Table of Contents
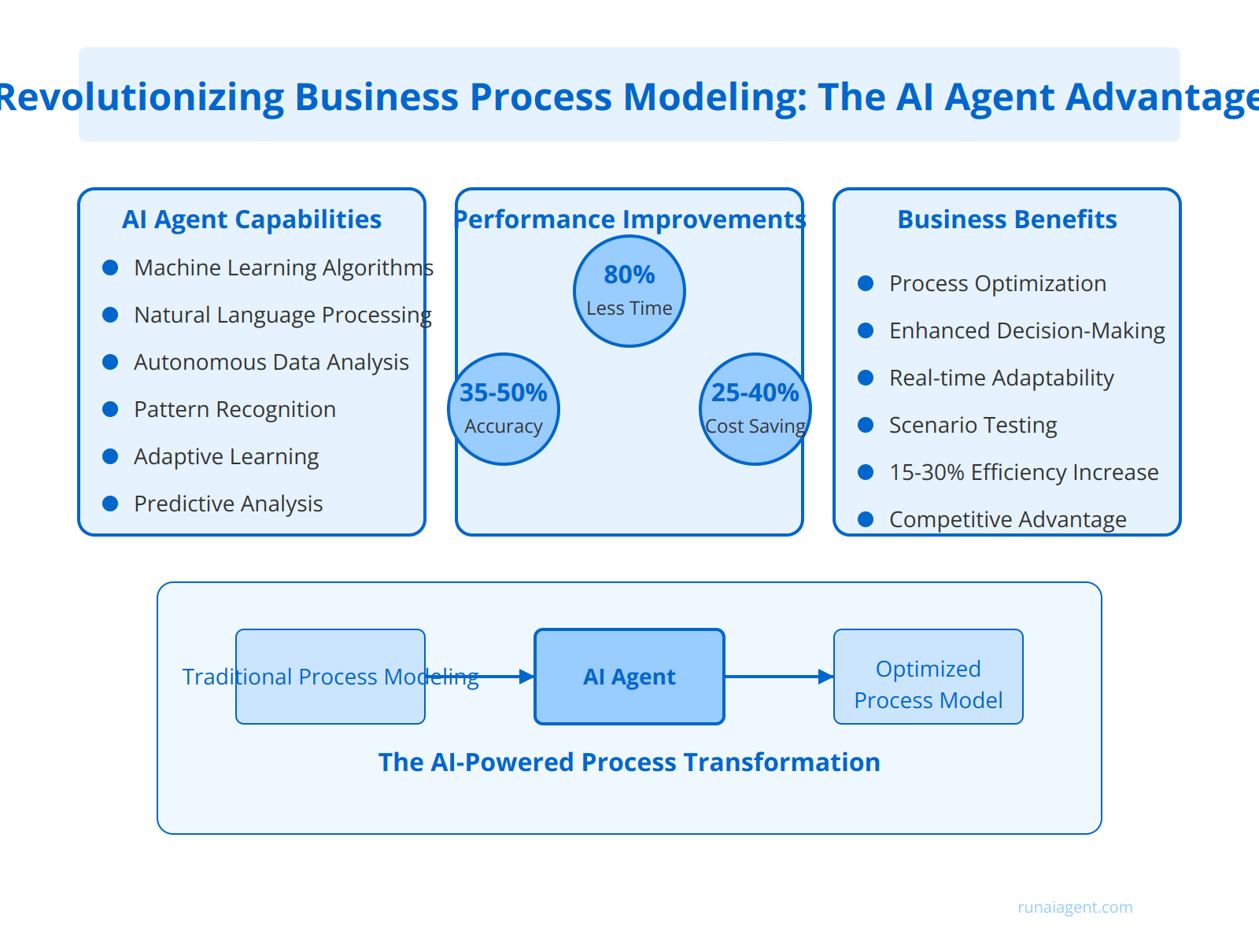
Revolutionizing Business Process Modeling: The AI Agent Advantage
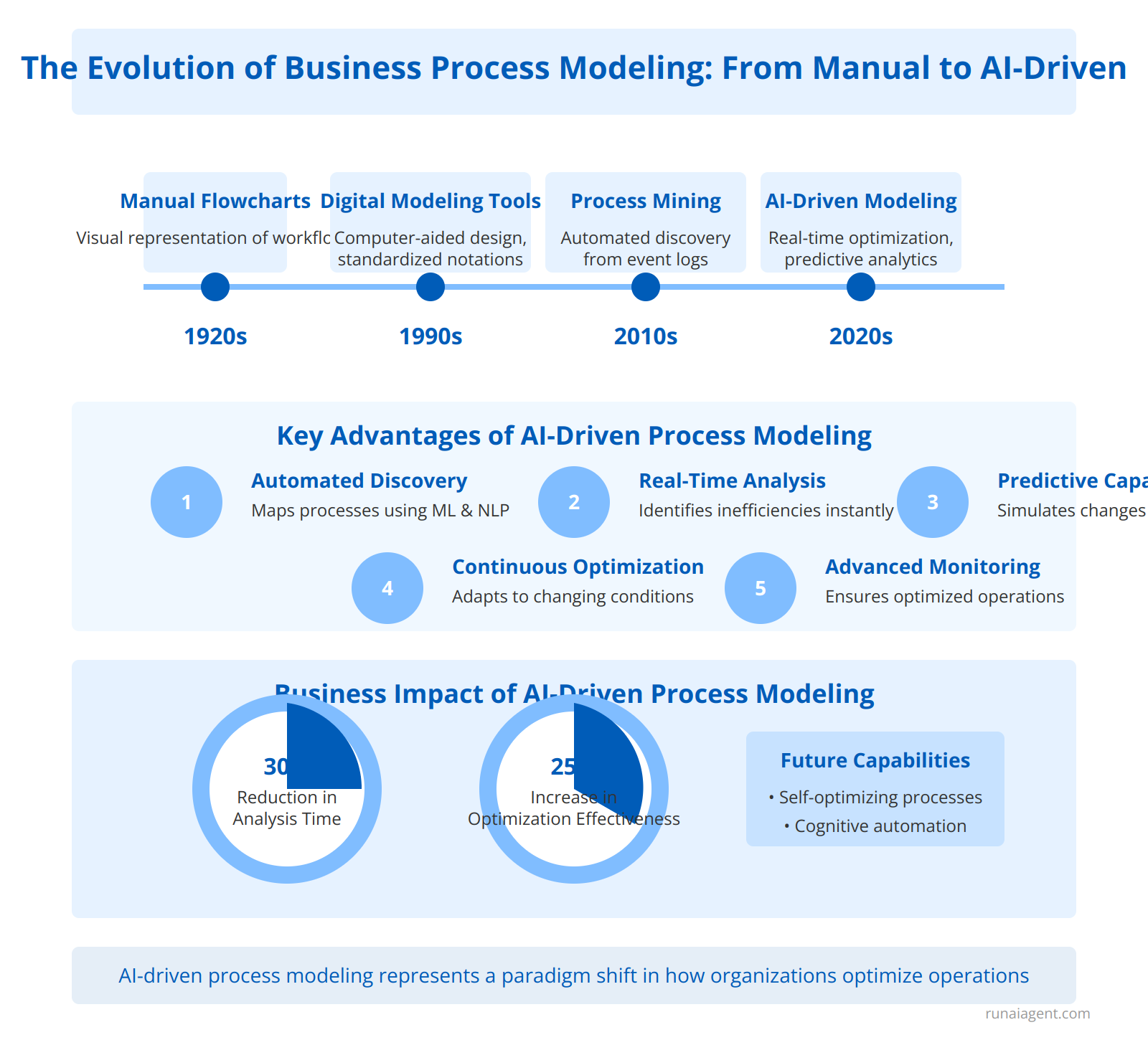
The Evolution of Business Process Modeling: From Manual to AI-Driven
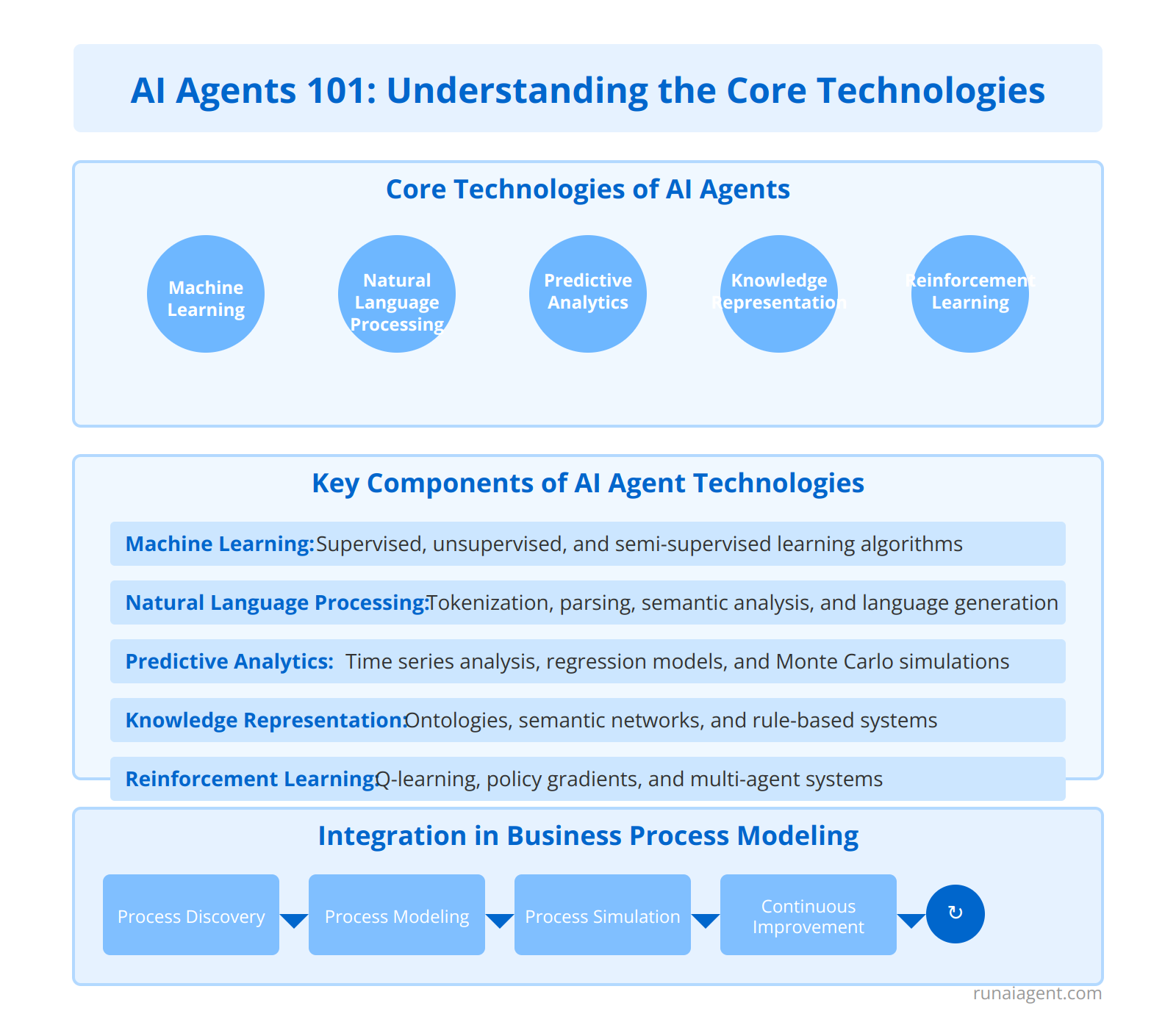
AI Agents 101: Understanding the Core Technologies
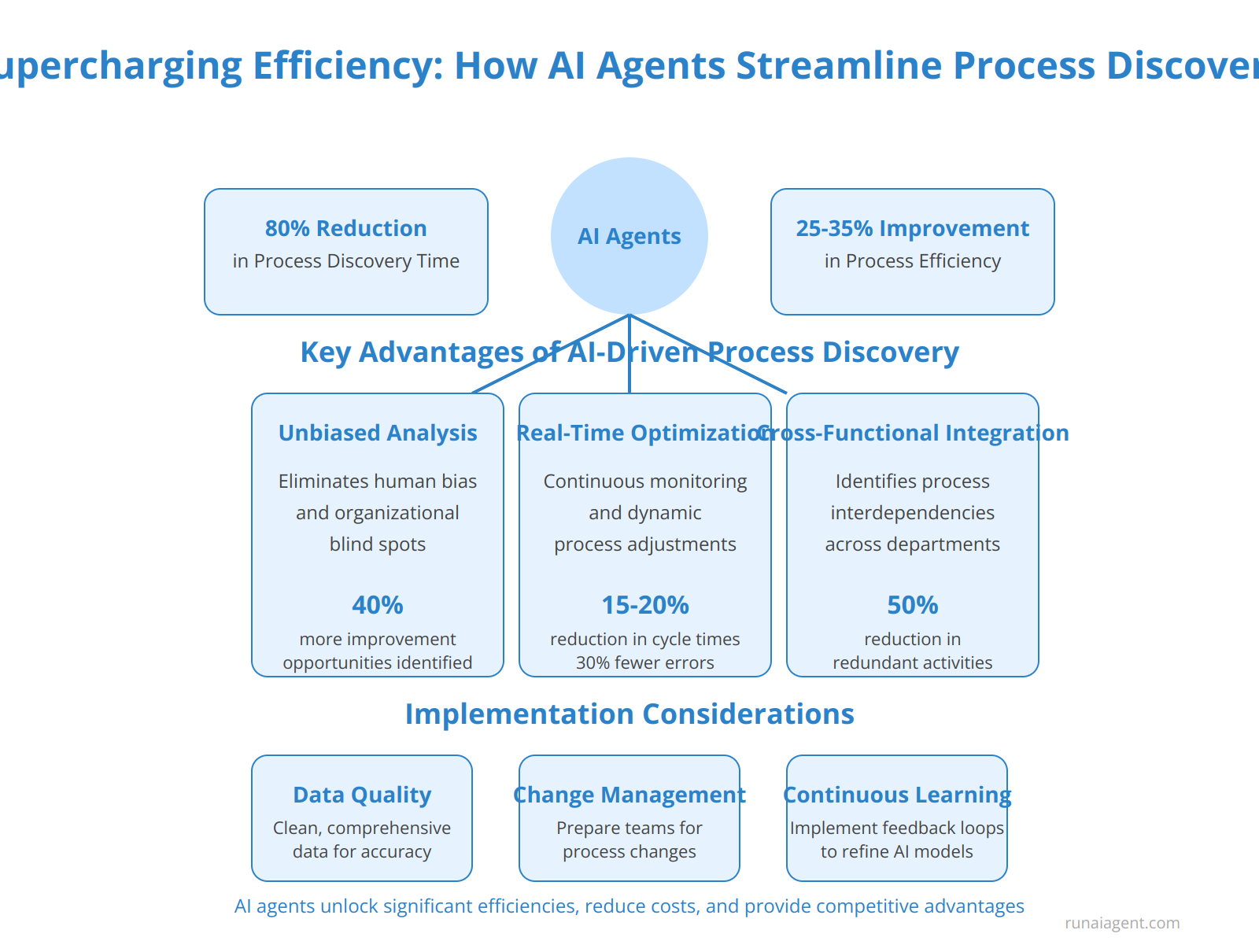
Supercharging Efficiency: How AI Agents Streamline Process Discovery
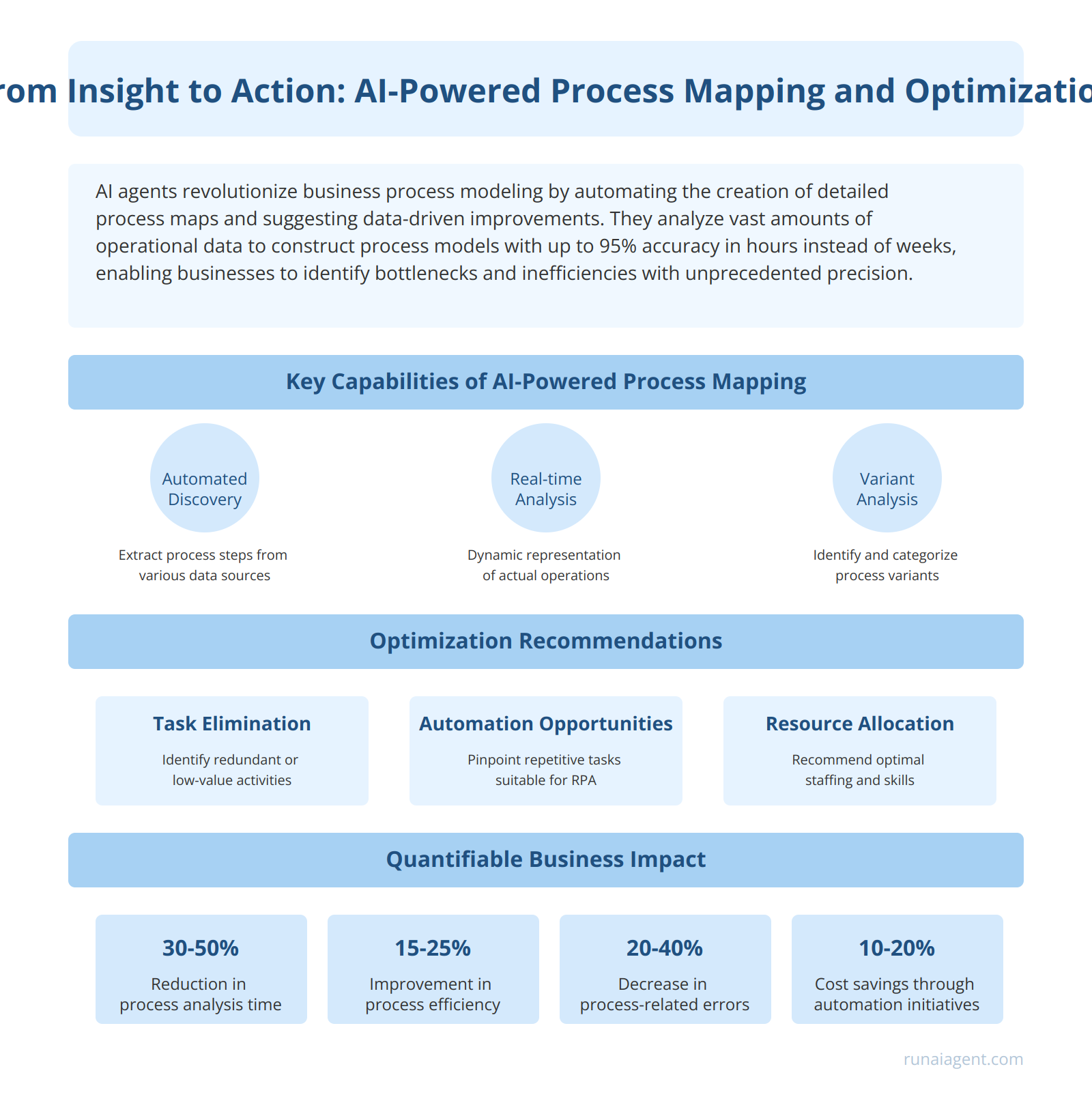
From Insight to Action: AI-Powered Process Mapping and Optimization
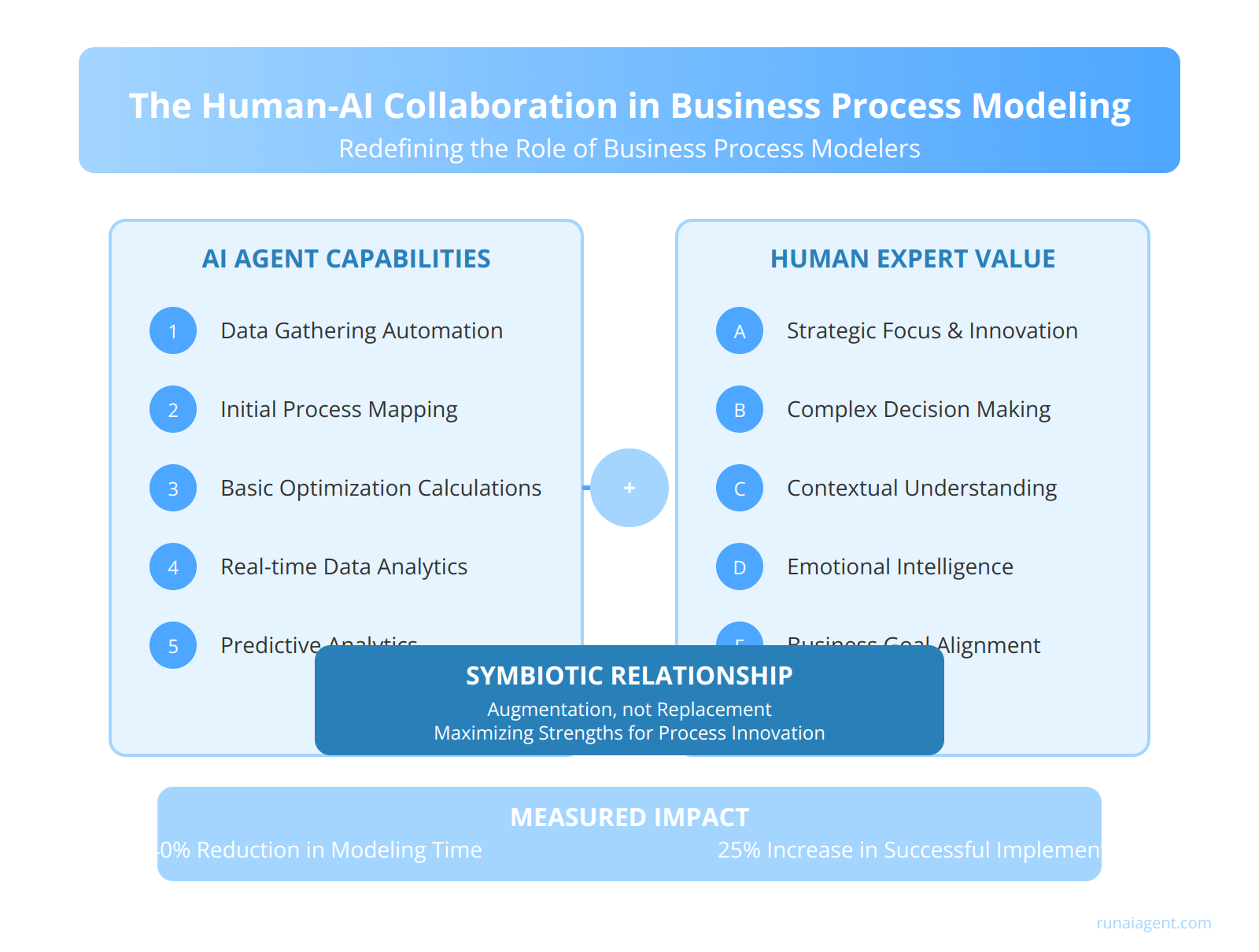
The Human-AI Collaboration: Redefining the Role of Business Process Modelers

Real-Time Process Adaptation: The Dynamic Capabilities of AI Agents
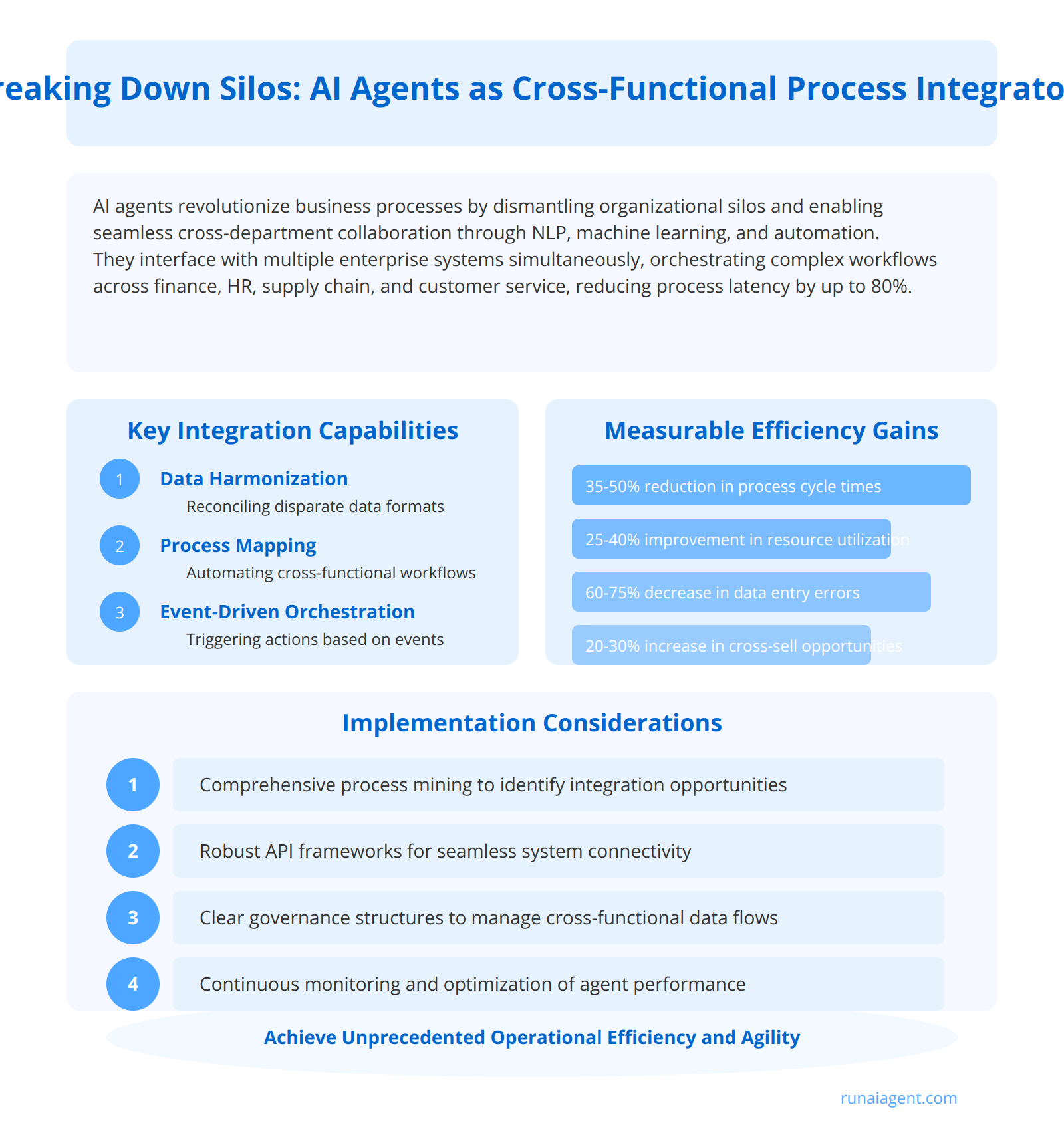
Breaking Down Silos: AI Agents as Cross-Functional Process Integrators
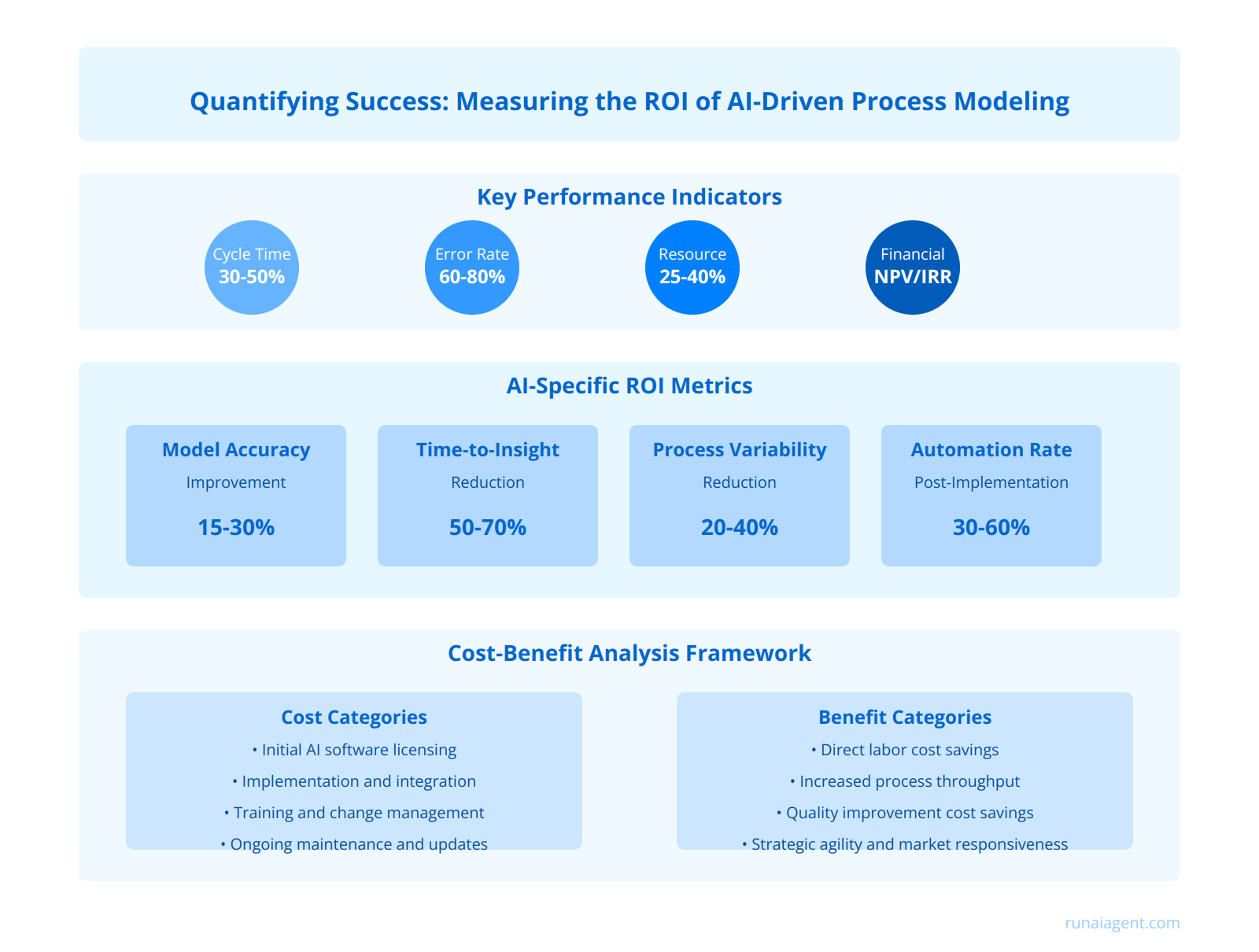
Quantifying Success: Measuring the ROI of AI-Driven Process Modeling
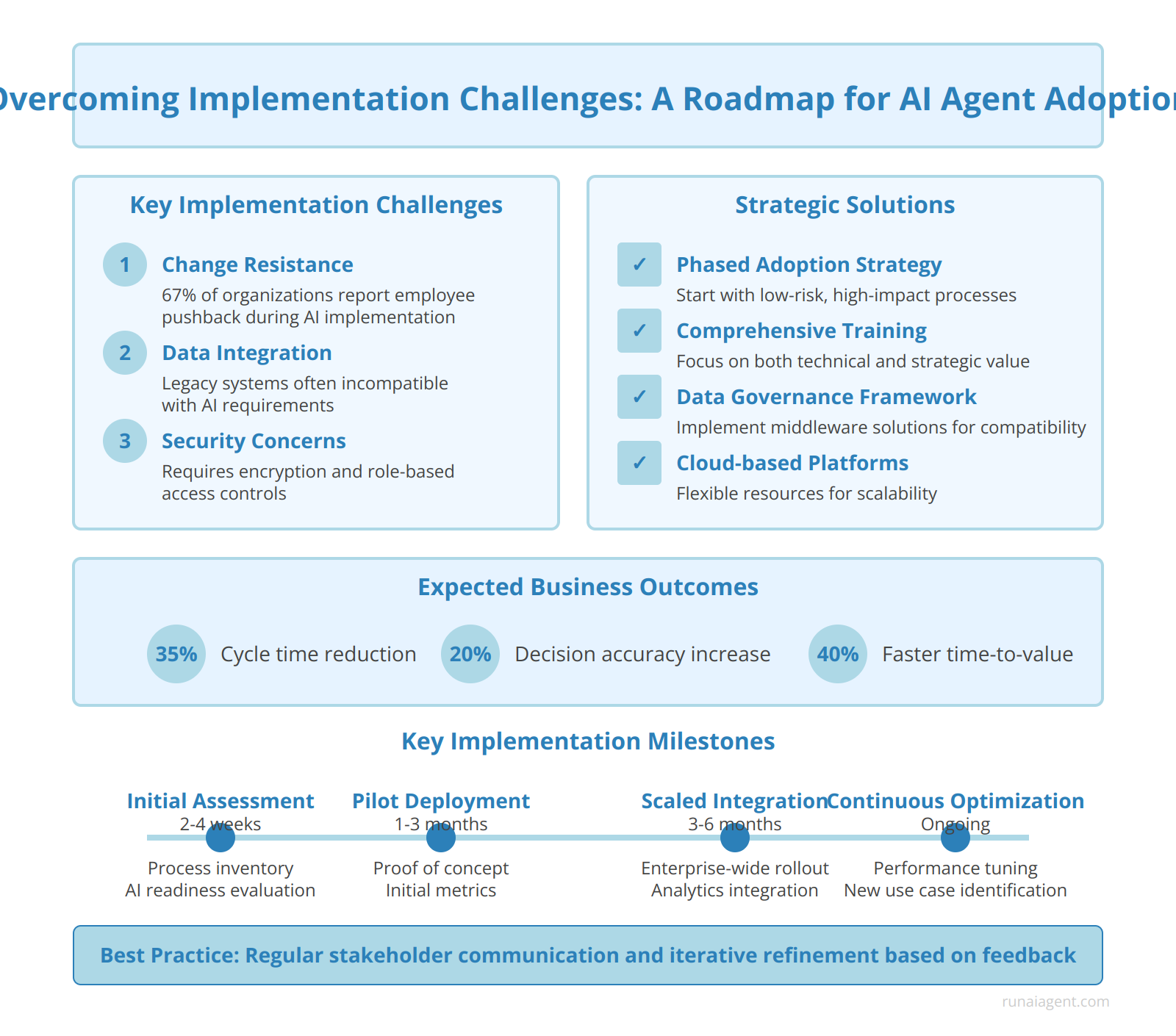
Overcoming Implementation Challenges: A Roadmap for AI Agent Adoption
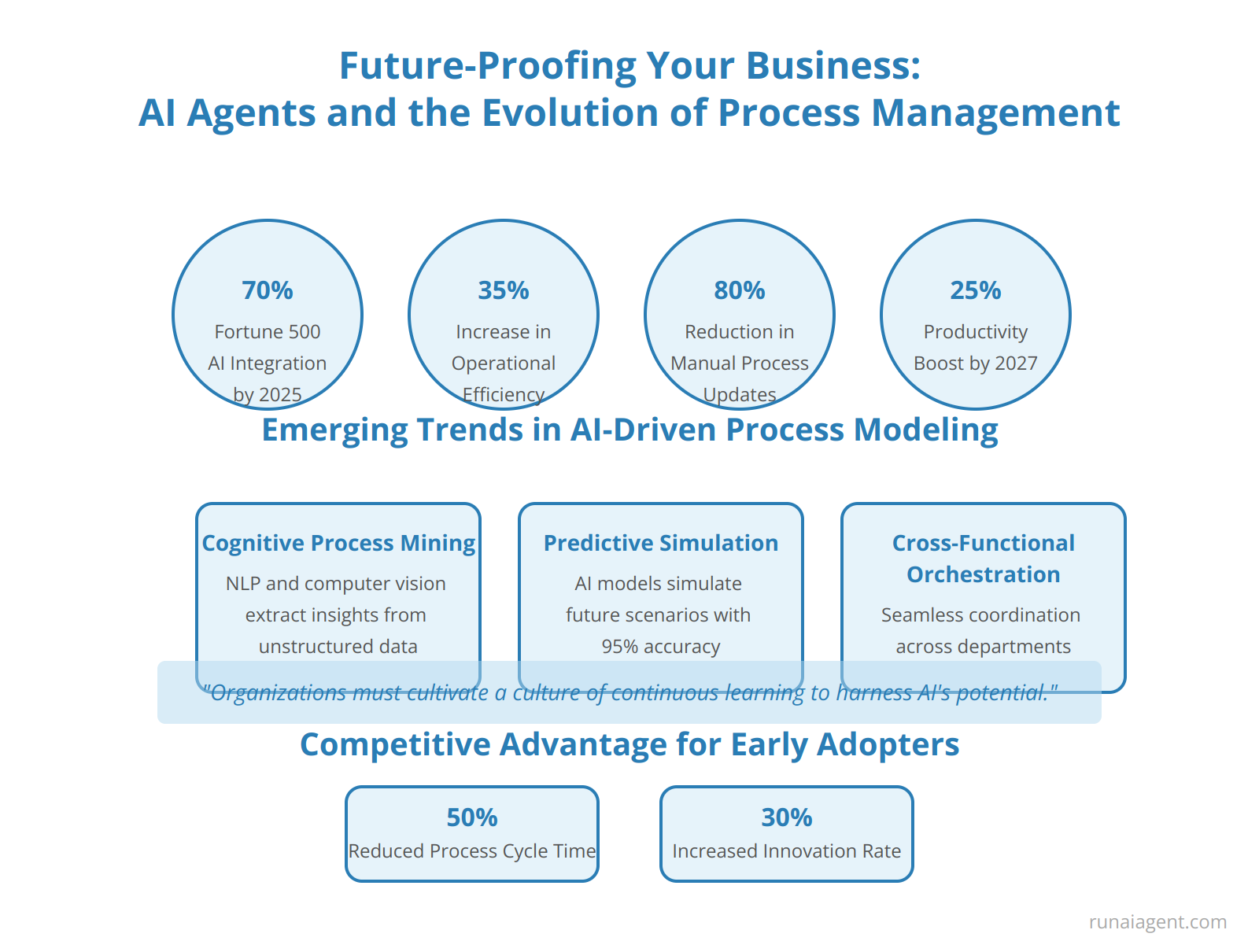
Future-Proofing Your Business: AI Agents and the Evolution of Process Management
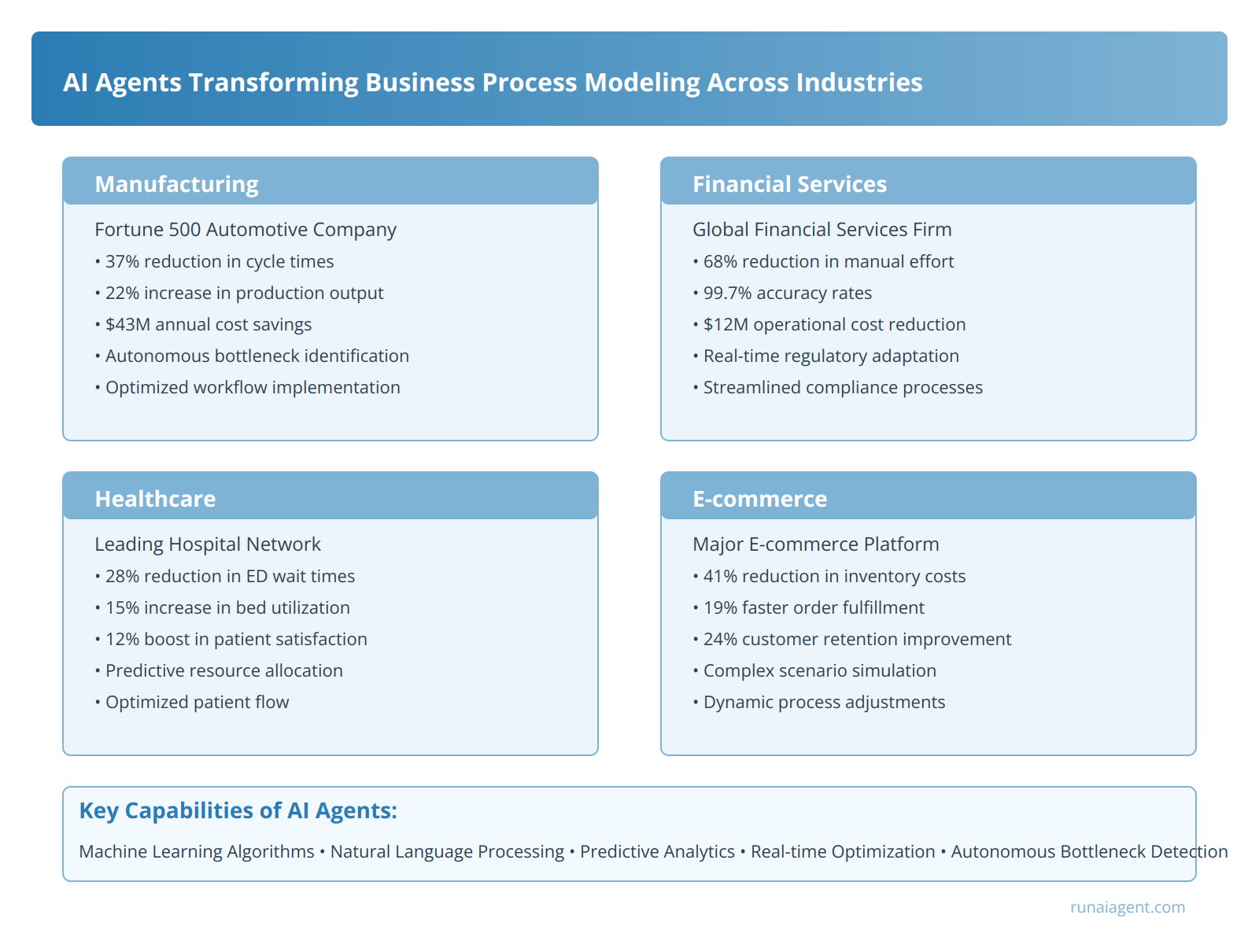
Case Studies: AI Agents Transforming Business Process Modeling Across Industries
