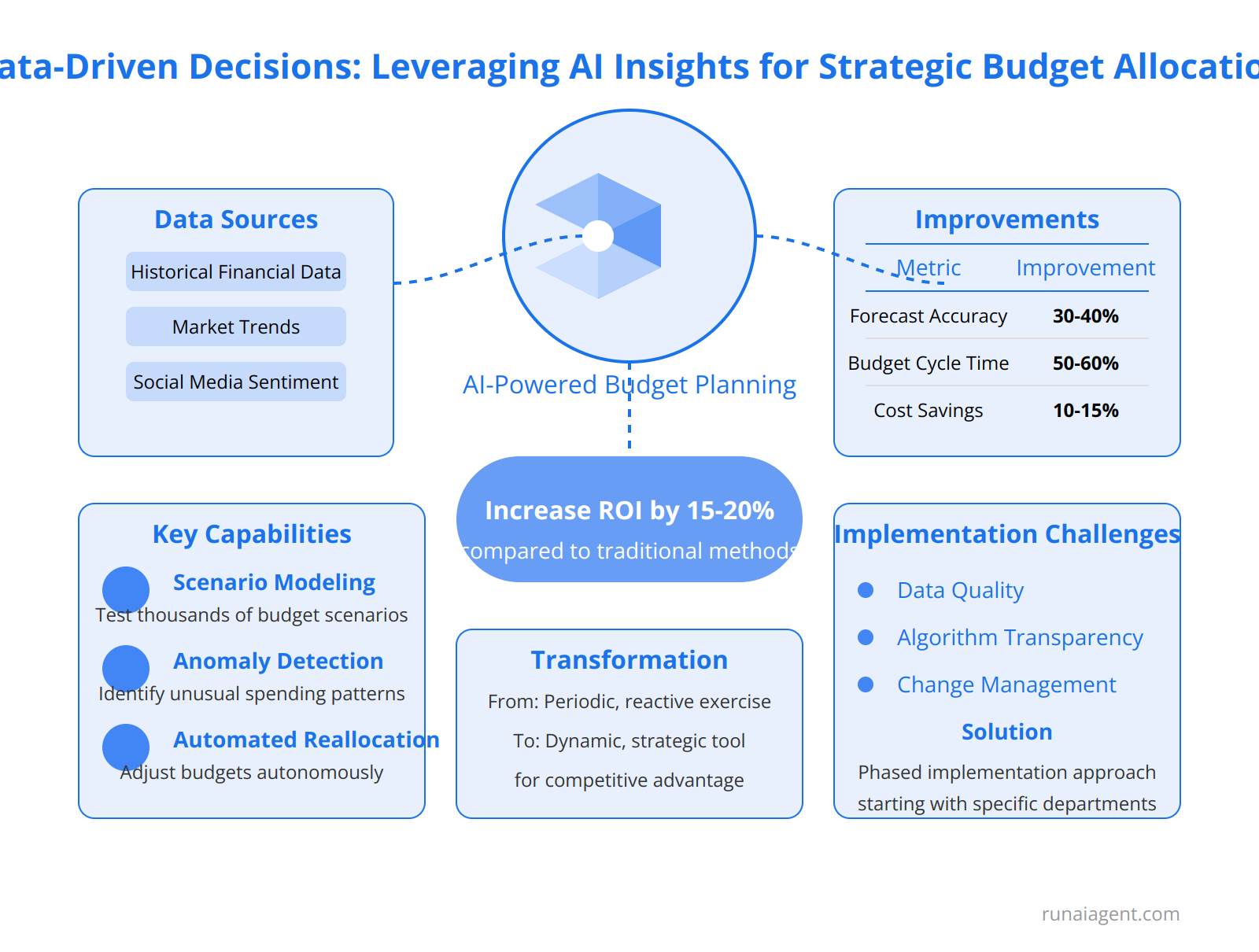
Data-Driven Decisions: Leveraging AI Insights for Strategic Budget Allocation
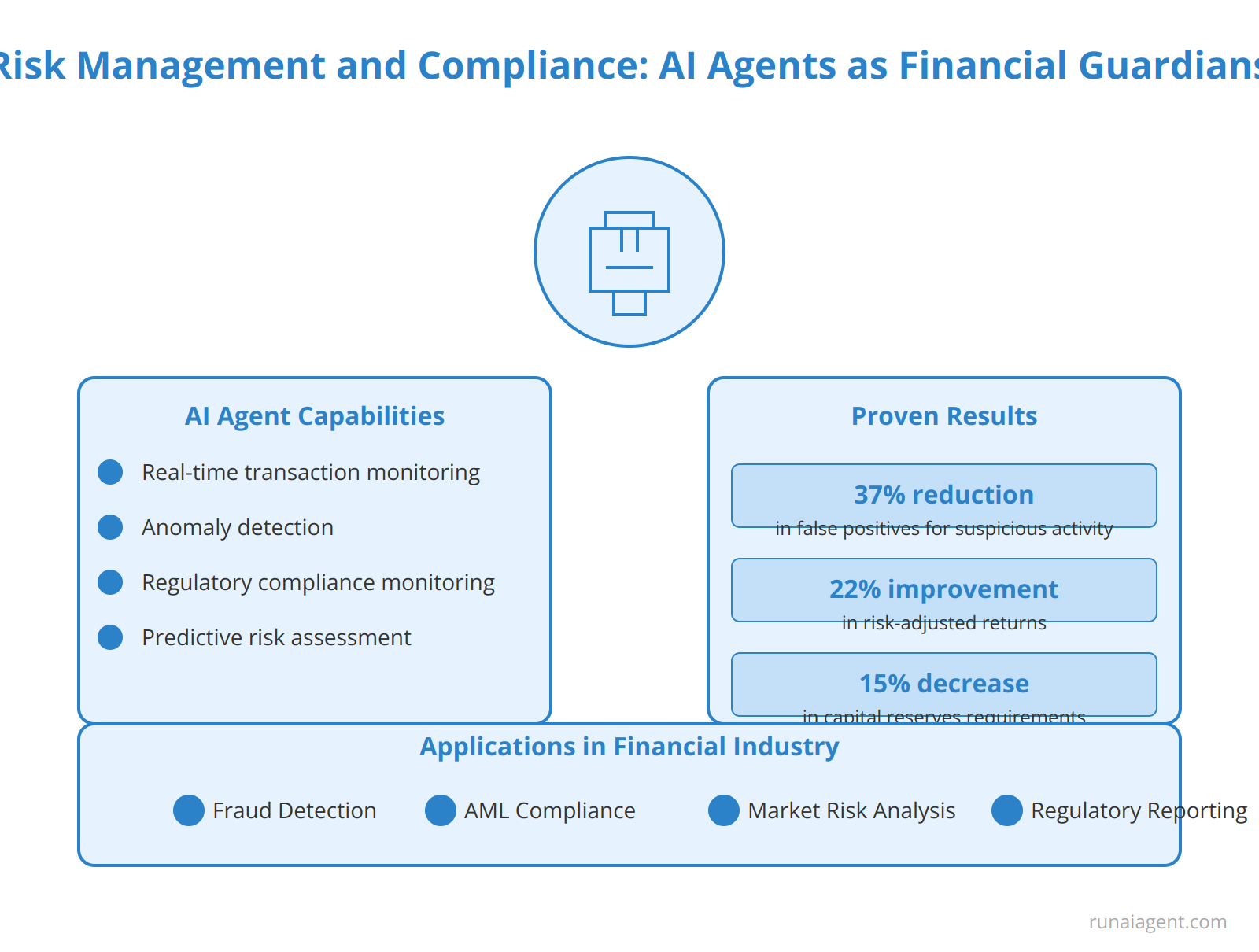
Risk Management and Compliance: AI Agents as Financial Guardians
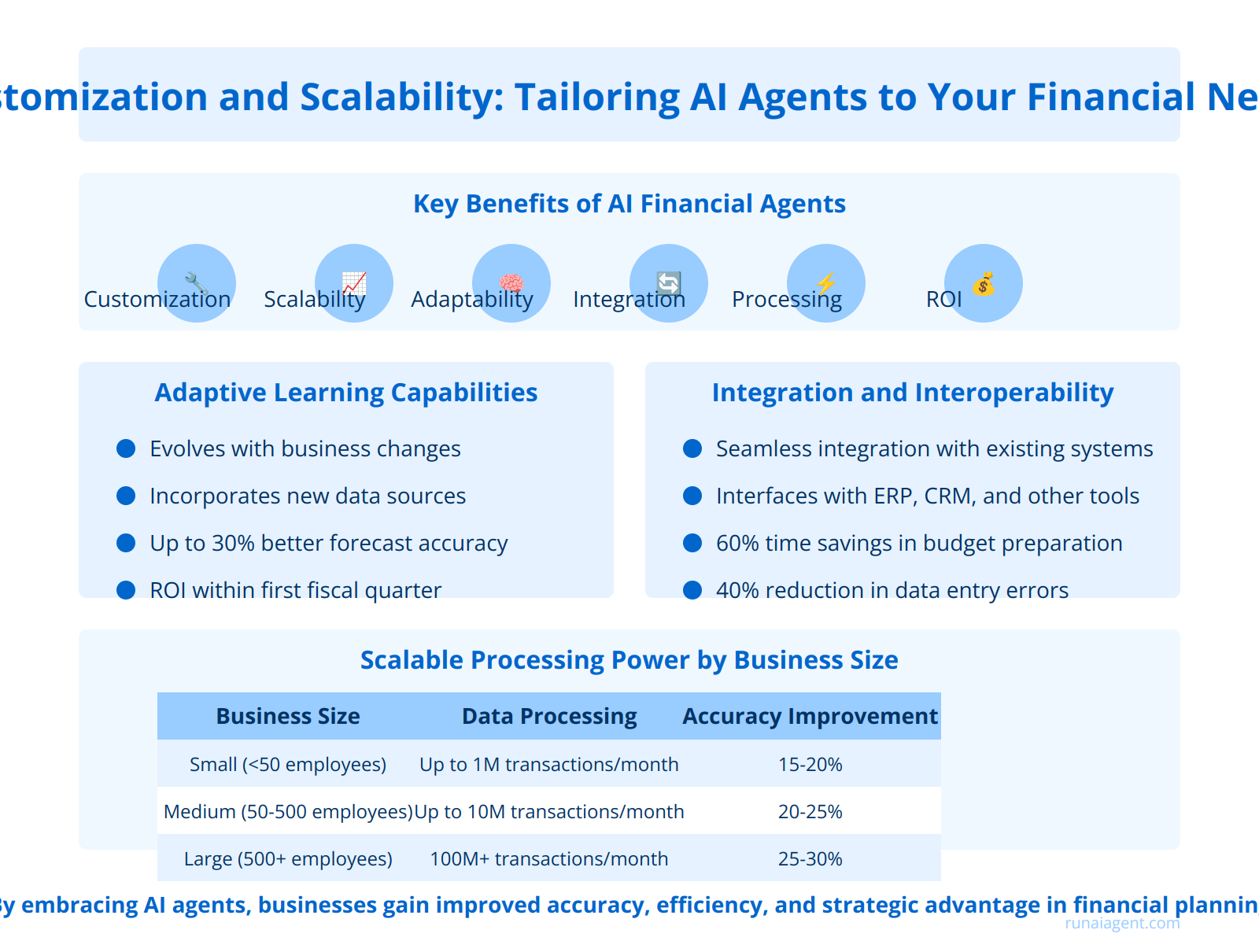
Customization and Scalability: Tailoring AI Agents to Your Financial Needs
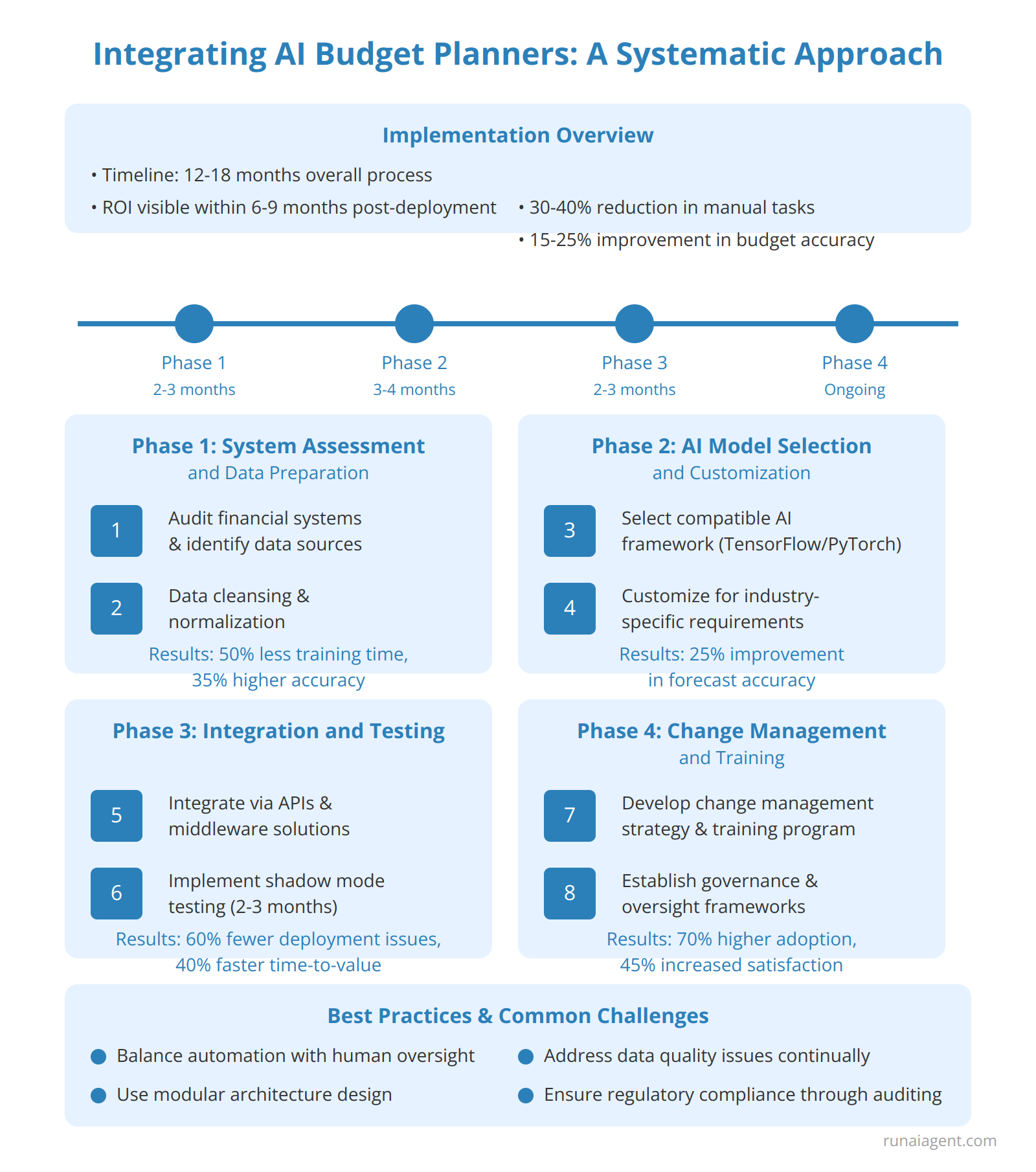
