Table of Contents
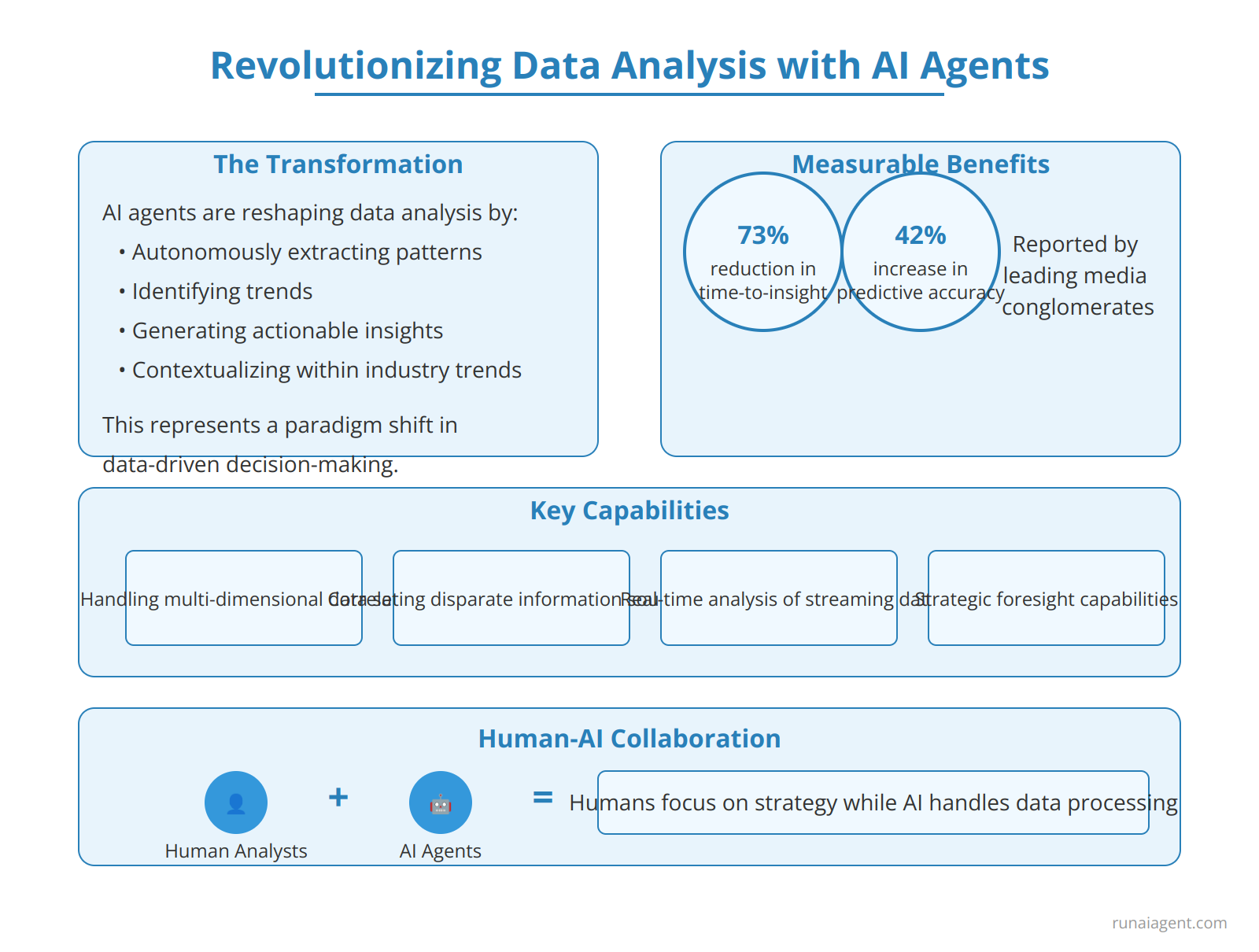
Revolutionizing Data Analysis: How AI Agents are Transforming the Role of Analytics Interpreters
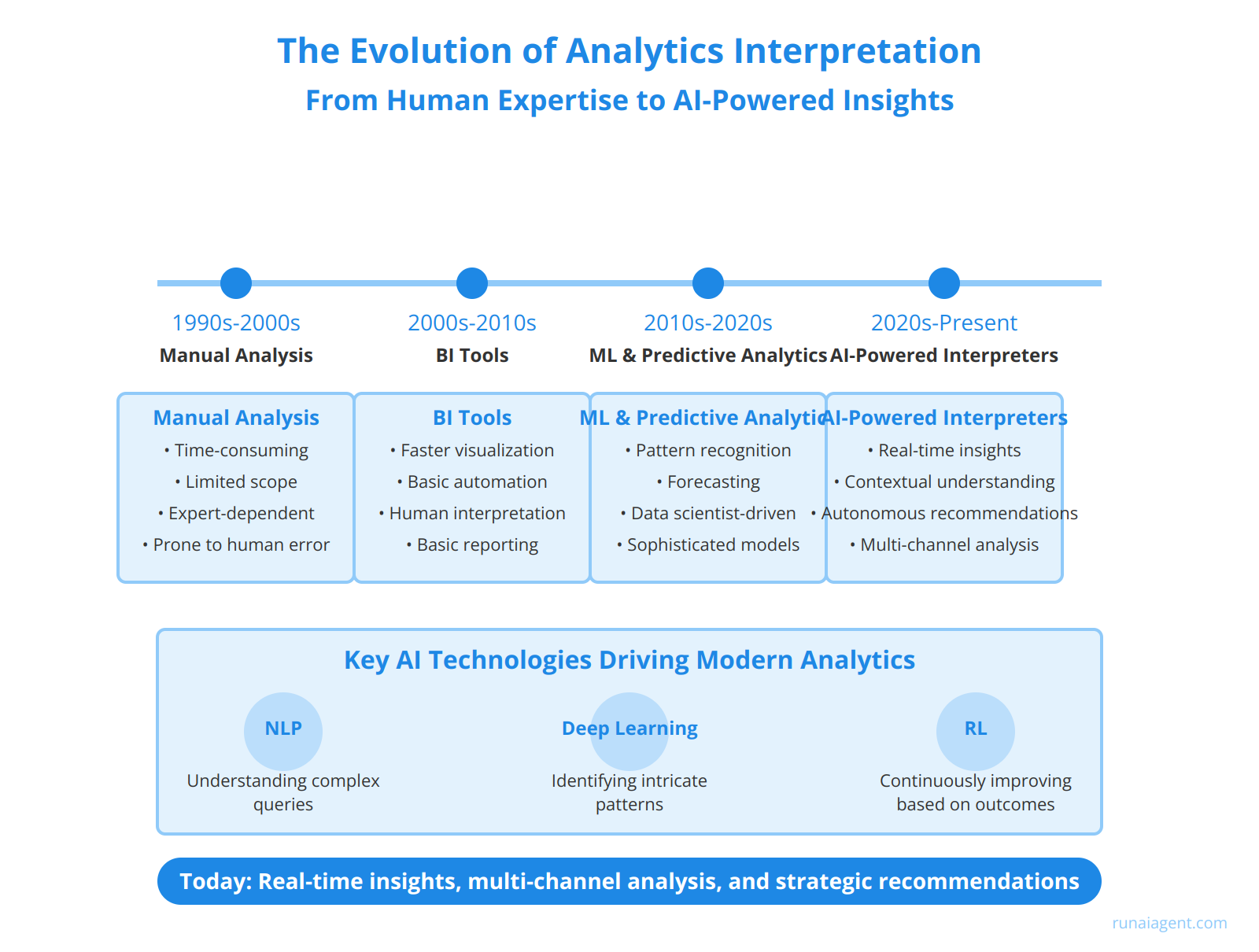
The Evolution of Analytics Interpretation: From Human Expertise to AI-Powered Insights
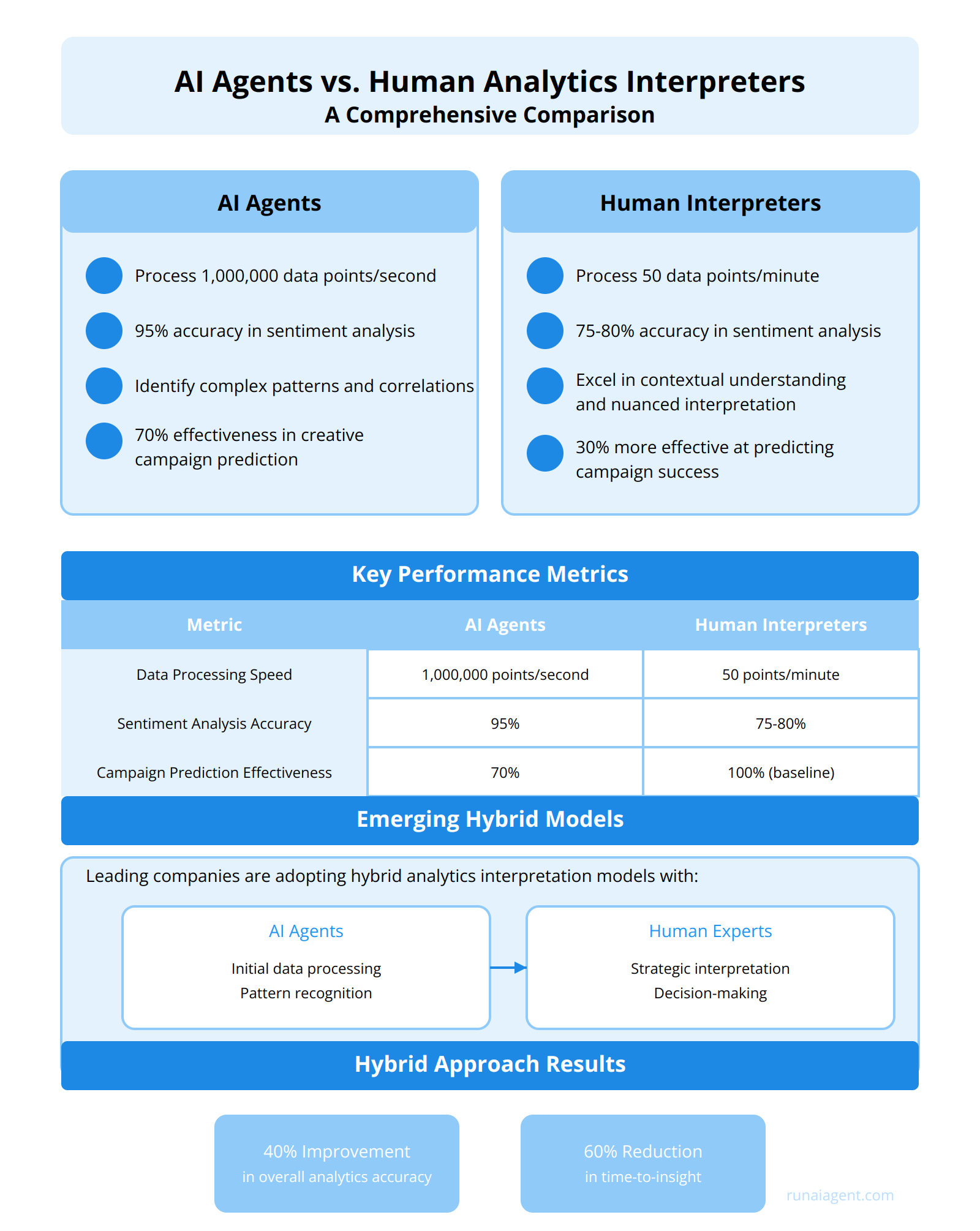
AI Agents vs. Human Analytics Interpreters: A Comprehensive Comparison
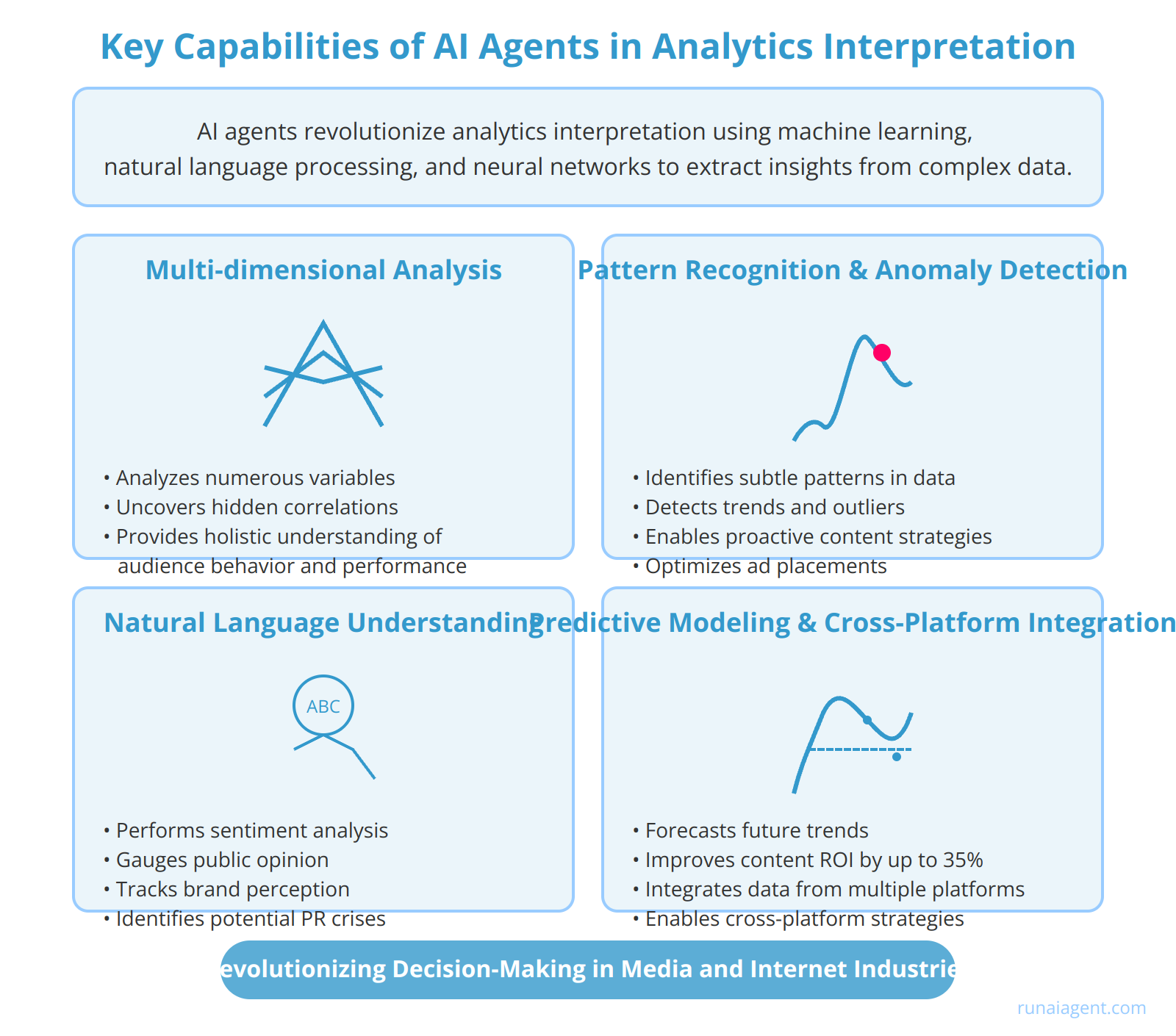
Key Capabilities of AI Agents in Analytics Interpretation
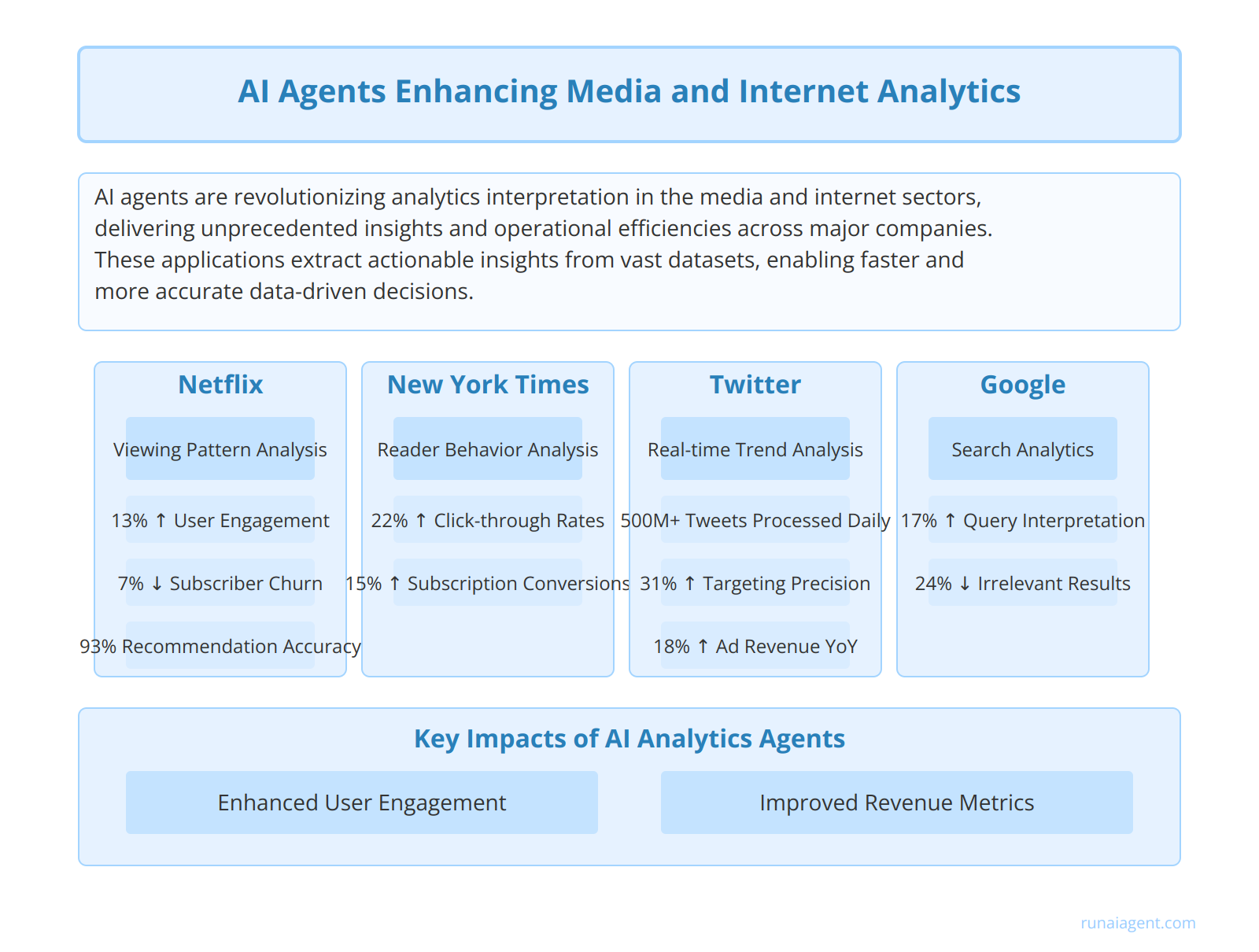
Real-World Applications: AI Agents Enhancing Media and Internet Analytics
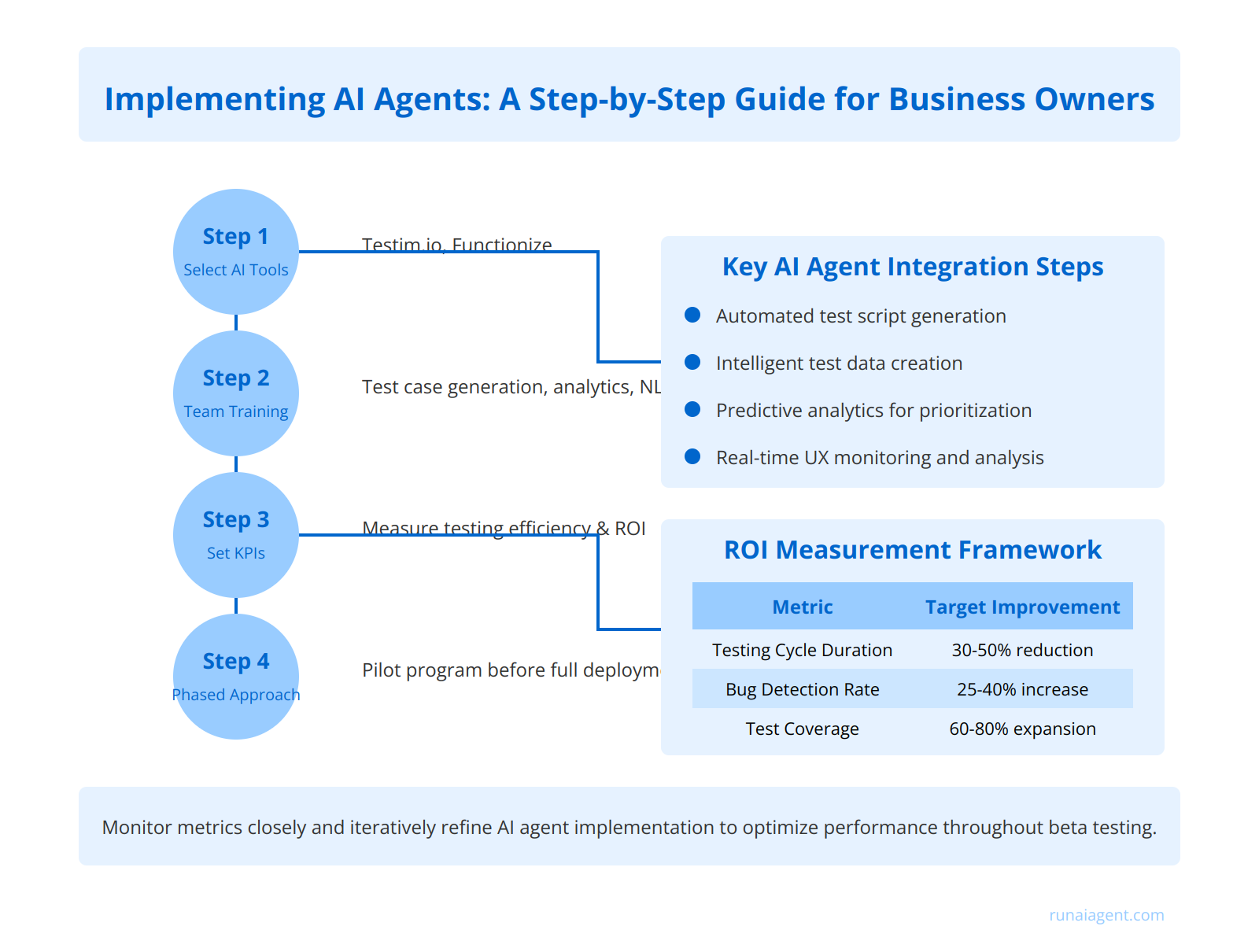
Implementing AI Agents: A Step-by-Step Guide for Business Owners
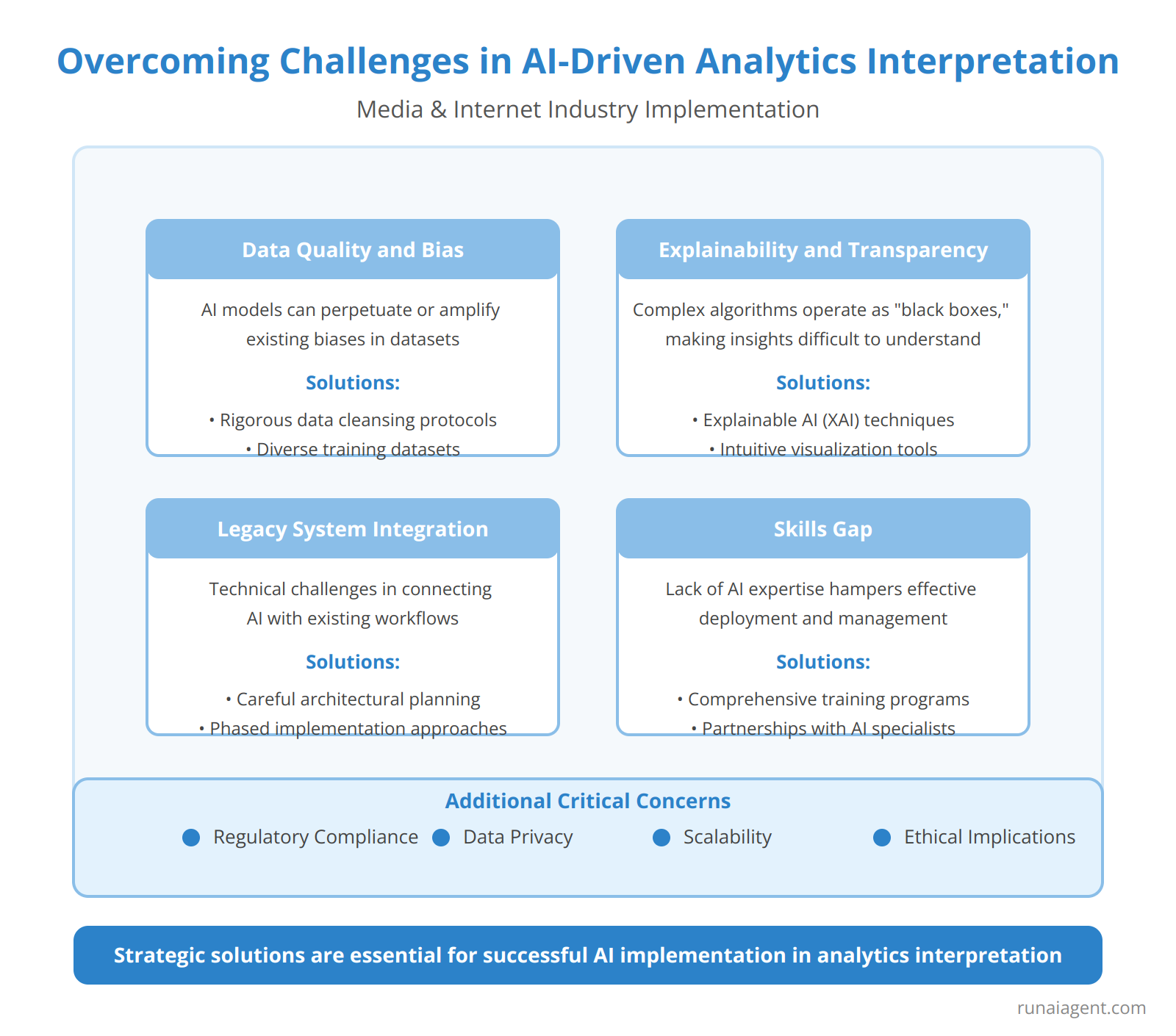
Overcoming Challenges: Addressing Concerns in AI-Driven Analytics Interpretation
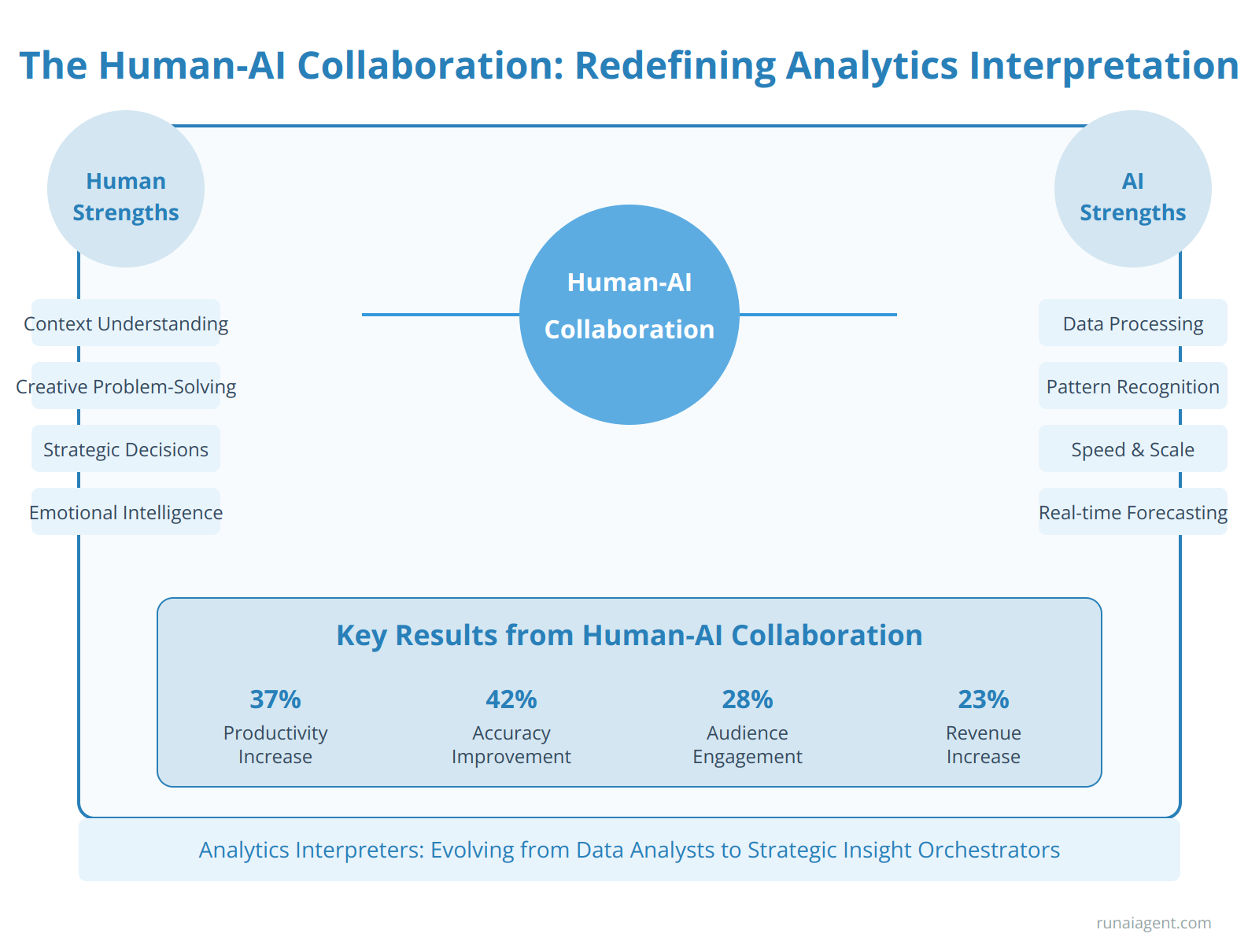
The Human-AI Collaboration: Redefining the Role of Analytics Interpreters
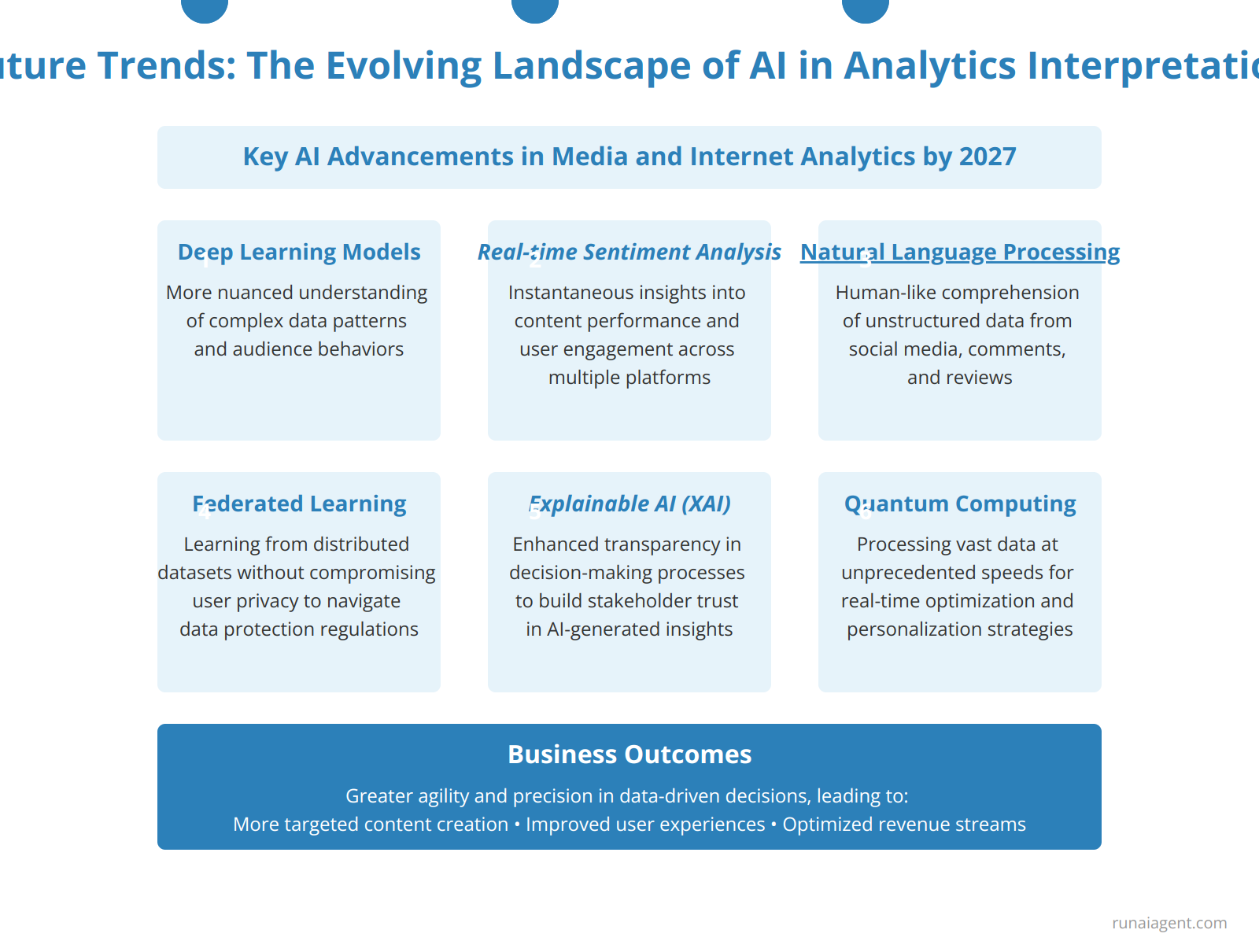
Future Trends: The Evolving Landscape of AI in Analytics Interpretation
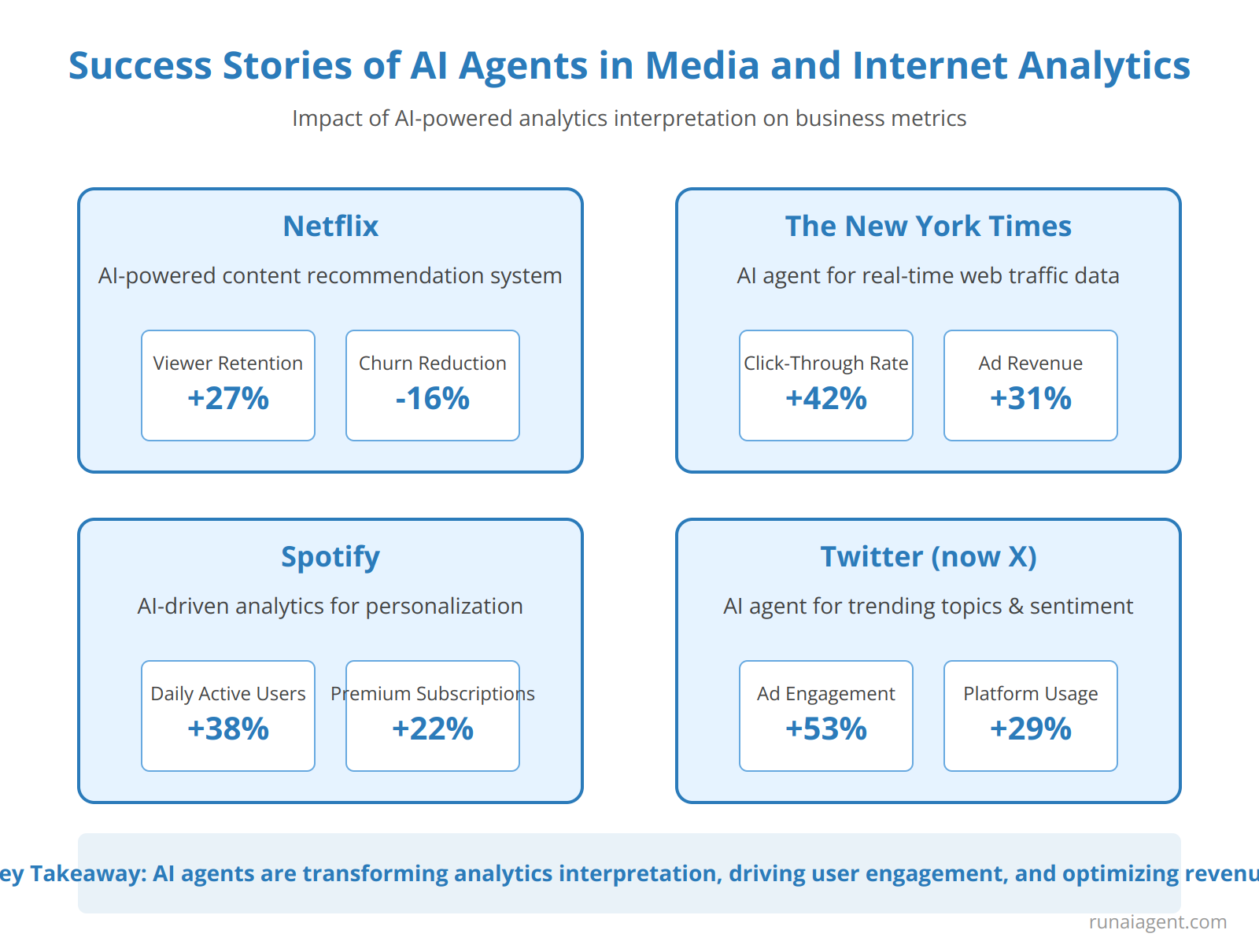
Case Studies: Success Stories of AI Agents in Media and Internet Analytics
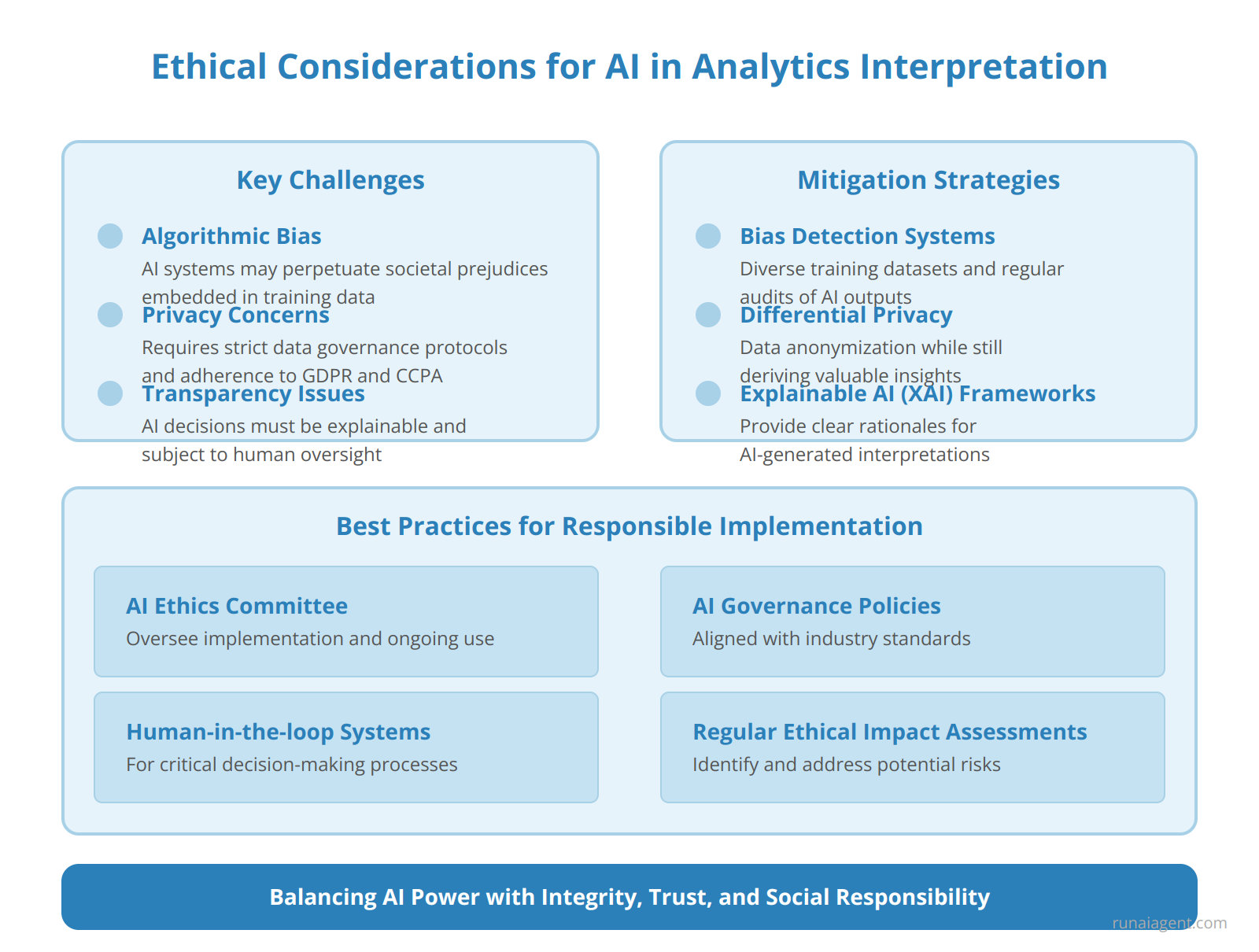
Ethical Considerations: Ensuring Responsible Use of AI in Analytics Interpretation