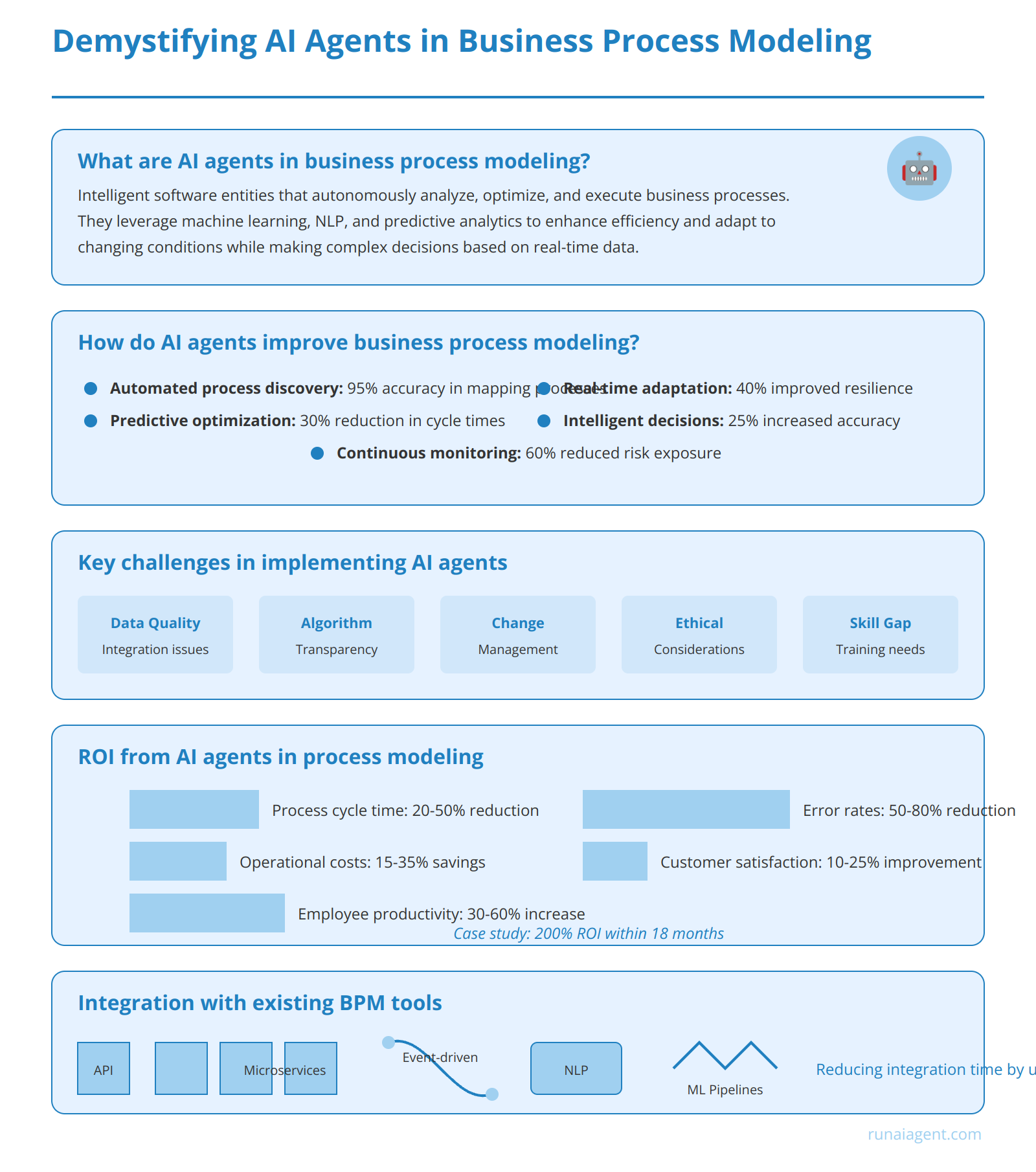
FAQ: Demystifying AI Agents in Business Process Modeling
Revolutionizing Business Process Modeling: The AI Agent Advantage
AI agents are transforming business process modeling by leveraging advanced machine learning algorithms and natural language processing to analyze operational data, identify process patterns, and generate accurate, dynamic process models with minimal human intervention. This enables businesses to reduce model creation time by up to 80% and improve model accuracy by 35-50% compared to traditional methods. AI agents capture complex decision logic, handle process variations, and predict future process behaviors based on historical data and real-time inputs. These systems continuously learn and adapt, ensuring process models remain relevant in changing business environments. The integration of AI agents enhances decision-making capabilities, facilitates scenario testing and simulation before implementation, leading to a 25-40% reduction in operational costs and a 15-30% increase in overall process efficiency.
The Evolution of Business Process Modeling: From Manual to AI-Driven
