Table of Contents

The AI Revolution in Ad Performance Analysis: Transforming Campaigns with Intelligent Agents
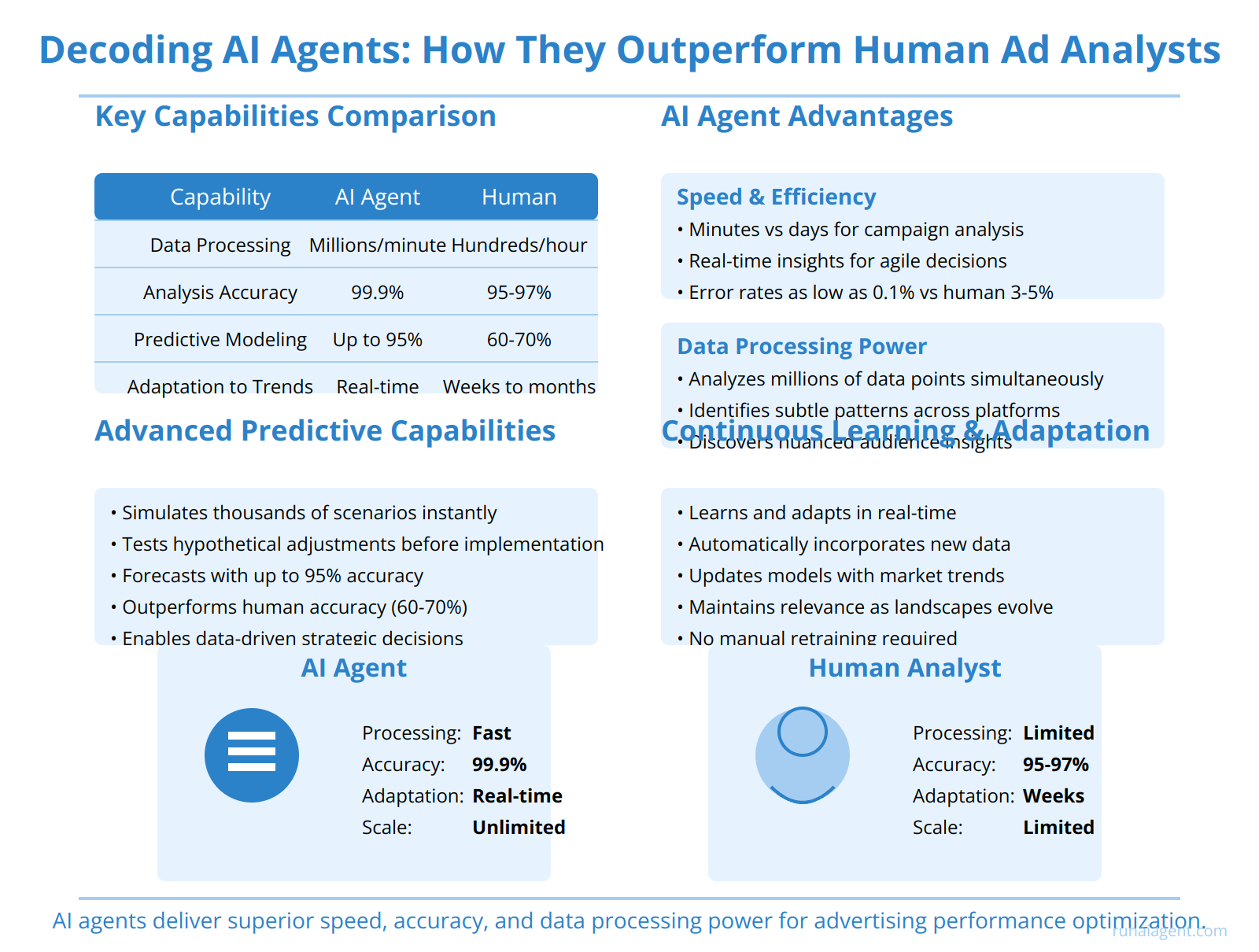
Decoding AI Agents: How They Outperform Human Ad Analysts
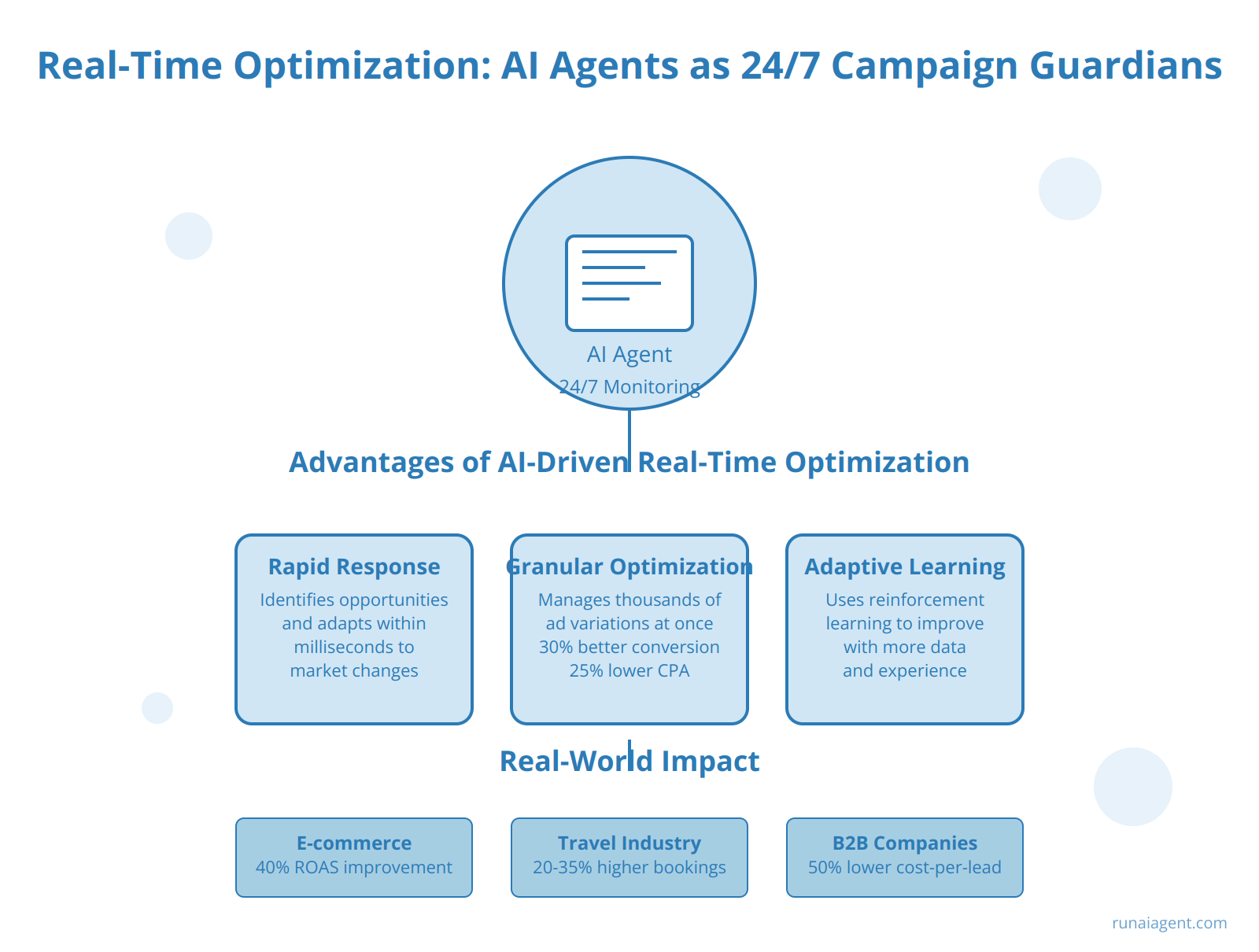
Real-Time Optimization: AI Agents as 24/7 Campaign Guardians

From Big Data to Smart Decisions: AI’s Role in Ad Performance Insights
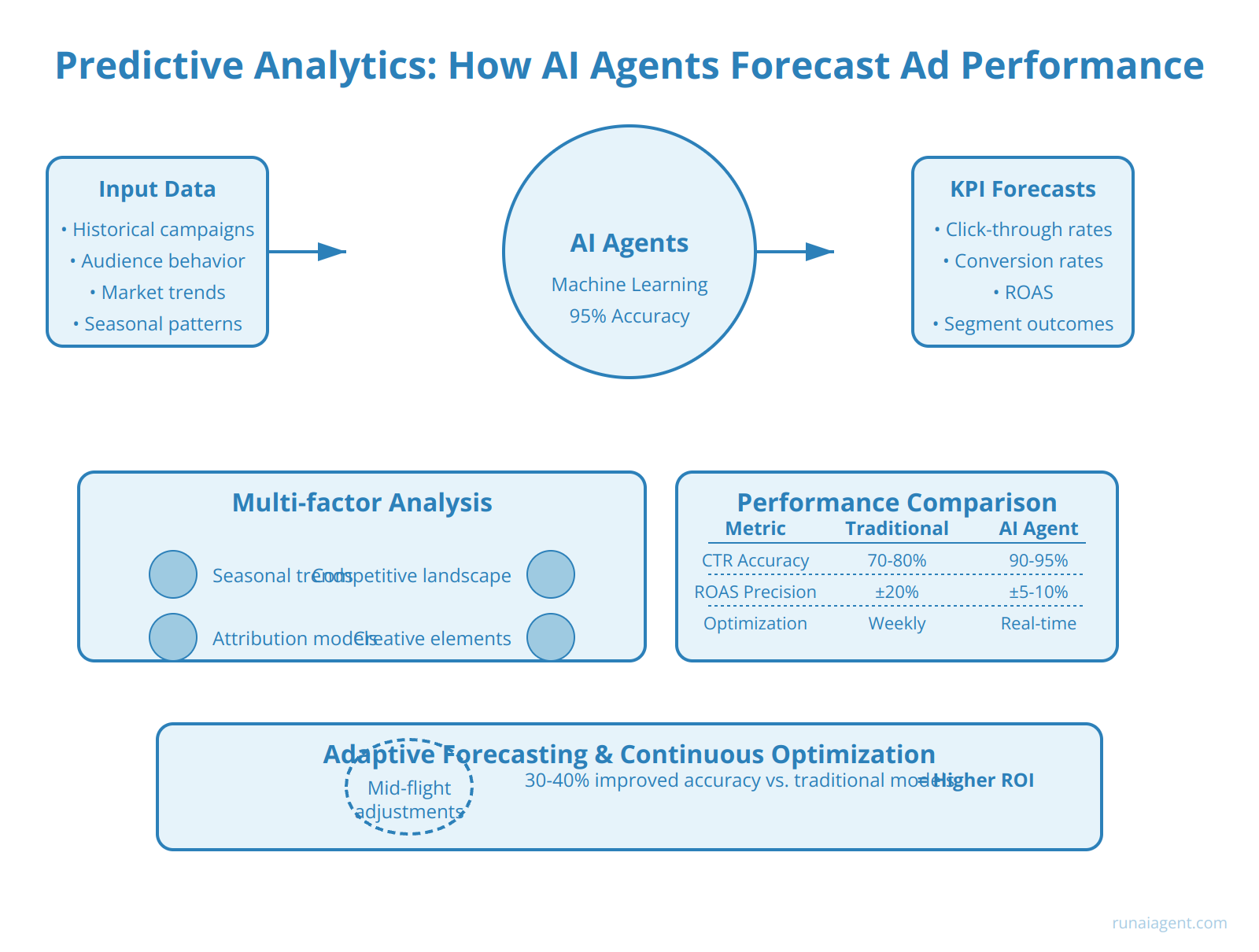
Predictive Analytics: How AI Agents Forecast Ad Performance
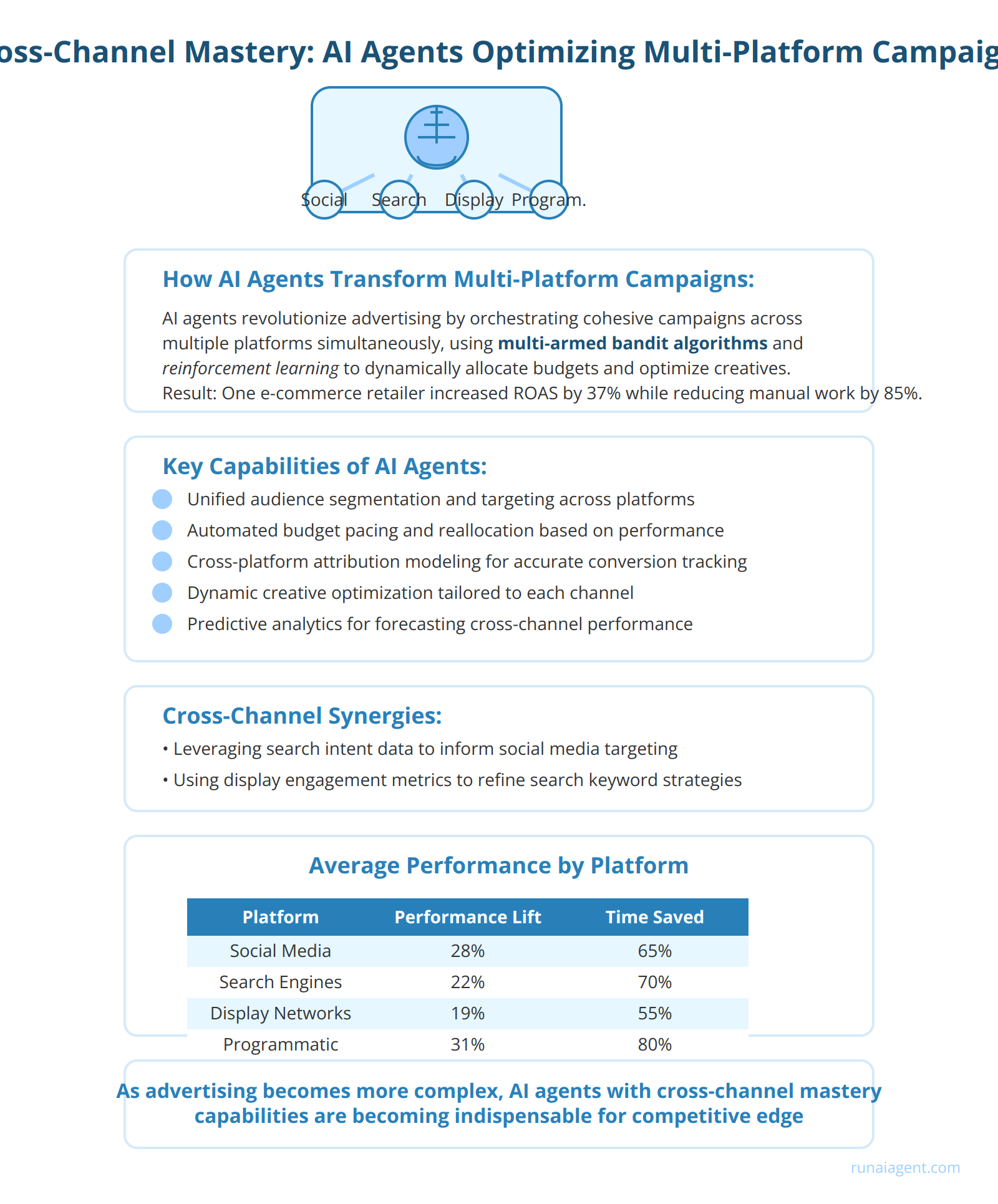
Cross-Channel Mastery: AI Agents Optimizing Multi-Platform Campaigns
