Table of Contents
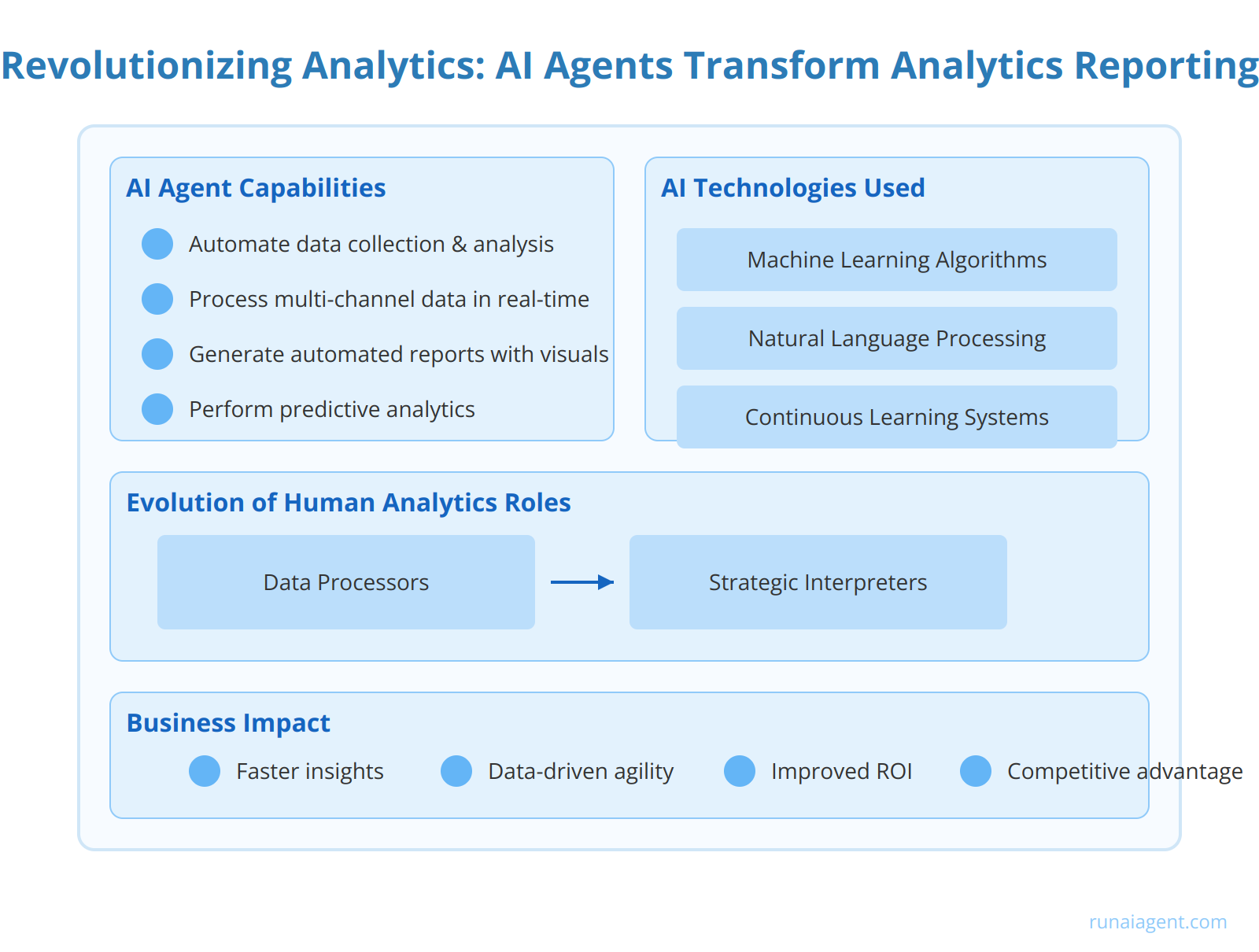
Revolutionizing Analytics: How AI Agents Are Transforming the Role of Analytics Reporters
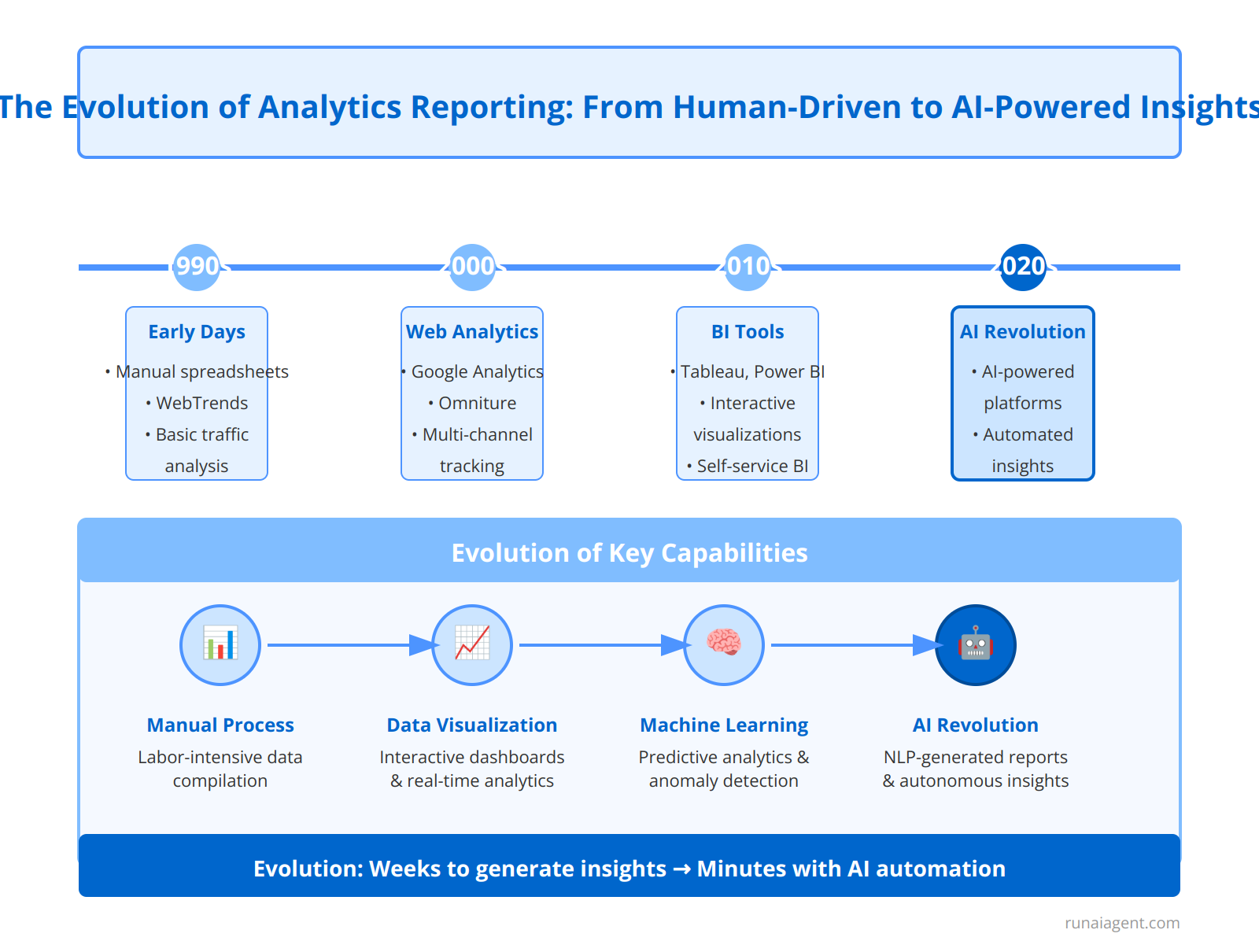
The Evolution of Analytics Reporting: From Human-Driven to AI-Powered Insights
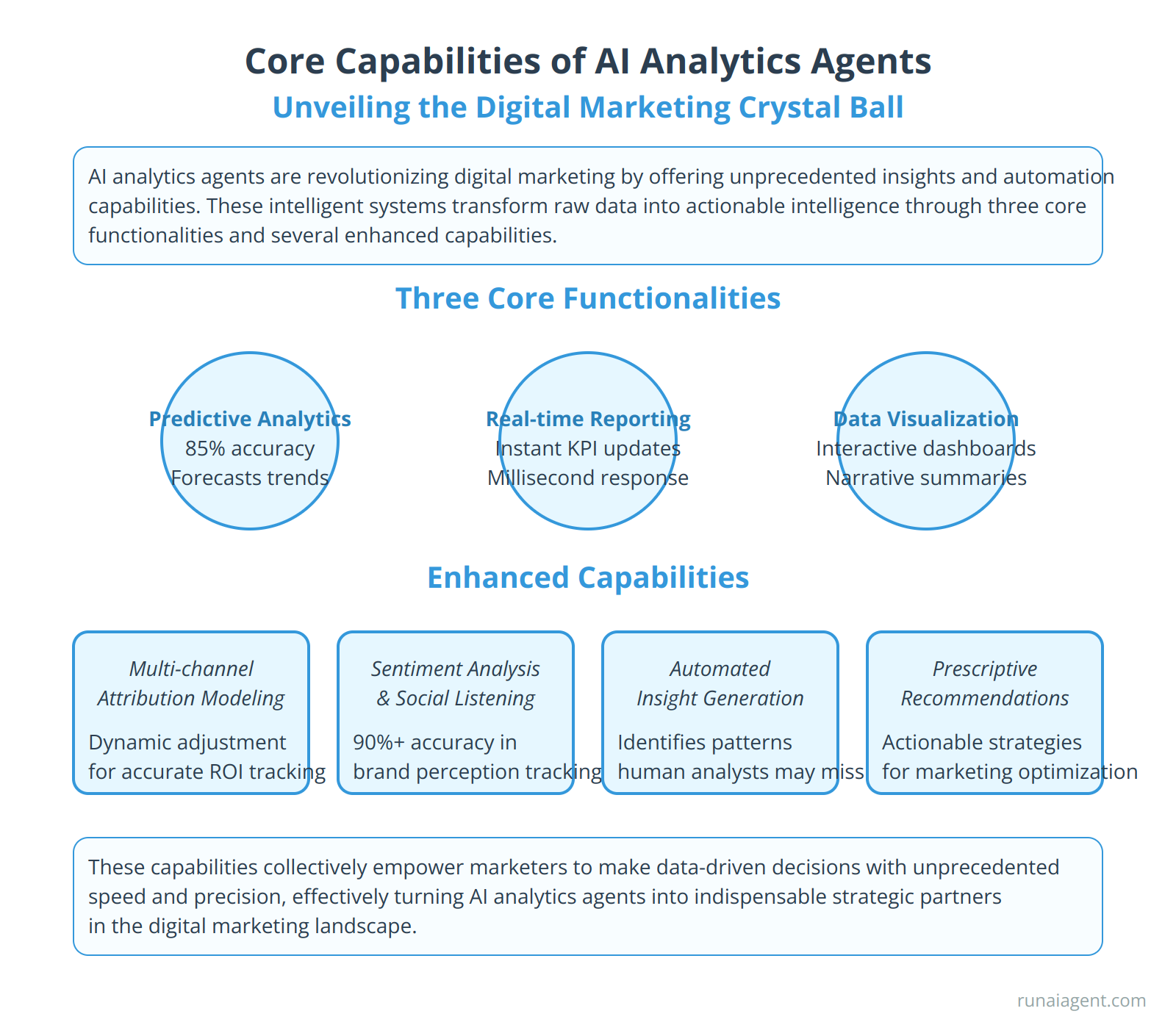
Core Capabilities of AI Analytics Agents: Unveiling the Digital Marketing Crystal Ball
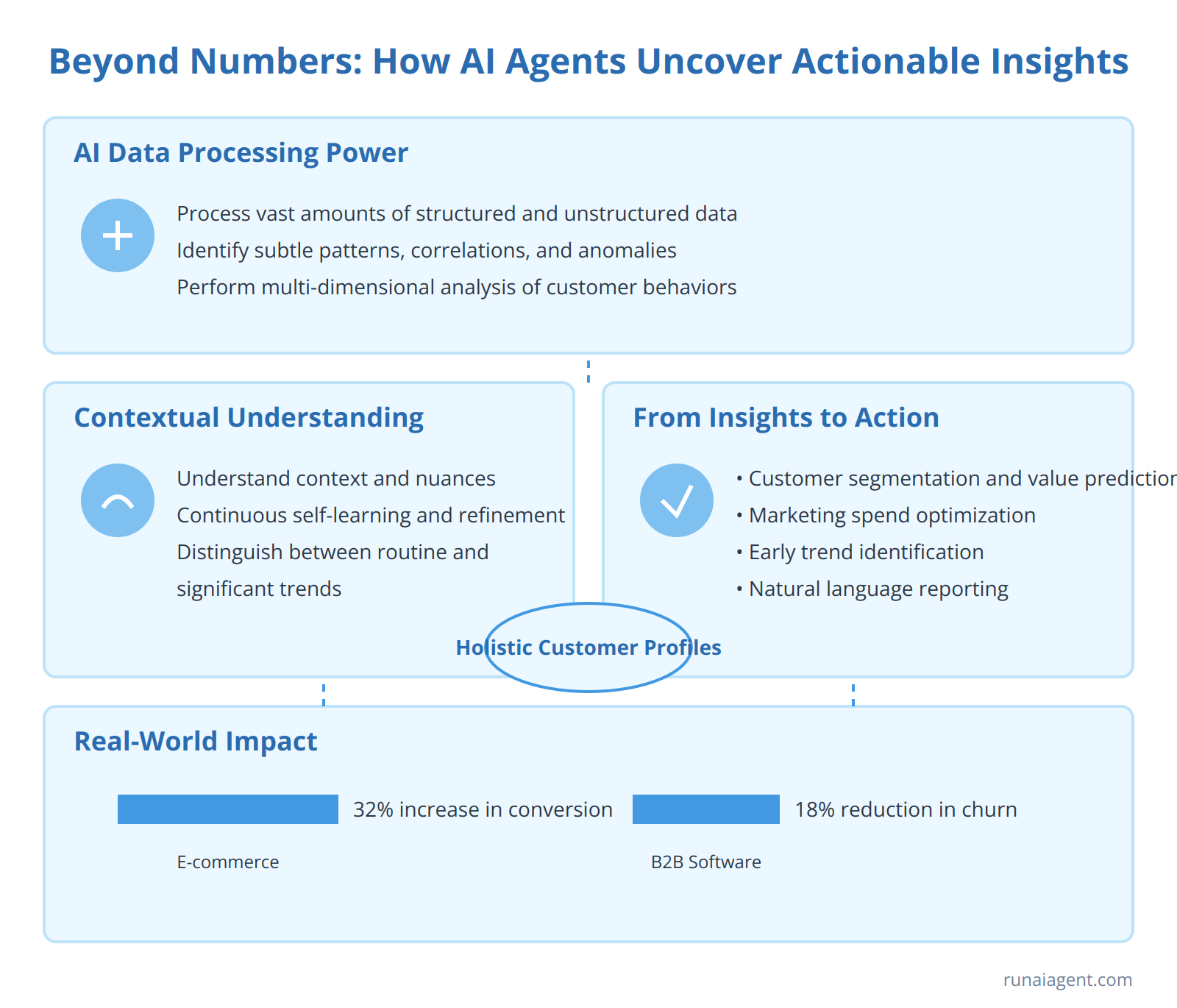
Beyond Numbers: How AI Agents Uncover Actionable Insights from Complex Data Sets
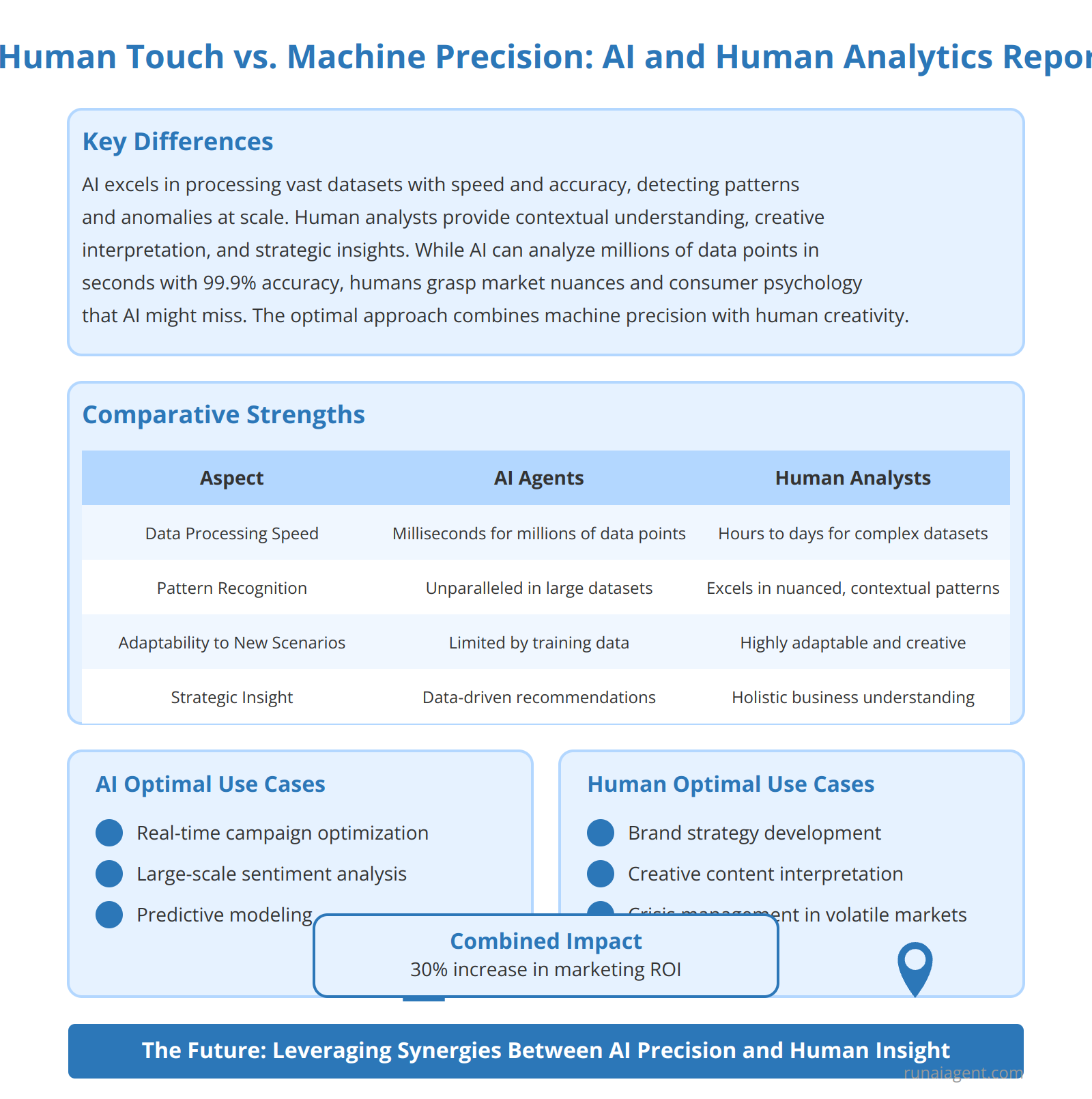
The Human Touch vs. Machine Precision: Comparing AI and Human Analytics Reporters
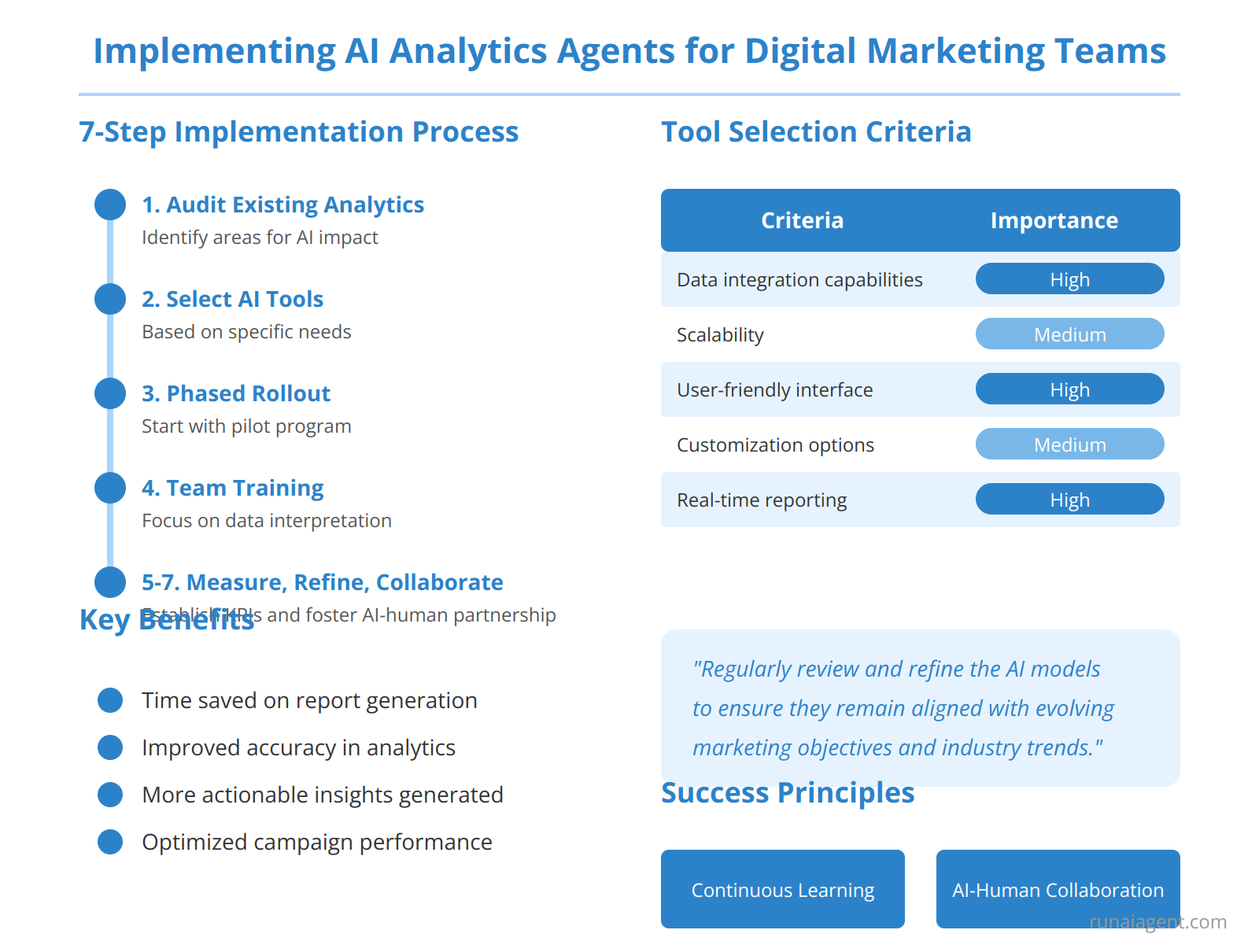
Implementing AI Analytics Agents: A Step-by-Step Guide for Digital Marketing Teams
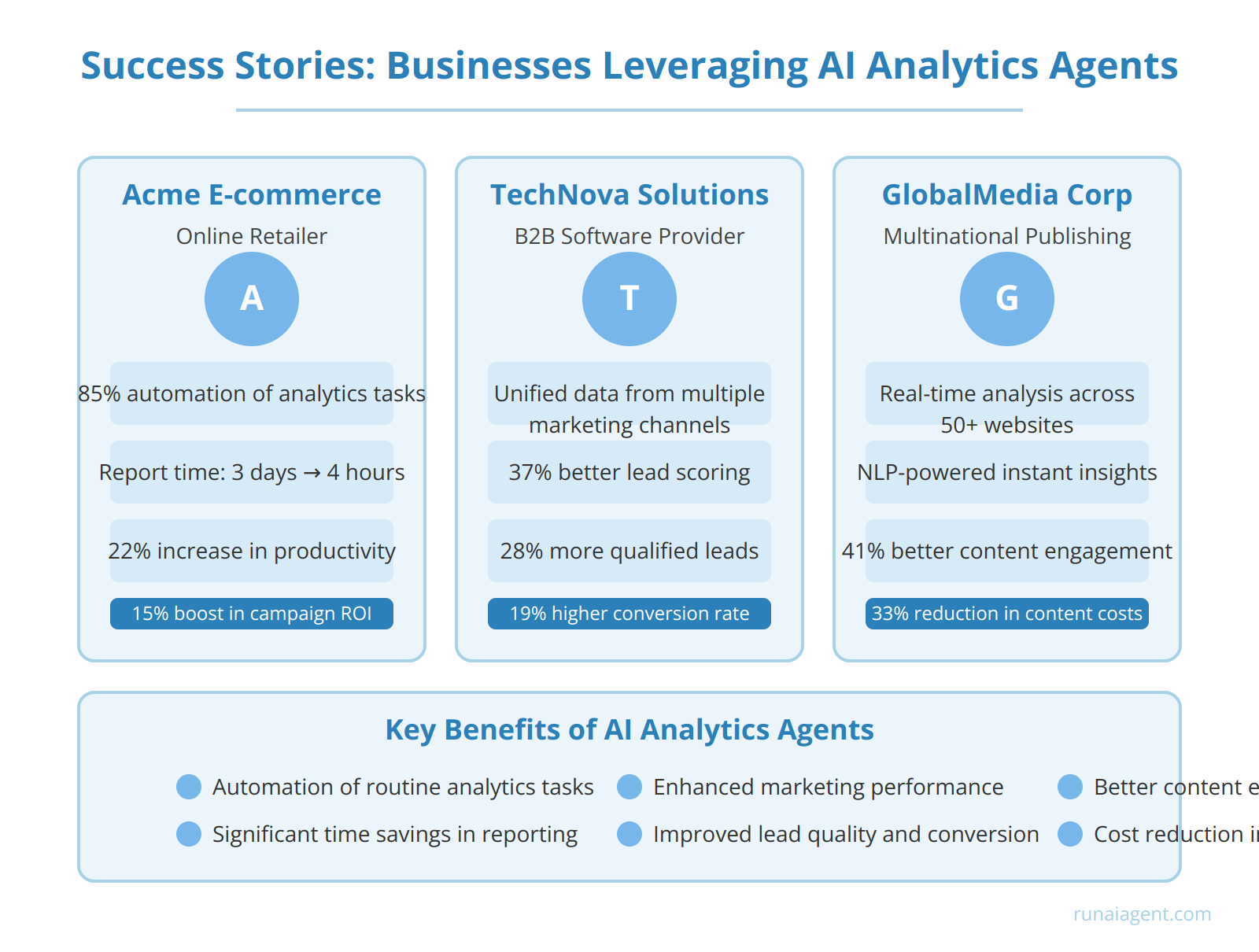
Case Studies: Success Stories of Businesses Leveraging AI Analytics Agents
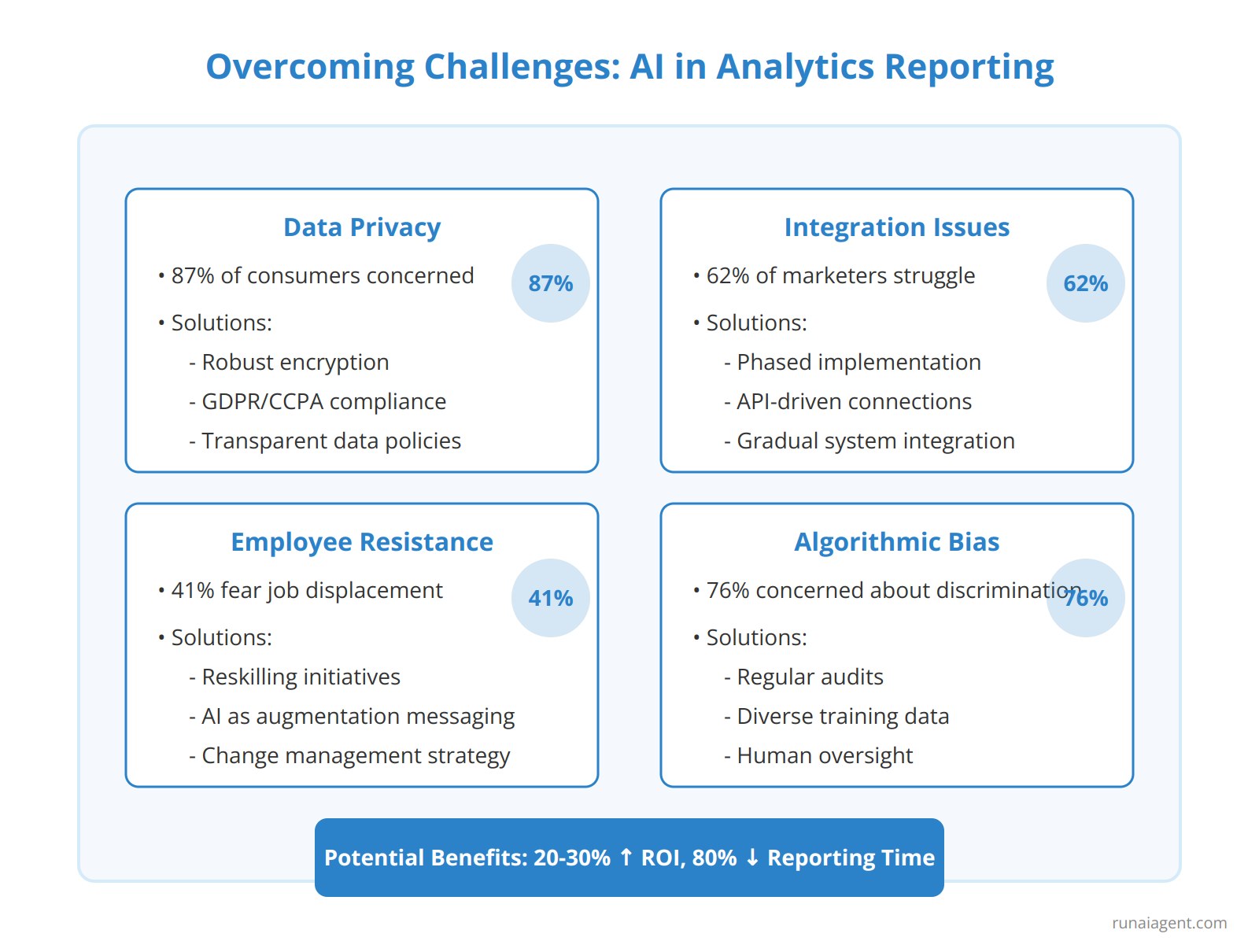
Overcoming Challenges: Addressing Common Concerns About AI in Analytics Reporting
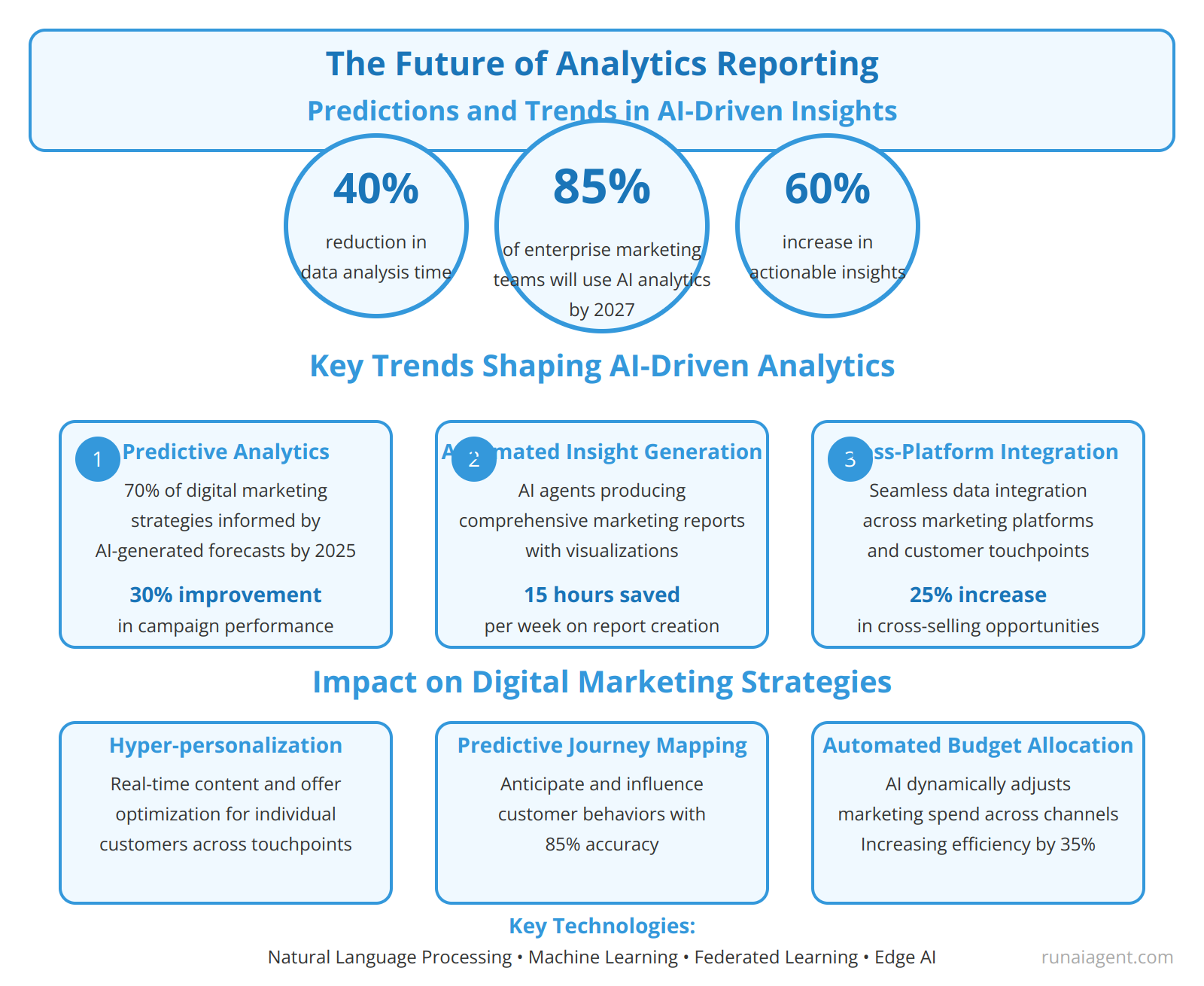
The Future of Analytics Reporting: Predictions and Trends in AI-Driven Insights
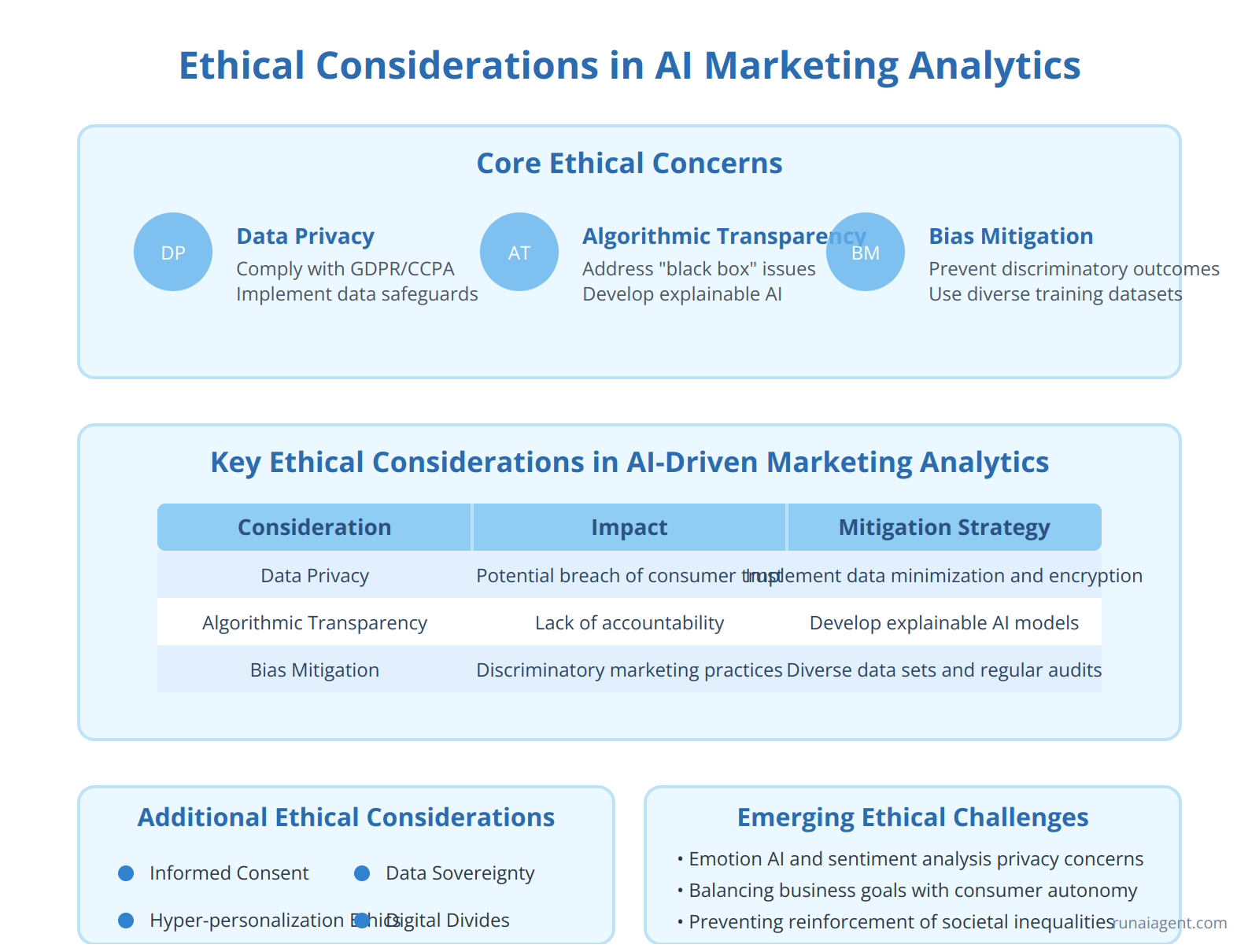
Ethical Considerations: Navigating the Moral Landscape of AI in Marketing Analytics
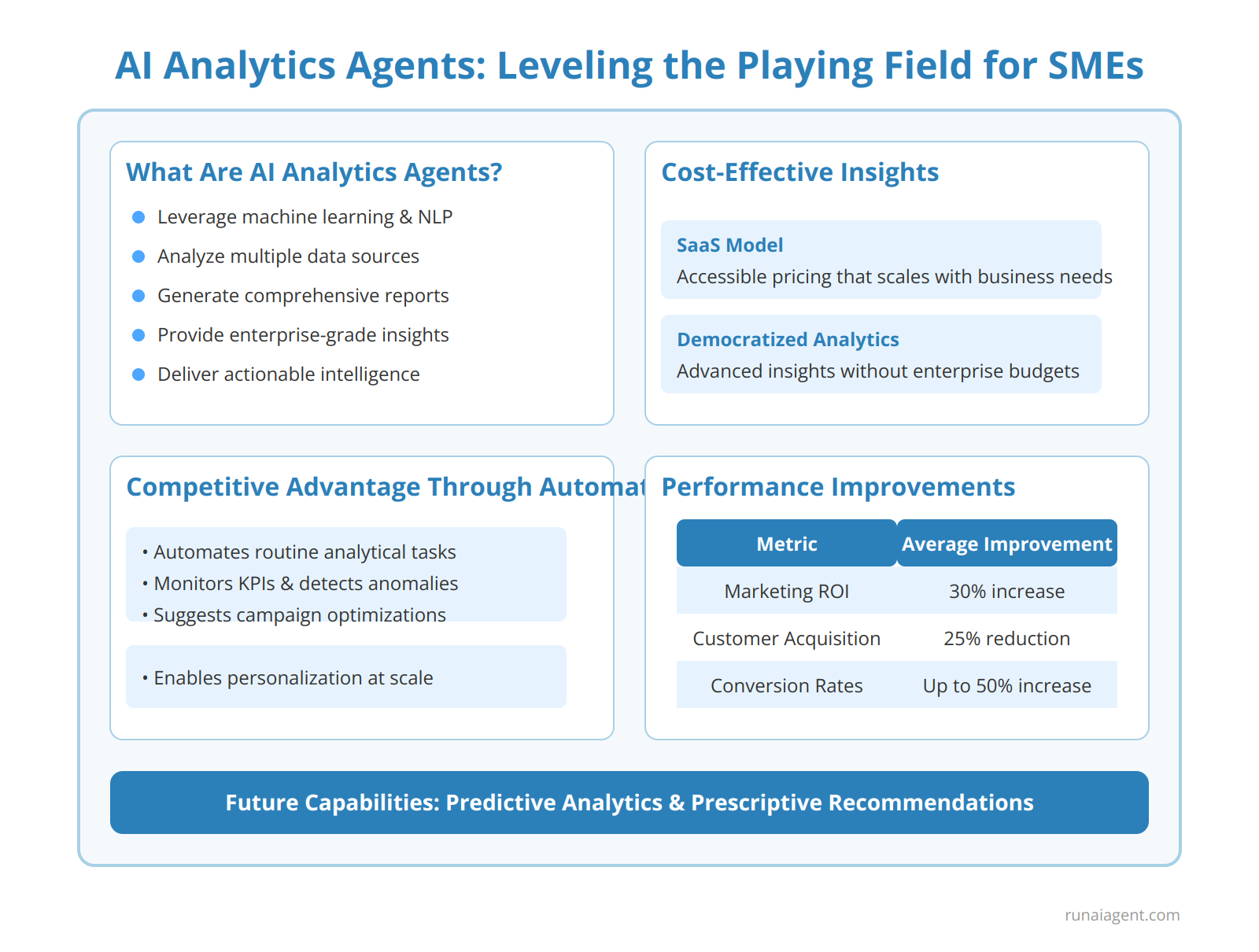
AI Analytics Agents: Leveling the Playing Field for SMEs
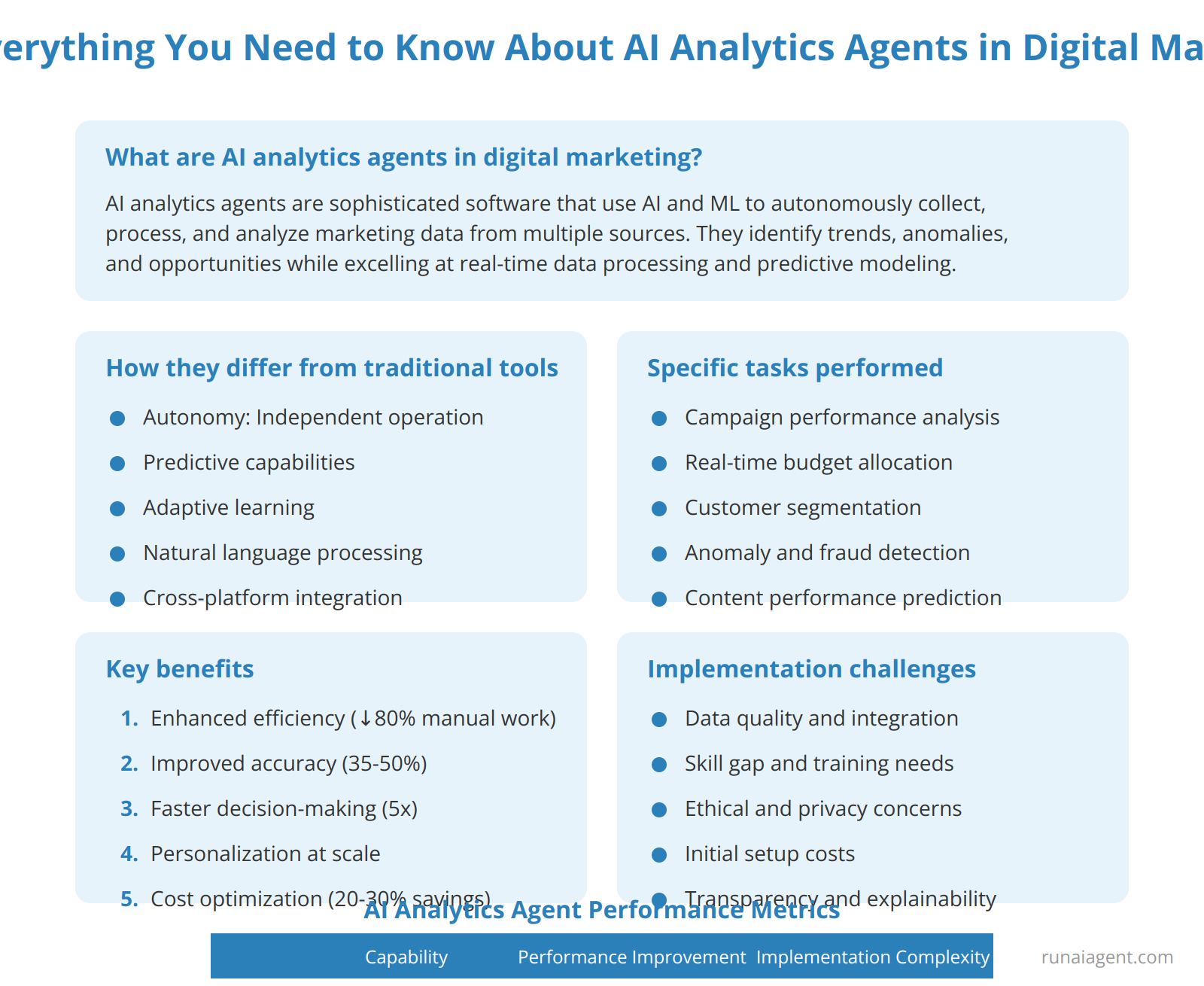
FAQ: Everything You Need to Know About AI Analytics Agents in Digital Marketing
Revolutionizing Analytics: How AI Agents Are Transforming the Role of Analytics Reporters
