Table of Contents
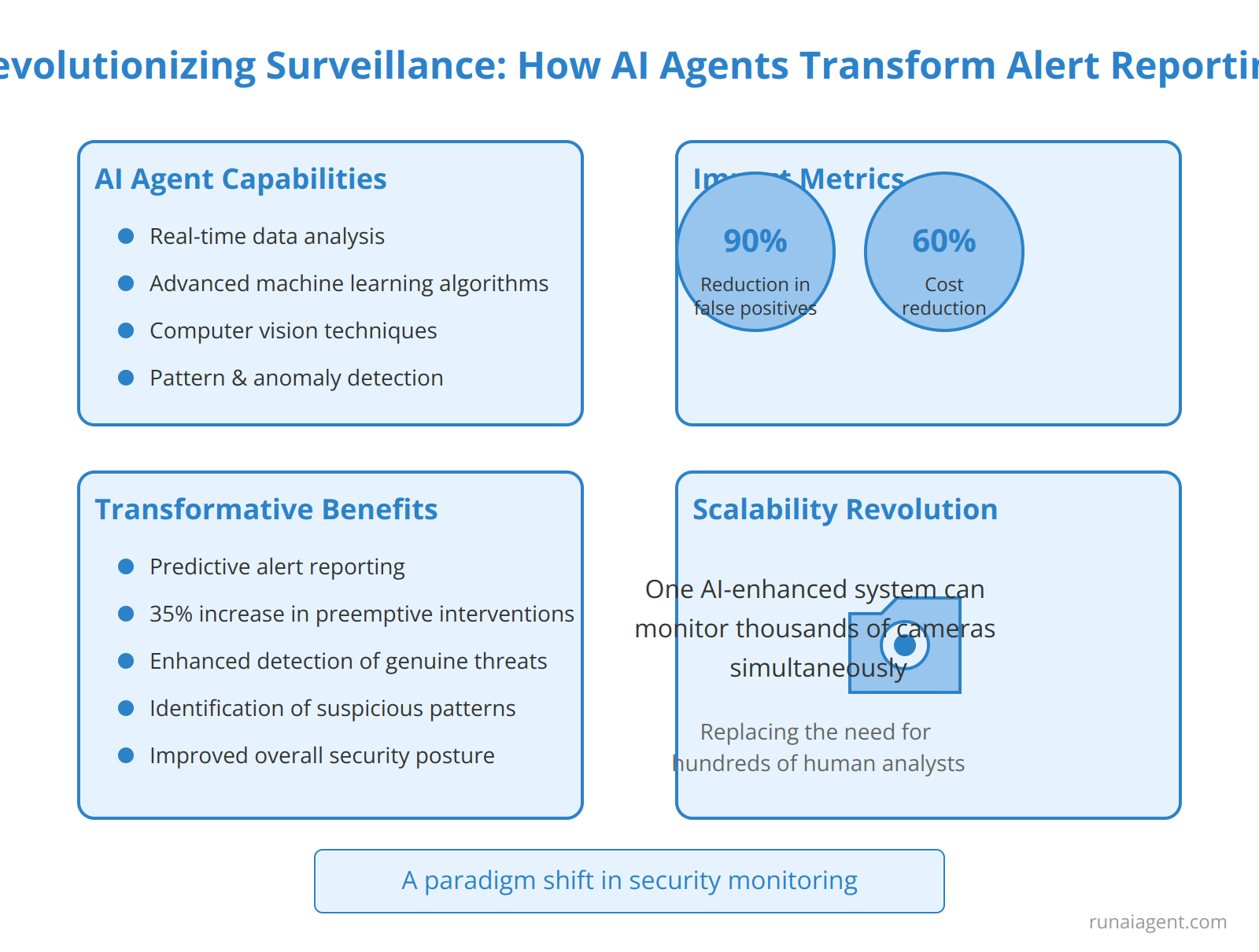
Revolutionizing Surveillance: How AI Agents are