Table of Contents
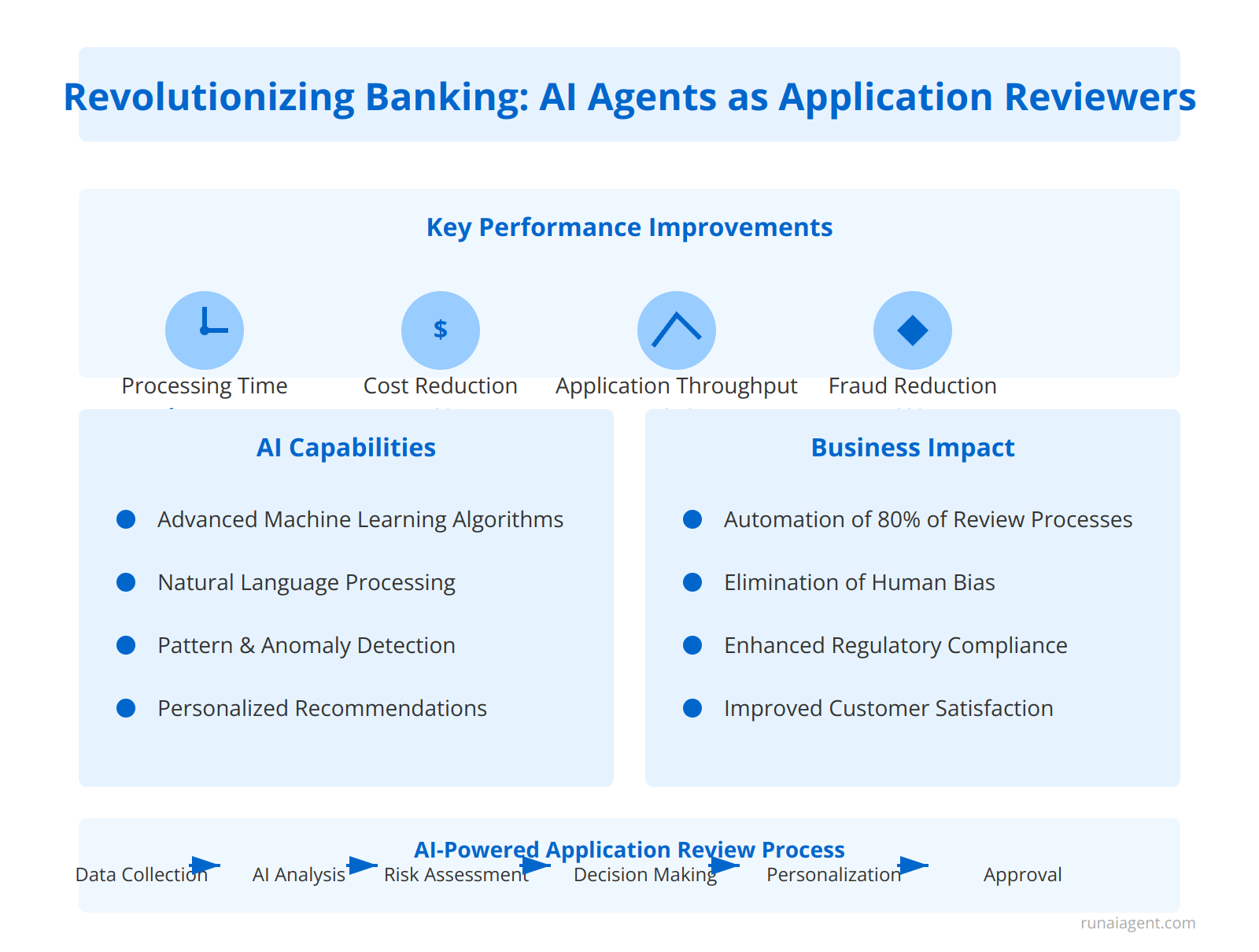
Revolutionizing Banking: AI Agents as Application Reviewers
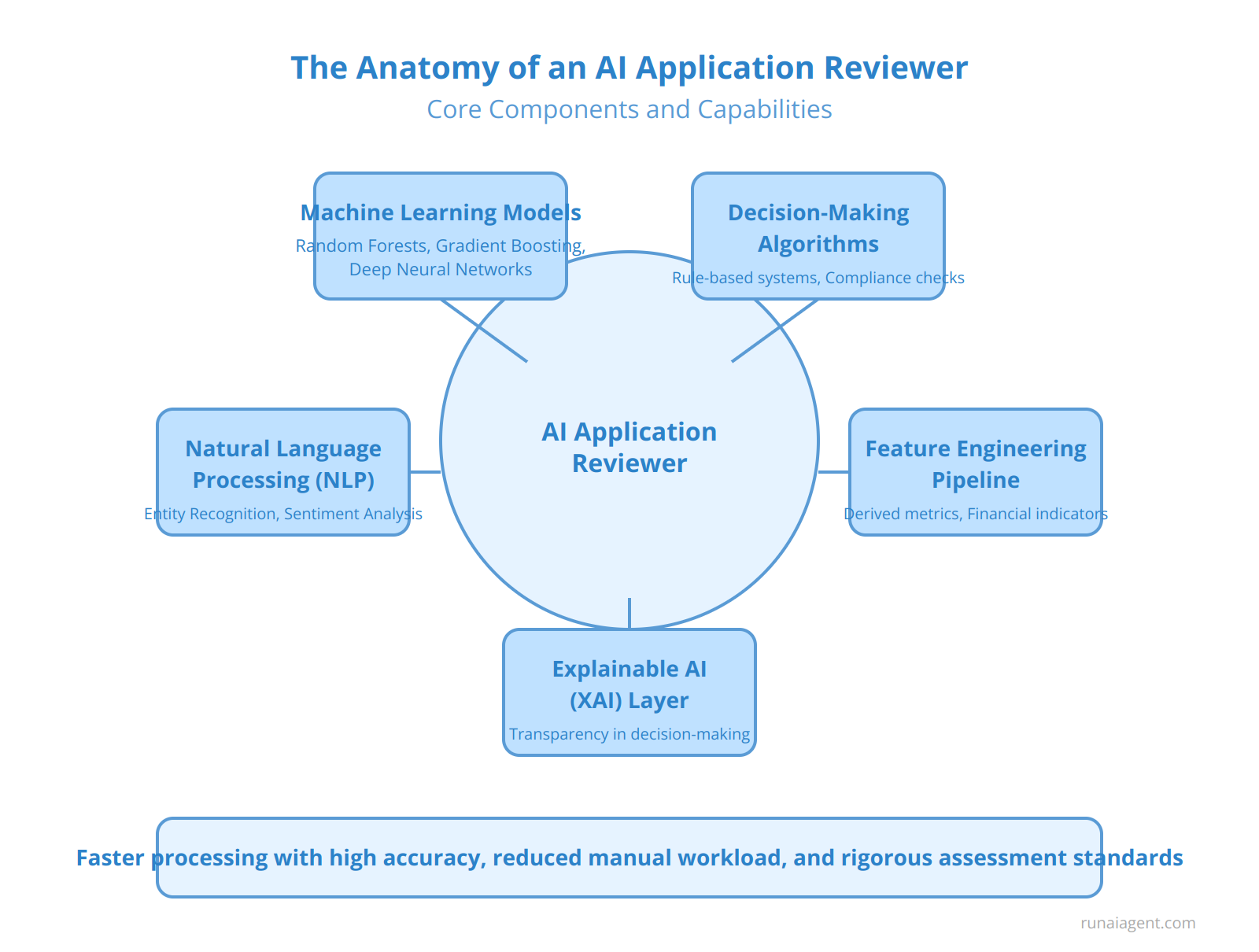
The Anatomy of an AI Application Reviewer: Core Components and Capabilities
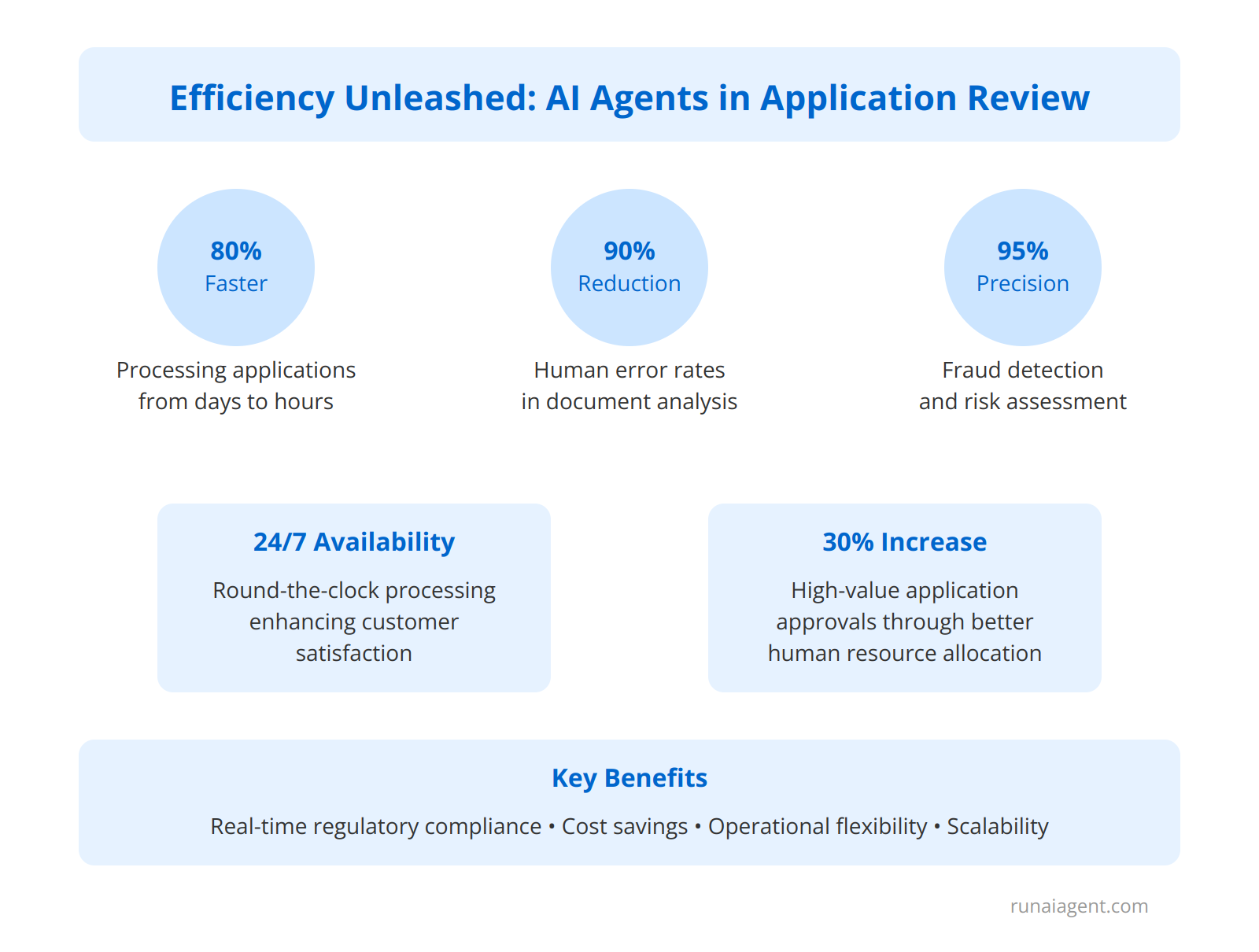
Efficiency Unleashed: How AI Agents Streamline the Application Review Process
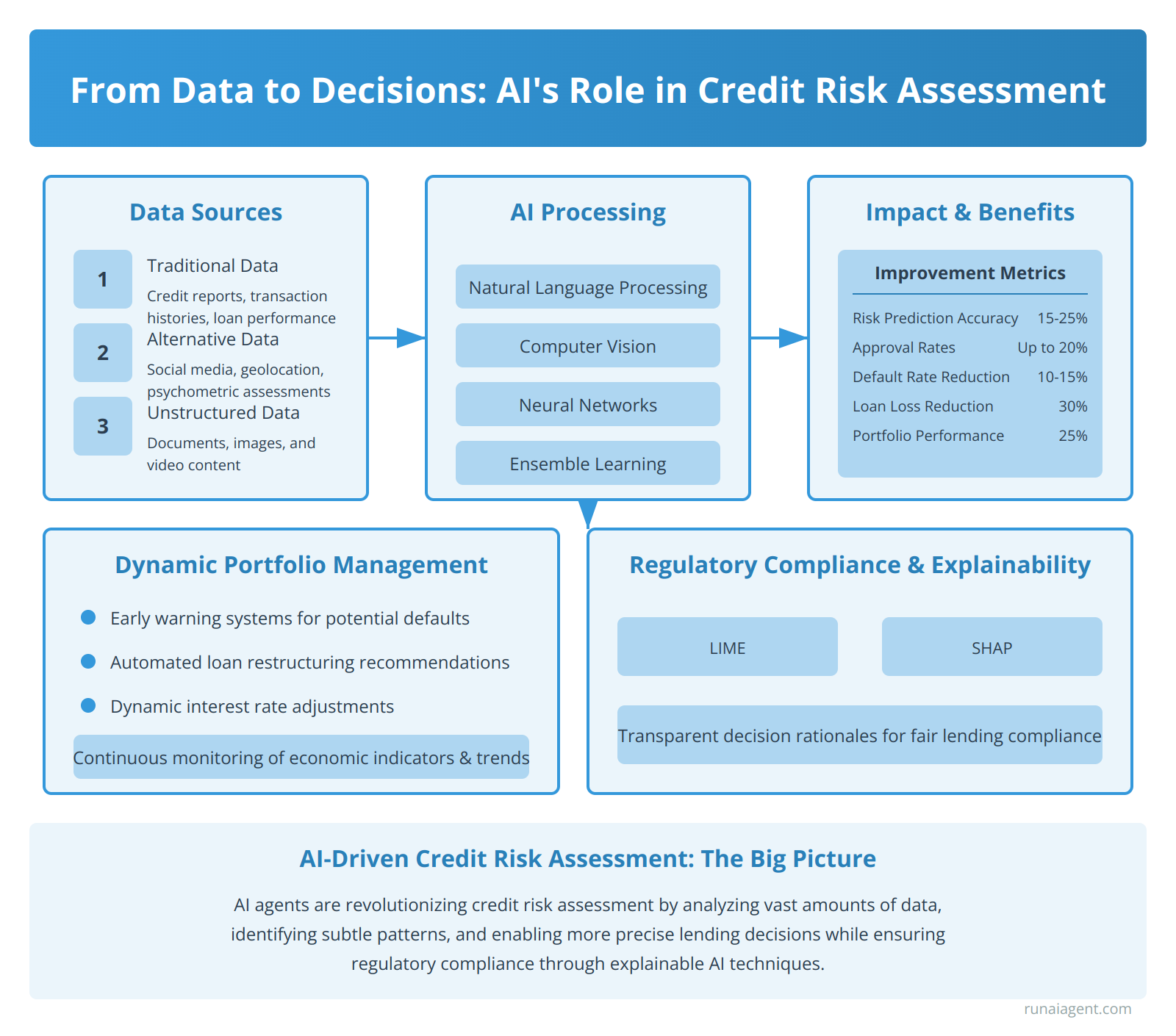
From Data to Decisions: AI’s Role in Credit Risk Assessment
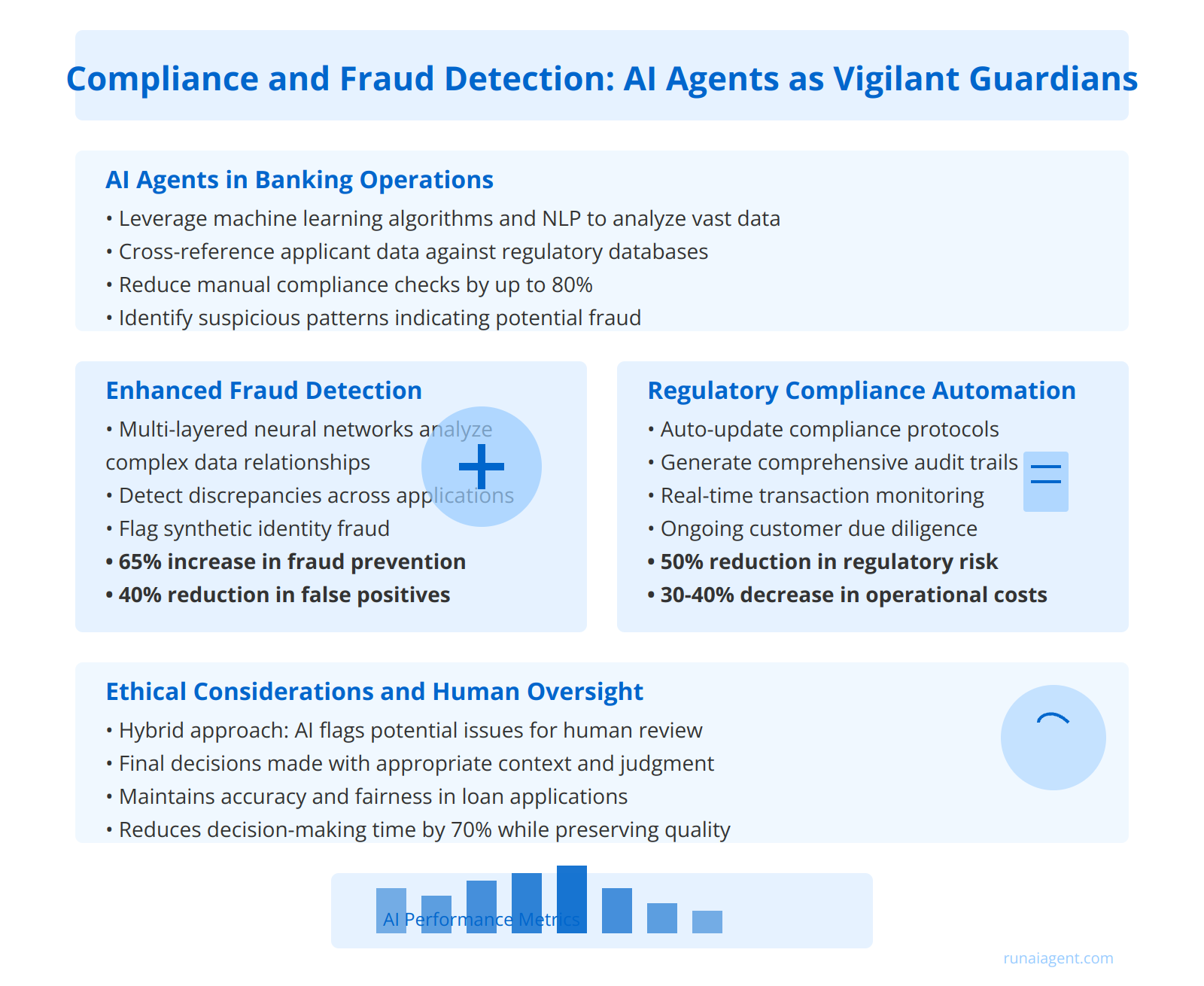
Compliance and Fraud Detection: AI Agents as Vigilant Guardians
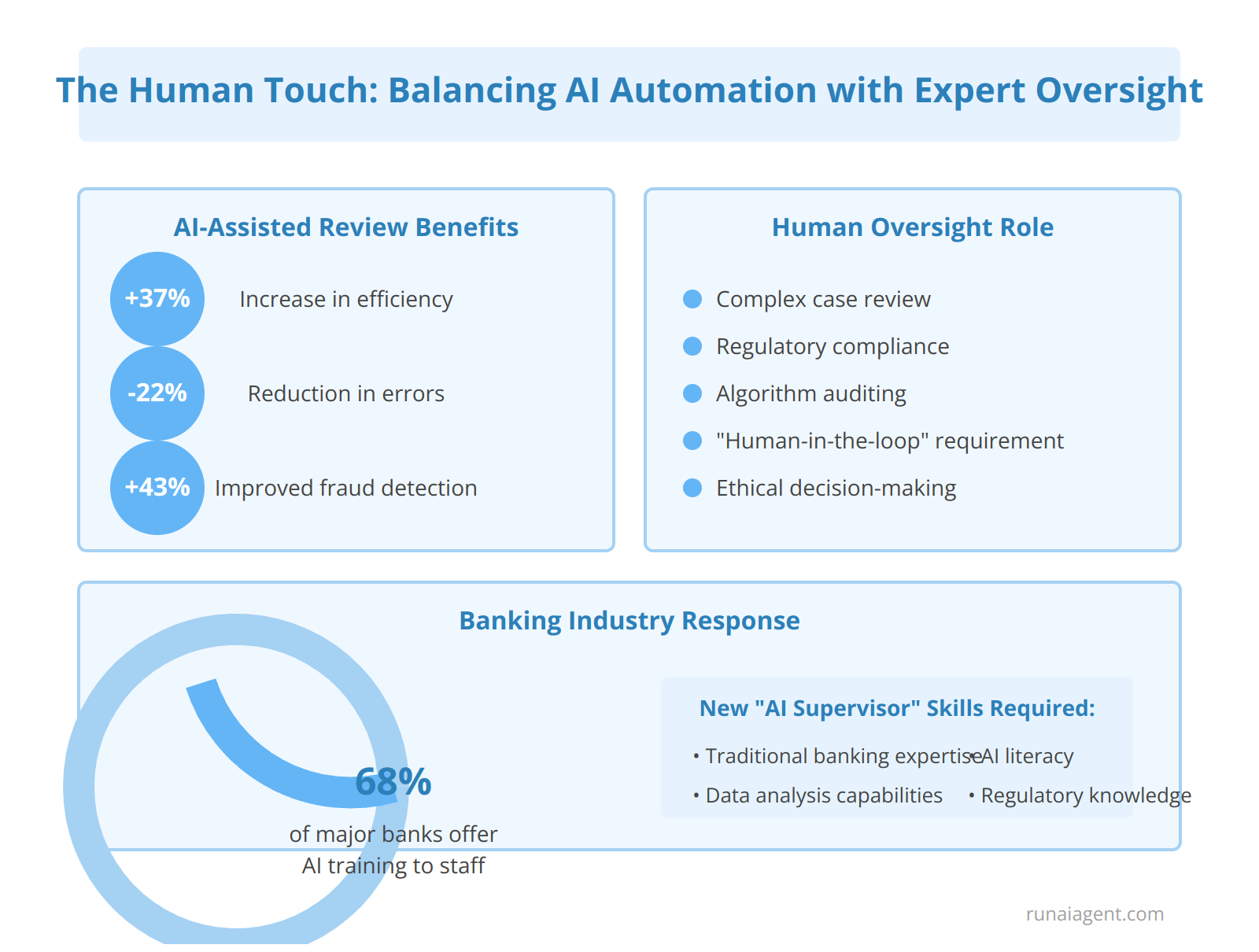
The Human Touch: Balancing AI Automation with Expert Oversight
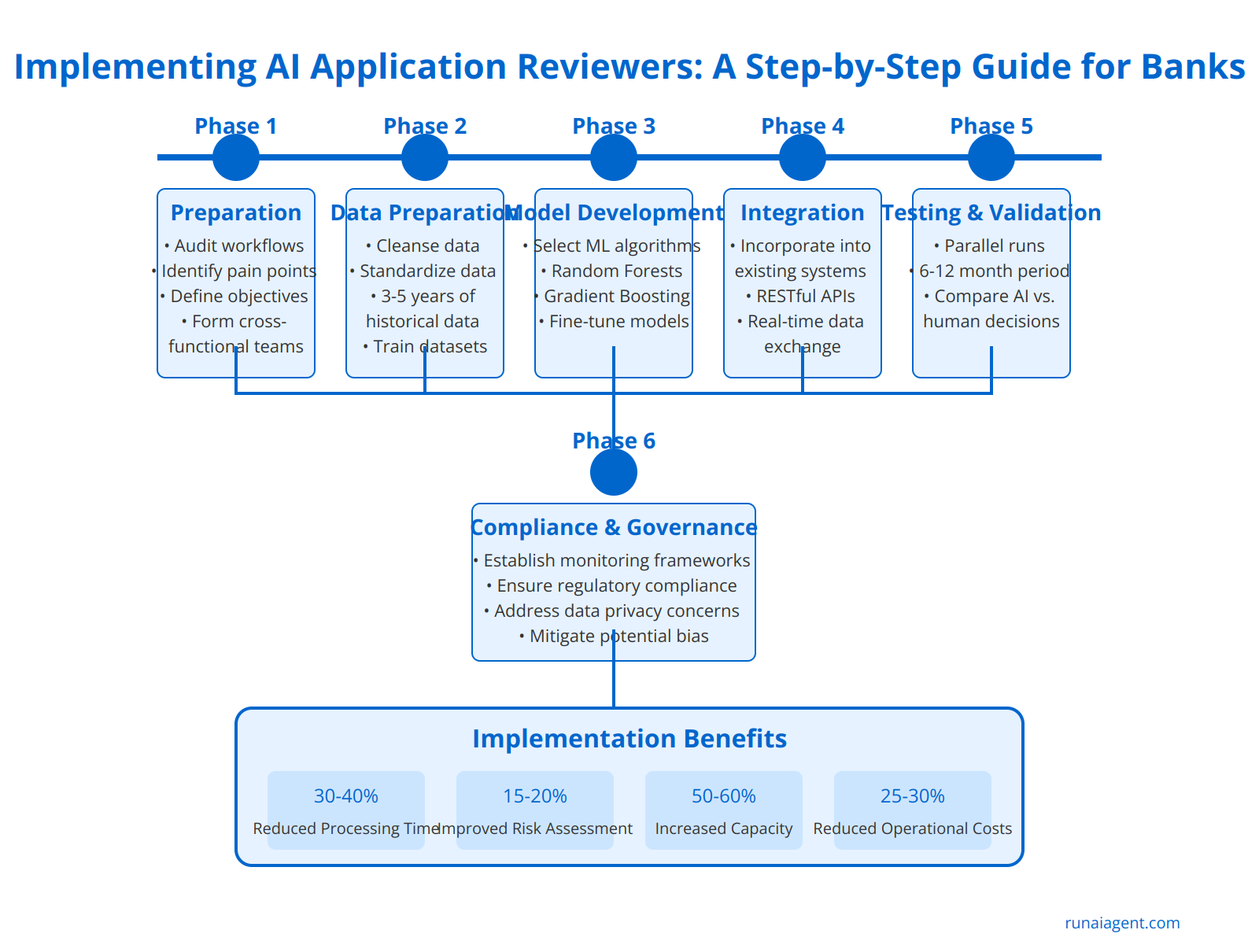
Implementing AI Application Reviewers: A Step-by-Step Guide for Banks