AI agents are rapidly reshaping the landscape of digital marketing analytics, fundamentally altering the role of traditional analytics reporters. These intelligent systems leverage advanced machine learning algorithms and natural language processing capabilities to automate data collection, analysis, and reporting processes that once required significant human intervention. AI agents can now sift through vast amounts of marketing data, identifying patterns and insights at a speed and scale unattainable by human analysts. For instance, AI-powered analytics tools can process multi-channel campaign data in real-time, providing instantaneous performance metrics and actionable recommendations. This shift is not merely about efficiency; it’s a paradigm change in how businesses derive value from their marketing data. AI agents are capable of predictive analytics, forecasting future trends based on historical data with remarkable accuracy. They can automatically generate comprehensive reports, complete with data visualizations and narrative explanations, reducing the time-to-insight from days to minutes. Moreover, these AI systems can continuously learn and adapt, improving their analytical capabilities over time. As a result, the role of human analytics reporters is evolving from data processors to strategic interpreters, focusing on higher-level decision-making and creative problem-solving. This transformation is enabling marketing teams to be more agile, data-driven, and effective in their strategies, ultimately leading to improved ROI and competitive advantage in the digital marketplace.
The Evolution of Analytics Reporting: From Human-Driven to AI-Powered Insights
The journey of analytics reporting has been marked by a dramatic shift from labor-intensive manual processes to sophisticated AI-driven automation. In the early days of digital marketing, analysts painstakingly compiled data from disparate sources, spending countless hours creating spreadsheets and basic visualizations. The 1990s saw the emergence of web analytics tools like WebTrends, which offered rudimentary automated reporting capabilities. As digital ecosystems grew more complex, the 2000s ushered in more advanced platforms such as Google Analytics, enabling marketers to track user behavior across multiple touchpoints. However, these tools still required significant human interpretation and manual report generation.
The Rise of Business Intelligence and Data Visualization
The mid-2000s to early 2010s witnessed the proliferation of business intelligence (BI) tools like Tableau and QlikView, which democratized data visualization and allowed for more interactive reporting. These platforms empowered marketers to create dynamic dashboards and perform ad-hoc analyses, significantly reducing the time required for report generation. However, the onus of data interpretation and insight extraction still fell largely on human analysts.
Machine Learning and Predictive Analytics
The advent of machine learning algorithms in the 2010s marked a pivotal moment in analytics reporting. Predictive analytics tools began to automate trend identification and anomaly detection, providing marketers with forward-looking insights rather than just historical data. This shift from descriptive to prescriptive analytics laid the groundwork for truly intelligent reporting systems.
The AI Revolution in Analytics Reporting