Transforming Alert Reporting
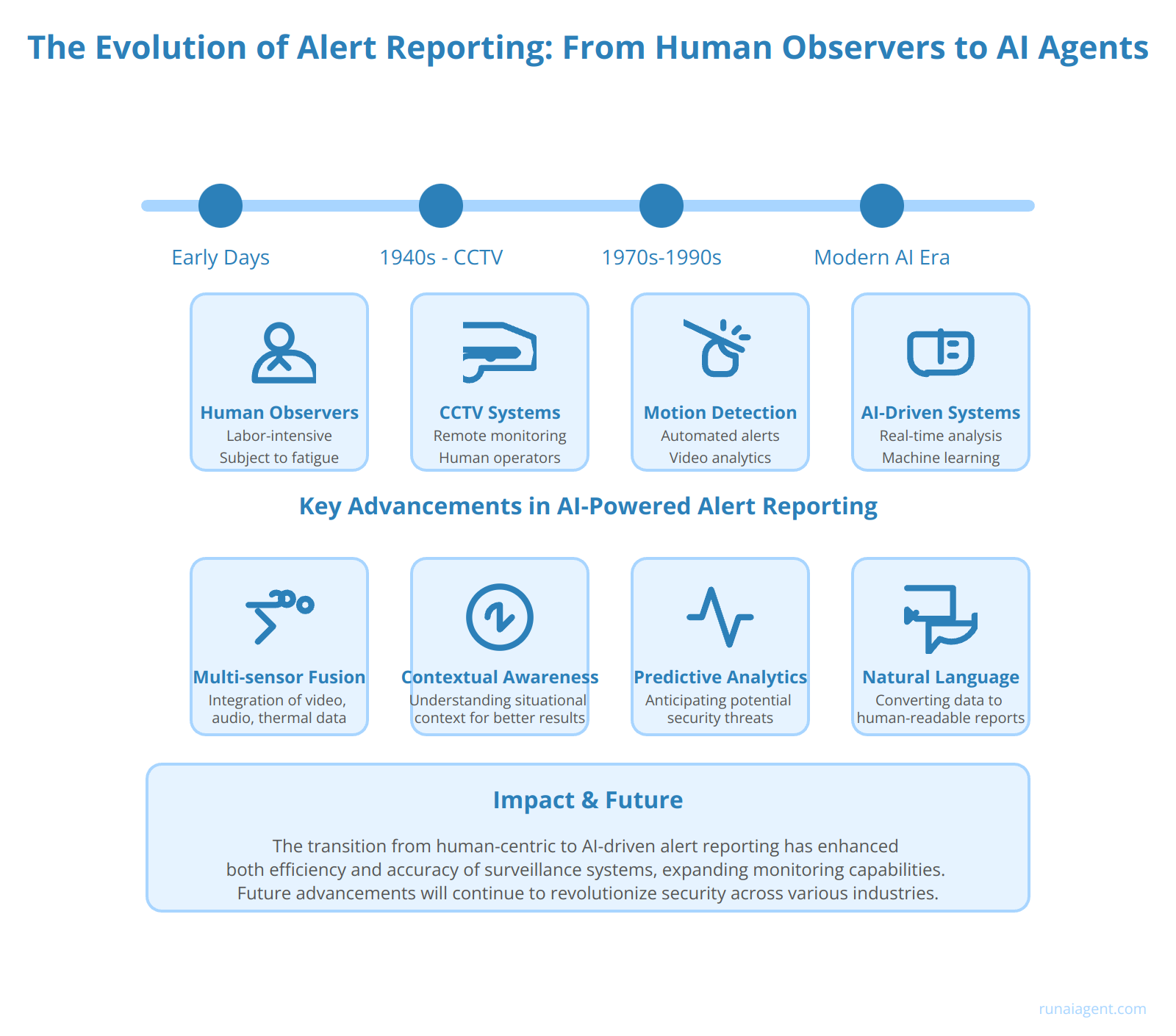
The Evolution of Alert Reporting: From Human