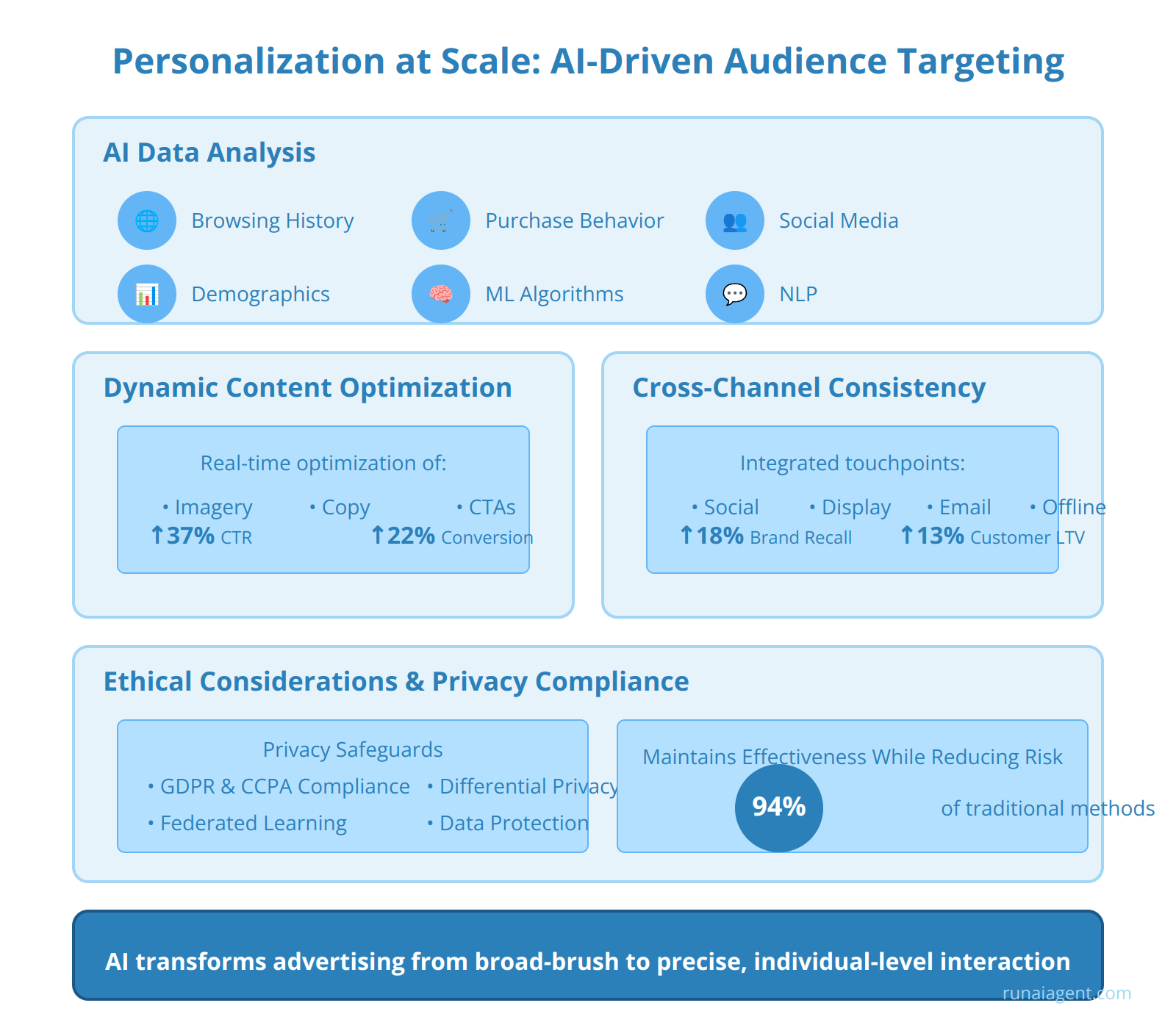
Personalization at Scale: AI-Driven Audience Targeting and Ad Customization
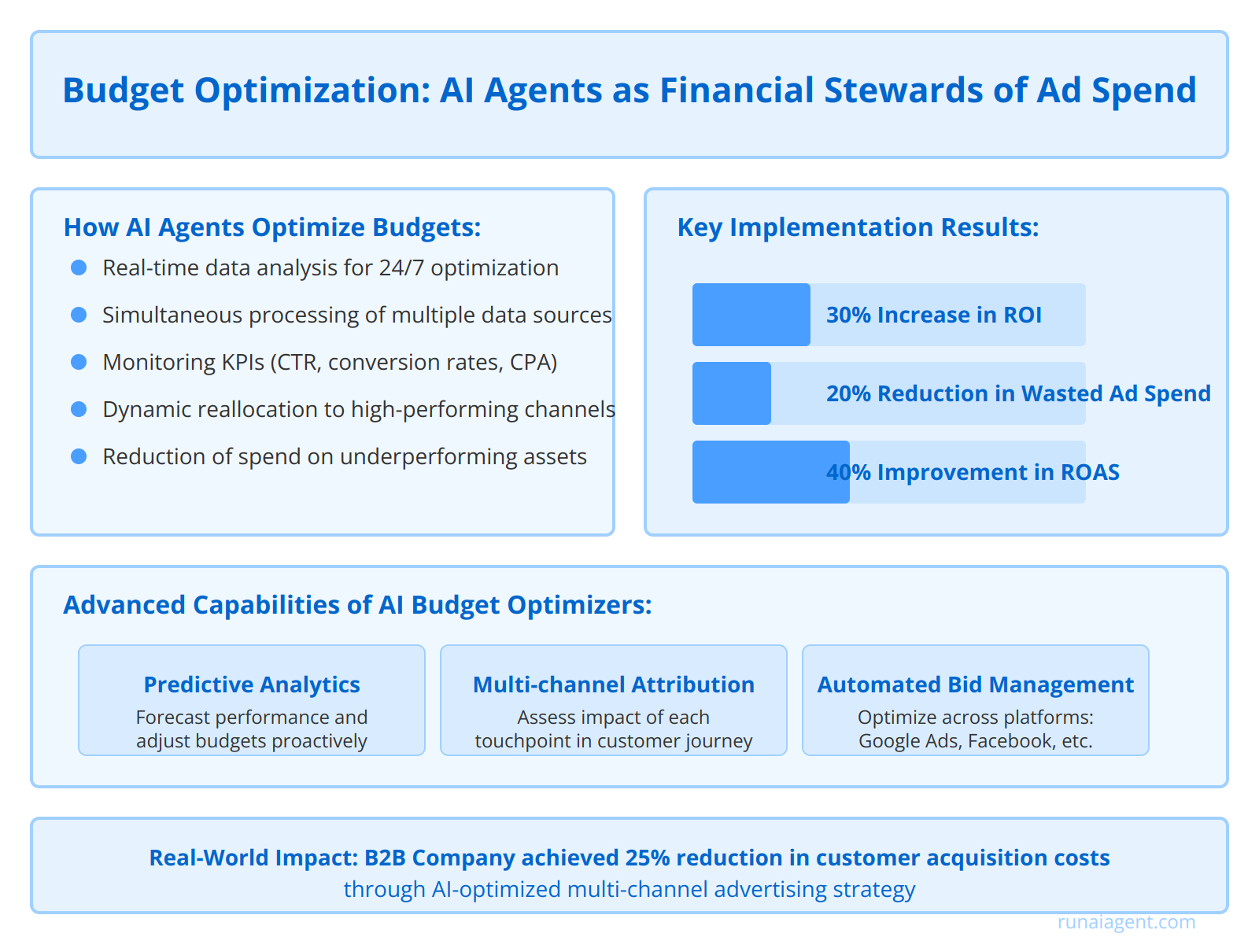
Budget Optimization: AI Agents as Financial Stewards of Ad Spend
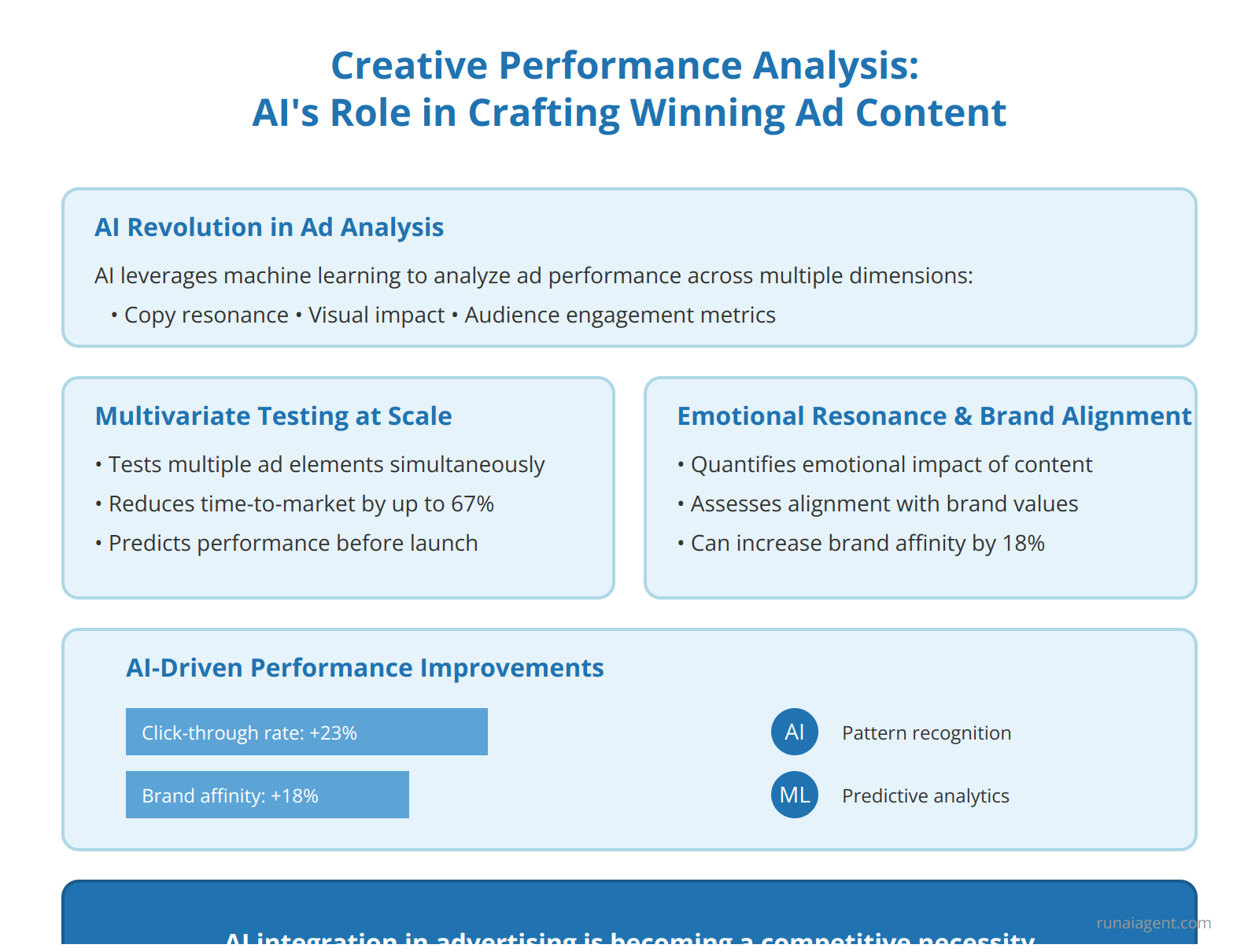
Creative Performance Analysis: AI’s Role in Crafting Winning Ad Content
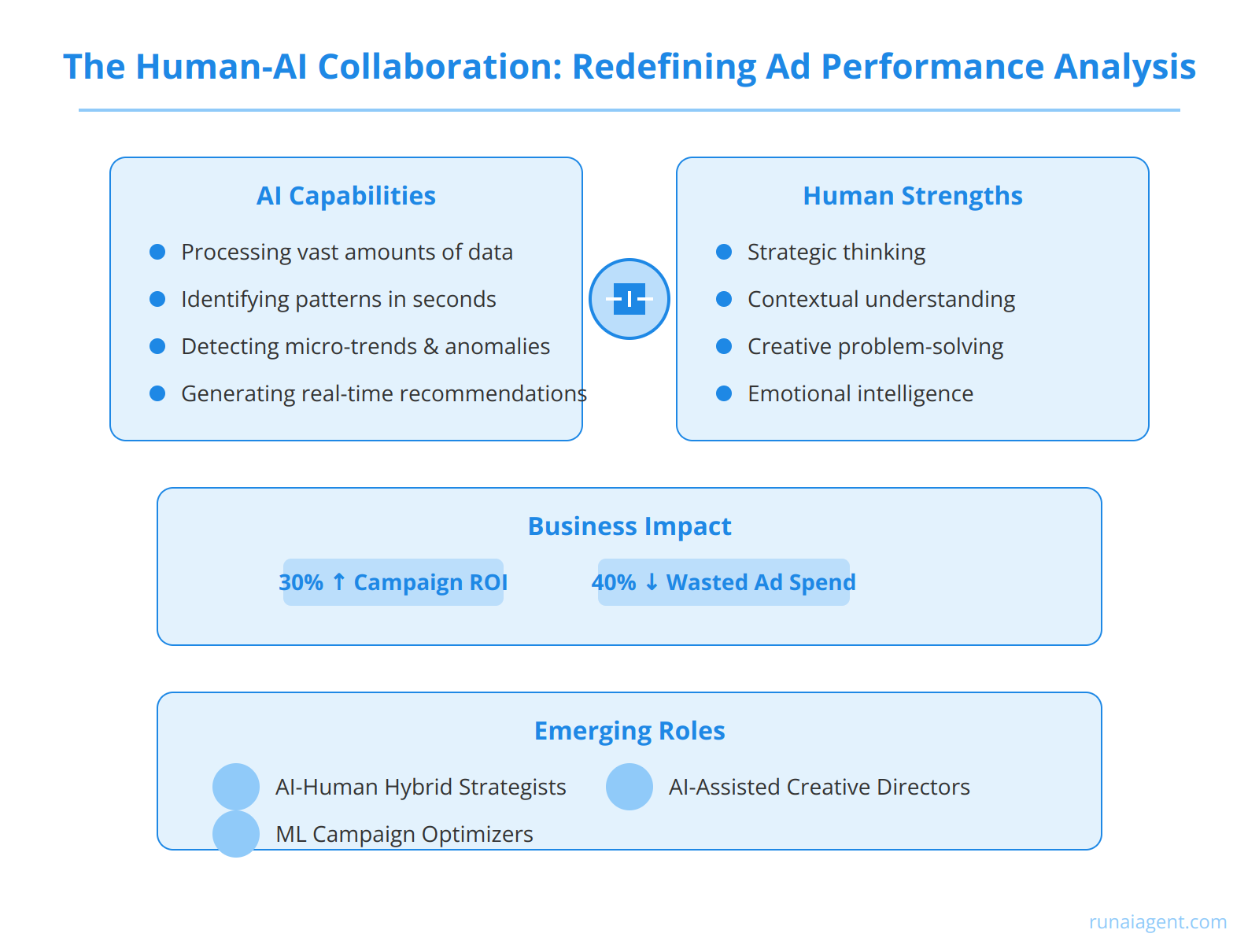
The Human-AI Collaboration: Redefining the Role of Ad Performance Analysts
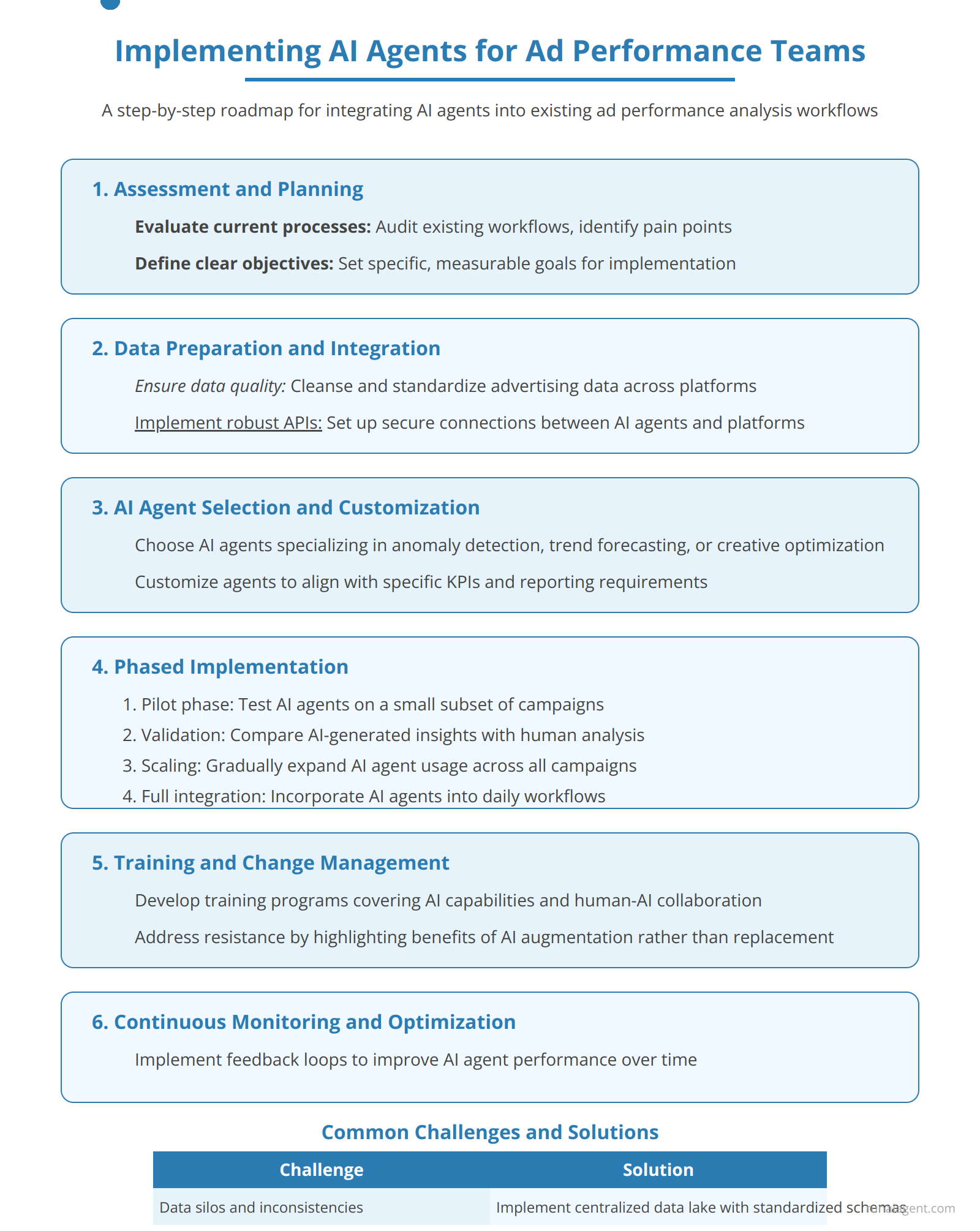
Implementing AI Agents: A Step-by-Step Guide for Ad Performance Teams