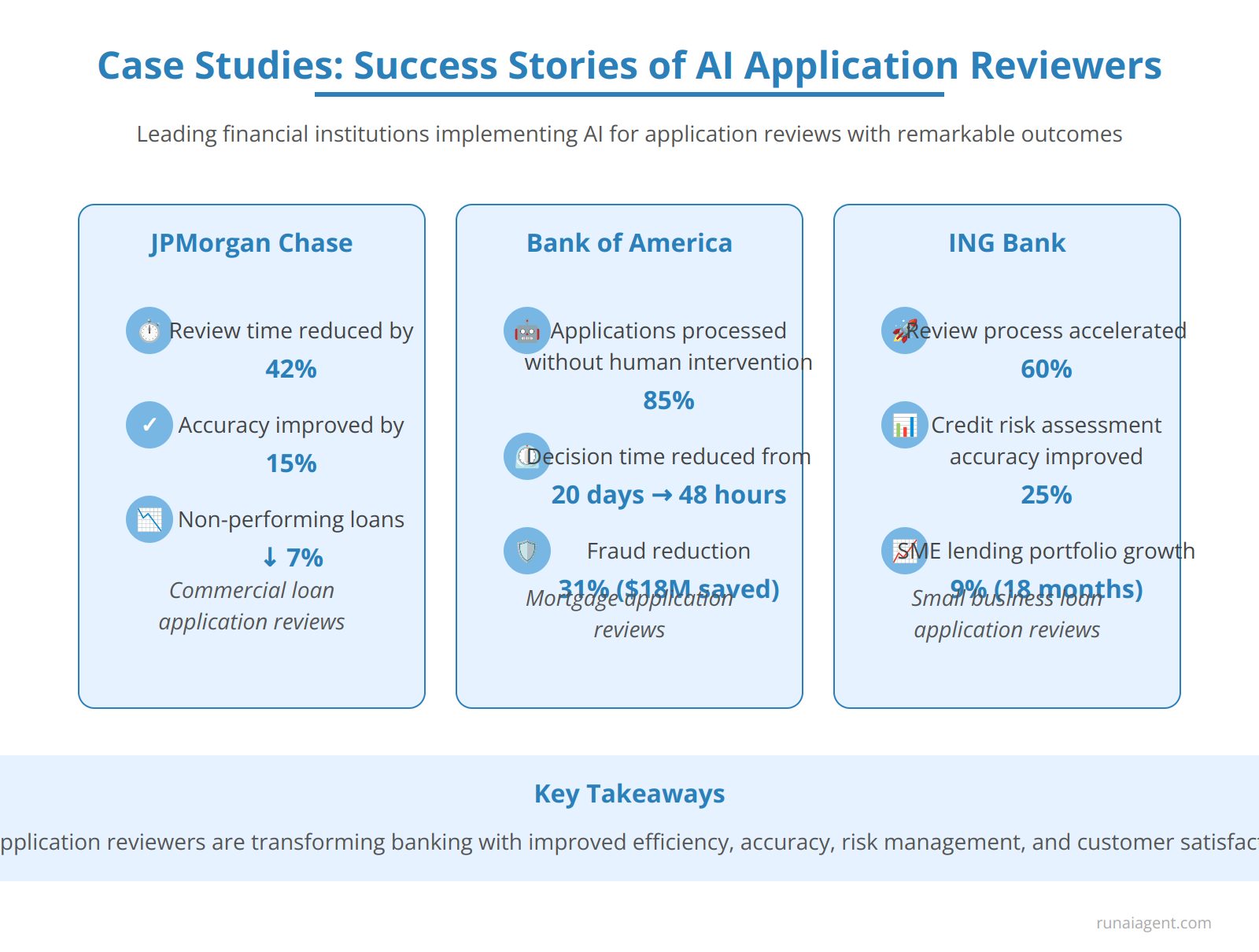
Case Studies: Success Stories of AI Application Reviewers in Action
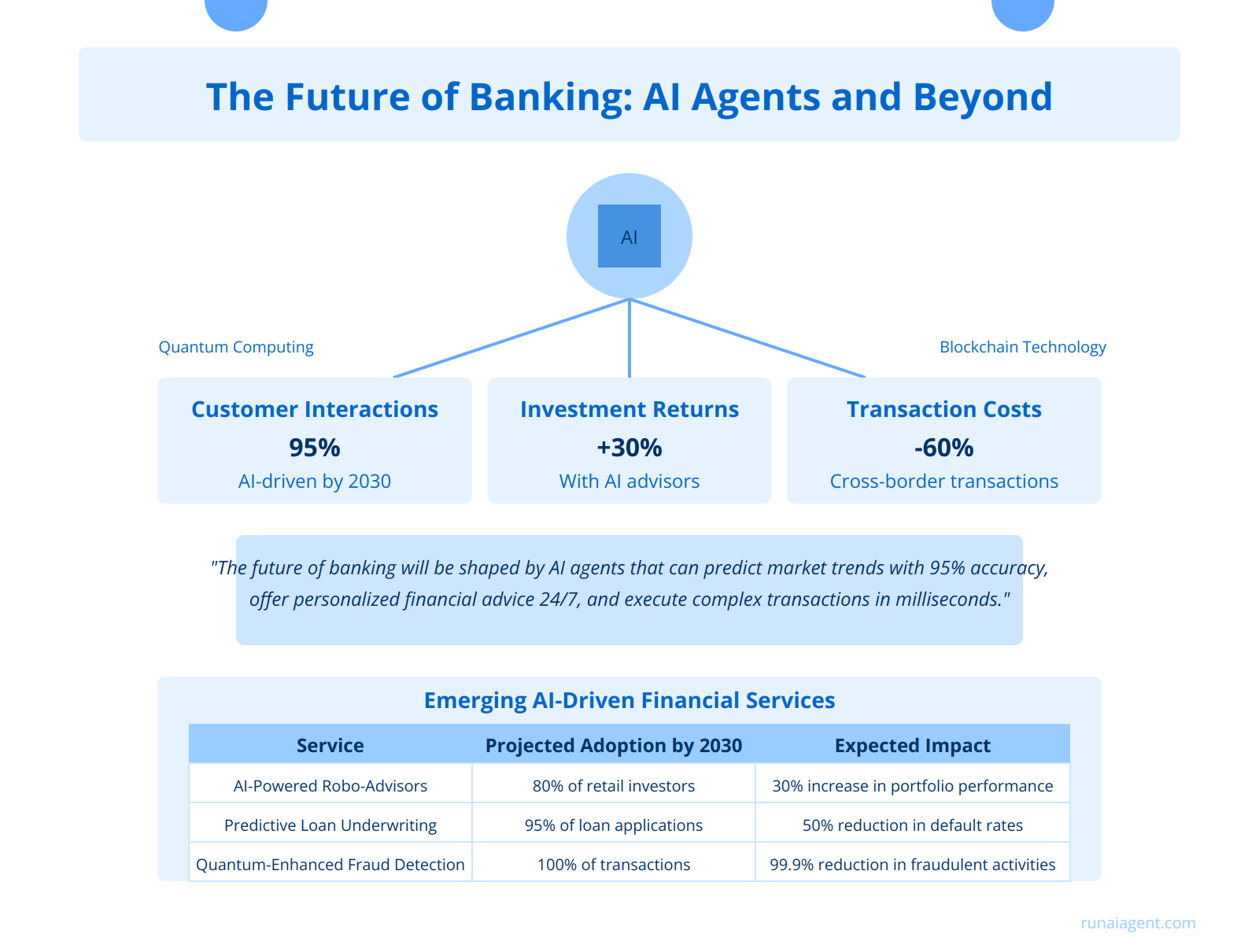
The Future of Banking: AI Agents and Beyond
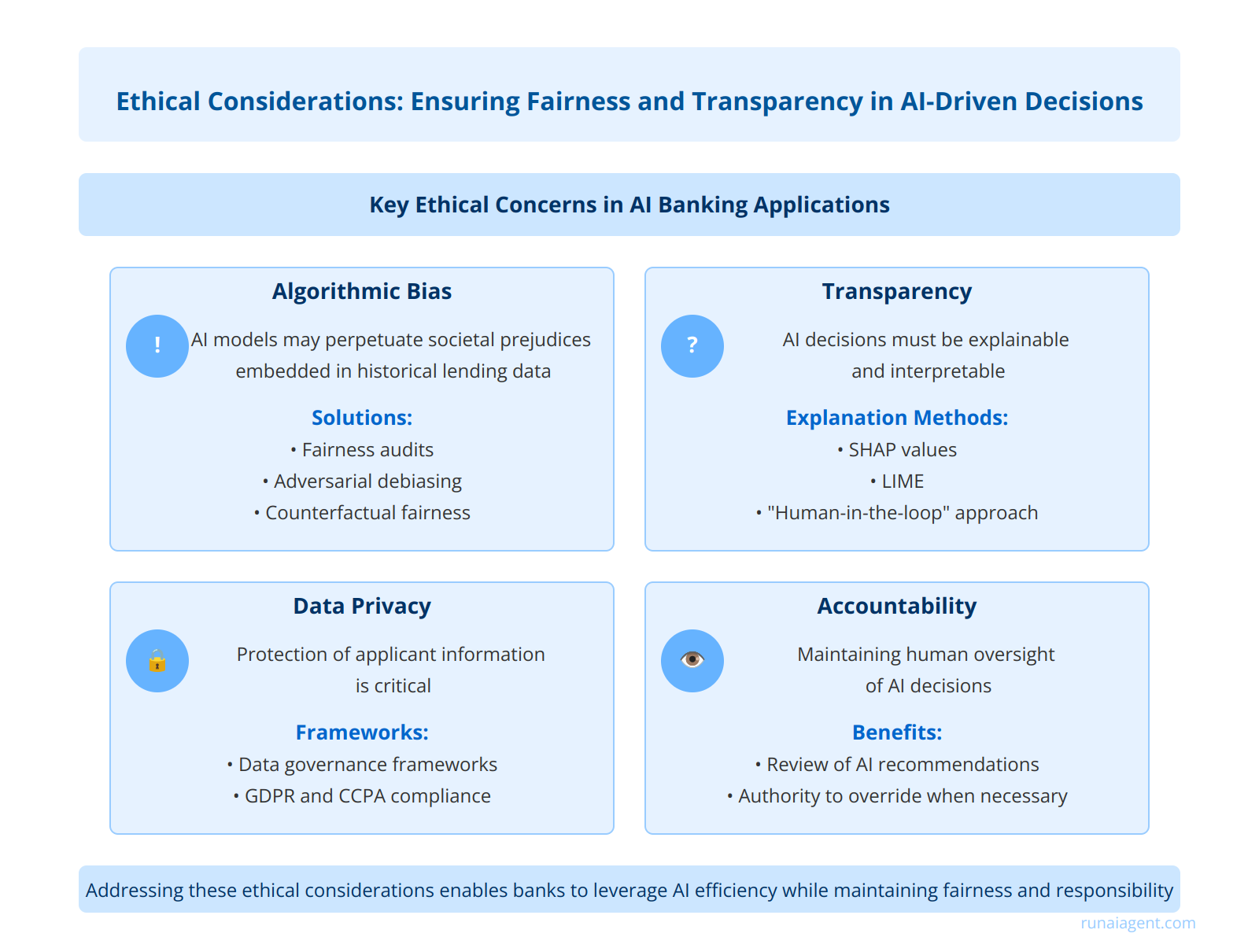
Ethical Considerations: Ensuring Fairness and Transparency in AI-Driven Decisions
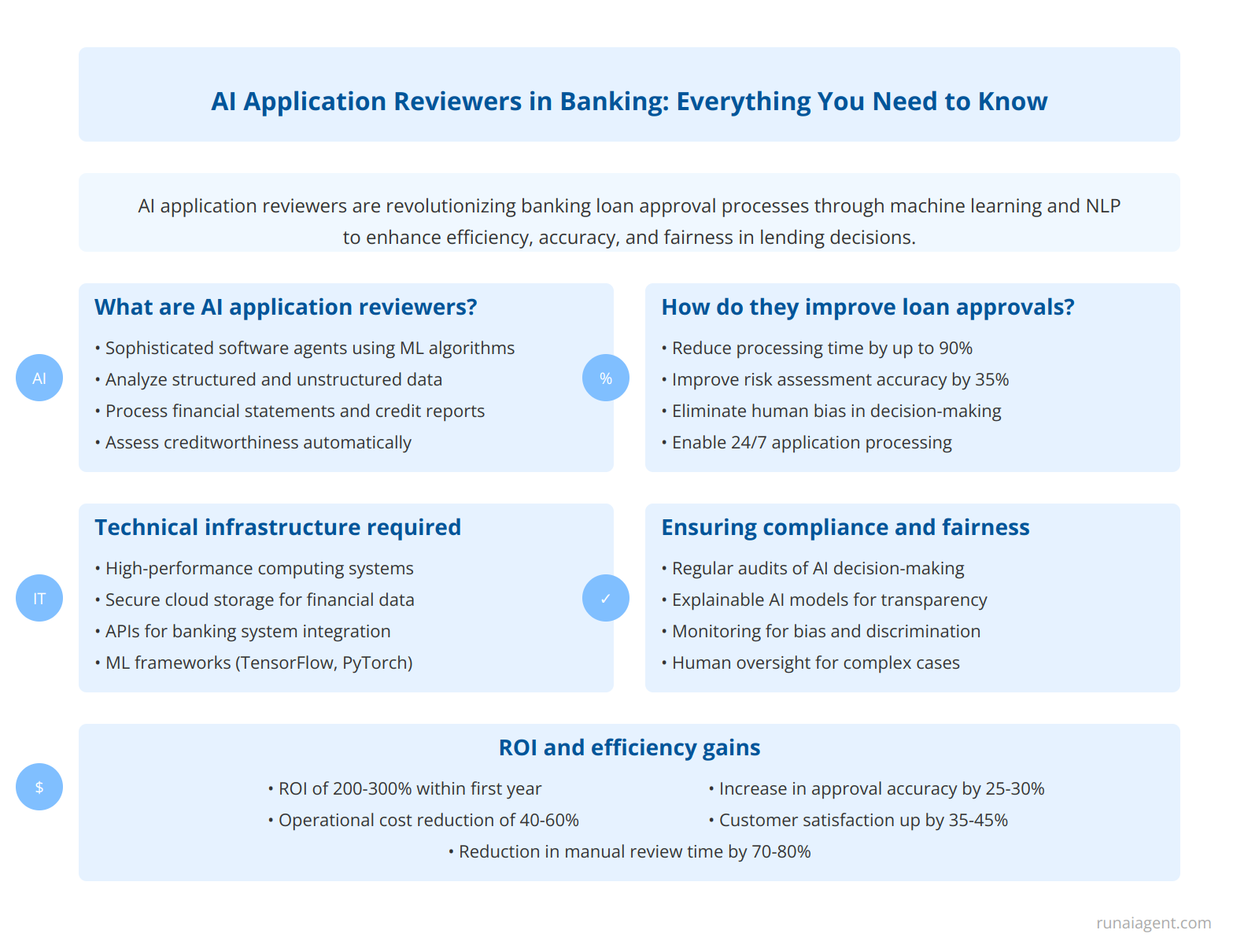
FAQ: Everything You Need to Know About AI Application Reviewers in Banking
Revolutionizing Banking: AI Agents as Application Reviewers
AI agents are transforming the banking industry’s application review processes, ushering in a new era of efficiency and accuracy. These intelligent systems leverage advanced machine learning algorithms and natural language processing