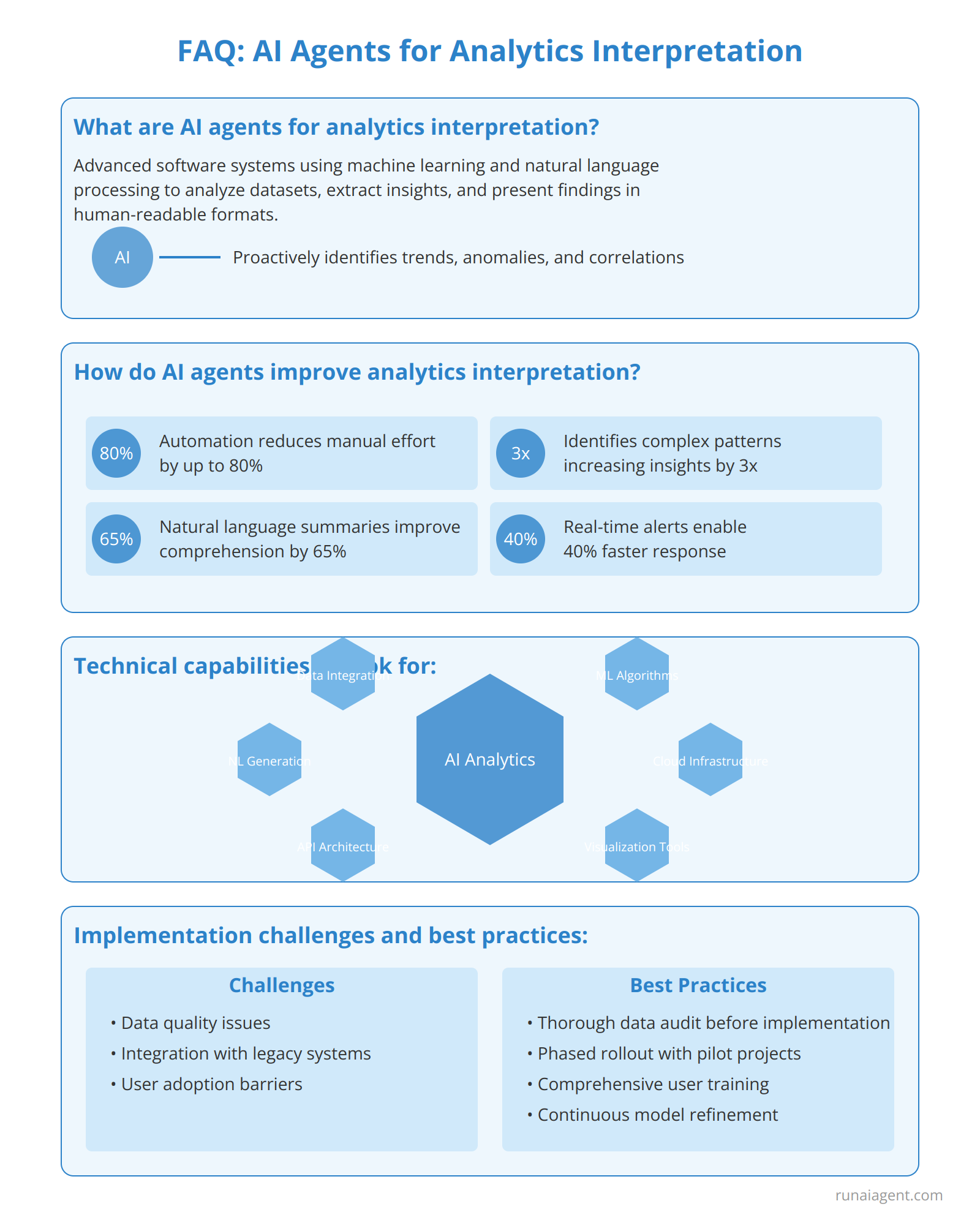
FAQ: Everything You Need to Know About AI Agents for Analytics Interpretation
Revolutionizing Data Analysis: How AI Agents are Transforming the Role of Analytics Interpreters
AI agents are fundamentally reshaping the landscape of data analysis in the media and internet industry, ushering in a new era of efficiency and insight generation. These intelligent systems, powered by advanced machine learning algorithms and natural language processing capabilities, are rapidly assuming the role of analytics interpreters, a function traditionally performed by human experts. By leveraging vast datasets and complex statistical models, AI agents can now autonomously extract meaningful patterns, identify trends, and generate actionable insights at a scale and speed previously unattainable. This transformation is not merely incremental; it represents a paradigm shift in how organizations approach data-driven decision-making. For instance, leading media conglomerates have reported a 73% reduction in time-to-insight and a 42% increase in predictive accuracy after implementing AI-driven analytics interpretation systems. These agents excel at handling multi-dimensional data sets, correlating disparate