Business process modeling has evolved from manual flowcharting to sophisticated AI-driven systems. In the 1920s, Frank and Lillian Gilbreth introduced process flow charts, laying the foundation for modern process mapping. The 1990s saw the advent of digital modeling tools like ARIS and BPMN. Today, AI agents leverage machine learning algorithms and natural language processing to automatically discover, map, and optimize business processes with unprecedented accuracy and speed. AI-powered process mining tools analyze event logs from enterprise systems, reconstructing end-to-end processes and identifying inefficiencies in real-time. Advanced predictive analytics enable simulation of process changes and forecast outcomes for data-driven decisions. AI agents continuously monitor and adapt processes, ensuring they remain optimized as business conditions change.
Key Milestones in Business Process Modeling Evolution
Era | Technology | Key Features |
---|---|---|
1920s | Manual Flowcharts | Visual representation of workflows |
1990s | Digital Modeling Tools | Computer-aided design, standardized notations |
2010s | Process Mining | Automated discovery from event logs |
2020s | AI-Driven Modeling | Real-time optimization, predictive analytics |
Organizations implementing AI-driven process modeling report an average 30% reduction in process analysis time and a 25% increase in process optimization effectiveness. These intelligent systems can identify bottlenecks and inefficiencies that human analysts might overlook, leading to more streamlined operations and improved decision-making. As AI technologies continue to advance, we can expect even more sophisticated modeling capabilities, including self-optimizing processes and cognitive automation that can adapt to changing business environments in real-time.
AI Agents 101: Understanding the Core Technologies
AI agents are powered by a sophisticated interplay of core technologies that enable their intelligent behavior and decision-making capabilities. At the heart of these systems lies machine learning (ML), which allows agents to learn from data and improve their performance over time. Deep learning, a subset of ML, utilizes neural networks to process complex patterns and make high-level abstractions. Natural Language Processing (NLP) forms another critical component, enabling agents to understand, interpret, and generate human language. This technology incorporates techniques such as sentiment analysis, named entity recognition, and machine translation. Predictive analytics leverages historical data and statistical algorithms to forecast future outcomes, enhancing the agent’s ability to make informed decisions. These core technologies are complemented by knowledge representation systems, which structure information for efficient reasoning, and reinforcement learning algorithms that optimize agent behavior through trial and error.