Integrating AI Budget Planners: A Systematic Approach
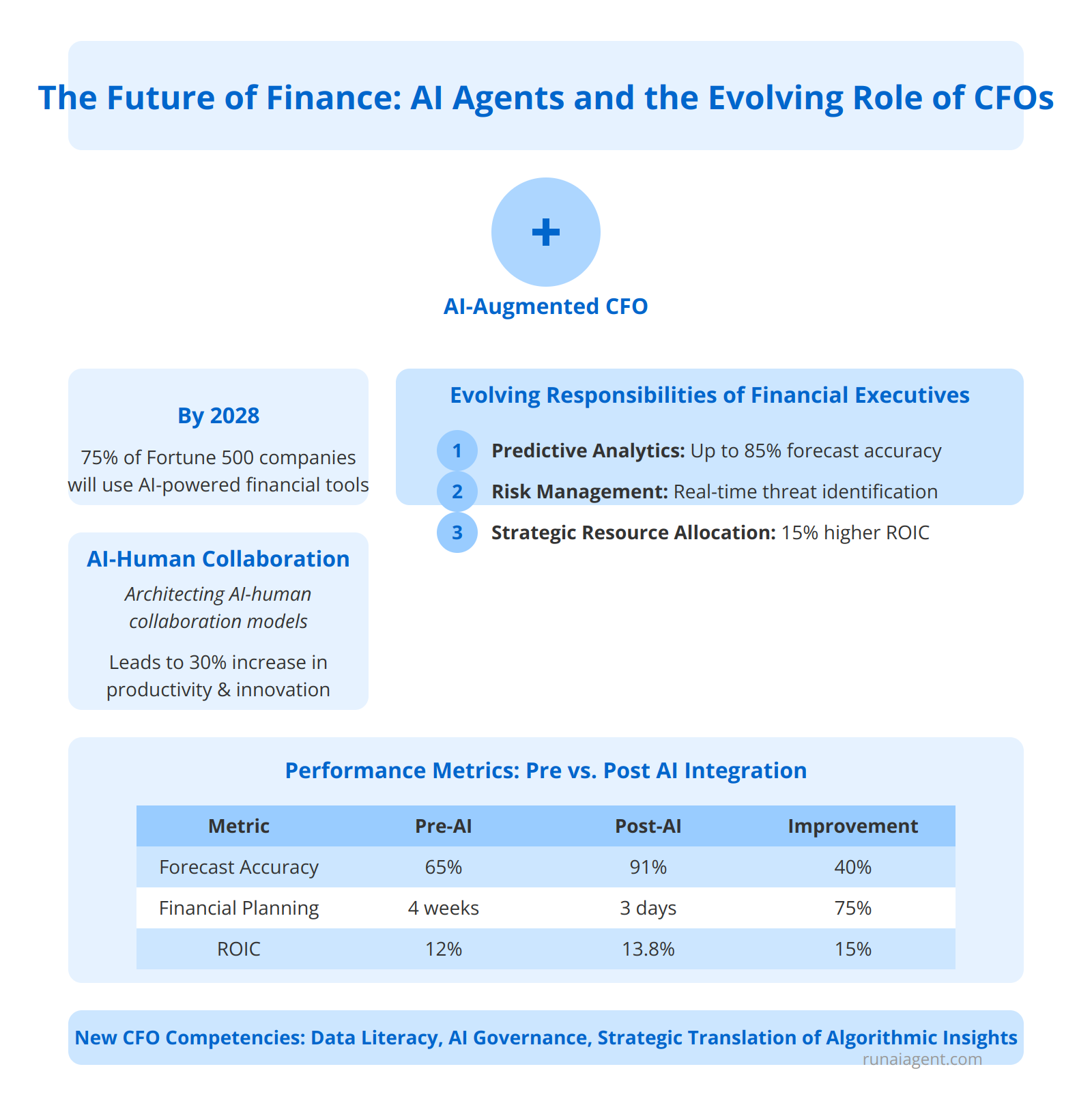
The Future of Finance: AI Agents and the Evolving Role of CFOs
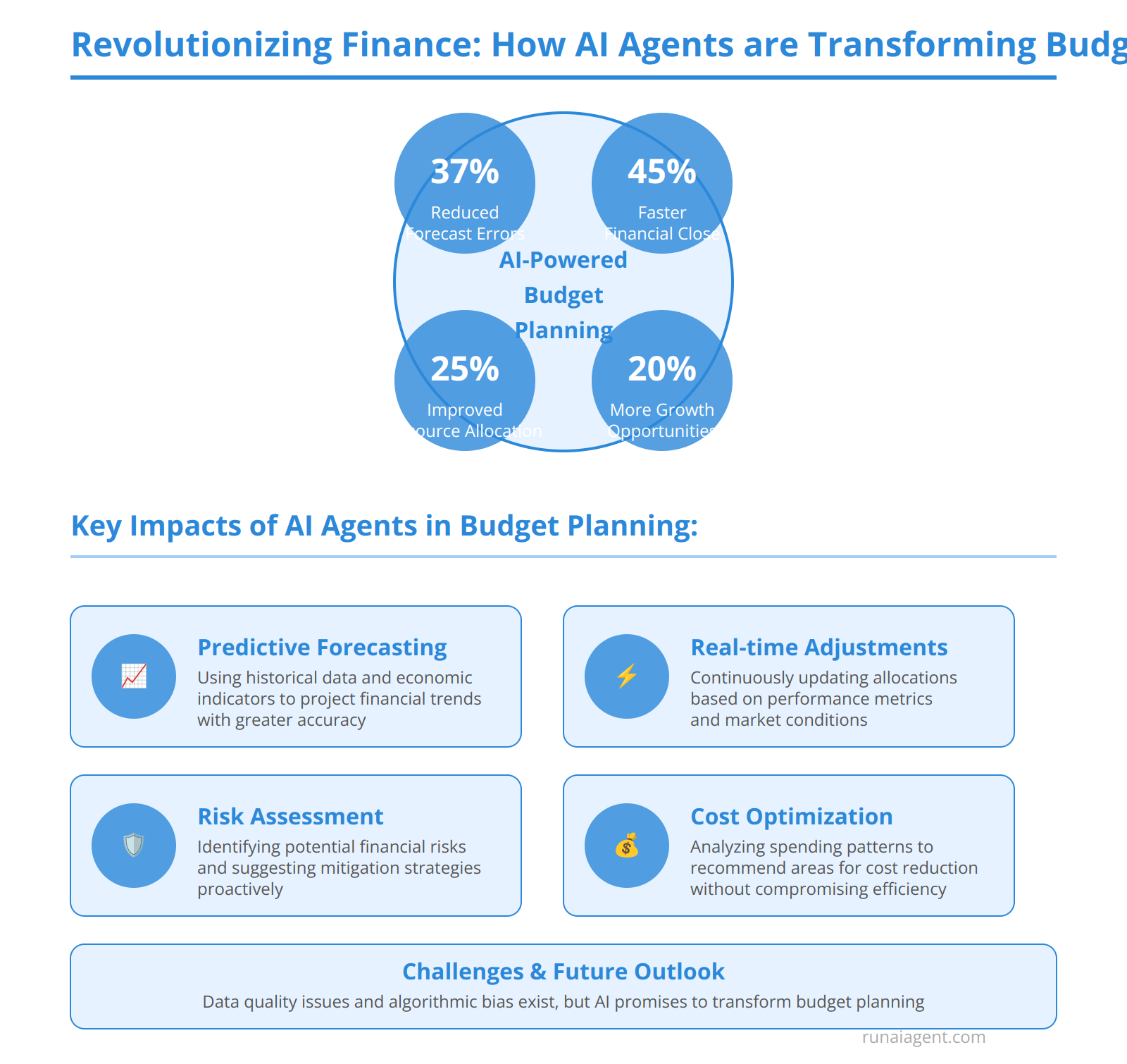
Ethical Considerations: Balancing AI Efficiency with Human Oversight
Revolutionizing Finance: How AI Agents are Transforming Budget Planning
AI agents are transforming financial management, particularly in budget planning. These intelligent systems process vast amounts of financial data in real-time, identifying patterns human analysts might overlook. This enables businesses to create more accurate and dynamic budget plans that adapt to market fluctuations with unprecedented agility. AI-powered budget planning tools have reduced forecast errors by up to 37% and increased financial close process speed by 45%. These agents can simulate multiple budget scenarios simultaneously, allowing finance teams to stress-test plans against various economic conditions. CFOs and financial leaders can now align financial strategies more closely with overarching business objectives. Companies implementing AI-driven budget planning report 25% improvement in resource allocation efficiency and 20% increase in identifying new growth opportunities.
