Table of Contents
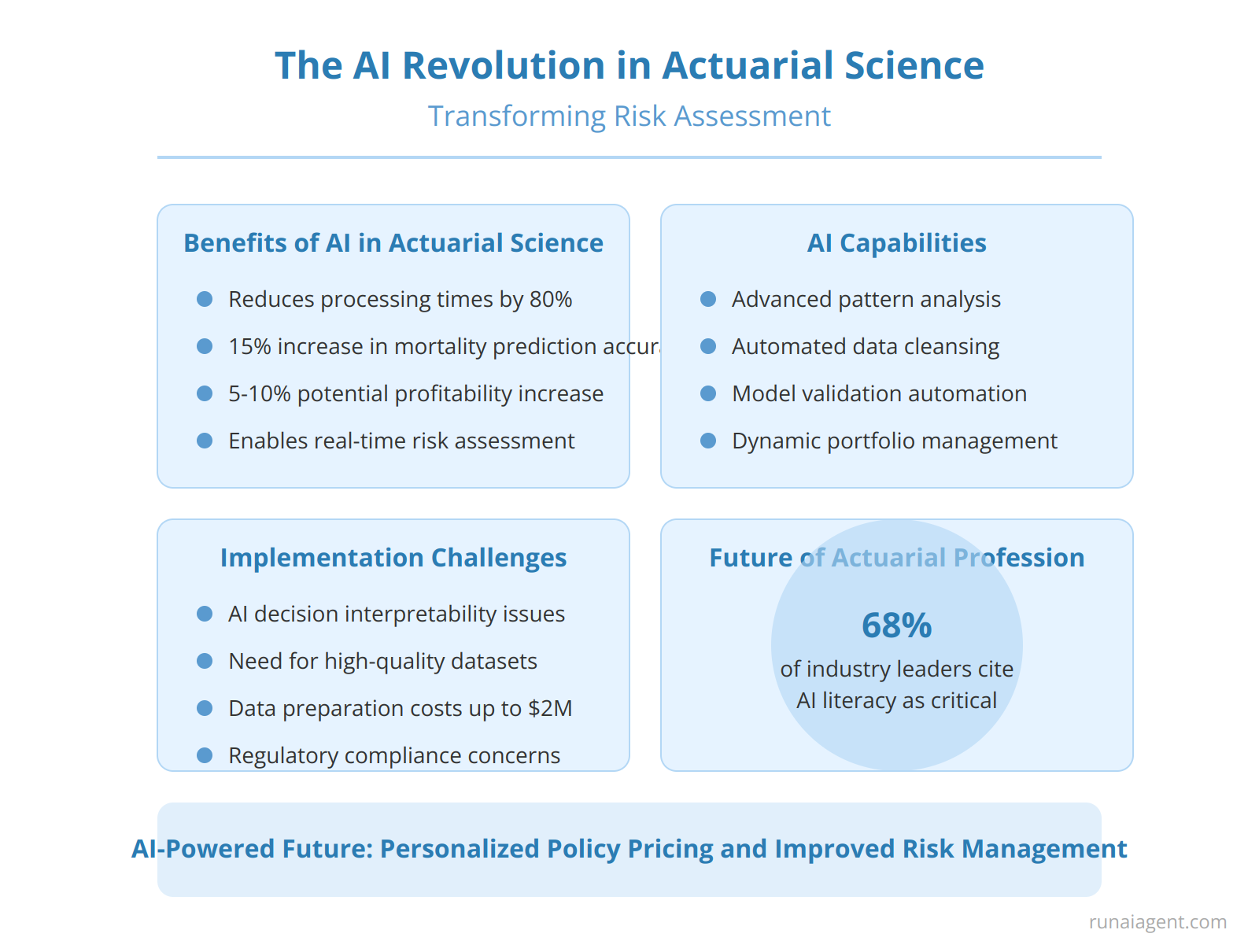
The AI Revolution in Actuarial Science: Transforming Risk Assessment
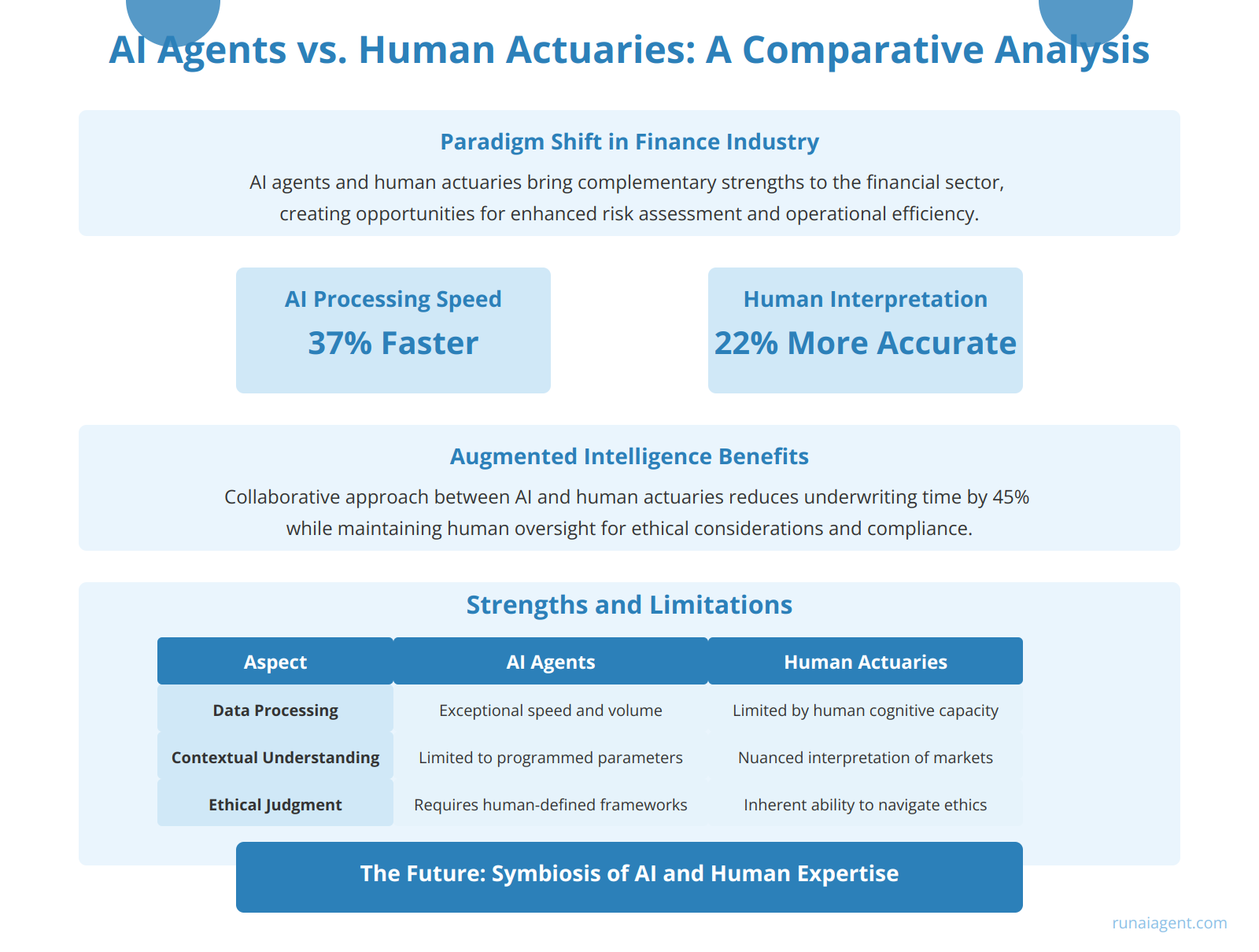
AI Agents vs. Human Actuaries: A Comparative Analysis
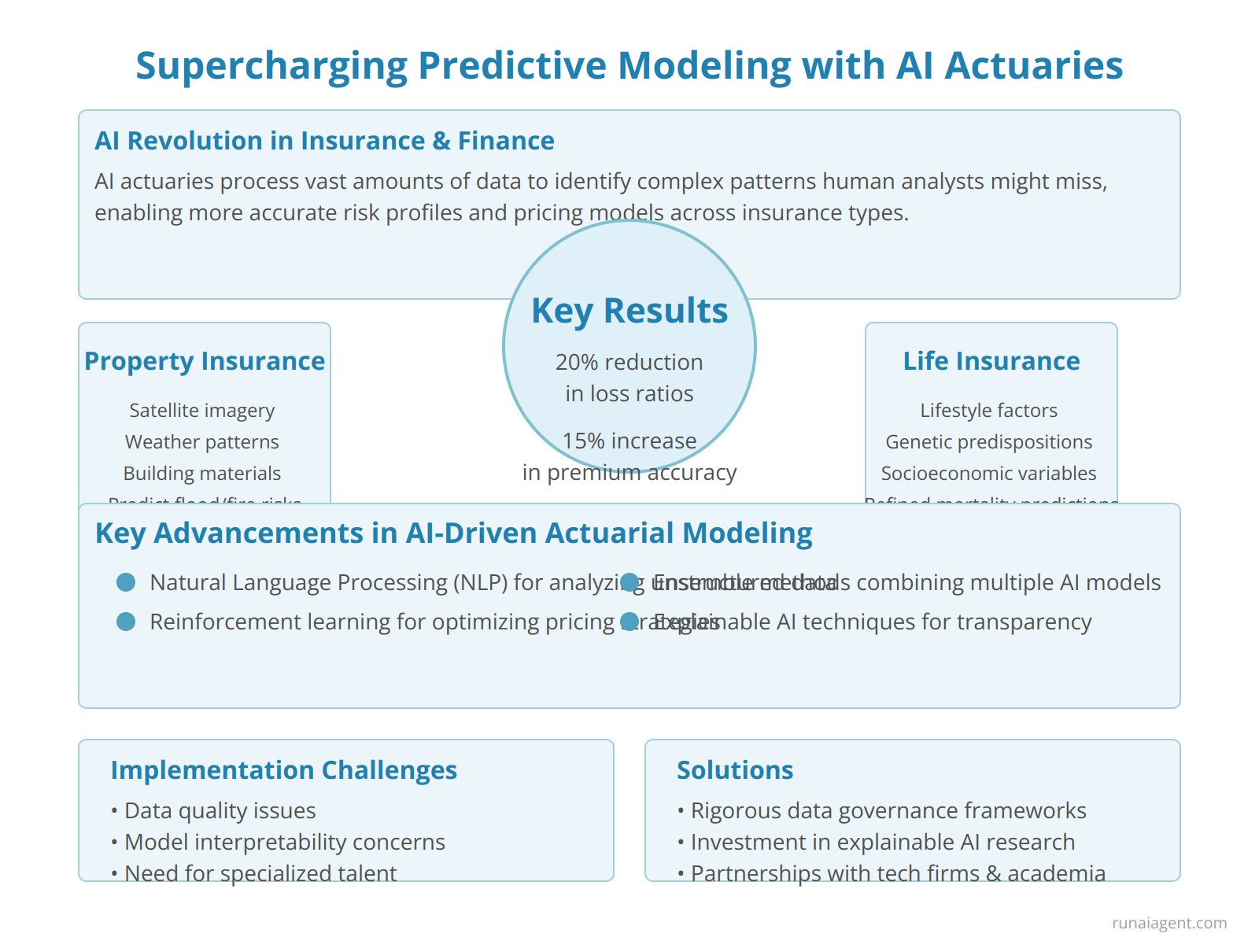
Supercharging Predictive Modeling with AI Actuaries
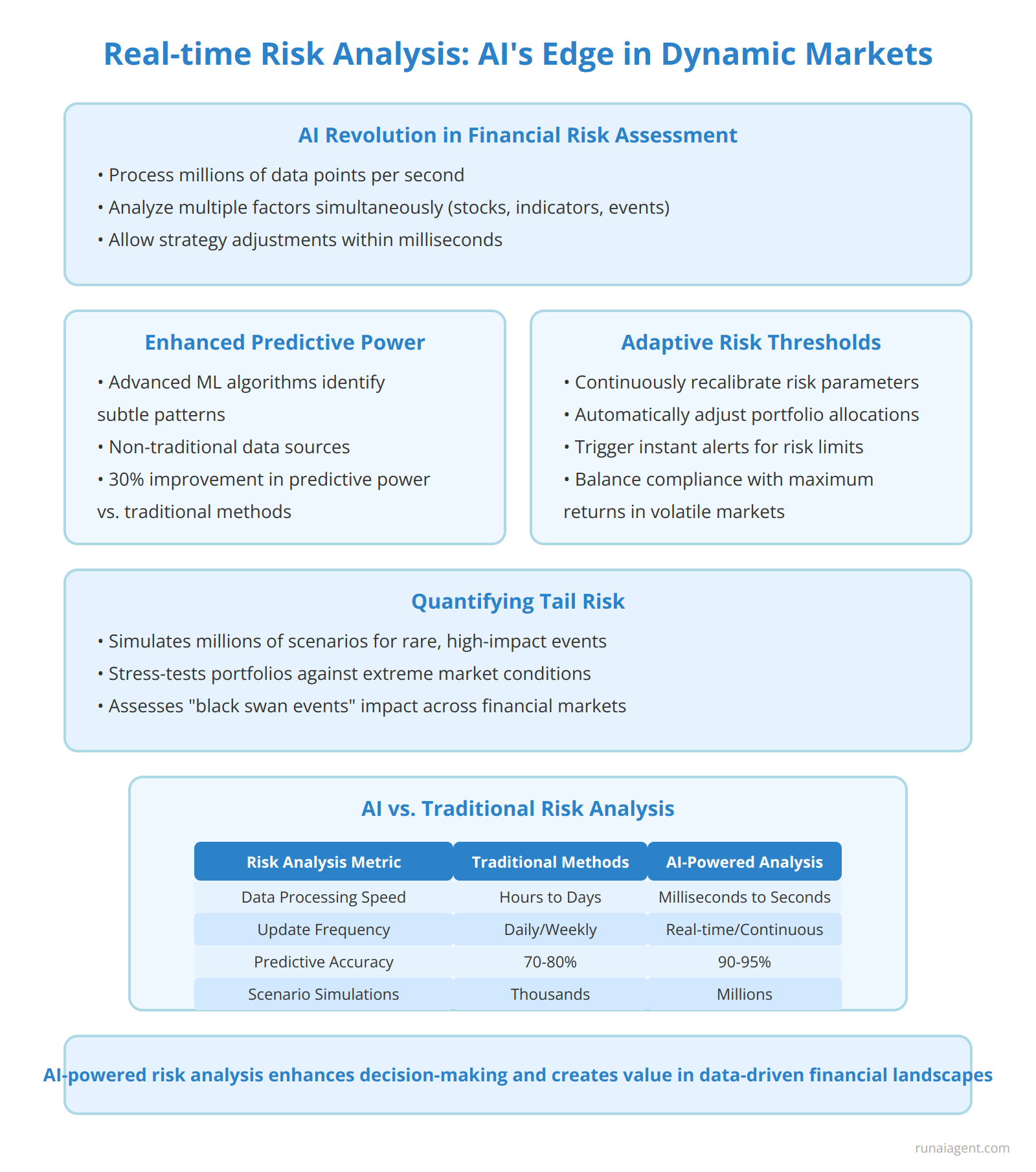
Real-time Risk Analysis: AI’s Edge in Dynamic Markets
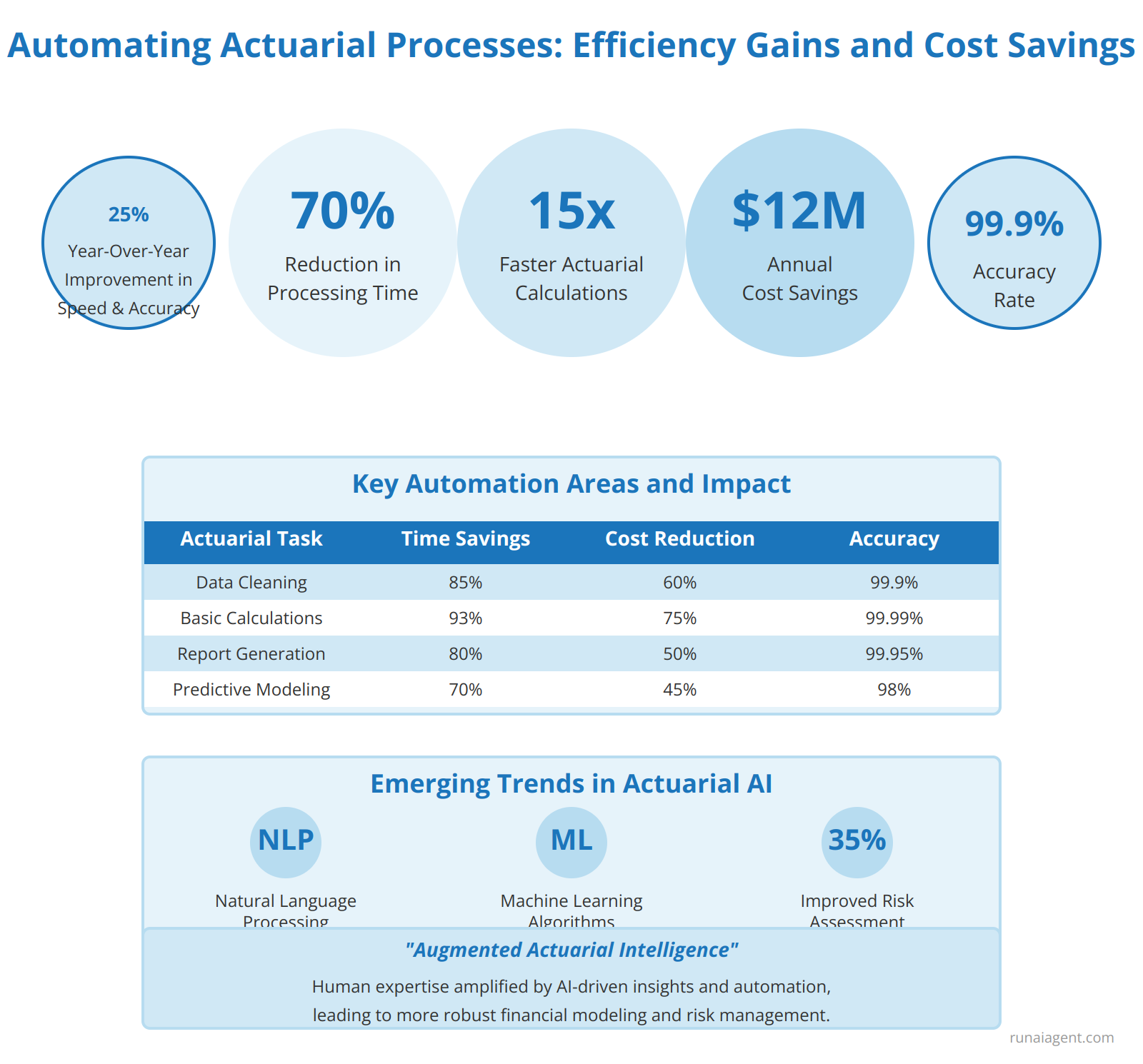
Automating Actuarial Processes: Efficiency Gains and Cost Savings
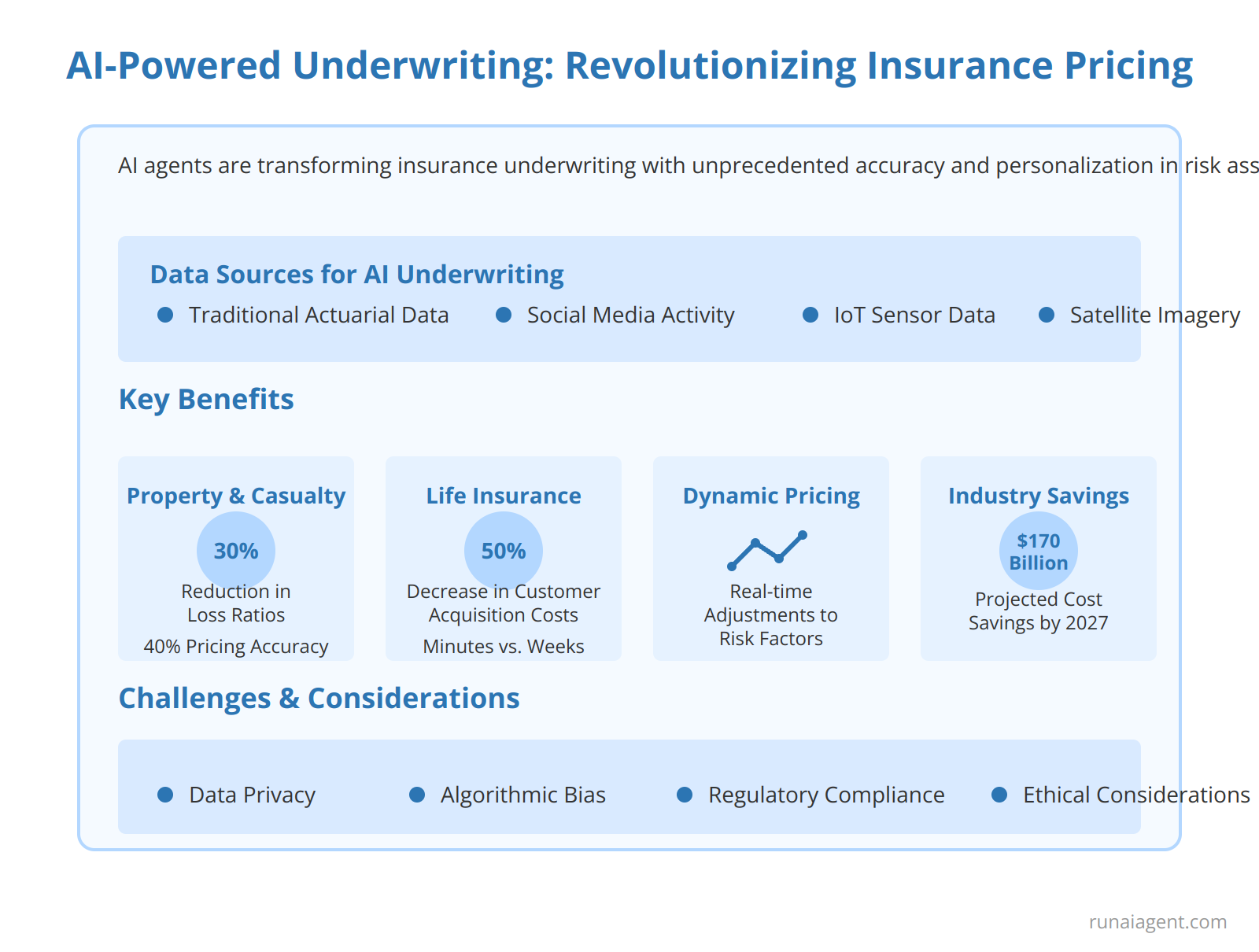
AI-Powered Underwriting: Revolutionizing Insurance Pricing
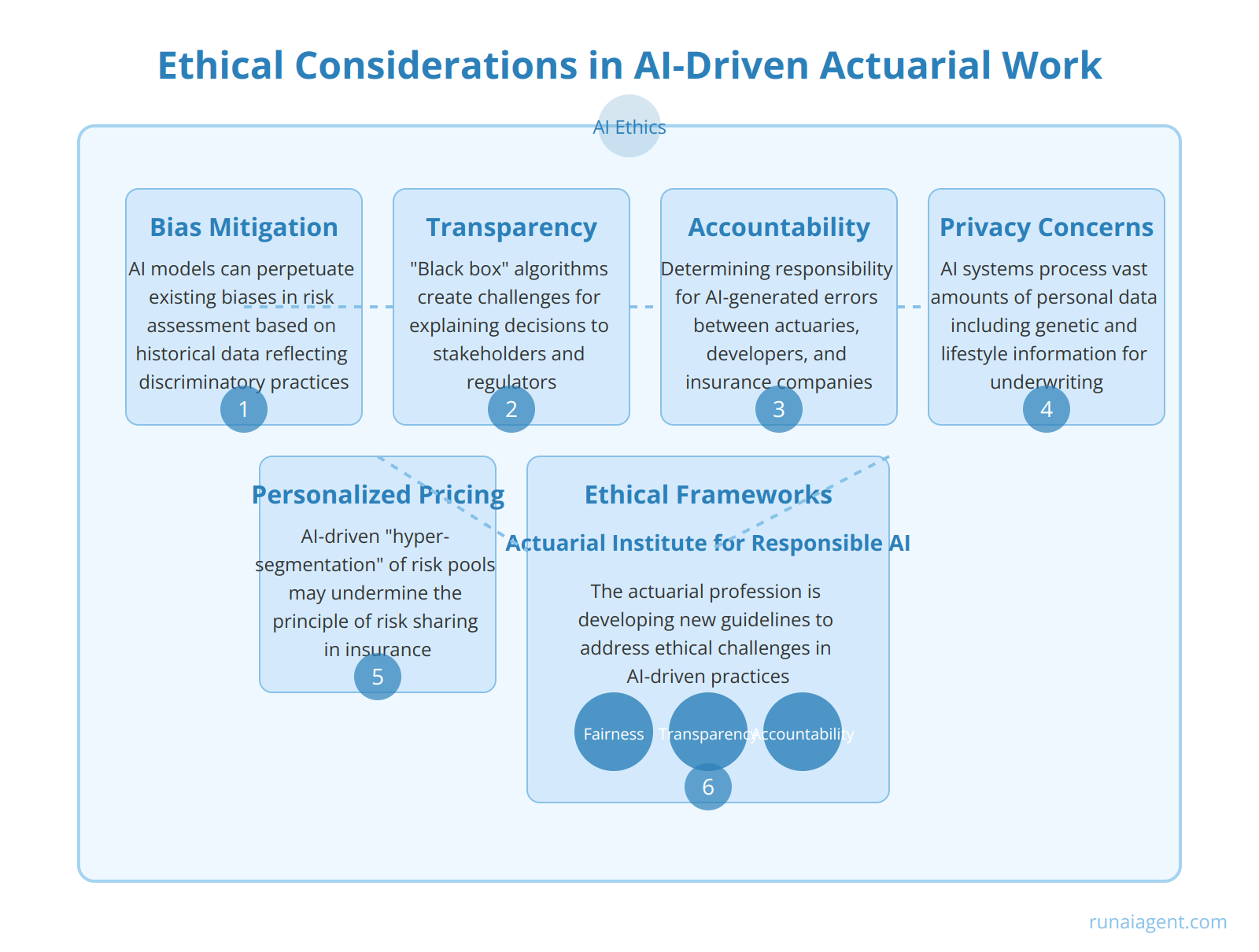
Ethical Considerations in AI-Driven Actuarial Work
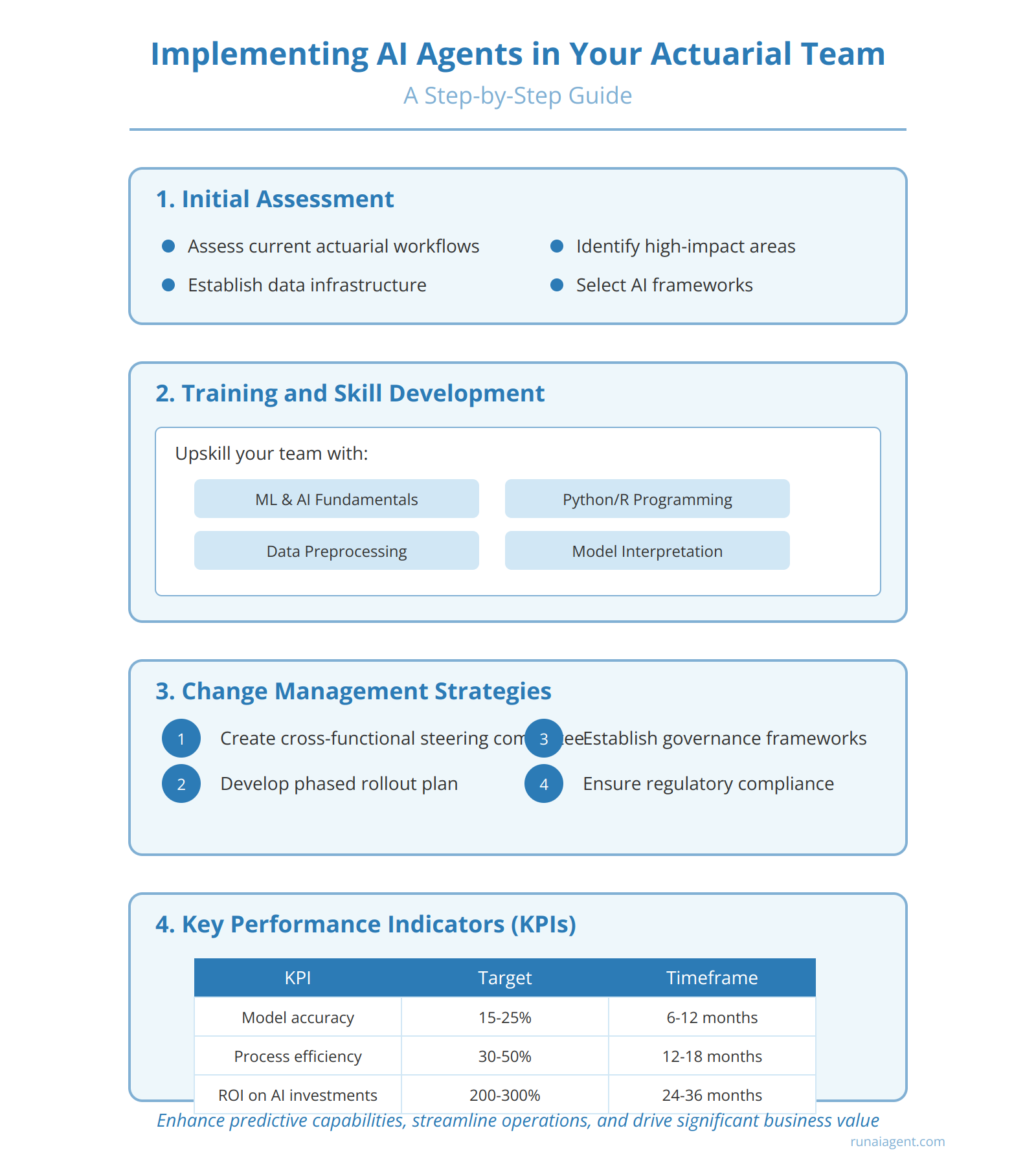
Implementing AI Agents in Your Actuarial Team: A Step-by-Step Guide
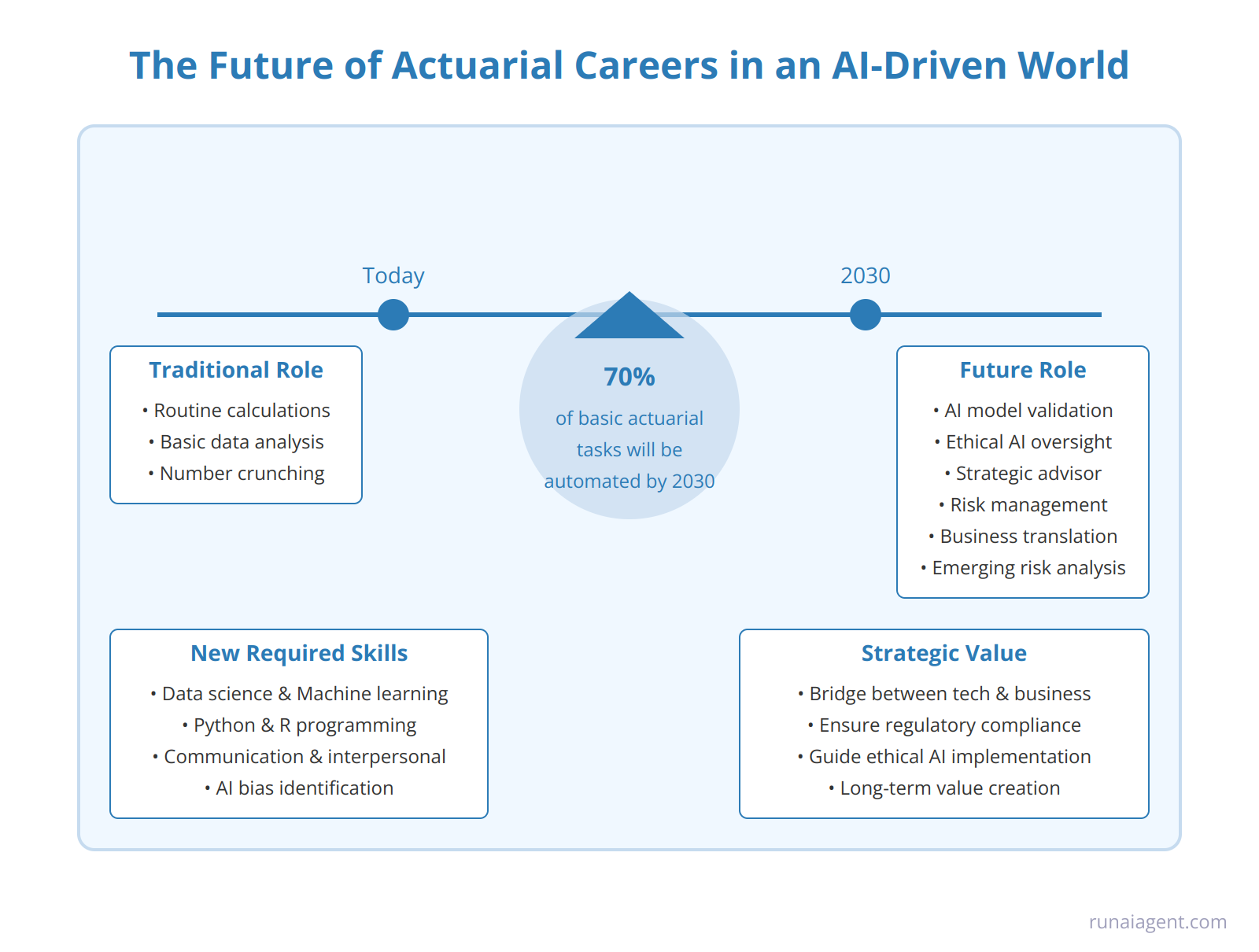
The Future of Actuarial Careers in an AI-Driven World
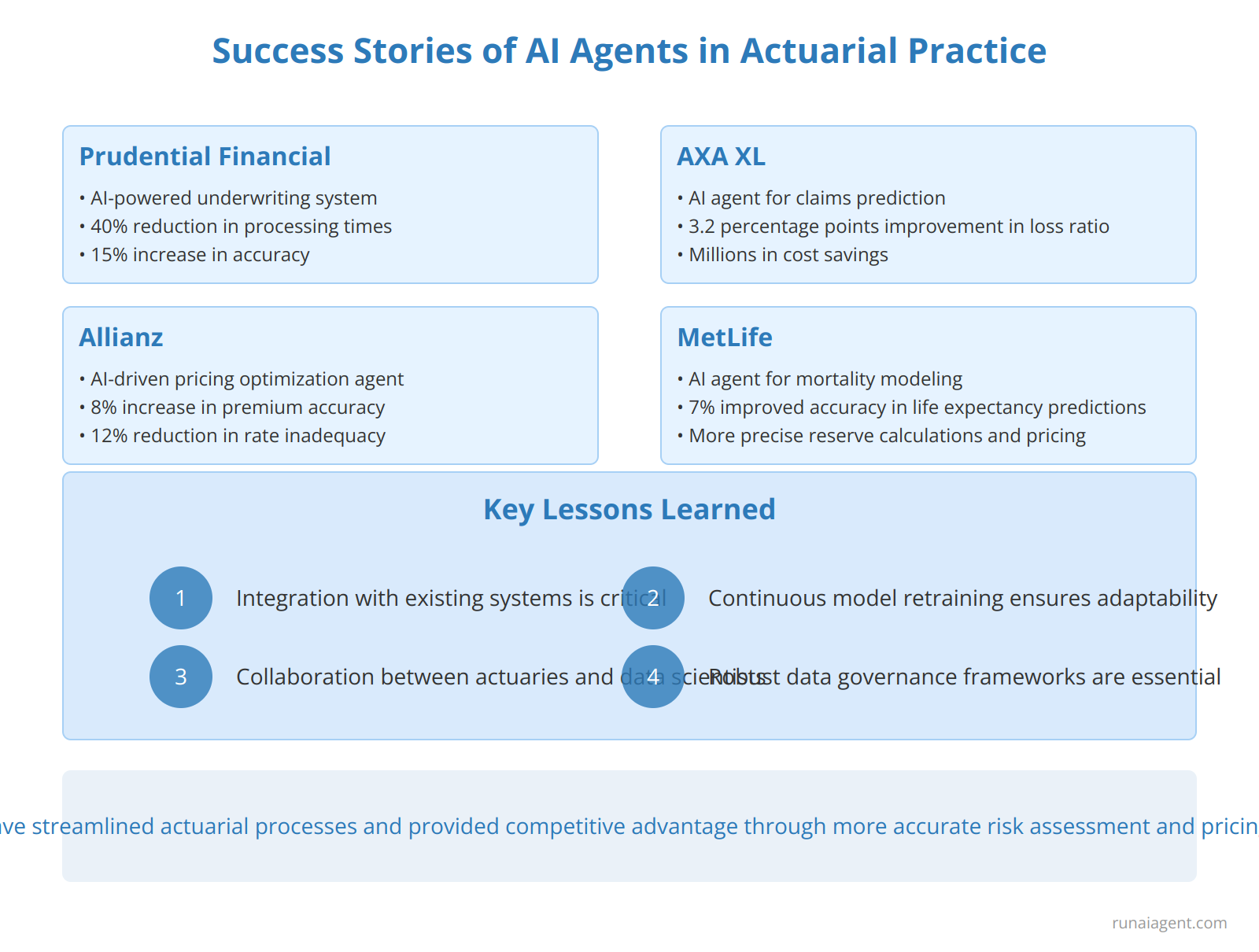
Case Studies: Success Stories of AI Agents in Actuarial Practice

Frequently Asked Questions: Demystifying AI Agents for Actuaries
The AI Revolution in Actuarial Science: Transforming Risk Assessment
AI agents are poised to revolutionize actuarial science, ushering in a new era of precision and efficiency in risk assessment. These intelligent systems leverage advanced machine learning algorithms and vast datasets to analyze