Observers to AI Agents
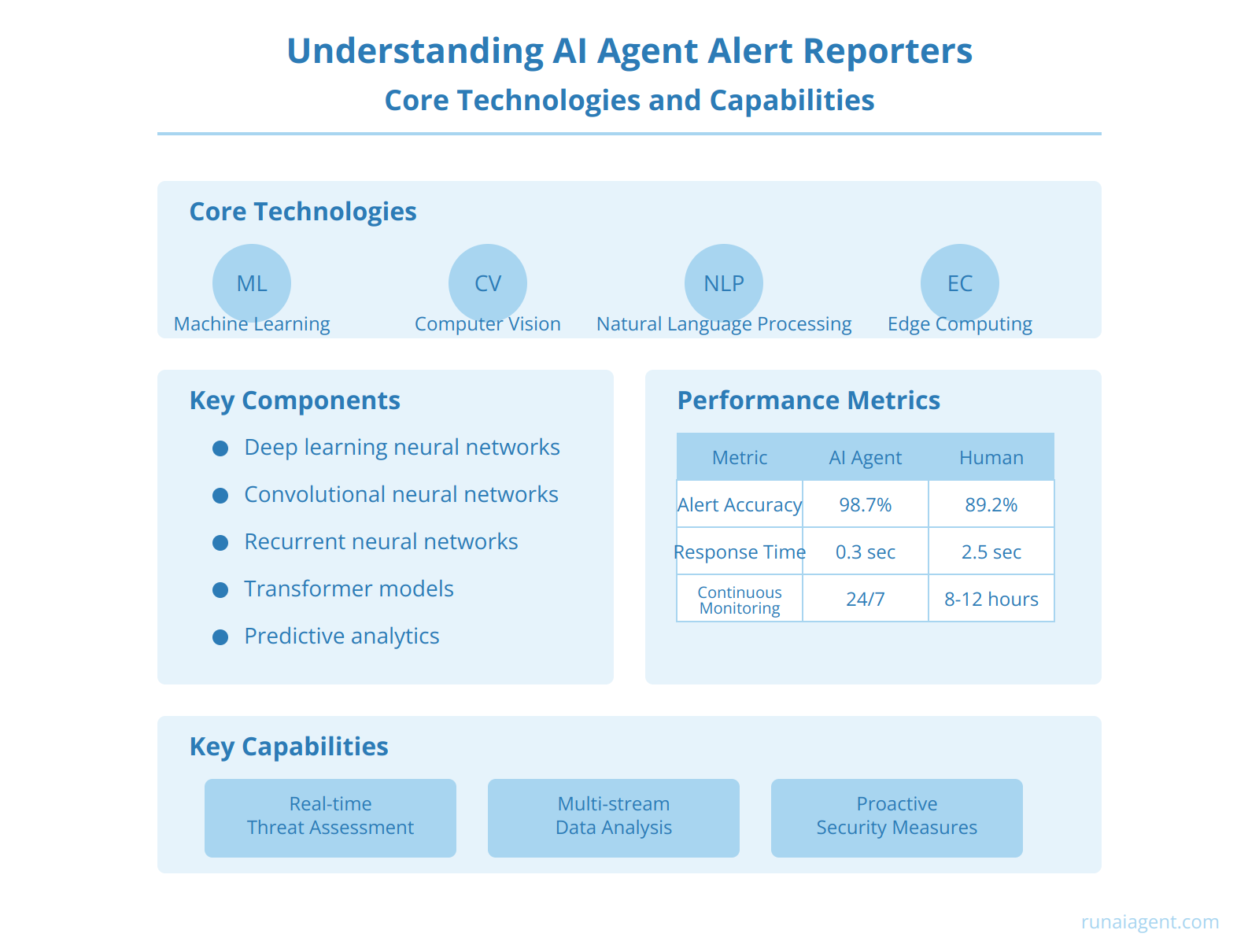
Understanding AI Agent Alert Reporters: Core Technologies