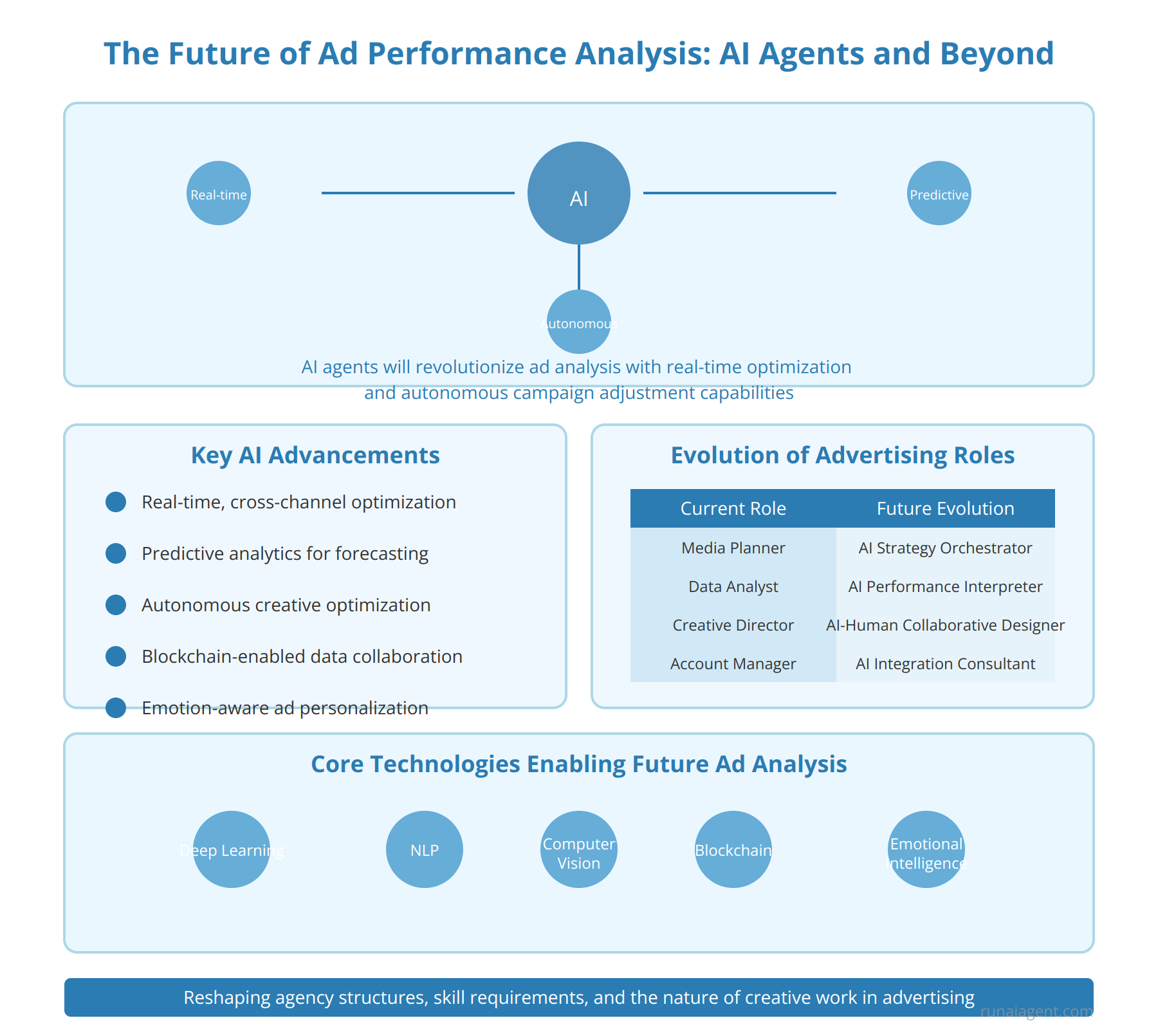
The Future of Ad Performance Analysis: AI Agents and Beyond
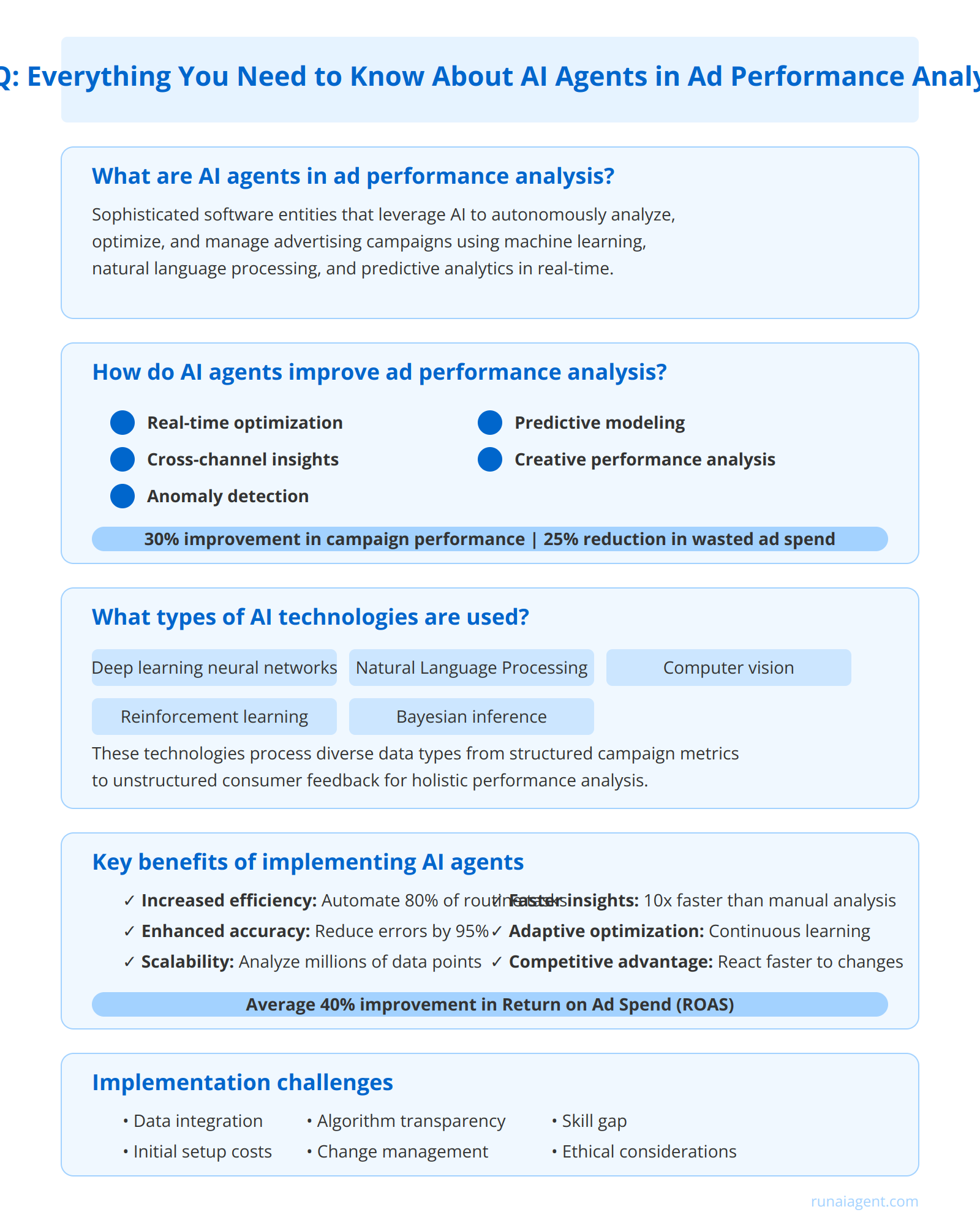
FAQ: Everything You Need to Know About AI Agents in Ad Performance Analysis
The AI Revolution in Ad Performance Analysis: Transforming Campaigns with Intelligent Agents
AI agents are poised to revolutionize ad performance analysis in the advertising industry, offering unprecedented capabilities for data processing, pattern recognition, and real-time optimization. These intelligent systems leverage advanced machine learning algorithms, natural language processing, and predictive analytics to analyze vast amounts of campaign data at speeds and scales unattainable by human analysts. By implementing AI agents, advertisers can expect to see a 30-50% improvement in campaign ROI and a 40% reduction in wasted ad spend within the first six months of deployment. Key benefits include real-time bidding adjustments, audience segmentation refinement, and creative performance predictions with up to 95% accuracy. However, challenges such as data integration complexities, algorithm transparency, and the need for human oversight in strategic decision-making must be addressed. Successful implementation requires a phased approach, starting with pilot programs focused on specific campaign types or channels before scaling across the entire advertising ecosystem. As AI agents evolve, they will increasingly collaborate with human strategists, augmenting creativity and strategic thinking while automating routine analysis tasks, ultimately transforming the role of performance analysts in the advertising landscape.