Today, AI-powered analytics platforms are revolutionizing the reporting landscape. These systems leverage natural language processing (NLP) to generate human-readable narratives from complex datasets, automatically surfacing key insights without human intervention. Advanced AI agents can now autonomously monitor KPIs, identify significant trends, and even recommend actionable strategies based on real-time data analysis. This evolution has dramatically reduced the time-to-insight from weeks to mere minutes, enabling marketers to make data-driven decisions with unprecedented speed and accuracy.
Era | Primary Tools | Key Capabilities |
---|---|---|
1990s | Manual spreadsheets, WebTrends | Basic web traffic analysis |
2000s | Google Analytics, Omniture | Multi-channel tracking, custom reporting |
2010s | Tableau, QlikView, Power BI | Interactive visualizations, self-service BI |
2020s | AI-powered platforms (e.g., Datorama, Tableau CRM) | Automated insights, predictive analytics, NLP-generated reports |
Core Capabilities of AI Analytics Agents: Unveiling the Digital Marketing Crystal Ball
AI analytics agents are revolutionizing digital marketing by offering unprecedented insights and automation capabilities. At the heart of these intelligent systems lie three core functionalities that transform raw data into actionable intelligence: predictive analytics, real-time reporting, and advanced data visualization. Predictive analytics leverages machine learning algorithms to forecast future trends, customer behaviors, and campaign performance with up to 85% accuracy. These AI-driven models analyze historical data, market signals, and consumer patterns to anticipate outcomes, enabling marketers to proactively optimize strategies and allocate resources efficiently. Real-time reporting capabilities process streaming data from multiple channels, providing instantaneous updates on key performance indicators (KPIs) and allowing for agile