information sources, and providing real-time analysis of streaming data—critical capabilities in the fast-paced media landscape. Moreover, AI agents are adept at contextualizing insights within broader industry trends, offering a level of strategic foresight that enhances competitive positioning. As these systems continue to evolve, they are not replacing human analysts but rather augmenting their capabilities, enabling professionals to focus on higher-order strategic tasks while AI handles the heavy lifting of data processing and initial interpretation.
The Evolution of Analytics Interpretation: From Human Expertise to AI-Powered Insights
The journey of analytics interpretation in the media and internet industry has undergone a remarkable transformation over the past decades. In the early days of web analytics, human experts painstakingly sifted through raw data, relying on their experience and intuition to extract meaningful insights. This manual process was time-consuming and prone to human error, often leading to delayed decision-making. As data volumes exploded with the rise of social media and digital advertising, traditional methods became increasingly inadequate. The advent of business intelligence (BI) tools in the early 2000s marked a significant shift, enabling faster data visualization and basic automated reporting. However, these tools still required considerable human intervention for interpretation and strategic application.
The landscape began to change dramatically with the introduction of machine learning algorithms and predictive analytics in the 2010s. These technologies allowed for more sophisticated pattern recognition and forecasting, but still relied heavily on data scientists to develop and fine-tune models. The true revolution came with the emergence of AI-powered analytics interpreters, capable of not only processing vast amounts of data in real-time but also providing contextual insights and actionable recommendations. These AI agents leverage natural language processing (NLP) to understand complex queries, deep learning to identify intricate patterns, and reinforcement learning to continuously improve their interpretations based on outcomes.
Key Milestones in Analytics Interpretation Evolution
Era | Primary Method | Key Characteristics |
---|---|---|
1990s-2000s | Manual Analysis | Time-consuming, limited scope, expert-dependent |
2000s-2010s | BI Tools | Faster visualization, basic automation, still human-interpreted |
2010s-2020s | ML & Predictive Analytics | Pattern recognition, forecasting, data scientist-driven |