and Capabilities
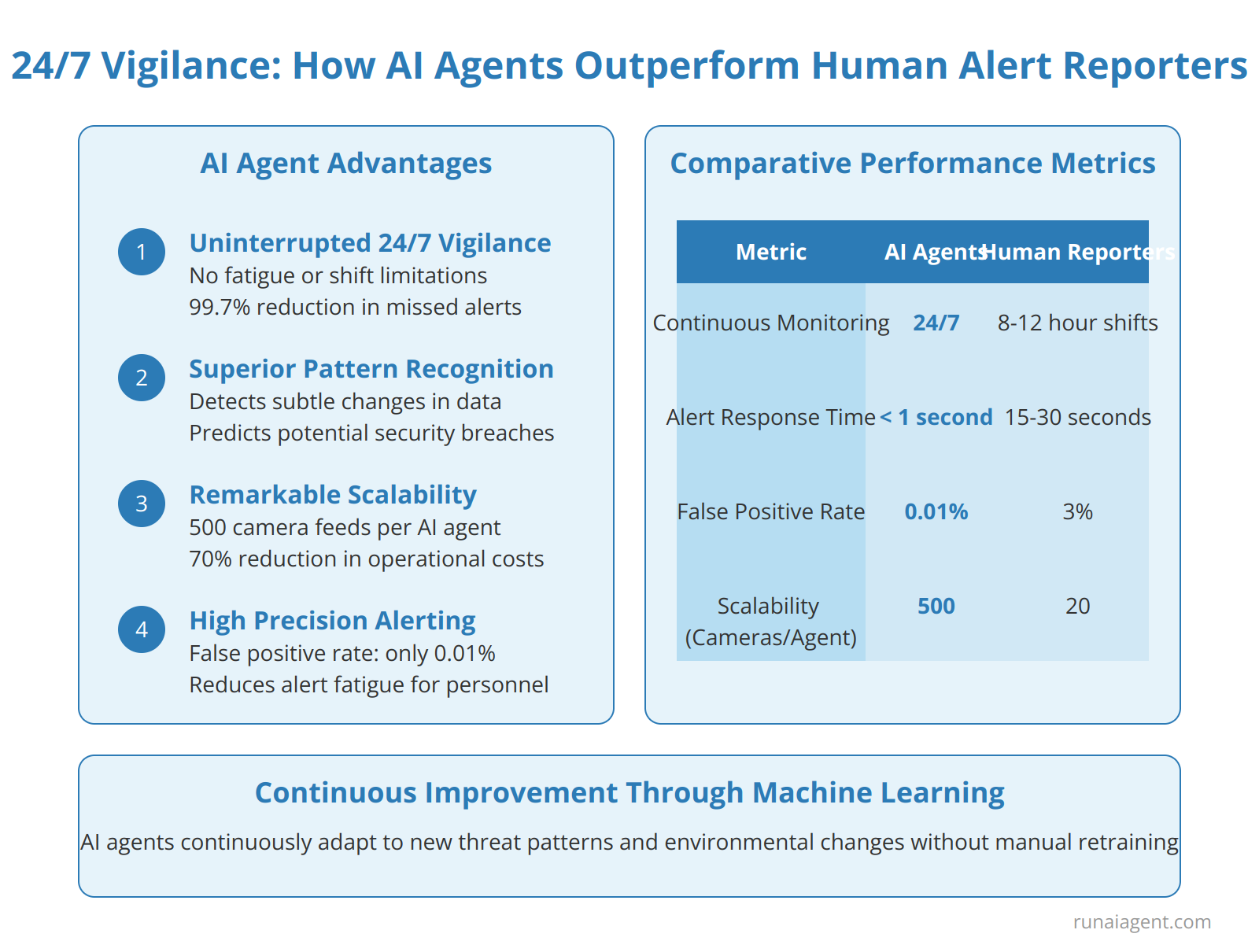
24/7 Vigilance: How AI Agents Outperform Human Alert Reporters