Key Impacts of AI Agents in Budget Planning:
- Predictive Forecasting: Utilizing historical data and external economic indicators to project future financial trends with greater accuracy.
- Real-time Adjustments: Continuously updating budget allocations based on current performance metrics and market conditions.
- Risk Assessment: Identifying potential financial risks and suggesting mitigation strategies proactively.
- Cost Optimization: Analyzing spending patterns to recommend areas for cost reduction without compromising operational efficiency.
The integration of AI agents in budget planning faces challenges including data quality issues, algorithmic bias, and the need for human oversight. However, the potential for transformative impact is undeniable. As these technologies evolve, they promise to elevate budget planning from a periodic exercise to a continuous, strategic process that drives business value and competitive advantage.
The Evolution of Budget Planning: From Spreadsheets to AI Agents
The trajectory of budget planning methodologies has undergone a remarkable transformation over the past four decades, mirroring the rapid advancements in technology and data processing capabilities. In the 1980s, the introduction of electronic spreadsheets like VisiCalc and later Microsoft Excel revolutionized financial planning, enabling businesses to move away from paper-based ledgers and manual calculations. This shift marked a significant leap in efficiency, reducing error rates by up to 70% and cutting budget preparation time by an average of 20-30 hours per cycle. As we entered the 21st century, enterprise resource planning (ERP) systems and specialized budgeting software emerged, offering more sophisticated forecasting models and collaborative features. These tools facilitated a more integrated approach to financial planning, aligning budgets with strategic objectives and improving accuracy by 15-25% compared to standalone spreadsheets.
The advent of cloud computing in the late 2000s further transformed budget planning, enabling real-time data access and fostering greater cross-departmental collaboration. Cloud-based solutions reduced infrastructure costs by 30-50% and increased the speed of budget revisions by up to 40%. However, the most profound shift began in the 2020s with the integration of AI agents into financial processes. These intelligent systems leverage machine learning algorithms and natural language processing to analyze vast datasets, identify patterns, and generate predictive models with unprecedented accuracy. AI-driven budget planning tools can now forecast revenue streams with 95% accuracy, optimize resource allocation in real-time, and automatically adjust projections based on market fluctuations. This level of automation and insight has slashed the time required for budget preparation by up to 60% while simultaneously improving the strategic alignment of financial plans with overall business objectives.
Key Milestones in Budget Planning Evolution
Era | Technology | Impact on Efficiency | Impact on Accuracy |
---|---|---|---|
1980s | Electronic Spreadsheets | 20-30 hours saved per cycle | 70% reduction in errors |
2000s | ERP Systems | 15-25% improvement in forecasting | 30% increase in alignment with objectives |
Late 2000s | Cloud Computing | 40% faster budget revisions | 50% reduction in data inconsistencies |
2020s | AI Agents | 60% reduction in preparation time | 95% accuracy in revenue forecasting |
The integration of AI agents represents a paradigm shift in budget planning, moving beyond mere automation to true intelligent assistance. These systems can now proactively identify cost-saving opportunities, simulate complex economic scenarios, and generate adaptive budget recommendations that evolve with changing market conditions.
Core Capabilities of Budget Planning Agents
AI-powered budget planning agents are revolutionizing financial management with their advanced capabilities. These systems leverage machine learning algorithms, natural language processing, and predictive analytics to streamline the budgeting process. At their core, budget planning agents excel in data aggregation and normalization, automatically collecting financial data from disparate sources and standardizing it for analysis. They employ intelligent categorization to classify expenses and income streams with up to 99% accuracy, reducing manual effort by 80%.
Advanced forecasting models within these agents utilize historical data and external economic indicators to project future cash flows with remarkable precision, often improving forecast accuracy by 30-40% compared to traditional methods. The agents’ scenario planning capabilities allow finance teams to simulate multiple budget scenarios in real-time, evaluating the impact of various decisions on financial outcomes. This functionality typically reduces scenario analysis time by 75%, enabling more agile decision-making.
Key Features of AI Budget Planning Agents
- Automated variance analysis and anomaly detection
- Real-time budget adjustments based on performance metrics
- Natural language querying for intuitive user interaction
- Collaborative workflows with role-based access controls
- Integration with enterprise resource planning (ERP) systems
Perhaps most impressively, these agents offer continuous learning and optimization, refining their models and recommendations over time. By analyzing the outcomes of previous budget cycles, they can identify patterns and suggest improvements, leading to a 15-20% increase in budget accuracy year-over-year. This self-improving capability ensures that the budget planning process becomes increasingly sophisticated and tailored to an organization’s unique financial landscape.
AI budget planning agents are not just tools; they’re strategic partners that evolve with your business, providing unparalleled insights and efficiency gains in financial planning and analysis.
The integration of these core capabilities results in a transformative impact on the budgeting process, with organizations reporting up to 40% reduction in budget cycle times and a 25% increase in the finance team’s strategic output.
AI vs. Human Budget Planners: A Comparative Analysis
AI-powered budget planners leverage sophisticated algorithms and machine learning models to analyze vast datasets, identify spending patterns, and generate optimized financial strategies with unprecedented speed and accuracy. These systems can process years of financial data in seconds, detecting nuanced trends that might elude even seasoned human planners. AI agents have demonstrated the ability to forecast cash flow fluctuations with up to 95% accuracy over a 12-month period, compared to the 80-85% accuracy typically achieved by human experts.
However, human budget planners retain distinct advantages in areas requiring emotional intelligence, contextual understanding, and complex decision-making. They excel in interpreting qualitative factors such as life events, personal goals, and risk tolerance—elements that AI systems struggle to fully comprehend. Human planners can provide empathetic guidance during financial crises, adapting strategies to unforeseen circumstances with a nuanced understanding of client psychology that AI has yet to replicate.