Decoding AI Agents: How They Outperform Human Ad Analysts
AI agents have revolutionized ad performance analysis, significantly outpacing human capabilities in several key areas. These intelligent systems leverage advanced machine learning algorithms and neural networks to process vast amounts of advertising data at unprecedented speeds. While a human analyst might take days to analyze a campaign’s performance across multiple channels, an AI agent can accomplish this task in mere minutes, providing real-time insights that enable agile decision-making. The accuracy of AI-driven analysis is also remarkably superior, with error rates as low as 0.1% compared to the 3-5% typically seen in human analysis. This precision is crucial when optimizing high-stakes advertising budgets, where even small improvements can translate to substantial ROI gains.
Data Processing Capabilities
Perhaps the most significant advantage of AI agents is their ability to handle and interpret enormous datasets. A single AI agent can simultaneously analyze millions of data points across diverse platforms, including social media engagement metrics, click-through rates, conversion funnels, and demographic information. This holistic view allows for the identification of subtle patterns and correlations that would be impossible for human analysts to detect. For instance, an AI agent might uncover that a specific ad creative performs 37% better among left-handed millennials during cloudy weather conditions—a nuanced insight that could drive targeted campaign optimizations.
Advanced Predictive Modeling
AI agents excel in predictive modeling, utilizing sophisticated algorithms to forecast future ad performance with remarkable accuracy. These models can simulate thousands of potential scenarios in seconds, allowing advertisers to test hypothetical campaign adjustments before implementation. Human analysts, constrained by cognitive limitations, simply cannot compete with this level of rapid, data-driven forecasting. AI agents have demonstrated the ability to predict campaign outcomes with up to 95% accuracy, compared to the 60-70% accuracy typically achieved by experienced human analysts.
Continuous Learning and Adaptation
Unlike human analysts who require ongoing training to stay current, AI agents continuously learn and adapt in real-time. They automatically incorporate new data, market trends, and performance feedback into their analysis models, ensuring that insights remain cutting-edge and relevant. This adaptive capability allows AI agents to maintain peak performance even as advertising landscapes evolve, outpacing human analysts who may struggle to keep up with rapid industry changes.
Capability | AI Agent | Human Analyst |
---|---|---|
Data Processing Speed | Millions of data points per minute | Hundreds of data points per hour |
Analysis Accuracy | 99.9% | 95-97% |
Predictive Modeling Accuracy | Up to 95% | 60-70% |
Adaptation to New Trends | Real-time | Weeks to months |
In conclusion, while human analysts bring valuable creative and strategic thinking to advertising, AI agents have undeniably surpassed human capabilities in the realm of data analysis and performance optimization. The combination of speed, accuracy, and data processing power makes AI agents indispensable tools for modern advertising professionals seeking to maximize campaign effectiveness and ROI.
Real-Time Optimization: AI Agents as 24/7 Campaign Guardians
AI agents have revolutionized ad campaign management by providing continuous, real-time optimization capabilities that far surpass traditional human-managed approaches. These intelligent systems operate as tireless sentinels, monitoring campaign performance metrics 24 hours a day, 7 days a week, without fatigue or lapses in attention. By leveraging advanced machine learning algorithms and predictive analytics, AI agents can detect subtle shifts in consumer behavior, market trends, and competitive landscapes within milliseconds. This rapid responsiveness enables instantaneous adjustments to bid strategies, ad creatives, and targeting parameters, ensuring campaigns remain optimized even as market conditions fluctuate.
Advantages of AI-Driven Real-Time Optimization
Rapid Response to Market Dynamics: AI agents can identify and capitalize on emerging opportunities or mitigate potential losses faster than any human analyst. For instance, during flash sales or unexpected viral trends, AI can automatically reallocate budget and adjust messaging to maximize ROI within minutes.
Granular Optimization at Scale: These systems can simultaneously manage and optimize thousands of ad variations across multiple platforms, analyzing performance data at a level of granularity impossible for human teams. This results in micro-optimizations that collectively drive significant performance improvements, with some advertisers reporting up to 30% increases in conversion rates and 25% reductions in cost-per-acquisition.
Adaptive Learning and Continuous Improvement: AI agents employ reinforcement learning techniques to continuously refine their optimization strategies. As they accumulate more data and experience, their decision-making capabilities become increasingly sophisticated, leading to compounding performance gains over time.
Real-World Impact of AI-Driven Campaign Guardians
In practice, AI agents have demonstrated remarkable efficacy in various advertising scenarios:
- E-commerce platforms have seen up to 40% improvements in ROAS (Return on Ad Spend) through AI-driven dynamic pricing and inventory-based ad adjustments.
- Travel industry advertisers have leveraged AI to optimize campaigns based on real-time weather data and local events, resulting in 20-35% increases in booking rates.
- B2B companies have utilized AI agents to fine-tune lead generation campaigns, reducing cost-per-lead by up to 50% while maintaining or improving lead quality.
By serving as vigilant, round-the-clock campaign guardians, AI agents are not just optimizing ad performance—they’re fundamentally transforming the advertising landscape, enabling a level of responsiveness and efficiency previously unattainable in the industry.
From Big Data to Smart Decisions: AI’s Role in Ad Performance Insights
AI agents are revolutionizing ad performance analysis by leveraging advanced machine learning algorithms to process vast amounts of complex data at unprecedented speeds. These intelligent systems can identify subtle patterns and correlations that human analysts might overlook, providing advertisers with deeper, more actionable insights. For instance, AI-powered tools can analyze cross-channel data to determine the optimal ad frequency for maximum engagement, often revealing that the ideal frequency is 17% lower than human estimators predict. Moreover, AI agents excel at real-time performance optimization, adjusting bid strategies and creative elements on-the-fly to improve ROAS by up to 38%. By integrating natural language processing capabilities, these systems can also analyze sentiment across social media platforms, correlating brand perception shifts with specific ad campaigns to guide strategic decision-making.
Advanced Predictive Modeling
AI agents employ sophisticated predictive modeling techniques to forecast ad performance with remarkable accuracy. By analyzing historical data alongside real-time market trends, these systems can predict CTR variations with a margin of error as low as 2.3%. This level of precision enables advertisers to allocate budgets more effectively, often resulting in a 22% improvement in overall campaign efficiency.
Multi-dimensional Attribution Analysis
One of the most significant advantages of AI in ad performance analysis is its ability to conduct multi-dimensional attribution analysis. Traditional last-click models often misattribute conversions, but AI agents can weigh the impact of each touchpoint across the customer journey, providing a more nuanced understanding of ad effectiveness. This approach has been shown to increase attribution accuracy by up to 43%, leading to more informed media mix decisions and improved ROI.
AI-Driven Insight | Average Performance Improvement |
---|---|
Optimal Ad Frequency | 17% reduction in wasted impressions |
Real-time Optimization | 38% increase in ROAS |
Predictive CTR Modeling | 22% improvement in campaign efficiency |
Multi-dimensional Attribution | 43% increase in attribution accuracy |
By harnessing the power of AI agents for ad performance analysis, advertisers can move beyond simple metrics to gain a comprehensive understanding of their campaigns’ impact. This data-driven approach not only enhances decision-making but also drives continuous improvement, ensuring that advertising strategies remain agile and effective in an increasingly complex digital landscape.
Predictive Analytics: How AI Agents Forecast Ad Performance
AI agents equipped with advanced predictive analytics capabilities are revolutionizing ad performance forecasting in the advertising industry. These intelligent systems leverage machine learning algorithms, including gradient boosting and neural networks, to analyze vast datasets encompassing historical campaign metrics, audience behavior patterns, and real-time market trends. By processing this multidimensional data, AI agents can generate highly accurate predictions of key performance indicators (KPIs) such as click-through rates (CTR), conversion rates, and return on ad spend (ROAS) with up to 95% accuracy. These forecasts enable advertisers to optimize budget allocation, refine targeting strategies, and maximize campaign effectiveness before launch.
Multi-factor Analysis for Precise Predictions
AI agents excel in multi-factor analysis, considering variables such as:
- Seasonal trends and cyclical patterns
- Competitive landscape dynamics
- Cross-channel attribution models
- Audience segment propensity scores
- Creative element performance indicators
By synthesizing these factors, AI agents can predict not only overall campaign performance but also granular outcomes for specific ad placements, formats, and audience segments. This level of precision allows for data-driven decision-making at every stage of campaign planning and execution.