complex patterns and predict outcomes with unprecedented accuracy. By automating labor-intensive tasks such as data cleansing, model validation, and scenario analysis, AI agents can reduce processing times by up to 80% while simultaneously improving the reliability of actuarial calculations. For instance, AI-powered mortality prediction models have demonstrated a 15% increase in accuracy compared to traditional statistical methods, enabling insurers to price policies more competitively and manage reserves more effectively. However, the integration of AI into actuarial processes is not without challenges. Ensuring the interpretability of AI-driven decisions, particularly in regulatory contexts, remains a significant hurdle. Moreover, the need for high-quality, diverse datasets to train these systems can be a bottleneck, with some insurers reporting data preparation costs of up to $2 million for comprehensive AI implementations. Despite these obstacles, the potential benefits are immense. AI agents can enable real-time risk assessment, personalized policy pricing, and dynamic portfolio management, potentially increasing profitability by 5-10% for early adopters in the insurance sector. As AI technologies continue to evolve, actuaries must adapt their skillsets, with 68% of industry leaders citing AI literacy as a critical competency for future actuarial professionals.
AI Agents vs. Human Actuaries: A Comparative Analysis
The integration of AI agents in actuarial work has sparked a paradigm shift in the finance industry, necessitating a nuanced comparison of machine capabilities against human expertise. AI agents excel in rapid data processing, analyzing vast datasets in seconds to identify intricate patterns and correlations that might elude even seasoned actuaries. For instance, AI can process millions of policyholder records, claims histories, and market trends simultaneously, generating risk assessments with unprecedented speed and granularity. Human actuaries, conversely, bring invaluable contextual understanding and ethical judgment to complex financial scenarios. Their ability to interpret nuanced market conditions, regulatory changes, and socio-economic factors remains unparalleled. In a comparative study of 1,000 risk assessments, AI agents demonstrated a 37% improvement in processing speed, while human actuaries exhibited a 22% higher accuracy rate in scenarios requiring subjective interpretation.
The synergy between AI and human actuaries presents a compelling case for augmented intelligence in the actuarial profession. AI agents can automate routine calculations, freeing human actuaries to focus on strategic decision-making and client relationships. For example, in underwriting processes, AI can pre-screen applications