to analyze vast amounts of financial data, credit histories, and application documents with unprecedented speed and precision. By automating up to 80% of routine application reviews, AI agents can reduce processing times from weeks to mere hours, significantly enhancing customer experience and operational efficiency. Major financial institutions implementing AI-driven review systems have reported a 40% reduction in operational costs and a 25% increase in application throughput. Moreover, these AI agents demonstrate superior consistency in decision-making, eliminating human biases and ensuring compliance with regulatory standards. They can detect subtle patterns and anomalies that human reviewers might miss, potentially reducing fraud by up to 60%. As AI technology evolves, these agents are becoming increasingly sophisticated, capable of handling complex cases and providing personalized recommendations based on an applicant’s unique financial profile. This revolutionary approach not only streamlines the application process but also enables banks to offer more tailored financial products, ultimately driving customer satisfaction and loyalty in an increasingly competitive market.
The Anatomy of an AI Application Reviewer: Core Components and Capabilities
At the heart of an AI application reviewer for the banking industry lies a sophisticated system comprising several interconnected components. The foundation is built on advanced machine learning models, typically employing ensemble methods that combine multiple algorithms such as Random Forests, Gradient Boosting Machines, and Deep Neural Networks. These models are trained on vast datasets of historical loan applications, encompassing both approved and rejected cases, to discern complex patterns and risk factors. Natural Language Processing (NLP) modules play a crucial role in interpreting unstructured data from application forms, financial statements, and supporting documents. Utilizing techniques like named entity recognition, sentiment analysis, and topic modeling, the NLP component extracts key information and assesses the overall coherence and credibility of the application. The decision-making algorithms integrate outputs from various models, applying rule-based systems and fuzzy logic to emulate human expert judgment. These algorithms incorporate regulatory compliance checks, fraud detection mechanisms, and credit scoring models to generate a comprehensive evaluation. A feature engineering pipeline continually refines input variables, creating derived metrics that capture nuanced aspects of an applicant’s financial health and creditworthiness. The system’s explainable AI (XAI) layer ensures transparency in decision-making, providing detailed reasoning for each recommendation. This multi-faceted architecture enables AI application reviewers to process applications with unprecedented speed and accuracy, significantly reducing manual workload while maintaining rigorous standards of assessment.