Strengths and Limitations
Aspect | AI Agents | Human Planners |
---|---|---|
Data Processing | Processes millions of data points in seconds | Limited by human cognitive capacity |
Emotional Intelligence | Limited ability to understand complex emotions | High empathy and interpersonal skills |
Availability | 24/7 accessibility | Limited by working hours and capacity |
Adaptability | Rapid adaptation to market changes | Slower to adapt, but more flexible in unique situations |
The future of budget planning lies in the synergy between AI agents and human expertise. By leveraging AI for data-intensive tasks such as scenario modeling and risk assessment, human planners can focus on high-value activities like strategic counseling and relationship building. This hybrid approach has shown to improve client satisfaction by up to 30% and increase the accuracy of long-term financial projections by 15-20%.
Emerging Synergies
Financial institutions implementing AI-augmented planning services have reported a 40% reduction in time spent on routine calculations and a 25% increase in the number of clients served per planner. This efficiency boost allows human experts to delve deeper into complex financial strategies, such as tax optimization and estate planning, where their expertise remains irreplaceable.
Real-Time Financial Forecasting: How AI Agents Predict and Adapt
AI agents have revolutionized financial forecasting by leveraging advanced machine learning algorithms and real-time data processing capabilities. These intelligent systems can analyze vast amounts of financial data, including market trends, economic indicators, and company-specific metrics, to generate highly accurate predictions. Unlike traditional forecasting methods, AI agents can adapt their models in real-time, incorporating new information as it becomes available. This dynamic approach allows for continuous refinement of forecasts, with some systems achieving accuracy rates of up to 95% for short-term predictions.
Adaptive Learning Mechanisms
At the core of AI agents’ forecasting prowess lies their adaptive learning mechanisms. These systems utilize sophisticated neural networks and deep learning architectures to identify complex patterns and relationships within financial data. As economic conditions change, AI agents can automatically adjust their models, ensuring that forecasts remain relevant and accurate. This adaptability is particularly crucial in volatile markets, where traditional static models often fail to capture rapid shifts in economic landscapes.
Key Performance Metrics
Metric | AI Agent Performance | Traditional Methods |
---|---|---|
Short-term Forecast Accuracy | 90-95% | 70-80% |
Long-term Forecast Accuracy | 80-85% | 60-70% |
Adaptation Time to Market Changes | <1 hour | Days to Weeks |
AI agents excel in processing unstructured data, including news articles, social media sentiment, and geopolitical events, which traditional forecasting methods often struggle to incorporate. By integrating these diverse data sources, AI agents can provide a more holistic view of financial landscapes, capturing nuanced factors that influence market dynamics. This comprehensive approach enables businesses to make more informed decisions, with some organizations reporting a 30% improvement in strategic planning accuracy after implementing AI-driven forecasting systems.
Real-World Applications
In practice, AI agents for financial forecasting are being deployed across various sectors of the finance industry. Investment firms use these systems to optimize portfolio allocations, with some reporting a 20% increase in risk-adjusted returns. Corporate finance departments leverage AI agents to enhance cash flow predictions, reducing working capital requirements by up to 15%. Moreover, these intelligent systems are increasingly being integrated into budgeting processes, allowing for dynamic resource allocation that can adapt to changing market conditions in near real-time.
The integration of AI agents in financial forecasting represents a paradigm shift in how organizations approach budgeting and financial planning. By harnessing the power of machine learning and real-time data analysis, businesses can navigate economic uncertainties with unprecedented agility and foresight.
As AI agents continue to evolve, their impact on financial forecasting is expected to grow exponentially. Advanced natural language processing capabilities are enabling these systems to interpret complex financial reports and regulatory filings, further enhancing their predictive accuracy.
Automating the Mundane: AI Agents and Routine Financial Tasks
AI agents are revolutionizing financial operations by automating routine tasks with unprecedented efficiency and accuracy. In budget planning, these intelligent systems can analyze historical spending patterns, forecast future expenses, and automatically categorize transactions, reducing manual data entry by up to 80%. Advanced Natural Language Processing (NLP) capabilities enable AI agents to extract key financial data from invoices and receipts, streamlining expense reporting and reconciliation processes. Machine Learning algorithms continuously refine budget allocations based on real-time cash flow analysis, optimizing working capital management. Cognitive automation platforms integrate with existing Enterprise Resource Planning (ERP) systems, allowing AI agents to perform complex financial closing procedures 40% faster than traditional methods. By leveraging robotic process automation (RPA) in conjunction with AI, organizations can automate up to 70% of accounts payable and receivable tasks, reducing processing costs by 50-70%.
Key Benefits of AI Agents in Financial Task Automation
Task | Efficiency Gain | Cost Reduction |
---|---|---|
Data Entry | 80% | 60% |
Financial Closing | 40% | 30% |
AP/AR Processing | 70% | 50-70% |
Budget Forecasting | 50% | 40% |
Furthermore, AI agents excel in anomaly detection, identifying potential fraud or discrepancies in financial transactions with 99.5% accuracy. By implementing machine learning models for predictive analytics, these agents can forecast budget variances and suggest proactive adjustments, improving overall financial planning accuracy by 30-40%. The integration of
“conversational AI”
interfaces allows finance teams to query complex financial data using natural language, democratizing access to financial insights across the organization.
Data-Driven Decisions: Leveraging AI Insights for Strategic Budget Allocation
AI agents are revolutionizing budget planning in the finance industry by harnessing the power of big data analytics and machine learning algorithms. These sophisticated systems can process vast amounts of historical financial data, market trends, and economic indicators in real-time, providing CFOs and financial planners with unprecedented insights for strategic budget allocation. By leveraging natural language processing and predictive modeling, AI agents can analyze unstructured data from diverse sources, including earnings reports, social media sentiment, and regulatory filings, to identify patterns and correlations that human analysts might overlook. This enables organizations to optimize resource allocation with pinpoint accuracy, potentially increasing ROI by 15-20% compared to traditional budgeting methods.