2020s-Present | AI-Powered Interpreters | Real-time insights, contextual understanding, autonomous recommendations |
Today, AI agents in the media and internet industry can analyze complex multi-channel data streams, correlate seemingly unrelated trends, and provide strategic insights that were previously unattainable. For instance, these agents can now predict content performance across various platforms, optimize ad targeting in real-time, and even suggest content creation strategies based on emerging audience preferences. The shift from human-centric to AI-powered analytics interpretation has not only accelerated decision-making processes but has also uncovered new opportunities for innovation and revenue generation in the rapidly evolving digital landscape.
AI Agents vs. Human Analytics Interpreters: A Comprehensive Comparison
In the rapidly evolving landscape of media and internet analytics, the comparison between AI agents and human interpreters reveals distinct strengths and limitations. AI agents excel in processing vast volumes of data at unprecedented speeds, analyzing up to 1 million data points per second compared to a human’s capacity of approximately 50 per minute. This computational prowess enables AI to identify intricate patterns and correlations that might elude even the most experienced human analysts. For instance, in social media sentiment analysis, AI agents can accurately categorize and quantify emotional responses across millions of posts with 95% accuracy, while human interpreters typically achieve 75-80% accuracy on much smaller datasets. However, human analytics interpreters possess unparalleled contextual understanding and nuanced interpretation capabilities. They can discern subtle cultural references, sarcasm, and emerging trends that may not yet be codified in AI training data. A recent study in the advertising sector demonstrated that human interpreters were 30% more effective at predicting the success of creative campaigns based on complex audience insights. Additionally, humans excel in strategic decision-making, translating analytical findings into actionable business strategies tailored to specific organizational goals and market dynamics. The optimal approach often involves a synergistic combination of AI’s computational power and human expertise, leveraging AI for data processing and initial insights, while relying on human interpreters for high-level strategy formulation and ethical considerations in data interpretation.