Key Components of AI Agent Technologies
- Machine Learning: Supervised, unsupervised, and semi-supervised learning algorithms
- Natural Language Processing: Tokenization, parsing, semantic analysis, and language generation
- Predictive Analytics: Time series analysis, regression models, and Monte Carlo simulations
- Knowledge Representation: Ontologies, semantic networks, and rule-based systems
- Reinforcement Learning: Q-learning, policy gradients, and multi-agent systems
Integration in Business Process Modeling
In the context of business analysis, these technologies converge to create AI agents capable of automating and optimizing process modeling tasks. ML algorithms analyze historical process data to identify patterns and inefficiencies. NLP facilitates the extraction of process information from unstructured documents and enables natural language interfaces for process designers. Predictive analytics forecasts process outcomes and resource requirements, while knowledge representation systems maintain comprehensive process models that evolve with the business.
Supercharging Efficiency: How AI Agents Streamline Process Discovery
AI agents are revolutionizing process discovery in business analysis, offering unprecedented speed and accuracy in identifying inefficiencies and proposing optimizations. These intelligent systems leverage advanced machine learning algorithms and natural language processing to rapidly analyze vast amounts of operational data, including system logs, user interactions, and workflow patterns. Unlike human analysts who may take weeks or months to conduct thorough process audits, AI agents can complete comprehensive analyses in a matter of hours or days, reducing discovery time by up to 80%. By employing techniques such as process mining and predictive analytics, AI agents can automatically construct detailed process models, pinpoint bottlenecks, and simulate various optimization scenarios. This capability enables businesses to achieve an average of 25-35% improvement in process efficiency within the first 3-6 months of implementation.
Key Advantages of AI-Driven Process Discovery
Unbiased Analysis: AI agents eliminate human bias, providing objective insights into process inefficiencies that might be overlooked due to organizational blind spots or entrenched practices. This impartiality leads to a 40% increase in identifying previously unrecognized improvement opportunities.