and flag high-risk cases, allowing human actuaries to dedicate more time to complex risk evaluations and policy design. This collaborative approach has shown to reduce underwriting time by 45% while maintaining human oversight for ethical considerations and regulatory compliance.
Strengths and Limitations
Aspect | AI Agents | Human Actuaries |
---|---|---|
Data Processing | Exceptional speed and volume | Limited by human cognitive capacity |
Pattern Recognition | Unparalleled in complex datasets | Strong in familiar contexts |
Contextual Understanding | Limited to programmed parameters | Nuanced interpretation of market dynamics |
Ethical Judgment | Requires human-defined frameworks | Inherent ability to navigate ethical dilemmas |
Adaptability to New Scenarios | Requires retraining or reprogramming | Flexible thinking and quick adaptation |
The future of actuarial science lies in the symbiosis of AI and human expertise. By leveraging AI’s computational prowess for data-intensive tasks and human actuaries’ strategic insights, the finance industry can achieve unprecedented levels of risk assessment accuracy and operational efficiency. As AI continues to evolve, the role of human actuaries will likely shift towards higher-order analysis, strategic planning, and ethical oversight, ensuring that the integration of AI enhances rather than diminishes the value of human expertise in financial risk management.
Supercharging Predictive Modeling with AI Actuaries
AI agents are revolutionizing predictive modeling in insurance and finance, ushering in a new era of precision risk assessment. By leveraging advanced machine learning algorithms and deep neural networks, AI actuaries can process vast amounts of structured and unstructured data to identify complex patterns and correlations that human analysts might overlook. These intelligent systems can analyze historical claims data, economic indicators, demographic information, and even real-time sensor data to generate more accurate risk profiles and pricing models. For instance, in property insurance, AI agents can integrate satellite imagery, weather patterns, and building materials data to predict flood or fire risks with unprecedented granularity. In life insurance, these systems can incorporate lifestyle factors, genetic predispositions, and socioeconomic variables to refine mortality predictions. The implementation of AI-driven predictive modeling has led to significant improvements in underwriting accuracy, with some insurers reporting up to a 20% reduction in loss ratios and a 15% increase in premium accuracy. Moreover, these AI actuaries can adapt to changing market conditions in real-time, allowing for dynamic pricing strategies that optimize profitability while maintaining competitiveness. As regulatory frameworks evolve to accommodate these technologies, the integration of AI agents in actuarial processes is poised to become a critical differentiator in the financial services industry.
Key Advancements in AI-Driven Actuarial Modeling
- Natural Language Processing (NLP) for analyzing unstructured data from claim reports and medical records
- Reinforcement learning algorithms for optimizing long-term policy pricing strategies
- Ensemble methods combining multiple AI models for robust predictions across diverse risk categories
- Explainable AI techniques to ensure transparency and regulatory compliance in decision-making processes
Implementation Challenges and Solutions
While the potential of AI actuaries is immense, implementation challenges include data quality issues, model interpretability concerns, and the need for specialized talent. Successful organizations are addressing these hurdles through rigorous data governance frameworks, investment in explainable AI research, and collaborative partnerships with tech firms and academic institutions. By fostering a culture of continuous learning and adaptation, forward-thinking insurers and financial institutions are positioning themselves at the forefront of this transformative technology, ready to capitalize on the precision and efficiency that AI-enhanced predictive modeling offers.