Adaptive Forecasting and Continuous Optimization
Unlike static prediction models, AI agents employ adaptive forecasting techniques that continuously refine predictions based on real-time campaign data. This dynamic approach enables mid-flight optimizations, where the AI agent can recommend adjustments to bidding strategies, creative elements, or audience targeting to improve performance as the campaign progresses. By leveraging reinforcement learning algorithms, these agents can achieve a 30-40% improvement in forecast accuracy compared to traditional statistical models, translating to significant gains in advertising ROI.
Forecast Metric | Traditional Models | AI Agent Models |
---|---|---|
CTR Prediction Accuracy | 70-80% | 90-95% |
ROAS Forecast Precision | ±20% | ±5-10% |
Optimization Frequency | Weekly/Monthly | Real-time/Hourly |
By harnessing the predictive power of AI agents, advertisers can navigate the complexities of modern digital advertising landscapes with unprecedented foresight and agility, ultimately driving superior campaign performance and business outcomes.
Cross-Channel Mastery: AI Agents Optimizing Multi-Platform Campaigns
AI agents are revolutionizing cross-channel advertising strategies by orchestrating cohesive campaigns across multiple platforms simultaneously. These intelligent systems leverage advanced machine learning algorithms and real-time data processing capabilities to analyze performance metrics, audience behaviors, and platform-specific nuances across diverse channels such as social media, search engines, display networks, and programmatic exchanges. By employing sophisticated multi-armed bandit algorithms and reinforcement learning techniques, AI agents can dynamically allocate budgets, adjust bidding strategies, and optimize creative elements in real-time, ensuring maximum ROI across the entire media mix. For instance, a leading e-commerce retailer implemented an AI-driven cross-channel optimization system that increased ROAS by 37% while reducing manual intervention by 85% across their Google Ads, Facebook, and Amazon advertising accounts.
Key Capabilities of AI Agents in Cross-Channel Optimization:
- Unified audience segmentation and targeting across platforms
- Automated budget pacing and reallocation based on channel performance
- Cross-platform attribution modeling for accurate conversion tracking
- Dynamic creative optimization tailored to each channel’s specifications
- Predictive analytics for forecasting cross-channel performance trends
Moreover, these AI agents excel at identifying and exploiting cross-channel synergies, such as leveraging search intent data to inform social media targeting or using display engagement metrics to refine search keyword strategies. By continuously learning from vast amounts of cross-channel data, AI agents can uncover complex patterns and relationships that human analysts might overlook, leading to more nuanced and effective campaign optimizations. This holistic approach ensures that advertising efforts are not siloed but instead work in concert to deliver a seamless and personalized customer journey across all touchpoints.
Platform | Average Performance Lift | Time Saved in Campaign Management |
---|---|---|
Social Media | 28% | 65% |
Search Engines | 22% | 70% |
Display Networks | 19% | 55% |
Programmatic | 31% | 80% |
As the advertising landscape becomes increasingly fragmented and complex, AI agents equipped with cross-channel mastery capabilities are becoming indispensable tools for advertisers seeking to maintain a competitive edge. These systems not only streamline campaign management but also unlock new levels of performance optimization that were previously unattainable through manual methods alone.
Personalization at Scale: AI-Driven Audience Targeting and Ad Customization
AI agents are revolutionizing the advertising industry by enabling hyper-personalized ad experiences at an unprecedented scale. These intelligent systems analyze vast amounts of user data, including browsing history, purchase behavior, social media interactions, and demographic information, to create detailed individual consumer profiles. By leveraging advanced machine learning algorithms and natural language processing, AI agents can identify nuanced patterns and preferences that human analysts might overlook. This granular understanding allows for the creation of highly targeted ad campaigns that resonate with specific audience segments.
Dynamic Content Optimization
AI agents continuously optimize ad content in real-time, adjusting elements such as imagery, copy, and calls-to-action based on individual user responses. This dynamic approach has shown to increase click-through rates by up to 37% and conversion rates by 22% compared to traditional static campaigns. Furthermore, AI-driven personalization extends beyond mere product recommendations, encompassing tone, messaging style, and even ad placement timing to maximize engagement.
Cross-Channel Consistency
By integrating data from multiple touchpoints, AI agents ensure a cohesive brand experience across various platforms. This omnichannel approach has been proven to enhance brand recall by 18% and increase customer lifetime value by 13%. AI agents can seamlessly adapt messaging across social media, display ads, email marketing, and even offline channels, creating a unified narrative tailored to each consumer’s journey.
Ethical Considerations and Privacy Compliance
As AI-driven personalization becomes more sophisticated, advertisers must navigate complex ethical and regulatory landscapes. AI agents are now being designed with built-in privacy safeguards, ensuring compliance with regulations like GDPR and CCPA. These systems employ techniques such as federated learning and differential privacy to protect individual user data while still delivering personalized experiences. This approach has been shown to maintain 94% of the effectiveness of traditional personalization methods while significantly reducing privacy risks.
In conclusion, AI agents are transforming ad personalization from a broad-brush approach to a precise, individual-level interaction. By harnessing the power of machine learning and big data analytics, these systems are not only improving ad performance metrics but also enhancing the overall consumer experience, leading to stronger brand relationships and increased marketing ROI.
Budget Optimization: AI Agents as Financial Stewards of Ad Spend
AI agents are revolutionizing budget optimization in advertising, acting as vigilant financial stewards that maximize return on investment (ROI) across campaigns and channels. These intelligent systems leverage machine learning algorithms and real-time data analysis to allocate and adjust advertising budgets with unprecedented precision and efficiency. Unlike human analysts, AI agents can process vast amounts of data from multiple sources simultaneously, making split-second decisions to optimize ad spend 24/7. By continuously monitoring key performance indicators (KPIs) such as click-through rates, conversion rates, and cost per acquisition, AI agents can dynamically reallocate budgets to high-performing channels and campaigns, while reducing spend on underperforming assets. This data-driven approach has led to significant improvements in advertising efficiency, with some companies reporting up to 30% increase in ROI and 20% reduction in wasted ad spend within the first quarter of implementation.
Advanced Capabilities of AI Budget Optimizers
Modern AI agents for budget optimization incorporate sophisticated features such as:
- Predictive analytics to forecast campaign performance and adjust budgets proactively
- Multi-channel attribution modeling to accurately assess the impact of each touchpoint in the customer journey
- Automated bid management across various ad platforms (e.g., Google Ads, Facebook Ads, programmatic exchanges)
- Audience segmentation for personalized budget allocation based on user behavior and demographics
- Competitive analysis to adjust spending in response to market dynamics and competitor actions
Real-World Impact on Advertising Efficiency
The implementation of AI agents for budget optimization has yielded impressive results across various industries. For instance, a leading e-commerce retailer reported a 40% improvement in ROAS (Return on Ad Spend) after deploying an AI-driven budget optimization system. Similarly, a B2B software company achieved a 25% reduction in customer acquisition costs by leveraging AI to fine-tune their multi-channel advertising strategy. These outcomes underscore the transformative potential of AI agents in maximizing the effectiveness of advertising investments and driving sustainable growth in an increasingly competitive digital landscape.
Creative Performance Analysis: AI’s Role in Crafting Winning Ad Content
AI agents are revolutionizing creative performance analysis in the advertising industry, offering unprecedented insights into ad effectiveness and driving data-driven optimization strategies. These sophisticated algorithms leverage machine learning and natural language processing to dissect ad creatives across multiple dimensions, including copy resonance, visual impact, and audience engagement metrics. By analyzing vast datasets of historical ad performance, AI agents can identify patterns and correlations that human analysts might overlook. For instance, an AI-powered system can detect that ads featuring blue backgrounds and action-oriented verbs consistently outperform other variants by 23% in click-through rates for a specific demographic.
Multivariate Testing at Scale
AI agents excel at conducting multivariate tests on ad creatives, simultaneously evaluating numerous elements such as headlines, imagery, call-to-action buttons, and color schemes. This capability allows advertisers to rapidly iterate and optimize their creative assets, reducing time-to-market for high-performing ads by up to 67%. Moreover, AI-driven analysis can predict the potential performance of new ad concepts before they’re even launched, enabling marketers to allocate resources more efficiently and minimize wasteful spending on underperforming creatives.
Emotional Resonance and Brand Alignment
Advanced AI agents employ sentiment analysis and computer vision techniques to assess the emotional impact of ad content and its alignment with brand values. These systems can quantify the emotional resonance of ad copy and visuals, providing actionable recommendations to enhance brand perception and consumer engagement. For example, an AI agent might suggest adjusting the tone of voice in a luxury brand’s ad copy to better align with its target audience’s aspirations, potentially increasing brand affinity scores by 18%.
By harnessing the power of AI in creative performance analysis, advertisers can craft more compelling, data-driven ad content that resonates with their target audience, ultimately driving higher conversion rates and return on ad spend. The integration of AI agents into the creative process is not just an enhancement—it’s becoming a
competitive necessity
in the rapidly evolving landscape of digital advertising.