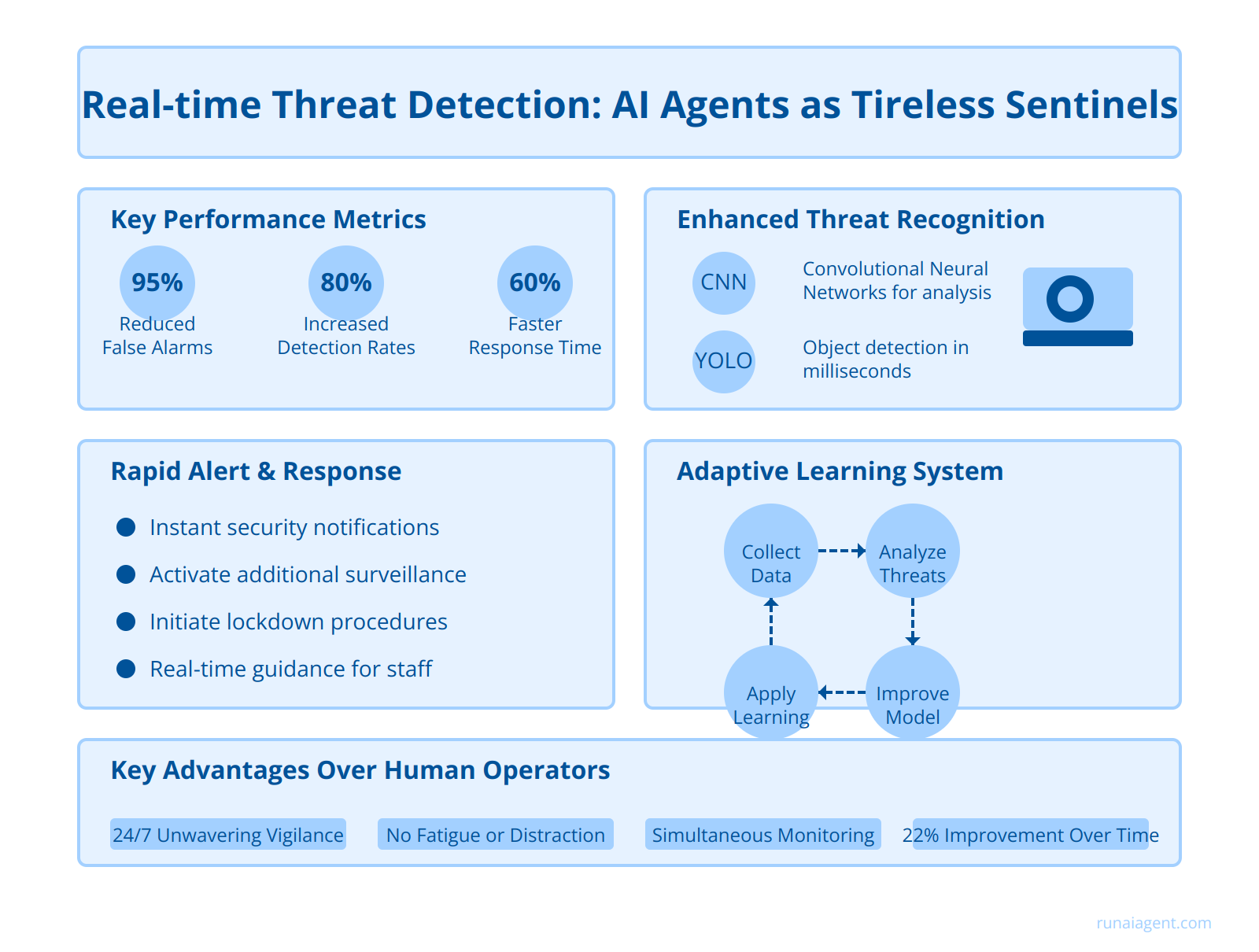
Real-time Threat Detection: AI Agents as Your Tireless Sentinels