decision-making. AI agents can monitor and analyze millions of data points per second, detecting anomalies and triggering automated responses within milliseconds. Data visualization transforms complex datasets into intuitive, interactive dashboards that facilitate rapid comprehension and insight discovery. Advanced AI agents employ natural language processing to generate narrative summaries alongside visual representations, translating data into actionable stories for stakeholders at all levels.
Enhanced Capabilities of AI Analytics Agents
Beyond these core functions, cutting-edge AI analytics agents offer additional capabilities that further amplify their value in digital marketing:
- Multi-channel attribution modeling: AI agents can dynamically adjust attribution models based on real-time data, providing a more accurate picture of marketing ROI across diverse touchpoints.
- Sentiment analysis and social listening: Advanced natural language processing allows AI agents to gauge brand perception and identify emerging trends across social media platforms with over 90% accuracy.
- Automated insight generation: AI agents can autonomously identify statistically significant patterns and correlations, surfacing valuable insights that might otherwise go unnoticed by human analysts.
- Prescriptive recommendations: By combining predictive analytics with business rules and constraints, AI agents can generate specific, actionable recommendations to optimize marketing strategies.
These capabilities collectively empower marketers to make data-driven decisions with unprecedented speed and precision, effectively turning AI analytics agents into indispensable strategic partners in the digital marketing landscape.