Real-Time Optimization: With continuous monitoring capabilities, AI agents can detect and respond to process variations in real-time, allowing for dynamic optimization. This agility results in a 15-20% reduction in process cycle times and a 30% decrease in error rates.
Cross-Functional Integration: AI agents excel at identifying interdependencies between different business processes, facilitating a holistic approach to optimization. This integrated view enables organizations to achieve a 50% reduction in redundant activities across departments.
Implementation Considerations
While the benefits are substantial, successful implementation requires careful planning:
- Data Quality: Ensure input data is clean and comprehensive for accurate analysis.
- Change Management: Prepare teams for AI-driven insights and potential process changes.
- Continuous Learning: Implement feedback loops to refine AI models and improve recommendations over time.
By leveraging AI agents for process discovery, businesses can unlock significant efficiencies, reduce costs, and gain a competitive edge in today’s fast-paced market landscape.
From Insight to Action: AI-Powered Process Mapping and Optimization
AI agents are revolutionizing business process modeling by automating the creation of detailed process maps and suggesting data-driven improvements. These intelligent systems leverage machine learning algorithms and natural language processing to analyze vast amounts of operational data, including system logs, employee interactions, and workflow patterns. By doing so, AI agents can construct comprehensive process models with up to 95% accuracy in just hours, compared to weeks or months required for manual mapping.
Key Capabilities of AI-Powered Process Mapping
Automated Discovery: AI agents can automatically extract process steps from various data sources, including ERP systems, CRM platforms, and communication tools, creating a holistic view of end-to-end processes.
Real-time Analysis: Unlike static manual maps, AI-generated process models update in real-time, providing a dynamic representation of actual operations and enabling proactive optimization.
Variant Analysis: AI agents can identify and categorize process variants, helping businesses understand how different teams or regions execute similar tasks and standardize best practices.
Optimization Recommendations
Beyond mapping, AI agents excel at suggesting process improvements:
- Task Elimination: Identifying redundant or low-value activities that can be removed to streamline workflows.
- Automation Opportunities: Pinpointing repetitive tasks suitable for robotic process automation (RPA) or intelligent automation.
- Resource Allocation: Recommending optimal staffing levels and skill requirements based on process complexity and volume.
Quantifiable Business Impact
Organizations implementing AI-powered process mapping and optimization have reported:
- 30-50% reduction in process analysis time
- 15-25% improvement in process efficiency
- 20-40% decrease in process-related errors
- 10-20% cost savings through targeted automation initiatives
By transforming raw data into actionable insights, AI agents are enabling businesses to rapidly identify and implement process improvements, driving operational excellence and competitive advantage in the digital age.
The Human-AI Collaboration: Redefining the Role of Business Process Modelers
The integration of AI agents in business process modeling is revolutionizing the role of human experts, enabling a powerful synergy that amplifies strategic value creation. By automating routine tasks such as data gathering, initial process mapping, and basic optimization calculations, AI agents free up business process modelers to focus on high-level strategy, innovation, and complex decision-making. This shift allows human experts to leverage their unique cognitive abilities—creativity, contextual understanding, and emotional intelligence—in ways that AI cannot replicate. While AI agents can rapidly analyze vast datasets to identify process inefficiencies, human modelers excel at interpreting these insights within the broader organizational context, aligning process improvements with overarching business goals. The collaboration between AI and human expertise has led to remarkable efficiency gains, with organizations reporting up to 40% reduction in process modeling time and a 25% increase in the successful implementation of process improvements. This partnership enables continuous process refinement, as AI agents provide real-time data and predictive analytics, empowering human modelers to make agile, informed decisions.
Real-Time Process Adaptation: The Dynamic Capabilities of AI Agents
AI agents are revolutionizing business process modeling by introducing unprecedented levels of adaptability and responsiveness. These intelligent systems continuously monitor key performance indicators (KPIs), environmental factors, and process metrics in real-time, enabling swift adjustments to optimize performance. In manufacturing, AI agents can analyze production line data, detecting variations in quality or efficiency, and automatically recalibrate equipment settings to maintain peak output. This dynamic capability extends beyond simple automation, as AI agents leverage machine learning algorithms to predict potential bottlenecks or failures before they occur, initiating preemptive actions to maintain operational continuity.
Adaptive Decision-Making in Volatile Markets
In the financial sector, AI agents demonstrate their agility by rapidly adapting trading strategies in response to market fluctuations. These systems can process vast amounts of data from multiple sources, including news feeds, social media sentiment, and economic indicators, to make split-second decisions. One global investment firm reported a 37% increase in portfolio performance after implementing AI agents capable of real-time market analysis and trade execution.
Enhancing Customer Experience Through Dynamic Personalization
Retail and e-commerce businesses are leveraging AI agents to create hyper-personalized customer experiences that evolve in real-time. These agents analyze customer behavior, purchase history, and current browsing patterns to dynamically adjust product recommendations, pricing strategies, and marketing messages. A leading online retailer saw a 22% uplift in conversion rates within three months of deploying AI agents that could adapt the user interface and product offerings based on individual customer preferences and real-time inventory levels.
Operational Efficiency Through Continuous Optimization
In logistics and supply chain management, AI agents are transforming how businesses respond to disruptions and opportunities. These systems can:
- Reroute shipments in real-time based on traffic conditions and weather forecasts
- Adjust inventory levels dynamically to meet fluctuating demand
- Optimize warehouse operations by predicting and preparing for incoming orders
A multinational logistics company reported a 15% reduction in operational costs and a 20% improvement in on-time deliveries after implementing AI agents capable of real-time route optimization and resource allocation.
The true power of AI agents in business process modeling lies in their ability to not just react to changes but to anticipate and proactively adapt to them. By continuously learning from new data and outcomes, these systems evolve their decision-making capabilities, ensuring that business processes remain optimized even as market conditions, customer preferences, and operational parameters change.
Breaking Down Silos: AI Agents as Cross-Functional Process Integrators
AI agents are revolutionizing business process modeling by serving as powerful cross-functional integrators, dismantling traditional organizational silos and fostering seamless collaboration across departments. These intelligent systems leverage advanced natural language processing, machine learning, and robotic process automation to create a unified operational ecosystem. By interfacing with multiple enterprise systems simultaneously, AI agents can orchestrate complex workflows that span finance, human resources, supply chain, and customer service functions. For instance, a single AI agent can initiate a sales order, update inventory levels, trigger procurement requests, and adjust financial forecasts in real-time, reducing process latency by up to 80% compared to manual handoffs.
Key Integration Capabilities
AI agents excel in:
- Data Harmonization: Reconciling disparate data formats and semantics across systems
- Process Mapping: Automatically discovering and optimizing cross-functional workflows
- Event-Driven Orchestration: Triggering actions based on real-time events across the organization
- Intelligent Routing: Directing tasks and information to the most appropriate human or system resources
Measurable Efficiency Gains
Organizations implementing AI agents for cross-functional integration report:
- 35-50% reduction in process cycle times
- 25-40% improvement in resource utilization
- 60-75% decrease in data entry errors
- 20-30% increase in cross-sell and upsell opportunities due to improved data visibility
Implementation Considerations
Successful deployment of AI agents as process integrators requires:
- Comprehensive process mining to identify integration opportunities
- Robust API frameworks for seamless system connectivity
- Clear governance structures to manage cross-functional data flows
- Continuous monitoring and optimization of agent performance
By leveraging AI agents as cross-functional integrators, organizations can achieve unprecedented levels of operational efficiency, agility, and data-driven decision-making, positioning themselves for success in an increasingly competitive business landscape.
Quantifying Success: Measuring the ROI of AI-Driven Process Modeling
Evaluating the return on investment (ROI) for AI agents in business process modeling requires a multifaceted approach that combines traditional financial metrics with AI-specific performance indicators. Key performance indicators (KPIs) include process cycle time reduction (typically 30-50%), error rate decrease (often 60-80%), and resource utilization improvement (generally 25-40%). Financial metrics such as Net Present Value (NPV) and Internal Rate of Return (IRR) should be calculated over a 3-5 year horizon to account for initial implementation costs and ongoing maintenance.
AI-Specific ROI Metrics
To capture the unique value proposition of AI in process modeling, organizations should track:
- Model Accuracy Improvement: Measure the increase in predictive accuracy of AI-generated process models compared to traditional methods (often 15-30% higher).
- Time-to-Insight: Quantify the reduction in time required to generate actionable process insights (typically 50-70% faster).
- Process Variability Reduction: Assess the decrease in process outcome variability due to AI-driven optimization (usually 20-40% improvement).