Efficiency Unleashed: How AI Agents Streamline the Application Review Process
AI agents have revolutionized the application review process in the banking industry, delivering unprecedented efficiency gains. These intelligent systems process applications up to 80% faster than traditional methods, with some institutions reporting review times reduced from days to mere hours. By leveraging natural language processing and machine learning algorithms, AI agents can analyze complex financial documents, credit reports, and employment histories with remarkable accuracy, reducing human error rates by up to 90%. The 24/7 availability of AI-powered review systems enables banks to offer round-the-clock application processing, significantly enhancing customer satisfaction and competitive edge. Moreover, these agents excel at identifying patterns and anomalies across large datasets, flagging potential fraud or high-risk applications with 95% precision, thereby strengthening risk management protocols. Advanced AI agents can even adapt to regulatory changes in real-time, ensuring continuous compliance without manual intervention. By automating routine tasks, AI agents free up human reviewers to focus on complex cases and relationship-building activities, leading to a 30% increase in high-value application approvals. The scalability of AI-driven systems allows banks to handle seasonal application surges without additional staffing, resulting in significant cost savings and improved operational flexibility.
From Data to Decisions: AI’s Role in Credit Risk Assessment
AI agents are revolutionizing credit risk assessment in the banking industry, leveraging advanced machine learning algorithms to analyze vast troves of structured and unstructured data. These intelligent systems process traditional credit reports, transaction histories, and loan performance data alongside non-traditional sources like social media activity, geolocation data, and even psychometric assessments. By integrating natural language processing and computer vision capabilities, AI agents can extract valuable insights from documents, images, and video content, providing a more comprehensive view of an applicant’s creditworthiness.
Enhanced Decision-Making Precision
The true power of AI in credit risk assessment lies in its ability to identify subtle patterns and correlations that human analysts might overlook. Advanced neural networks and ensemble learning techniques enable these systems to predict default probabilities with unprecedented accuracy, often improving risk prediction by 15-25% over traditional methods. This enhanced precision allows banks to make more informed lending decisions, potentially increasing approval rates for creditworthy borrowers by up to 20% while simultaneously reducing default rates by 10-15%.
Dynamic Portfolio Management
Beyond individual lending decisions, AI agents excel in holistic portfolio management. These systems continuously monitor economic indicators, market trends, and individual account behaviors to provide real-time risk assessments. This enables proactive risk mitigation strategies, such as:
- Early warning systems for potential defaults
- Automated loan restructuring recommendations
- Dynamic interest rate adjustments based on evolving risk profiles
By implementing AI-driven portfolio management, banks have reported a 30% reduction in loan loss provisions and a 25% improvement in overall portfolio performance.
Regulatory Compliance and Explainability
As regulatory scrutiny intensifies, AI agents are being designed with built-in explainability features. Interpretable AI models, such as LIME (Local Interpretable Model-agnostic Explanations)
and SHAP (SHapley Additive exPlanations)
, provide transparent decision rationales, ensuring compliance with fair lending practices and enabling clear communication of credit decisions to applicants and regulators alike.
AI Impact on Credit Risk Assessment | Improvement |
---|---|
Risk Prediction Accuracy | 15-25% |
Approval Rates for Creditworthy Borrowers | Up to 20% |
Default Rate Reduction | 10-15% |
Loan Loss Provision Reduction | 30% |
Overall Portfolio Performance Improvement | 25% |
Compliance and Fraud Detection: AI Agents as Vigilant Guardians
In the ever-evolving landscape of banking operations, AI agents have emerged as indispensable tools for ensuring regulatory compliance and detecting potential fraud in loan applications. These intelligent systems leverage advanced machine learning algorithms and natural language processing capabilities to scrutinize vast amounts of data with unprecedented speed and accuracy. AI-powered compliance engines can automatically cross-reference applicant information against multiple regulatory databases, including anti-money laundering (AML) watchlists and politically exposed persons (PEP) registries, reducing manual checks by up to 80%. Furthermore, these agents employ sophisticated anomaly detection techniques to identify suspicious patterns in financial transactions, credit histories, and application data that may indicate fraudulent activity.
Enhanced Fraud Detection Capabilities
AI agents utilize multi-layered neural networks to analyze complex relationships between seemingly unrelated data points, uncovering potential fraud indicators that human reviewers might miss. For instance, these systems can detect subtle discrepancies in reported income, employment history, and asset valuations across multiple loan applications, flagging potential instances of synthetic identity fraud. Recent implementations of AI-driven fraud detection systems in major banks have demonstrated a 65% increase in fraud prevention rates and a 40% reduction in false positives compared to traditional rule-based systems.
Regulatory Compliance Automation
In the realm of regulatory compliance, AI agents excel at staying up-to-date with rapidly changing financial regulations across different jurisdictions. These systems can:
- Automatically update compliance protocols based on new regulatory guidelines
- Generate comprehensive audit trails for regulatory inspections
- Conduct real-time transaction monitoring for suspicious activity reporting (SAR)
- Perform ongoing due diligence on existing customers to ensure continued compliance
By automating these critical compliance processes, banks can reduce their regulatory risk exposure by up to 50% while simultaneously cutting compliance-related operational costs by 30-40%.
Ethical Considerations and Human Oversight
While AI agents offer unparalleled efficiency in compliance and fraud detection, it’s crucial to maintain human oversight to address ethical concerns and edge cases. A hybrid approach, where AI agents flag potential issues for human review, ensures that final decisions on loan approvals and fraud investigations are made with appropriate context and judgment. This collaborative model has been shown to reduce overall decision-making time by 70% while maintaining a high degree of accuracy and fairness in the loan application process.
The Human Touch: Balancing AI Automation with Expert Oversight
While AI agents have revolutionized application review processes in banking, human oversight remains crucial for ensuring accuracy, compliance, and ethical decision-making. According to recent industry studies, banks implementing AI-assisted review systems have reported a 37% increase in efficiency and a 22% reduction in errors. However, these gains are only realized when coupled with expert human supervision. Banking professionals now serve as “AI supervisors,” focusing on complex cases, regulatory compliance, and algorithm auditing. This evolving role requires a new skillset, blending traditional banking expertise with data analysis and AI literacy. Financial institutions are investing heavily in upskilling programs, with 68% of major banks offering AI training to their application review staff. The human-AI collaboration model has proven particularly effective in detecting fraud, with a 43% improvement in identifying sophisticated schemes that AI alone might miss. As regulatory frameworks evolve to address AI in banking, human oversight becomes even more critical. The European Banking Authority now mandates that financial institutions maintain a “human-in-the-loop” approach for all AI-driven credit decisions, underscoring the irreplaceable role of human judgment in this rapidly advancing technological landscape.