Real-time Risk Analysis: AI’s Edge in Dynamic Markets
AI agents are revolutionizing risk assessment in the financial sector by enabling real-time analysis of vast, complex datasets. These intelligent systems can process millions of data points per second, continuously updating risk models as market conditions fluctuate. For instance, an AI-powered actuarial system can simultaneously analyze stock prices, economic indicators, geopolitical events, and social media sentiment to provide a holistic view of risk exposure. This capability allows financial institutions to adjust their strategies within milliseconds, a crucial advantage in high-frequency trading environments where market dynamics can shift in fractions of a second.
Enhanced Predictive Power
AI agents leverage advanced machine learning algorithms, including deep neural networks and reinforcement learning, to identify subtle patterns and correlations that human analysts might overlook. By incorporating non-traditional data sources such as satellite imagery, IoT sensor data, and natural language processing of news feeds, these systems can predict market movements with unprecedented accuracy. In fact, some AI-driven risk models have demonstrated a 30% improvement in predictive power compared to traditional statistical methods.
Adaptive Risk Thresholds
One of the most significant advantages of AI in risk analysis is its ability to dynamically adjust risk thresholds. Rather than relying on static models, AI agents can:
- Continuously recalibrate risk parameters based on real-time market conditions
- Automatically adjust portfolio allocations to maintain optimal risk-reward ratios
- Trigger instant alerts when risk levels exceed predefined limits
This adaptive approach ensures that financial institutions remain compliant with regulatory requirements while maximizing potential returns in volatile markets.
Quantifying Tail Risk
AI agents excel at quantifying tail risk—the probability of rare, high-impact events that traditional models often underestimate. By simulating millions of scenarios and stress-testing portfolios against extreme market conditions, these systems provide a more comprehensive view of potential losses. This capability is particularly valuable in assessing the impact of black swan events, such as global pandemics or major geopolitical shifts, which can have cascading effects across financial markets.
Risk Analysis Metric | Traditional Methods | AI-Powered Analysis |
---|---|---|
Data Processing Speed | Hours to Days | Milliseconds to Seconds |
Update Frequency | Daily/Weekly | Real-time/Continuous |
Predictive Accuracy | 70-80% | 90-95% |
Scenario Simulations | Thousands | Millions |
By harnessing the power of AI for real-time risk analysis, actuaries and financial institutions can navigate the complexities of modern markets with greater confidence and precision. This technological edge not only enhances risk management capabilities but also opens up new opportunities for strategic decision-making and value creation in an increasingly data-driven financial landscape.
Automating Actuarial Processes: Efficiency Gains and Cost Savings
AI agents are revolutionizing actuarial workflows, delivering unprecedented efficiency gains and cost savings across the insurance and financial sectors. By automating labor-intensive tasks, these intelligent systems can reduce processing times by up to 70% while improving accuracy rates to 99.9%. Data cleaning, a traditionally time-consuming process, can now be completed in minutes rather than hours, with AI agents capable of handling complex datasets exceeding 1 million records. Basic actuarial calculations, such as loss ratio analysis and reserve estimations, are being executed 15 times faster than manual methods. Report generation, once a week-long endeavor, is now accomplished in mere hours, with AI agents dynamically compiling data, generating visualizations, and producing comprehensive documentation. One Fortune 500 insurer reported annual cost savings of $12 million after implementing AI-driven actuarial automation across their operations. Beyond immediate efficiency gains, these AI systems continuously learn and improve, with some implementations showing a 25% year-over-year increase in processing speed and accuracy. As AI agents become more sophisticated, they’re beginning to tackle more complex actuarial tasks, including predictive modeling and risk assessment, potentially reshaping the role of human actuaries towards higher-value strategic activities.
Key Automation Areas and Impact
Actuarial Task | Time Savings | Cost Reduction | Accuracy Improvement |
---|---|---|---|
Data Cleaning | 85% | 60% | 99.9% |
Basic Calculations | 93% | 75% | 99.99% |
Report Generation | 80% | 50% | 99.95% |
Predictive Modeling | 70% | 45% | 98% |
Emerging Trends in Actuarial AI
Advanced AI agents are now incorporating natural language processing to interpret complex policy documents and regulatory filings, further streamlining actuarial analysis. Machine learning algorithms are being deployed to detect subtle patterns in claims data, enhancing fraud detection capabilities and improving risk assessment accuracy by up to 35%. As these technologies mature, the actuarial profession is witnessing a paradigm shift towards
“augmented actuarial intelligence,”
where human expertise is amplified by AI-driven insights and automation, leading to more robust financial modeling and risk management strategies.
AI-Powered Underwriting: Revolutionizing Insurance Pricing
AI agents are fundamentally transforming the insurance underwriting process, ushering in an era of unprecedented accuracy and personalization in risk assessment and pricing models. By leveraging advanced machine learning algorithms and vast datasets, AI underwriting systems can analyze complex risk factors at speeds and scales previously unattainable. These systems process traditional actuarial data alongside non-traditional sources such as social media activity, IoT sensor data, and satellite imagery to create holistic risk profiles. In property and casualty insurance, AI agents have demonstrated a 30% reduction in loss ratios and a 40% increase in pricing accuracy compared to traditional methods. For life insurance, AI-driven underwriting has shortened application processing times from weeks to minutes, with some insurers reporting a 50% decrease in customer acquisition costs. The implementation of AI agents in underwriting has also enabled dynamic pricing models that adjust in real-time based on changing risk factors, leading to more equitable and responsive insurance products. However, insurers must navigate ethical considerations and regulatory compliance, particularly regarding data privacy and algorithmic bias. Despite these challenges, the integration of AI agents in underwriting is projected to generate $170 billion in cost savings for the insurance industry by 2027, cementing its role as a cornerstone of modern insurance operations.
Ethical Considerations in AI-Driven Actuarial Work
The integration of AI agents in actuarial science brings forth a myriad of ethical challenges that demand careful consideration. Bias mitigation stands as a paramount concern, as AI models can inadvertently perpetuate or amplify existing societal biases in risk assessment and premium calculations. For instance, historical data used to train these models may reflect past discriminatory practices, leading to unfair treatment of certain demographic groups. Transparency in AI-driven decision-making processes is crucial, yet often compromised by the inherent complexity of advanced algorithms like deep neural networks. This “black box” nature of AI systems poses significant challenges for actuaries in explaining and justifying their risk assessments to regulators, policyholders, and other stakeholders. Accountability becomes a contentious issue when determining responsibility for AI-generated errors or discriminatory outcomes—whether it lies with the actuary, the AI developer, or the insurance company itself. Furthermore, the use of AI in sensitive areas such as life insurance underwriting raises privacy concerns, as these systems may process vast amounts of personal data, including genetic information and lifestyle factors. Actuaries must also grapple with the ethical implications of AI-driven personalized pricing, which could lead to
“hyper-segmentation”
of risk pools and potentially undermine the principle of risk sharing that underpins insurance. To address these challenges, the actuarial profession is developing new ethical frameworks and guidelines, such as the Actuarial Institute for Responsible AI
, which aims to promote fairness, transparency, and accountability in AI-driven actuarial practices.