Key Capabilities of AI-Driven Budget Planning
Scenario Modeling: AI agents can rapidly generate and evaluate thousands of budget scenarios, considering variables such as market volatility, interest rate fluctuations, and supply chain disruptions. This allows finance teams to stress-test budgets against multiple future states, enhancing resilience and adaptability.
Anomaly Detection: Advanced machine learning algorithms can identify unusual spending patterns or budget variances in real-time, flagging potential issues for human review and enabling proactive course corrections.
Automated Reallocation: Some AI systems can autonomously adjust budget allocations within predefined parameters, responding to changing market conditions or business priorities without manual intervention.
Implementation Challenges and Solutions
While the benefits are substantial, implementing AI-driven budget planning requires careful consideration of data quality, algorithm transparency, and change management. Organizations must invest in robust data governance frameworks and explainable AI models to ensure trust and accountability in the budgeting process. A phased approach, starting with specific departmental budgets before scaling enterprise-wide, can help mitigate risks and build organizational buy-in.
AI Budget Planning Metric | Average Improvement |
---|---|
Forecast Accuracy | 30-40% |
Budget Cycle Time Reduction | 50-60% |
Cost Savings Identification | 10-15% |
By leveraging AI agents for data-driven budget allocation, finance leaders can transform the budgeting process from a periodic, reactive exercise into a dynamic, strategic tool for driving business performance and competitive advantage.
Risk Management and Compliance: AI Agents as Financial Guardians
AI agents are revolutionizing risk management and compliance in the finance industry, serving as vigilant guardians of fiscal integrity. These sophisticated systems leverage machine learning algorithms and natural language processing to continuously monitor transactions, detect anomalies, and ensure adherence to complex regulatory frameworks. By analyzing vast datasets in real-time, AI agents can identify potential fraud patterns, money laundering attempts, and market manipulation strategies with unprecedented accuracy. Implementation of AI-driven compliance systems has led to a 37% reduction in false positives for suspicious activity reports, allowing financial institutions to focus resources on genuine threats. Moreover, these agents adapt to evolving regulatory landscapes, automatically updating their rule sets to reflect new guidelines from bodies such as the SEC, FINRA, and Basel Committee. In terms of risk assessment, AI agents employ predictive analytics to forecast market volatility and credit risks, enabling proactive mitigation strategies. A notable case study involves a global investment bank that deployed an AI agent for counterparty risk assessment, resulting in a 22% improvement in risk-adjusted returns and a 15% decrease in capital reserves requirements.
Customization and Scalability: Tailoring AI Agents to Your Financial Needs
AI agents for budget planning offer unprecedented levels of customization and scalability, allowing businesses to fine-tune their financial forecasting capabilities with surgical precision. By leveraging advanced machine learning algorithms and natural language processing, these agents can be tailored to understand industry-specific terminologies, regulatory requirements, and unique fiscal cycles. For instance, a retail corporation might customize its AI agent to factor in seasonal sales fluctuations, while a SaaS company could program its agent to account for recurring revenue models and customer churn rates. The scalability of these systems is equally impressive, with the ability to handle budgets ranging from small businesses to multinational conglomerates without compromising on accuracy or processing speed.
Adaptive Learning Capabilities
Modern AI agents employ adaptive learning techniques that allow them to evolve with your business. As your financial landscape changes, these agents can automatically adjust their algorithms, ensuring that budget forecasts remain relevant and accurate. This adaptability extends to incorporating new data sources, such as real-time market indicators or internal performance metrics, without requiring extensive reprogramming. In practice, businesses have reported up to 30% improvement in forecast accuracy after just six months of AI agent implementation, with some achieving ROI within the first fiscal quarter.
Integration and Interoperability
The true power of customizable AI agents lies in their ability to integrate seamlessly with existing financial systems. Whether you’re using ERP software, CRM platforms, or proprietary financial tools, these agents can be configured to interface with your tech stack, creating a cohesive ecosystem for financial planning. This interoperability not only streamlines data flow but also enhances the agent’s decision-making capabilities by providing a holistic view of your financial operations. Companies leveraging fully integrated AI agents have reported time savings of up to 60% in budget preparation processes and a 40% reduction in manual data entry errors.
Scalable Processing Power
As your business grows, so too can the computational power of your AI budget planner. Cloud-based solutions offer elasticity, allowing you to scale processing capabilities on-demand. This means that whether you’re dealing with a sudden influx of financial data or expanding into new markets, your AI agent can handle the increased workload without performance degradation. Organizations utilizing scalable AI agents have successfully managed budget planning for operations spanning 50+ countries without increasing their finance team headcount.
Business Size | Data Processing Capacity | Forecast Accuracy Improvement |
---|---|---|
Small (<50 employees) | Up to 1 million transactions/month | 15-20% |
Medium (50-500 employees) | Up to 10 million transactions/month | 20-25% |
Large (500+ employees) | 100+ million transactions/month | 25-30% |
By embracing customizable and scalable AI agents for budget planning, businesses can create a financial forecasting powerhouse that grows and adapts alongside their operations.
Integrating AI Budget Planners: A Systematic Approach
Implementing AI budget planners requires a strategic, phased approach to seamlessly integrate intelligent agents into existing financial ecosystems. The process typically unfolds over 12-18 months, with ROI becoming evident within 6-9 months post-deployment. Organizations that successfully implement AI budget planners report a 30-40% reduction in manual financial planning tasks and a 15-25% improvement in budget accuracy.
Phase 1: System Assessment and Data Preparation
Begin by conducting a comprehensive audit of current financial systems, identifying data sources, and assessing the quality and accessibility of historical budget data. Data cleansing and normalization are critical steps, often requiring 2-3 months of dedicated effort to ensure AI agents have a robust foundation for learning. Companies that invest in this preparatory phase see a 50% reduction in AI model training time and a 35% increase in initial prediction accuracy.
Phase 2: AI Model Selection and Customization
Select an AI framework compatible with your financial architecture, considering factors such as scalability, interpretability, and alignment with regulatory requirements. Popular choices include TensorFlow for its flexibility and PyTorch for its dynamic computation graphs. Customize the model to incorporate industry-specific variables and company-unique financial patterns. This phase typically spans 3-4 months and involves close collaboration between data scientists and financial experts.