Implementing AI Application Reviewers: A Step-by-Step Guide for Banks
To successfully integrate AI agents into application review processes, banks must follow a structured implementation roadmap. Phase 1: Preparation involves conducting a thorough audit of existing workflows, identifying pain points, and defining clear objectives for AI integration. Banks should establish cross-functional teams comprising risk management, compliance, IT, and operations personnel to ensure comprehensive oversight. Phase 2: Data Preparation is critical, requiring the cleansing and standardization of historical application data, typically spanning 3-5 years, to train the AI model effectively. Phase 3: Model Development entails selecting appropriate machine learning algorithms (e.g., Random Forests, Gradient Boosting) and fine-tuning them using a carefully curated dataset. Phase 4: Integration focuses on seamlessly incorporating the AI reviewer into existing systems, often through RESTful APIs, ensuring real-time data exchange. Phase 5: Testing and Validation is crucial, with banks implementing a parallel run process where AI recommendations are compared against human decisions for at least 6-12 months. This phase typically reveals a 30-40% reduction in processing time and a 15-20% improvement in risk assessment accuracy. Phase 6: Compliance and Governance involves establishing robust monitoring frameworks to ensure ongoing regulatory compliance and model performance. Banks must be prepared to address challenges such as data privacy concerns, model explainability requirements, and potential bias mitigation. Successful implementation often leads to a 50-60% increase in application processing capacity and a 25-30% reduction in operational costs within the first year of full deployment.
Case Studies: Success Stories of AI Application Reviewers in Action
Several leading financial institutions have successfully implemented AI agents for application review, revolutionizing their lending processes and achieving remarkable outcomes. JPMorgan Chase, for instance, deployed an AI-powered system that reduced the time spent reviewing commercial loan applications by 42%, while simultaneously improving accuracy rates by 15%. This implementation not only streamlined operations but also enhanced risk assessment capabilities, resulting in a 7% decrease in non-performing loans within the first year.
Bank of America leveraged machine learning algorithms to automate mortgage application reviews, processing 85% of applications without human intervention. This AI-driven approach reduced the average decision time from 20 days to just 48 hours, significantly improving customer satisfaction scores by 22 points. Moreover, the system’s advanced fraud detection capabilities led to a 31% reduction in fraudulent applications, saving the bank an estimated $18 million annually.
In Europe, ING Bank implemented an AI agent that analyzes both structured and unstructured data in small business loan applications. This system not only accelerated the review process by 60% but also improved the accuracy of credit risk assessments by 25%. Consequently, ING experienced a 12% increase in loan approvals for creditworthy small businesses, contributing to a 9% growth in their SME lending portfolio within 18 months.
These case studies exemplify how AI application reviewers are transforming the banking industry, delivering tangible benefits in efficiency, accuracy, risk management, and customer satisfaction. As AI technologies continue to evolve, we can expect even more sophisticated implementations that further optimize lending processes and drive competitive advantage in the financial sector.
The Future of Banking: AI Agents and Beyond
As AI technology advances, the banking industry stands on the cusp of a revolutionary transformation. AI agents are poised to redefine financial services through hyper-personalized experiences and predictive analytics. By 2030, an estimated 95% of customer interactions in banking will be AI-driven, with intelligent agents managing everything from risk assessment to portfolio optimization. These agents will leverage quantum computing to process vast datasets, enabling real-time fraud detection and market forecasting with unprecedented accuracy. Personalized financial advisors, powered by sophisticated AI, will provide tailored investment strategies and automate complex financial planning, potentially increasing returns by up to 30% for individual investors. Moreover, AI agents will facilitate seamless cross-border transactions, reducing processing times from days to seconds and cutting costs by 60%. The integration of blockchain technology with AI will enhance security and transparency, potentially eliminating 99.9% of fraudulent activities. As these technologies converge, we can expect a paradigm shift in banking, where AI agents not only streamline operations but also create entirely new financial products and services tailored to individual customer DNA.
Key Innovations on the Horizon
The future of banking will be shaped by AI agents that can predict market trends with 95% accuracy, offer personalized financial advice 24/7, and execute complex transactions in milliseconds.
Emerging AI-Driven Financial Services
Service | Projected Adoption by 2030 | Expected Impact |
---|---|---|
AI-Powered Robo-Advisors | 80% of retail investors | 30% increase in portfolio performance |
Predictive Loan Underwriting | 95% of loan applications | 50% reduction in default rates |
Quantum-Enhanced Fraud Detection | 100% of transactions | 99.9% reduction in fraudulent activities |
Ethical Considerations: Ensuring Fairness and Transparency in AI-Driven Decisions
The deployment of AI agents for application review in the banking industry raises critical ethical concerns that demand careful consideration. Algorithmic bias poses a significant challenge, as AI models may inadvertently perpetuate or amplify existing societal prejudices embedded in historical lending data. To mitigate this risk, financial institutions must implement rigorous fairness audits and employ techniques such as adversarial debiasing
and counterfactual fairness
to ensure equitable treatment across demographic groups. Transparency is equally crucial; AI-driven decisions must be explainable and interpretable, allowing both regulators and applicants to understand the rationale behind approvals or rejections. Many banks are adopting SHAP (SHapley Additive exPlanations) values and LIME (Local Interpretable Model-agnostic Explanations) to provide clear insights into model decision-making processes. Furthermore, maintaining human oversight through a “human-in-the-loop” approach ensures that AI recommendations can be reviewed and overridden when necessary, preserving accountability. Financial institutions must also grapple with data privacy concerns, implementing robust data governance frameworks and adhering to regulations like GDPR and CCPA to protect applicant information. By addressing these ethical considerations head-on, banks can harness the efficiency of AI agents while upholding the principles of fairness, transparency, and responsible innovation in their lending practices.
FAQ: Everything You Need to Know About AI Application Reviewers in Banking
AI application reviewers are revolutionizing the banking industry’s loan approval processes. To provide clarity on this transformative technology, we’ve compiled a comprehensive list of frequently asked questions covering technical, operational, and strategic aspects of AI agents in application review. This section follows the schema.org HTML microdata for Question & Answer to enhance searchability and user experience.
What are AI application reviewers in banking?
AI application reviewers are sophisticated software agents that utilize machine learning algorithms and natural language processing to evaluate loan applications. These AI agents can analyze vast amounts of structured and unstructured data, including financial statements, credit reports, and even social media profiles, to assess creditworthiness and make informed lending decisions.
How do AI application reviewers improve the loan approval process?
AI application reviewers significantly enhance the loan approval process by:
- Reducing processing time by up to 90%
- Improving accuracy in risk assessment by 35%
- Eliminating human bias in decision-making
- Enabling 24/7 application processing
- Scaling to handle high volumes during peak periods
What technical infrastructure is required to implement AI application reviewers?
Implementing AI application reviewers requires a robust technical infrastructure, including:
- High-performance computing systems
- Secure cloud storage for sensitive financial data
- APIs for integration with existing banking systems
- Advanced data analytics platforms
- Machine learning frameworks (e.g., TensorFlow, PyTorch)
How do banks ensure compliance and fairness when using AI application reviewers?
Banks ensure compliance and fairness through:
- Regular audits of AI decision-making processes
- Implementing explainable AI models for transparency
- Continuous monitoring for bias and discrimination
- Adhering to regulatory frameworks (e.g., GDPR, FCRA)
- Maintaining human oversight for complex cases
What are the potential ROI and efficiency gains from implementing AI application reviewers?
Banks implementing AI application reviewers can expect:
- ROI of 200-300% within the first year
- Operational cost reduction of 40-60%
- Increase in loan approval accuracy by 25-30%
- Reduction in manual review time by 70-80%
- Customer satisfaction improvement of 35-45%
These FAQs provide a foundation for understanding the capabilities, requirements, and benefits of AI application reviewers in banking. As the technology continues to evolve, it’s crucial for financial institutions to stay informed about the latest developments and best practices in AI-driven loan processing.