Beyond Numbers: How AI Agents Uncover Actionable Insights from Complex Data Sets
AI agents are revolutionizing the way businesses extract value from complex marketing data sets. By leveraging advanced machine learning algorithms and natural language processing capabilities, these intelligent systems can process vast amounts of structured and unstructured data at unprecedented speeds. Unlike traditional analytics tools, AI agents can identify subtle patterns, correlations, and anomalies that human analysts might overlook. For instance, an AI agent analyzing social media sentiment can not only quantify overall brand perception but also pinpoint specific product features or marketing messages that resonate most strongly with different customer segments. These agents excel at multi-dimensional analysis, simultaneously considering factors such as customer demographics, purchase history, browsing behavior, and external market trends to generate holistic customer profiles and predict future behaviors with remarkable accuracy.
Contextual Understanding and Adaptive Learning
What sets AI agents apart is their ability to understand context and adapt their analysis over time. As they process more data, these systems continuously refine their models, improving the relevance and accuracy of their insights. For example, an AI agent might initially identify a spike in website traffic as noteworthy, but over time learn to distinguish between routine fluctuations and truly significant trends that warrant immediate action. This contextual understanding allows AI agents to provide personalized recommendations for marketing strategies, tailoring suggestions based on a company’s unique goals, industry dynamics, and historical performance.