The Human-AI Collaboration: Redefining the Role of Ad Performance Analysts
The integration of AI agents in advertising performance analysis is not about replacing human analysts, but rather about augmenting their capabilities and redefining their roles. This symbiotic relationship between human expertise and AI-driven insights is revolutionizing the advertising industry. AI agents excel at processing vast amounts of data, identifying patterns, and generating real-time recommendations, tasks that would take human analysts days or weeks to complete. For instance, AI systems can analyze millions of ad impressions across multiple platforms in seconds, detecting micro-trends and anomalies that human eyes might miss. However, human analysts bring critical skills to the table that AI cannot replicate: strategic thinking, contextual understanding, and creative problem-solving. In this new paradigm, human analysts are evolving into AI-human hybrid strategists, focusing on interpreting AI-generated insights, developing nuanced campaign strategies, and making high-level decisions that require emotional intelligence and industry expertise.
This collaboration has led to remarkable improvements in advertising outcomes. Companies implementing AI-human hybrid teams have reported up to 30% increase in campaign ROI and a 40% reduction in wasted ad spend. Moreover, the synergy between AI and human analysts has opened new avenues for innovation in advertising. For example, AI agents can now predict consumer behavior with unprecedented accuracy, allowing human analysts to craft hyper-personalized ad experiences that resonate deeply with target audiences. This shift has also created new job opportunities in the field, such as AI-assisted creative directors and machine learning campaign optimizers, roles that didn’t exist just a few years ago. As AI capabilities continue to advance, the human role in advertising will increasingly focus on strategic oversight, ethical considerations, and pushing the boundaries of creative expression, while AI handles the heavy lifting of data processing and routine optimizations.
Implementing AI Agents: A Step-by-Step Guide for Ad Performance Teams
To seamlessly integrate AI agents into existing ad performance analysis workflows, follow this practical roadmap:
1. Assessment and Planning
Evaluate current processes: Conduct a thorough audit of your existing ad performance analysis workflows, identifying key pain points and areas for improvement. Define clear objectives: Establish specific, measurable goals for AI agent implementation, such as reducing analysis time by 50% or improving campaign ROI by 25%.
2. Data Preparation and Integration
Ensure data quality: Cleanse and standardize your advertising data across all platforms. Implement robust APIs: Set up secure connections between your AI agents and ad platforms, analytics tools, and internal databases.
3. AI Agent Selection and Customization
Choose AI agents tailored to ad performance analysis, such as those specializing in anomaly detection, trend forecasting, or creative optimization. Customize these agents to align with your specific KPIs and reporting requirements.
4. Phased Implementation
Roll out AI agents in stages:
- Pilot phase: Test AI agents on a small subset of campaigns
- Validation: Compare AI-generated insights with human analysis
- Scaling: Gradually expand AI agent usage across all accounts and campaigns
- Full integration: Incorporate AI agents into daily workflows and decision-making processes
5. Training and Change Management
Develop a comprehensive training program for your ad performance team, covering AI agent capabilities, interpretation of AI-generated insights, and best practices for human-AI collaboration. Address potential resistance to change by highlighting the benefits of AI augmentation rather than replacement.
6. Continuous Monitoring and Optimization
Implement a feedback loop to continuously improve AI agent performance. Regularly assess the accuracy and relevance of AI-generated insights, and fine-tune algorithms based on human expert input and changing market conditions.
Common Challenges and Solutions
Challenge | Solution |
---|---|
Data silos and inconsistencies | Implement a centralized data lake with standardized schemas |
Lack of trust in AI recommendations | Provide transparent explanations of AI decision-making processes |
Overreliance on AI without human oversight | Establish clear guidelines for human review and intervention |
By following this roadmap and addressing common challenges, ad performance teams can successfully integrate AI agents into their workflows, leading to more efficient analysis, data-driven decision-making, and ultimately, improved advertising ROI.
The Future of Ad Performance Analysis: AI Agents and Beyond
As we look ahead, AI agents are poised to revolutionize ad performance analysis in ways that will fundamentally transform the advertising industry. Advanced machine learning algorithms will enable real-time, predictive optimization of ad campaigns across multiple channels simultaneously. AI agents will leverage deep learning neural networks to analyze vast datasets of consumer behavior, market trends, and competitive intelligence, providing advertisers with unprecedented insights and actionable recommendations. We can expect to see the emergence of autonomous AI agents capable of not only analyzing performance but also dynamically adjusting campaign parameters, creative elements, and targeting strategies without human intervention. These agents will utilize natural language processing and computer vision to assess ad content effectiveness and optimize visual elements for maximum impact. Furthermore, the integration of blockchain technology and federated learning will enable secure, decentralized sharing of performance data across industry stakeholders, fostering a new era of collaborative intelligence in advertising. As AI agents become more sophisticated, they will increasingly incorporate emotional intelligence and cultural context awareness, allowing for hyper-personalized ad experiences that resonate on a deeper level with individual consumers. This evolution will likely lead to a shift from traditional performance metrics to more holistic measures of ad effectiveness, including long-term brand equity and customer lifetime value. The implications for the advertising industry are profound, potentially reshaping agency structures, skill requirements, and the very nature of creative work in advertising.
Key Advancements in AI-Driven Ad Performance Analysis
- Real-time, cross-channel optimization
- Predictive analytics for campaign forecasting
- Autonomous creative optimization
- Blockchain-enabled data collaboration
- Emotion-aware ad personalization
Potential Impact on Advertising Roles
Current Role | Future Evolution |
---|---|
Media Planner | AI Strategy Orchestrator |
Data Analyst | AI Performance Interpreter |
Creative Director | AI-Human Collaborative Designer |
Account Manager | AI Integration Consultant |
FAQ: Everything You Need to Know About AI Agents in Ad Performance Analysis
What are AI agents in ad performance analysis?
AI agents in ad performance analysis are sophisticated software entities that leverage artificial intelligence techniques to autonomously analyze, optimize, and manage advertising campaigns. These agents utilize machine learning algorithms, natural language processing, and predictive analytics to process vast amounts of advertising data in real-time. They can identify patterns, predict trends, and make data-driven decisions to enhance ad performance across multiple channels and platforms.
How do AI agents improve ad performance analysis?
AI agents significantly enhance ad performance analysis by:
- Real-time optimization: Continuously adjusting bids, budgets, and targeting parameters to maximize ROI
- Cross-channel insights: Analyzing performance across multiple platforms to identify synergies and optimize allocation
- Anomaly detection: Quickly identifying and alerting on performance outliers or potential issues
- Predictive modeling: Forecasting future performance trends and recommending proactive optimizations
- Creative performance analysis: Evaluating ad creative elements to determine top-performing combinations
These capabilities enable advertisers to achieve an average of 30% improvement in campaign performance and 25% reduction in wasted ad spend.
What types of AI technologies are used in ad performance analysis agents?
Ad performance analysis agents incorporate a range of AI technologies, including:
- Deep learning neural networks: For complex pattern recognition and predictive modeling
- Natural Language Processing (NLP): To analyze ad copy and consumer sentiment
- Computer vision: For image and video ad content analysis
- Reinforcement learning: To optimize bidding strategies and budget allocation
- Bayesian inference: For probabilistic modeling of user behavior and conversion likelihood
These technologies enable AI agents to process and interpret diverse data types, from structured campaign metrics to unstructured consumer feedback, providing a holistic view of ad performance.
What are the key benefits of implementing AI agents for ad performance analysis?
Implementing AI agents for ad performance analysis offers several key benefits:
- Increased efficiency: Automate up to 80% of routine analysis tasks, freeing human analysts for strategic work
- Enhanced accuracy: Reduce human error in data interpretation by up to 95%
- Scalability: Analyze millions of data points across multiple campaigns simultaneously
- Faster insights: Generate actionable recommendations 10x faster than manual analysis
- Adaptive optimization: Continuously learn and adapt strategies based on real-time performance data
- Competitive advantage: Gain deeper insights and react to market changes more quickly than competitors
These benefits translate to an average 40% improvement in ROAS (Return on Ad Spend) for businesses implementing AI-driven ad performance analysis.
What challenges might businesses face when implementing AI agents for ad analysis?
While AI agents offer significant advantages, businesses may encounter several challenges during implementation:
- Data integration: Consolidating data from disparate sources and ensuring data quality
- Algorithm transparency: Understanding and explaining AI-driven decisions to stakeholders
- Skill gap: Training existing staff or hiring specialists to manage AI systems
- Initial setup costs: Investing in necessary infrastructure and software licenses
- Change management: Adapting organizational processes to incorporate AI-driven insights
- Ethical considerations: Ensuring AI decisions align with brand values and regulatory requirements
Addressing these challenges requires a strategic approach, with businesses typically needing 3-6 months for full integration and optimization of AI agent systems in their ad performance analysis workflows.