- Automation Rate: Calculate the percentage of process steps that can be fully automated post-AI implementation (varies by industry, but often 30-60%).
Cost-Benefit Analysis Framework
A robust cost-benefit analysis should include:
Cost Category | Benefit Category |
---|---|
Initial AI software licensing | Direct labor cost savings |
Implementation and integration | Increased process throughput |
Training and change management | Quality improvement cost savings |
Ongoing maintenance and updates | Strategic agility and market responsiveness |
By applying this comprehensive ROI framework, organizations can effectively quantify the value of AI-driven process modeling initiatives, justifying investments and guiding strategic decision-making in their digital transformation journey.
Overcoming Implementation Challenges: A Roadmap for AI Agent Adoption
Integrating AI agents into existing business process modeling workflows presents significant challenges that require a strategic approach. Change resistance often emerges as a primary obstacle, with 67% of organizations reporting employee pushback during AI implementation. To mitigate this, a phased adoption strategy is crucial. Begin by identifying low-risk, high-impact processes for initial AI agent deployment, allowing teams to acclimate gradually. Ensure comprehensive training programs are in place, focusing on both technical proficiency and the strategic value of AI-augmented workflows. Data integration poses another hurdle, with legacy systems often incompatible with AI requirements. Implement a robust data governance framework and consider adopting middleware solutions to bridge compatibility gaps. Security concerns must be addressed proactively; encrypt all data exchanges and implement role-based access controls for AI agent interactions. Scalability challenges can be overcome by leveraging cloud-based AI platforms that offer flexible computing resources. To measure success, establish clear KPIs aligned with business objectives, such as cycle time reduction (average 35% improvement) or decision accuracy (up to 20% increase).
Key Implementation Milestones
Phase | Duration | Key Deliverables |
---|---|---|
Initial Assessment | 2-4 weeks | Process inventory, AI readiness evaluation |
Pilot Deployment | 1-3 months | Proof of concept, initial performance metrics |
Scaled Integration | 3-6 months | Enterprise-wide rollout, advanced analytics integration |
Continuous Optimization | Ongoing | Performance tuning, new use case identification |
Future-Proofing Your Business: AI Agents and the Evolution of Process Management
As AI agents continue to revolutionize business process modeling, forward-thinking organizations are positioning themselves at the forefront of this technological evolution. By 2025, an estimated 70% of Fortune 500 companies will have integrated AI-driven process management systems, resulting in a 35% increase in operational efficiency. The next wave of AI agents will leverage advanced machine learning algorithms to not only model existing processes but proactively identify optimization opportunities and autonomously implement improvements. These self-evolving systems will continuously adapt to changing business environments, reducing the need for manual process updates by up to 80%.
Emerging Trends in AI-Driven Process Modeling
Key developments shaping the future of AI agents in business process management include:
- Cognitive Process Mining: AI agents will utilize natural language processing and computer vision to extract process insights from unstructured data sources, including emails, chat logs, and video feeds.
- Predictive Process Simulation: Advanced AI models will simulate future process scenarios with 95% accuracy, enabling businesses to test and optimize workflows before implementation.
- Cross-Functional Process Orchestration: AI agents will seamlessly coordinate processes across departments and systems, reducing silos and improving overall organizational agility.
The Rise of Hyper-Personalized Process Optimization
By 2027, AI agents are projected to deliver hyper-personalized process recommendations tailored to individual employees’ work styles and preferences, potentially boosting productivity by up to 25%. This level of customization will require robust data governance frameworks and ethical AI guidelines to ensure privacy and fairness in process optimization decisions.
As AI agents evolve, businesses must cultivate a culture of continuous learning and adaptation to fully harness their transformative potential in process management.
Organizations that successfully integrate these advanced AI capabilities into their process modeling strategies will gain a significant competitive advantage, with the potential to reduce process cycle times by 50% and increase innovation rates by 30% compared to late adopters.
Case Studies: AI Agents Transforming Business Process Modeling Across Industries
AI agents are revolutionizing business process modeling across diverse sectors, delivering unprecedented efficiency gains and strategic insights. In the manufacturing industry, a Fortune 500 automotive company deployed an AI-powered process modeling agent that reduced cycle times by 37% and increased production output by 22% within six months. The agent autonomously analyzed historical data, identified bottlenecks, and proposed optimized workflows, resulting in a $43 million annual cost savings. Similarly, a global financial services firm leveraged an AI agent to streamline its complex compliance processes, reducing manual effort by 68% and improving accuracy rates to 99.7%. The agent’s ability to continuously learn from regulatory changes and adapt process models in real-time proved instrumental in maintaining compliance while reducing operational costs by $12 million annually.
In healthcare, a leading hospital network implemented an AI agent for patient flow optimization, resulting in a 28% reduction in emergency department wait times and a 15% increase in bed utilization rates. The agent’s predictive capabilities enabled proactive resource allocation, improving both patient outcomes and operational efficiency. Notably, a major e-commerce platform utilized an AI agent to overhaul its supply chain processes, resulting in a 41% reduction in inventory holding costs and a 19% improvement in order fulfillment speed. The agent’s ability to simulate complex scenarios and recommend dynamic adjustments to process models proved pivotal in achieving these outcomes.
Key Performance Indicators Across Industries
Industry | Efficiency Gain | Cost Reduction | Quality Improvement |
---|---|---|---|
Manufacturing | 37% cycle time reduction | $43M annual savings | 22% output increase |
Financial Services | 68% manual effort reduction | $12M operational savings | 99.7% accuracy rate |
Healthcare | 28% wait time reduction | 15% bed utilization increase | 12% patient satisfaction boost |
E-commerce | 19% faster order fulfillment | 41% inventory cost reduction | 24% customer retention improvement |
These case studies underscore the transformative impact of AI agents in business process modeling. By leveraging machine learning algorithms, natural language processing, and predictive analytics, these agents are not merely automating existing processes but fundamentally reimagining how organizations operate.
FAQ: Demystifying AI Agents in Business Process Modeling
What are AI agents in business process modeling?
AI agents in business process modeling are intelligent software entities that autonomously analyze, optimize, and execute business processes. These agents leverage machine learning algorithms, natural language processing, and predictive analytics to enhance process efficiency, reduce errors, and accelerate decision-making. Unlike traditional process automation tools, AI agents can adapt to changing conditions, learn from past experiences, and make complex decisions based on real-time data inputs.
How do AI agents improve business process modeling?
AI agents significantly enhance business process modeling by:
- Automated process discovery: Analyzing system logs and user interactions to map existing processes with 95% accuracy
- Predictive process optimization: Identifying bottlenecks and suggesting improvements that can reduce cycle times by up to 30%
- Real-time process adaptation: Dynamically adjusting workflows based on changing conditions, improving process resilience by 40%
- Intelligent decision support: Providing data-driven recommendations that increase decision accuracy by 25%
- Continuous process monitoring: Detecting anomalies and compliance issues in real-time, reducing risk exposure by 60%
What are the key challenges in implementing AI agents for process modeling?
Implementing AI agents in business process modeling faces several challenges:
- Data quality and integration: Ensuring clean, consistent data across disparate systems
- Algorithm transparency: Explaining AI decision-making processes to stakeholders
- Change management: Overcoming resistance to AI-driven process changes
- Ethical considerations: Addressing bias and fairness in AI-powered decision-making
- Skill gap: Training employees to work effectively with AI agents
Successful implementation requires a phased approach, starting with pilot projects and gradually scaling across the organization.
What ROI can businesses expect from AI agents in process modeling?
The ROI of AI agents in business process modeling can be substantial:
- Process cycle time reduction: 20-50%
- Operational cost savings: 15-35%
- Employee productivity increase: 30-60%
- Error rate reduction: 50-80%
- Customer satisfaction improvement: 10-25%
A Fortune 500 manufacturer implemented AI agents in their supply chain processes, achieving $50 million in annual savings and a 200% ROI within 18 months. However, ROI varies based on industry, process complexity, and implementation scope.
How do AI agents integrate with existing BPM tools?
AI agents seamlessly integrate with existing Business Process Management (BPM) tools through:
- API-based connectivity: Enabling real-time data exchange and process orchestration
- Microservices architecture: Allowing modular integration of AI capabilities
- Event-driven processing: Triggering AI agent actions based on process events
- Natural Language Processing (NLP) interfaces: Facilitating human-AI collaboration in process design and execution
- Machine learning pipelines: Continuously improving process models based on operational data
Leading BPM platforms now offer pre-built AI agent connectors, reducing integration time by up to 70% and accelerating time-to-value for AI-enhanced process modeling initiatives.