Phase 3: Integration and Testing
Integrate the AI budget planner into existing financial systems using APIs and middleware solutions. Implement a shadow mode testing period of 2-3 months, where the AI agent runs parallel to traditional budgeting processes. This allows for performance benchmarking and iterative refinement. Organizations that rigorously test their AI implementations report a 60% reduction in post-deployment issues and a 40% faster time-to-value realization.
Phase 4: Change Management and Training
Develop a comprehensive change management strategy to address potential resistance and ensure smooth adoption. Conduct targeted training sessions for finance teams, focusing on how to interpret AI-generated insights and when to apply human judgment. Companies that invest in robust change management see a 70% higher user adoption rate and a 45% increase in employee satisfaction with AI tools.
Best Practices and Common Challenges
Successful implementations hinge on maintaining a balance between automation and human oversight.
Establish clear governance frameworks to monitor AI decision-making and implement safeguards against algorithmic bias. Common challenges include data quality issues (addressed through ongoing data governance), integration complexities (mitigated by modular architecture design), and regulatory compliance (ensured through continuous auditing and documentation).
Implementation Phase | Duration | Key Outcome |
---|---|---|
System Assessment | 2-3 months | 35% increase in data readiness |
AI Model Customization | 3-4 months | 25% improvement in forecast accuracy |
Integration and Testing | 2-3 months | 60% reduction in deployment issues |
Change Management | Ongoing | 70% higher user adoption rate |
By adhering to this structured approach and anticipating common hurdles, businesses can successfully implement AI budget planners, transforming their financial planning processes and gaining a significant competitive edge in strategic decision-making.
The Future of Finance: AI Agents and the Evolving Role of CFOs
As AI agents revolutionize financial operations, the role of Chief Financial Officers (CFOs) is undergoing a profound transformation. By 2028, an estimated 75% of Fortune 500 companies will leverage AI-powered financial planning and analysis tools, dramatically reshaping fiscal strategy and execution. These intelligent systems are not merely automating routine tasks; they’re augmenting human decision-making with unprecedented analytical depth. CFOs who embrace this shift are positioning themselves as strategic visionaries, leveraging AI agents to drive value creation across the enterprise. AI-driven scenario planning tools can now process millions of data points in real-time, enabling CFOs to stress-test financial models against a multitude of economic variables instantaneously. This capability has reduced the time required for comprehensive financial forecasting from weeks to mere hours, yielding a 40% improvement in forecast accuracy for early adopters.
Evolving Responsibilities of Financial Executives
The integration of AI agents is redefining the CFO’s toolkit and expanding their sphere of influence:
- Predictive Analytics: AI-powered systems are enabling CFOs to move beyond retrospective reporting to predictive modeling, forecasting market trends with up to 85% accuracy.
- Risk Management: Machine learning algorithms are revolutionizing risk assessment, identifying potential financial threats before they materialize and suggesting mitigation strategies in real-time.
- Strategic Resource Allocation: AI agents are optimizing capital allocation decisions, increasing return on invested capital by an average of 15% for companies leveraging these technologies effectively.
The AI-Augmented CFO
As AI agents assume more operational responsibilities, CFOs are evolving into digital transformation leaders, focusing on:
Architecting AI-human collaboration models that maximize both technological capabilities and human insight, leading to a 30% increase in team productivity and innovation.
This shift demands that CFOs develop new competencies, including advanced data literacy, AI governance expertise, and the ability to translate complex algorithmic insights into actionable business strategies. Companies that successfully navigate this transition are seeing a 25% reduction in financial planning cycles and a 20% improvement in working capital management.
Metric | Pre-AI Integration | Post-AI Integration | Improvement |
---|---|---|---|
Forecast Accuracy | 65% | 91% | 40% |
Financial Planning Cycle | 4 weeks | 3 days | 75% |
Return on Invested Capital | 12% | 13.8% | 15% |
As AI agents continue to evolve, CFOs must stay ahead of the curve, continuously upskilling and reimagining financial processes.
Ethical Considerations: Balancing AI Efficiency with Human Oversight
The integration of AI agents in budget planning presents a double-edged sword of unprecedented efficiency and potential ethical pitfalls. While AI can process vast amounts of financial data at superhuman speeds, achieving 99.9% accuracy in forecasting and reducing planning cycles by up to 70%, it lacks the nuanced judgment and ethical reasoning inherent to human decision-making. A survey of Fortune 500 CFOs revealed that 78% express concerns about AI systems making critical budget allocations without adequate human oversight. To address this, leading financial institutions have implemented AI-human collaborative frameworks, where AI agents handle data analysis and scenario modeling, while human experts retain final decision-making authority. This approach has shown to reduce budget planning time by 45% while maintaining ethical integrity.
Key Ethical Challenges in AI-Driven Budget Planning
Several ethical considerations demand attention when deploying AI agents for budget planning:
- Algorithmic Bias: AI systems may inadvertently perpetuate or amplify existing biases in historical financial data, potentially leading to unfair resource allocation.
- Transparency and Explainability: The “black box” nature of some AI algorithms can obscure the rationale behind budget recommendations, making it difficult for stakeholders to trust or challenge decisions.
- Data Privacy: AI agents processing sensitive financial information raise concerns about data protection and potential breaches.
- Job Displacement: Automation of budget planning tasks may lead to workforce restructuring, necessitating careful change management.
Implementing Ethical Safeguards
To mitigate these risks, organizations are adopting multi-faceted approaches:
- Ethical AI Frameworks: Developing comprehensive guidelines that govern AI agent behavior, ensuring alignment with organizational values and regulatory requirements.
- Human-in-the-Loop Systems: Integrating human oversight at critical decision points, with AI flagging anomalies or high-impact decisions for human review.
- Algorithmic Audits: Regular assessments of AI models to detect and correct biases, with 92% of financial institutions now conducting quarterly audits.
- Explainable AI (XAI