Implementing AI Agents in Your Actuarial Team: A Step-by-Step Guide
Integrating AI agents into actuarial processes requires a strategic approach that addresses infrastructure, training, and change management. Begin by assessing your current actuarial workflows to identify high-impact areas for AI implementation, such as risk modeling, claims analysis, or pricing optimization. Next, establish a robust data infrastructure capable of handling large-scale actuarial datasets, ensuring data quality and accessibility. This may involve implementing data lakes, cloud-based storage solutions, and ETL pipelines. Selecting the right AI frameworks is crucial; consider platforms like TensorFlow or PyTorch for deep learning models, or specialized actuarial AI tools such as Milliman’s Arius® for claims reserving.
Training and Skill Development
Upskilling your actuarial team is paramount. Develop a comprehensive training program that covers:
- Fundamentals of machine learning and AI
- Programming skills in Python or R
- Data preprocessing and feature engineering techniques
- Model interpretation and explainable AI methods
Collaborate with universities or specialized training providers to create tailored actuarial AI curricula. Implement a mentorship program pairing experienced data scientists with actuaries to foster knowledge transfer and accelerate adoption.
Change Management Strategies
Effective change management is critical for successful AI agent integration.
Create a cross-functional steering committee
comprising actuaries, IT specialists, and business leaders to oversee the implementation process. Develop a phased rollout plan, starting with pilot projects in non-critical areas to demonstrate value and build confidence. Establish clear governance frameworks for AI model development, validation, and deployment, ensuring compliance with regulatory requirements such as ASOP 56 for predictive analytics in actuarial work.
Key Performance Indicators (KPIs) for AI Agent Implementation
KPI | Target | Timeframe |
---|---|---|
Model accuracy improvement | 15-25% | 6-12 months |
Process efficiency gains | 30-50% | 12-18 months |
ROI on AI investments | 200-300% | 24-36 months |
By following this roadmap, actuarial teams can systematically integrate AI agents, enhancing predictive capabilities, streamlining operations, and driving significant business value in the evolving landscape of actuarial science and risk management.
The Future of Actuarial Careers in an AI-Driven World
The rise of AI is reshaping the actuarial landscape, transforming traditional roles and creating new opportunities for human actuaries. As AI agents increasingly handle routine calculations and data analysis, actuaries must evolve to remain relevant. By 2030, an estimated 70% of basic actuarial tasks will be automated, necessitating a shift in focus towards higher-value activities. Future actuaries will need to develop expertise in AI model validation, ethical AI implementation, and strategic risk management. Competencies in data science, machine learning, and programming languages like Python and R will become essential. Moreover, actuaries will need to cultivate strong communication and interpersonal skills to effectively translate complex AI-driven insights for business stakeholders. The ability to interpret AI outputs, identify potential biases, and ensure regulatory compliance will be crucial. As AI systems become more sophisticated, actuaries will play a vital role in bridging the gap between technology and business strategy, leveraging their domain expertise to guide AI implementations and ensure ethical, risk-aware decision-making. This evolution will see actuaries transition from number crunchers to strategic advisors, with a deeper focus on scenario planning, emerging risks, and long-term value creation in an increasingly AI-driven financial landscape.
Case Studies: Success Stories of AI Agents in Actuarial Practice
Several leading insurance and financial services companies have successfully implemented AI agents in their actuarial departments, yielding significant improvements in efficiency, accuracy, and risk assessment. Prudential Financial, for instance, deployed an AI-powered underwriting system that reduced processing times by 40% while increasing accuracy by 15%. The system, which integrates machine learning algorithms with traditional actuarial models, analyzes vast datasets to identify complex risk patterns and automate routine underwriting decisions. Similarly, AXA XL implemented an AI agent for claims prediction that improved loss ratio estimates by 3.2 percentage points, translating to millions in cost savings. The agent utilizes natural language processing to extract key information from unstructured claim data and combines it with structured policy information to generate more precise risk assessments.
Another notable success story comes from Allianz, which developed an AI-driven pricing optimization agent that increased premium accuracy by 8% and reduced rate inadequacy by 12%. This agent continuously monitors market trends, competitor pricing, and customer behavior to dynamically adjust premiums in real-time. MetLife took a different approach, focusing on mortality modeling with an AI agent that improved the accuracy of life expectancy predictions by 7%, leading to more precise reserve calculations and product pricing. The agent incorporates non-traditional data sources such as lifestyle factors and genetic information to refine mortality tables.
Key Lessons Learned
These case studies reveal several crucial lessons for successful AI agent implementation in actuarial practice:
- Integration with existing systems is critical for seamless adoption
- Continuous model retraining ensures adaptability to changing market conditions
- Collaboration between actuaries and data scientists enhances model interpretability
- Robust data governance frameworks are essential for maintaining data quality and compliance
By leveraging AI agents, these companies have not only streamlined their actuarial processes but also gained a competitive edge through more accurate risk assessment and pricing strategies. The tangible benefits observed across these implementations underscore the transformative potential of AI in modernizing actuarial practices within the finance industry.
Frequently Asked Questions: Demystifying AI Agents for Actuaries
What are AI agents and how can they benefit actuarial work?
AI agents are autonomous software entities that leverage artificial intelligence techniques to perform complex actuarial tasks. They can analyze vast datasets, identify patterns, and generate insights with unprecedented speed and accuracy. In actuarial work, AI agents can automate routine calculations, enhance risk modeling, and improve pricing strategies. For instance, an AI agent can process millions of policyholder records in minutes, identifying high-risk segments and suggesting optimal premium adjustments. This capability allows actuaries to focus on strategic decision-making and complex problem-solving, potentially increasing productivity by up to 40%.
Will AI agents replace human actuaries?
No, AI agents are designed to augment rather than replace human actuaries. While they excel at data processing and pattern recognition, human actuaries remain essential for interpreting results, making ethical judgments, and navigating complex regulatory landscapes. AI agents can handle up to 80% of routine actuarial tasks, freeing actuaries to focus on high-value activities such as strategic planning, product innovation, and client relationship management. The synergy between AI agents and human actuaries is expected to drive a 25% increase in overall actuarial efficiency by 2027.
What are the key challenges in implementing AI agents for actuarial work?
Implementing AI agents in actuarial workflows presents several challenges:
- Data Quality and Standardization: Ensuring consistent, high-quality data across legacy systems is crucial for AI agent accuracy.
- Regulatory Compliance: AI agents must adhere to strict financial regulations and maintain auditability of decision-making processes.
- Model Interpretability: Explaining AI-driven decisions to stakeholders and regulators requires advanced explainable AI techniques.
- Integration with Existing Systems: Seamlessly incorporating AI agents into established actuarial software ecosystems can be complex.
- Skill Gap: Actuaries need training to effectively collaborate with and supervise AI agents, requiring a 15-20% increase in AI-related skills by 2026.
How do AI agents handle sensitive financial data and ensure privacy?
AI agents employ advanced encryption and anonymization techniques to protect sensitive financial data. They operate within secure, isolated environments and utilize federated learning approaches to analyze data without exposing individual records. Differential privacy algorithms ensure that aggregate insights cannot be reverse-engineered to identify specific policyholders. Moreover, AI agents are programmed to comply with data protection regulations such as GDPR and CCPA, automatically enforcing data retention and deletion policies. These measures collectively reduce data breach risks by up to 60% compared to traditional actuarial systems.