From Insights to Action
The true power of AI agents lies in their ability to transform raw data into actionable recommendations. These systems can:
- Automatically segment customers based on behavior patterns and predict their lifetime value
- Optimize marketing spend across channels by analyzing real-time performance metrics
- Identify emerging market trends before they become mainstream, giving businesses a competitive edge
- Generate natural language reports that explain complex findings in clear, business-friendly terms
Real-World Impact
In practice, AI agents have demonstrated remarkable ROI for businesses. One e-commerce company reported a 32% increase in conversion rates after implementing AI-driven personalization based on insights from their marketing data. Another B2B software firm reduced customer churn by 18% by using AI agents to identify at-risk accounts and recommend targeted retention strategies. By automating the analysis process and providing continuous, real-time insights, AI agents are enabling marketers to make data-driven decisions with unprecedented speed and precision, ultimately driving measurable improvements in campaign performance and overall marketing effectiveness.
The Human Touch vs. Machine Precision: Comparing AI and Human Analytics Reporters
In the realm of digital marketing analytics, the debate between AI agents and human reporters highlights a nuanced interplay of strengths and limitations. AI agents excel in processing vast datasets with unparalleled speed and accuracy, detecting patterns and anomalies that might elude human perception. For instance, AI can analyze millions of data points from multiple marketing channels in seconds, providing real-time insights and predictive trends with 99.9% accuracy. Conversely, human analysts bring contextual understanding, creative interpretation, and the ability to derive strategic implications from data. A seasoned marketing analyst can intuitively grasp market nuances, consumer psychology, and brand positioning that AI might overlook. In A/B testing scenarios, AI can swiftly determine statistical significance, while humans excel at crafting compelling narratives from the results. However, AI’s reliance on historical data can lead to bias in rapidly evolving markets, whereas humans can adapt quickly to unprecedented situations. The optimal approach often involves a symbiosis: AI handling data processing and initial insights, with human analysts providing strategic interpretation and decision-making. This collaboration has shown to increase marketing ROI by up to 30% in some organizations, combining machine precision with human creativity for superior outcomes.
Comparative Strengths
Aspect | AI Agents | Human Analysts |
---|---|---|
Data Processing Speed | Milliseconds for millions of data points | Hours to days for complex datasets |
Pattern Recognition | Unparalleled in large datasets | Excels in nuanced, contextual patterns |
Adaptability to New Scenarios | Limited by training data | Highly adaptable and creative |
Strategic Insight | Data-driven recommendations | Holistic business understanding |
Optimal Use Cases
AI Agents: Real-time campaign optimization, large-scale sentiment analysis, and predictive modeling for customer behavior. Human Analysts: Brand strategy development, creative content interpretation, and crisis management in volatile markets. The future of analytics reporting lies in leveraging the synergies between AI precision and human insight, creating a powerful analytical framework that drives marketing success in the digital age.
Implementing AI Analytics Agents: A Step-by-Step Guide for Digital Marketing Teams
To seamlessly integrate AI analytics agents into existing digital marketing workflows, teams must follow a structured approach. Begin by conducting a comprehensive audit of current analytics processes, identifying areas where AI can provide the most significant impact. Next, evaluate and select AI-powered analytics tools that align with your specific needs, considering factors such as data integration capabilities, scalability, and user-friendly interfaces. Implement a phased rollout strategy, starting with a pilot program in a single marketing channel to minimize disruption. Prioritize team training and upskilling, focusing on data interpretation, AI model understanding, and ethical considerations in AI-driven decision-making. Establish clear KPIs to measure the effectiveness of AI analytics agents, such as time saved on report generation, accuracy improvements, and actionable insights generated.
Regularly review and refine the AI models to ensure they remain aligned with evolving marketing objectives and industry trends.
Foster a culture of continuous learning and adaptation, encouraging team members to collaborate with AI agents as augmented intelligence partners rather than replacements. By following this systematic approach, digital marketing teams can harness the full potential of AI analytics agents to drive data-driven strategies and optimize campaign performance.
Key Implementation Steps:
1. Audit existing analytics processes
2. Select appropriate AI-powered tools
3. Execute phased rollout strategy
4. Conduct comprehensive team training
5. Establish performance metrics
6. Continuously refine AI models
7. Foster a collaborative AI-human workflow
Tool Selection Criteria:
Criteria | Importance |
---|---|
Data integration capabilities | High |
Scalability | Medium |
User-friendly interface | High |
Customization options | Medium |
Real-time reporting | High |
Case Studies: Success Stories of Businesses Leveraging AI Analytics Agents
Several forward-thinking companies have reaped substantial benefits from implementing AI analytics agents in their digital marketing operations. Acme E-commerce, a mid-sized online retailer, deployed an AI-powered analytics agent that revolutionized their reporting processes. Within six months, the agent automated 85% of routine analytics tasks, reducing report generation time from 3 days to just 4 hours. This efficiency gain translated to a 22% increase in marketing team productivity and a 15% boost in campaign ROI. Similarly, TechNova Solutions, a B2B software provider, leveraged an AI analytics agent to unify data from disparate marketing channels. The agent’s predictive capabilities improved lead scoring accuracy by 37%, resulting in a 28% increase in qualified leads and a 19% higher conversion rate. Perhaps most impressively, GlobalMedia Corp, a multinational publishing house, implemented an AI agent for real-time content performance analysis across 50+ websites. The agent’s natural language processing capabilities enabled instant insights generation, leading to a 41% improvement in content engagement metrics and a 33% reduction in content production costs. These case studies underscore the transformative potential of AI analytics agents in driving data-driven decision-making and optimizing marketing performance across diverse business contexts.
Overcoming Challenges: Addressing Common Concerns About AI in Analytics Reporting
Implementing AI agents for analytics reporting in digital marketing presents several challenges that businesses must navigate. Data privacy remains a paramount concern, with 87% of consumers expressing worries about how their data is used. To address this, organizations must implement robust encryption protocols, adhere to GDPR and CCPA regulations, and provide transparent data usage policies. Integration issues often arise when incorporating AI agents into existing analytics infrastructures. A survey of 500 marketing professionals revealed that 62% struggled with seamless integration of AI tools. Overcoming this requires a phased approach, starting with API-driven connections and gradually moving towards deeper system integrations. Employee resistance can significantly hinder adoption, with 41% of workers expressing fear of job displacement due to AI. To mitigate this, companies should focus on reskilling initiatives, emphasizing AI as an augmentation rather than a replacement for human expertise. Implementing a change management strategy that includes clear communication, hands-on training, and showcasing early wins can increase adoption rates by up to 70%. Additionally, addressing algorithmic bias is crucial, as 76% of consumers are concerned about AI-driven discrimination. Regular audits, diverse training data, and human oversight in decision-making processes can help ensure fair and accurate analytics reporting. By proactively addressing these concerns, businesses can unlock the full potential of AI in analytics, potentially increasing marketing ROI by 20-30% and reducing reporting time by up to 80%.
The Future of Analytics Reporting: Predictions and Trends in AI-Driven Insights
The landscape of analytics reporting is poised for a revolutionary transformation, driven by advancements in AI agent technologies. Industry experts forecast a seismic shift in how digital marketers harness data-driven insights. By 2027, an estimated 85% of enterprise-level marketing teams will integrate AI-powered analytics agents into their core reporting workflows, resulting in a 40% reduction in time spent on data analysis and a 60% increase in actionable insights generated. These AI agents will leverage natural language processing and machine learning algorithms to autonomously collect, analyze, and synthesize vast amounts of cross-channel marketing data. Emerging technologies such as federated learning and edge AI will enable real-time processing of sensitive customer data while maintaining stringent privacy standards. This will empower marketers to make data-driven decisions with unprecedented speed and accuracy.
Key Trends Shaping AI-Driven Analytics
Predictive Analytics Evolution: AI agents will move beyond descriptive and diagnostic analytics to focus on predictive and prescriptive insights. By 2025, 70% of digital marketing strategies will be informed by AI-generated forecasts, with a 30% improvement in campaign performance metrics.
Automated Insight Generation: Natural language generation capabilities will allow AI agents to autonomously produce comprehensive marketing reports, complete with data visualizations and strategic recommendations. This is expected to save marketing teams an average of 15 hours per week on report creation.
Cross-Platform Data Integration: Advanced AI agents will seamlessly integrate data from diverse marketing platforms, social media channels, and customer touchpoints, providing a holistic view of marketing performance. This integration is projected to increase cross-selling opportunities by 25% and improve customer retention rates by 20%.
Impact on Digital Marketing Strategies
The proliferation of AI-driven analytics will fundamentally reshape digital marketing strategies:
- Hyper-personalization at scale, with AI agents enabling real-time content and offer optimization for individual customers across all digital touchpoints.
- Predictive customer journey mapping, allowing marketers to anticipate and influence customer behaviors with 85% accuracy.
- Automated budget allocation, with AI agents dynamically adjusting marketing spend across channels to maximize ROI, potentially increasing overall marketing efficiency by 35%.