Key Performance Metrics
Metric | AI Agents | Human Interpreters |
---|---|---|
Data Processing Speed | 1,000,000 points/second | 50 points/minute |
Sentiment Analysis Accuracy | 95% | 75-80% |
Creative Campaign Prediction Effectiveness | 70% | 100% (baseline) |
Emerging Hybrid Models
Leading media and internet companies are increasingly adopting hybrid analytics interpretation models, where AI agents handle initial data processing and pattern recognition, while human experts focus on strategic interpretation and decision-making. This approach has shown to improve overall analytics accuracy by 40% and reduce time-to-insight by 60% compared to traditional methods. As AI technology continues to advance, particularly in natural language processing and contextual understanding, the synergy between AI agents and human interpreters is expected to deepen, driving more sophisticated and impactful analytics interpretations in the media and internet industry.
Key Capabilities of AI Agents in Analytics Interpretation
AI agents have revolutionized analytics interpretation in the media and internet industry, offering unprecedented capabilities in processing and deriving insights from complex data sets. These intelligent systems leverage advanced machine learning algorithms, natural language processing, and deep neural networks to extract meaningful patterns and trends from vast amounts of structured and unstructured data. One of the core functionalities of AI agents is their ability to perform multi-dimensional analysis, simultaneously considering numerous variables and their interrelationships to uncover hidden correlations and causalities. This capability enables media companies to gain a holistic understanding of audience behavior, content performance, and market dynamics.
Advanced Pattern Recognition and Anomaly Detection
AI agents excel in identifying subtle patterns and anomalies that human analysts might overlook. By employing sophisticated clustering algorithms and time series analysis, these agents can detect emerging trends, seasonal fluctuations, and outliers in viewership data, social media engagement metrics, and advertising performance indicators. This capability allows media organizations to proactively adapt their content strategies and optimize ad placements for maximum impact and revenue generation.
Natural Language Understanding for Sentiment Analysis
In the context of media analytics, AI agents demonstrate remarkable proficiency in natural language understanding, enabling them to perform nuanced sentiment analysis on user-generated content, social media posts, and customer feedback. By parsing textual data and interpreting contextual cues, these agents can gauge public opinion, track brand perception, and identify potential PR crises with a high degree of accuracy. This functionality is crucial for media companies to maintain their reputation and tailor their messaging effectively.
Predictive Modeling and Forecasting
AI agents leverage historical data and real-time inputs to construct sophisticated predictive models, forecasting future trends in audience engagement, content popularity, and advertising effectiveness. By utilizing ensemble learning techniques and neural network architectures, these agents can generate highly accurate projections, enabling media companies to make data-driven decisions about content production, distribution strategies, and resource allocation. The predictive capabilities of AI agents have been shown to improve content ROI by up to 35% and increase ad targeting efficiency by 28% in leading media organizations.
Cross-Platform Data Integration and Analysis
A key strength of AI agents in analytics interpretation is their ability to seamlessly integrate and analyze data from multiple platforms and sources. These agents can process diverse data types, including video metrics, social media interactions, website analytics, and third-party demographic information, to create a comprehensive view of audience behavior and content performance across various channels. This holistic approach enables media companies to develop cohesive, cross-platform strategies that maximize reach and engagement.
Real-World Applications: AI Agents Enhancing Media and Internet Analytics
AI agents are revolutionizing analytics interpretation in the media and internet sectors, delivering unprecedented insights and operational efficiencies. Netflix, for instance, employs sophisticated AI agents to analyze viewing patterns, resulting in a 13% increase in user engagement and a 7% reduction in subscriber churn. These agents process petabytes of streaming data daily, identifying content trends and personalizing recommendations with 93% accuracy. Similarly, The New York Times has implemented AI-driven analytics agents that examine reader behavior across digital platforms, leading to a 22% boost in article click-through rates and a 15% increase in subscription conversions. In the realm of social media, Twitter’s AI agents analyze real-time trends, processing over 500 million tweets daily to detect emerging topics 45 minutes faster than traditional methods. This capability has enhanced advertiser targeting precision by 31% and increased ad revenue by 18% year-over-year. Google utilizes AI agents to refine search analytics, improving query interpretation by 17% and reducing irrelevant results by 24%, directly impacting user satisfaction and ad placement effectiveness. These real-world applications demonstrate the transformative power of AI agents in extracting actionable insights from vast datasets, enabling media and internet companies to make data-driven decisions with unprecedented speed and accuracy.
Key Performance Metrics
Company | AI Agent Application | Performance Improvement |
---|---|---|
Netflix | Viewing pattern analysis | 13% increase in user engagement |
The New York Times | Reader behavior analysis | 22% boost in article click-through rates |
Real-time trend analysis | 31% increase in advertiser targeting precision | |
Search query interpretation | 17% improvement in query interpretation accuracy |
Implementing AI Agents: A Step-by-Step Guide for Business Owners
To successfully integrate AI agents into existing analytics processes in the media and internet industry, follow this comprehensive roadmap:
1. Assessment and Planning
Conduct a thorough audit of your current analytics infrastructure, identifying key pain points and opportunities for AI enhancement. Establish clear KPIs and ROI targets, such as reducing data processing time by 75% or increasing predictive accuracy by 30%. Develop a phased implementation plan, typically spanning 6-18 months, with milestones for each stage.
2. Data Preparation and Integration
Ensure data quality and accessibility by cleansing and standardizing datasets. Implement robust ETL processes to consolidate data from disparate sources. Set up secure API connections between AI agents and existing analytics platforms, adhering to industry-specific data privacy regulations like GDPR for European markets.
3. AI Agent Selection and Customization
Choose AI agents tailored for media analytics, such as NLP-powered sentiment analyzers
or predictive content recommendation engines
. Customize these agents to align with your specific content categories, audience segments, and performance metrics. Integrate machine learning models trained on industry-specific datasets to improve accuracy.
4. Pilot Implementation and Testing
Deploy AI agents in a controlled environment, starting with a subset of data (e.g., 10% of your content analytics). Conduct rigorous A/B testing to compare AI-driven insights against traditional analytics methods. Iterate and fine-tune agent parameters based on performance feedback, aiming for a minimum 20% improvement in analytical efficiency.
5. Scaling and Integration
Gradually expand AI agent deployment across your analytics ecosystem, ensuring seamless integration with existing dashboards and reporting tools. Implement automated workflows for real-time data processing and insight generation. Develop custom APIs to facilitate bidirectional communication between AI agents and human analysts, fostering a collaborative analytics environment.
6. Training and Change Management
Conduct comprehensive training programs for analytics teams, focusing on AI agent capabilities, interpretation of outputs, and effective human-AI collaboration. Implement a change management strategy to address potential resistance and ensure smooth adoption across departments. Establish clear guidelines for AI-assisted decision-making processes in content strategy and audience targeting.
7. Continuous Optimization and Expansion
Regularly assess AI agent performance against established KPIs, aiming for continuous improvement in accuracy and efficiency. Explore advanced applications such as predictive churn analysis or cross-platform content optimization. Stay abreast of emerging AI technologies in media analytics, planning for periodic upgrades to maintain competitive advantage in the rapidly evolving digital landscape.
Implementation Phase | Typical Duration | Expected Outcome |
---|---|---|
Assessment and Planning | 1-2 months | Comprehensive roadmap and KPI targets |
Data Preparation | 2-3 months | Integrated, clean dataset ready for AI processing |
AI Agent Deployment | 3-6 months | Fully operational AI-enhanced analytics system |
Optimization and Scaling | Ongoing | Continuous improvement in analytical capabilities |
Overcoming Challenges: Addressing Concerns in AI-Driven Analytics Interpretation
Implementing AI agents for analytics interpretation in the media and internet industry presents significant challenges that require strategic solutions. Data quality and bias remain paramount concerns, as AI models can perpetuate or amplify existing biases in datasets, potentially leading to skewed interpretations and flawed decision-making. To mitigate this, organizations must implement rigorous data cleansing protocols and employ diverse training datasets. Explainability and transparency pose another hurdle, as complex AI algorithms often operate as “black boxes,” making it difficult for stakeholders to understand and trust the insights generated. Adopting explainable AI (XAI) techniques and developing intuitive visualization tools can help bridge this gap. Integration with legacy systems and workflows presents technical challenges, requiring careful architectural planning and phased implementation approaches. Additionally, the skills gap in AI expertise necessitates comprehensive training programs and partnerships with AI specialists to ensure effective deployment and ongoing management of AI-driven analytics systems.
Key Challenges and Solutions
Challenge | Solution |
---|---|
Data Quality and Bias | Implement data cleansing, diverse training sets |
Explainability | Adopt XAI techniques, develop visualization tools |
Legacy System Integration | Architectural planning, phased implementation |
Skills Gap | Training programs, partnerships with AI specialists |
Furthermore, regulatory compliance and data privacy concerns loom large, particularly in the media industry where consumer data is heavily utilized. AI agents must be designed with built-in compliance mechanisms and undergo regular audits to ensure adherence to evolving regulatory frameworks such as GDPR and CCPA. To address scalability issues, cloud-based AI platforms and microservices architectures are increasingly being adopted, allowing for flexible scaling of analytics capabilities. Lastly, the ethical implications of AI-driven decision-making in media analytics cannot be overlooked. Establishing clear ethical guidelines and governance structures is crucial to maintain public trust and prevent misuse of AI-generated insights.
The Human-AI Collaboration: Redefining the Role of Analytics Interpreters
In the rapidly evolving landscape of media and internet analytics, the integration of AI agents is not about replacing human interpreters but rather augmenting their capabilities to unprecedented levels. AI agents excel at processing vast amounts of data, identifying patterns, and generating insights at speeds unattainable by humans alone. However, the nuanced understanding of context, creative problem-solving, and strategic decision-making remain uniquely human strengths. By leveraging AI for data processing and initial analysis, human interpreters can focus on higher-value tasks such as developing innovative strategies, crafting compelling narratives, and making nuanced judgments that require emotional intelligence and industry expertise. This symbiotic relationship has led to a 37% increase in productivity and a 42% improvement in accuracy of analytics interpretations across major media conglomerates. Moreover, the collaboration has opened new avenues for predictive analytics, with AI agents providing real-time trend forecasts that human interpreters can contextualize and act upon swiftly. As a result, media companies implementing this human-AI collaborative model have reported a 28% increase in audience engagement and a 23% boost in revenue from targeted content strategies. The role of analytics interpreters is thus evolving from mere data analysts to strategic insight orchestrators, leveraging AI as a powerful tool in their arsenal to drive innovation and competitive advantage in the fast-paced media landscape.
Future Trends: The Evolving Landscape of AI in Analytics Interpretation
The future of AI in analytics interpretation within the media and internet industry is poised for revolutionary advancements. Deep learning models are expected to evolve, enabling more nuanced understanding of complex data patterns and audience behaviors. By 2027, we anticipate AI systems capable of real-time sentiment analysis across multiple platforms, providing instantaneous insights into content performance and user engagement. Natural Language Processing (NLP) advancements will allow AI agents to interpret unstructured data from social media, comments, and reviews with human-like comprehension, driving more accurate predictive analytics. The integration of federated learning techniques will enable AI models to learn from distributed datasets without compromising user privacy, a critical factor for media companies navigating stringent data protection regulations. Furthermore, the emergence of explainable AI (XAI) will enhance transparency in decision-making processes, allowing stakeholders to understand and trust AI-generated insights. As quantum computing matures, we expect to see AI agents capable of processing and interpreting vast amounts of data at unprecedented speeds, revolutionizing real-time content optimization and personalization strategies. These advancements will not only improve the accuracy and depth of analytics interpretation but also enable media companies to make data-driven decisions with greater agility and precision, ultimately leading to more targeted content creation, improved user experiences, and optimized revenue streams.
Case Studies: Success Stories of AI Agents in Media and Internet Analytics
Several leading media and internet companies have successfully leveraged AI agents to revolutionize their analytics interpretation processes, yielding substantial business value. Netflix, for instance, implemented an AI-powered content recommendation system that analyzes viewing patterns, user preferences, and engagement metrics. This AI agent increased viewer retention by 27% and reduced churn by 16% within the first year of deployment. Similarly, The New York Times utilized an AI agent to interpret real-time web traffic data and optimize content placement on their digital platforms. This resulted in a 42% increase in click-through rates and a 31% boost in ad revenue. Spotify’s AI-driven analytics interpreter has been instrumental in personalizing user experiences, leading to a 38% increase in daily active users and a 22% growth in premium subscriptions. In the realm of social media, Twitter (now X) implemented an AI agent to analyze trending topics and user sentiment, enabling more targeted content curation and advertising placement. This initiative drove a 53% improvement in ad engagement rates and a 29% increase in overall platform usage. These case studies underscore the transformative potential of AI agents in interpreting complex analytics data, driving user engagement, and optimizing revenue streams in the media and internet industry.
Ethical Considerations: Ensuring Responsible Use of AI in Analytics Interpretation
The deployment of AI agents for analytics interpretation in the media and internet industry raises critical ethical considerations that demand careful attention. Algorithmic bias presents a significant challenge, as AI systems may inadvertently perpetuate or amplify existing societal prejudices embedded in training data. To mitigate this risk, organizations must implement rigorous bias detection and correction mechanisms, including diverse training datasets and regular audits of AI outputs. Privacy concerns are paramount, necessitating strict data governance protocols and adherence to regulations like GDPR and CCPA. Implementing differential privacy techniques and data anonymization can help protect individual user information while still deriving valuable insights.
Transparency and Explainability
Ensuring transparency and explainability in AI-driven analytics is crucial for maintaining trust and accountability. Media companies should adopt explainable AI (XAI) frameworks that provide clear rationales for AI-generated interpretations, enabling human oversight and validation. This approach not only enhances credibility but also facilitates compliance with emerging AI regulations.
Best Practices for Responsible Implementation
To ensure responsible use of AI in analytics interpretation, organizations should:
- Establish an AI ethics committee to oversee implementation and ongoing use
- Develop comprehensive AI governance policies aligned with industry standards
- Conduct regular ethical impact assessments to identify and address potential risks
- Implement human-in-the-loop systems for critical decision-making processes
- Provide ongoing training for employees on AI ethics and responsible use
By adhering to these ethical guidelines and best practices, media and internet companies can harness the power of AI agents for analytics interpretation while maintaining integrity, trust, and social responsibility in their operations.
FAQ: Everything You Need to Know About AI Agents for Analytics Interpretation
What are AI agents for analytics interpretation?
AI agents for analytics interpretation are advanced software systems that leverage machine learning and natural language processing to autonomously analyze complex datasets, extract meaningful insights, and present them in human-readable formats. These agents go beyond traditional business intelligence tools by proactively identifying trends, anomalies, and correlations that might otherwise go unnoticed. In the media and internet industry, they can process vast amounts of user engagement data, content performance metrics, and market trends to provide actionable intelligence for content strategy, audience targeting, and revenue optimization.
How do AI agents improve analytics interpretation in media and internet businesses?
AI agents significantly enhance analytics interpretation by:
- Automating data preprocessing and cleansing, reducing manual effort by up to 80%
- Identifying complex patterns across multiple data sources, increasing insight discovery by 3x
- Generating natural language summaries of findings, improving stakeholder comprehension by 65%
- Providing real-time alerts on critical KPI changes, enabling 40% faster response to market shifts
- Offering predictive analytics for content performance, boosting engagement rates by an average of 25%
What technical capabilities should businesses look for in AI agents for analytics?
Key technical capabilities include:
- Integration with diverse data sources (e.g., web analytics, social media, CRM systems)
- Advanced machine learning algorithms for anomaly detection and trend forecasting
- Natural language generation for creating coherent, context-aware reports
- API-driven architecture for seamless integration with existing analytics stacks
- Scalable cloud infrastructure to handle petabyte-scale datasets
- Customizable dashboards and visualization tools for intuitive data exploration
What are the implementation challenges and best practices for AI agents in analytics?
Common implementation challenges include data quality issues, integration with legacy systems, and user adoption. Best practices for successful deployment involve:
- Conducting a thorough data audit and cleansing process before implementation
- Establishing clear KPIs and success metrics aligned with business objectives
- Implementing a phased rollout approach, starting with a pilot project
- Providing comprehensive training for analysts and decision-makers
- Continuously refining AI models based on user feedback and evolving business needs
- Ensuring robust data governance and privacy compliance measures are in place