As these AI-driven analytics capabilities mature, digital marketers who successfully integrate these technologies into their strategies will gain a significant competitive advantage, driving higher engagement rates, conversion rates, and ultimately, revenue growth.
Ethical Considerations: Navigating the Moral Landscape of AI in Marketing Analytics
The integration of AI agents in marketing analytics reporting raises critical ethical concerns that demand careful consideration. Data privacy stands at the forefront, as AI systems process vast amounts of consumer information, potentially infringing on individual rights. Marketers must implement robust safeguards to ensure compliance with regulations like GDPR and CCPA, which mandate transparent data collection practices and user consent. Algorithmic transparency presents another challenge, as the complexity of AI models often creates a “black box” effect, making it difficult to explain decision-making processes to stakeholders. This opacity can erode trust and hinder accountability, necessitating the development of interpretable AI systems.
Bias mitigation in AI algorithms is paramount to prevent discriminatory outcomes in marketing campaigns. Unchecked biases can lead to unfair targeting, exclusion of certain demographics, or perpetuation of harmful stereotypes. To address this, organizations must implement rigorous testing protocols, diverse training datasets, and ongoing monitoring of AI outputs. Ethical AI frameworks, such as the IEEE’s Ethically Aligned Design, provide guidelines for responsible development and deployment of AI in marketing contexts.
Key Ethical Considerations in AI-Driven Marketing Analytics
Consideration | Impact | Mitigation Strategy |
---|---|---|
Data Privacy | Potential breach of consumer trust | Implement data minimization and encryption |
Algorithmic Transparency | Lack of accountability | Develop explainable AI models |
Bias Mitigation | Discriminatory marketing practices | Diverse data sets and regular audits |
The ethical use of AI in marketing analytics also encompasses informed consent and data sovereignty. Consumers must be empowered to make informed decisions about how their data is used, with clear opt-in/opt-out mechanisms. Additionally, the concept of data sovereignty challenges marketers to respect cultural and national boundaries in data processing and storage. As AI agents become more sophisticated, the potential for hyper-personalization raises questions about the ethical limits of persuasion and manipulation in marketing strategies.
Emerging Ethical Challenges
Looking ahead, the ethical landscape of AI in marketing analytics is evolving rapidly.
The rise of emotion AI and sentiment analysis technologies introduces new ethical dilemmas around the boundaries of personal privacy and emotional manipulation.
Marketers must grapple with the responsible use of these powerful tools, balancing business objectives with respect for consumer autonomy. Furthermore, the potential for AI to exacerbate digital divides and reinforce societal inequalities through targeted marketing practices demands ongoing scrutiny and proactive measures to ensure equitable outcomes.
AI Analytics Agents: Leveling the Playing Field for SMEs
AI analytics agents are revolutionizing the digital marketing landscape for small to medium enterprises (SMEs), providing enterprise-grade insights at a fraction of the cost. These intelligent systems leverage advanced machine learning algorithms and natural language processing to analyze vast amounts of data, delivering actionable intelligence that was once the exclusive domain of large corporations with hefty budgets. By automating complex data analysis tasks, AI agents can process information from multiple sources—including website traffic, social media engagement, customer behavior patterns, and competitor activities—to generate comprehensive reports and predictive models. This capability allows SMEs to make data-driven decisions with unprecedented accuracy and speed.
Cost-Effective Insights
The economic impact of AI analytics agents for SMEs is substantial. Traditional analytics solutions often require significant investment in software licenses, hardware, and specialized personnel. In contrast, AI-powered analytics platforms operate on a more accessible Software-as-a-Service (SaaS) model, with pricing structures that scale with business needs. This democratization of advanced analytics enables SMEs to compete more effectively with larger rivals, as they can now access sophisticated market intelligence and customer insights without breaking the bank.
Competitive Advantage Through Automation
AI agents excel at automating routine analytical tasks, freeing up human resources to focus on strategy and creative endeavors. For instance, these agents can continuously monitor key performance indicators (KPIs), detect anomalies in real-time, and even suggest optimizations for marketing campaigns. This level of automation not only increases efficiency but also reduces the likelihood of human error in data interpretation. SMEs leveraging AI analytics agents report up to 30% improvement in marketing ROI and a 25% reduction in customer acquisition costs within the first year of implementation.
Personalization at Scale
One of the most powerful capabilities of AI analytics agents is their ability to enable personalization at scale. By analyzing individual customer interactions across multiple touchpoints, these agents can create detailed customer profiles and segment audiences with remarkable precision. This granular understanding allows SMEs to tailor their marketing messages and product offerings to specific customer needs, resulting in higher engagement rates and improved customer loyalty. Some SMEs have seen conversion rates increase by up to 50% through AI-driven personalization strategies.
Metric | Average Improvement |
---|---|
Marketing ROI | 30% |
Customer Acquisition Cost | 25% reduction |
Conversion Rates | Up to 50% increase |
As AI analytics agents continue to evolve, they are increasingly incorporating predictive analytics and prescriptive recommendations, further enhancing their value to SMEs. These advanced features allow businesses to anticipate market trends, forecast demand, and proactively address potential issues before they impact the bottom line. By leveraging these powerful tools, SMEs are not just surviving in the digital age—they’re thriving and competing on a level playing field with industry giants.
FAQ: Everything You Need to Know About AI Analytics Agents in Digital Marketing
What are AI analytics agents in digital marketing?
AI analytics agents in digital marketing are sophisticated software entities that leverage artificial intelligence and machine learning algorithms to autonomously collect, process, and analyze vast amounts of marketing data. These agents go beyond traditional analytics tools by proactively identifying trends, anomalies, and opportunities within complex datasets. They can integrate with multiple data sources, including web analytics, social media metrics, CRM systems, and advertising platforms, to provide a holistic view of marketing performance. AI analytics agents excel at real-time data processing, predictive modeling, and generating actionable insights without human intervention.
How do AI analytics agents differ from traditional marketing analytics tools?
AI analytics agents represent a significant leap forward from traditional marketing analytics tools in several key areas:
- Autonomy: AI agents can operate independently, making decisions and taking actions without constant human oversight.
- Predictive Capabilities: They use advanced machine learning models to forecast future trends and outcomes with high accuracy.
- Adaptive Learning: AI agents continuously improve their performance by learning from new data and past interactions.
- Natural Language Processing: Many AI agents can understand and generate human-like reports and recommendations.
- Cross-platform Integration: They seamlessly connect and analyze data from multiple marketing channels and tools.
What specific tasks can AI analytics agents perform in digital marketing?
AI analytics agents are capable of performing a wide range of sophisticated tasks in digital marketing:
- Automated campaign performance analysis and optimization
- Real-time budget allocation across marketing channels
- Predictive customer segmentation and personalization
- Anomaly detection in marketing metrics and fraud prevention
- Competitive intelligence gathering and market trend analysis
- Attribution modeling and customer journey mapping
- Sentiment analysis of customer feedback and social media mentions
- Content performance prediction and recommendation
- Automated A/B testing and multivariate experimentation
- Dynamic pricing optimization based on market conditions
What are the key benefits of implementing AI analytics agents in digital marketing?
Implementing AI analytics agents in digital marketing offers numerous benefits:
1. Enhanced Efficiency: AI agents can process and analyze data 24/7, reducing manual work by up to 80%.
2. Improved Accuracy: Machine learning algorithms can detect patterns and insights that humans might miss, increasing analytical accuracy by 35-50%.
3. Faster Decision-Making: Real-time analysis and recommendations enable marketers to make data-driven decisions 5x faster.
4. Personalization at Scale: AI agents can create and manage thousands of personalized customer segments simultaneously.
5. Predictive Insights: Forecasting capabilities allow marketers to anticipate trends and customer behavior with up to 85% accuracy.
6. Cost Optimization: Automated budget allocation and performance optimization can lead to a 20-30% reduction in wasted ad spend.
7. Competitive Advantage: Early adopters of AI analytics agents report gaining market share 2-3x faster than competitors.
What challenges might marketers face when implementing AI analytics agents?
While AI analytics agents offer significant advantages, marketers should be aware of potential implementation challenges:
- Data Quality and Integration: Ensuring clean, consistent data across multiple sources can be complex.
- Skill Gap: Teams may need upskilling to effectively work with and interpret AI-driven insights.
- Ethical Considerations: Balancing personalization with privacy concerns and regulatory compliance is crucial.
- Initial Setup Costs: Implementing sophisticated AI systems may require significant upfront investment.
- Change Management: Adapting organizational processes to leverage AI insights can be challenging.
- Transparency and Explainability: Understanding the decision-making process of AI agents may be difficult.
- Overreliance on Automation: Maintaining human oversight and creativity alongside AI-driven processes is essential.
AI Analytics Agent Capability | Average Performance Improvement | Implementation Complexity |
---|---|---|
Campaign Optimization | 25-40% | Medium |
Customer Segmentation | 50-70% | High |
Predictive Analytics | 30-50% | High |
Real-time Personalization | 40-60% | Very High |
Attribution Modeling | 20-35% | Medium |