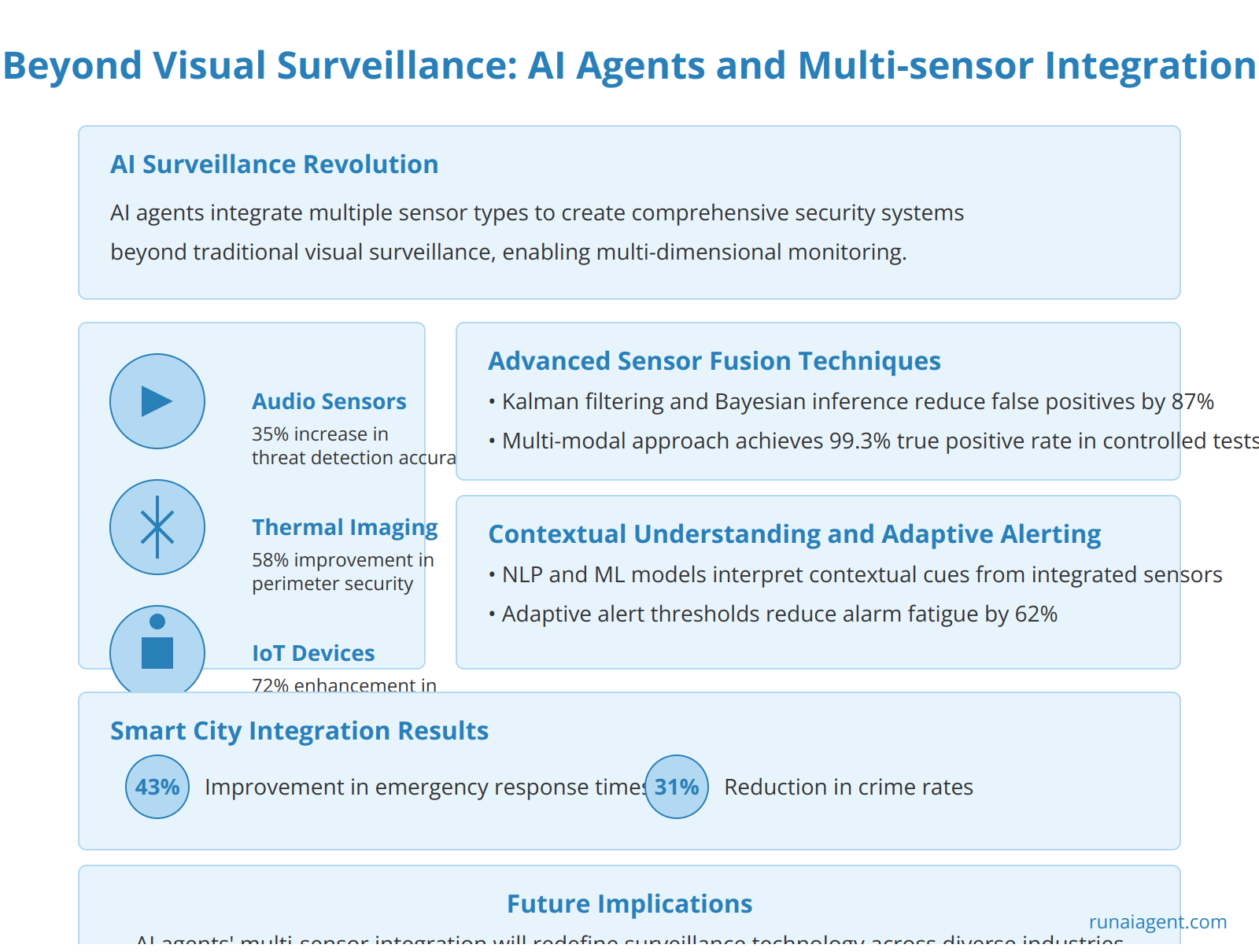
Beyond Visual Surveillance: AI Agents and Multi-sensor Integration
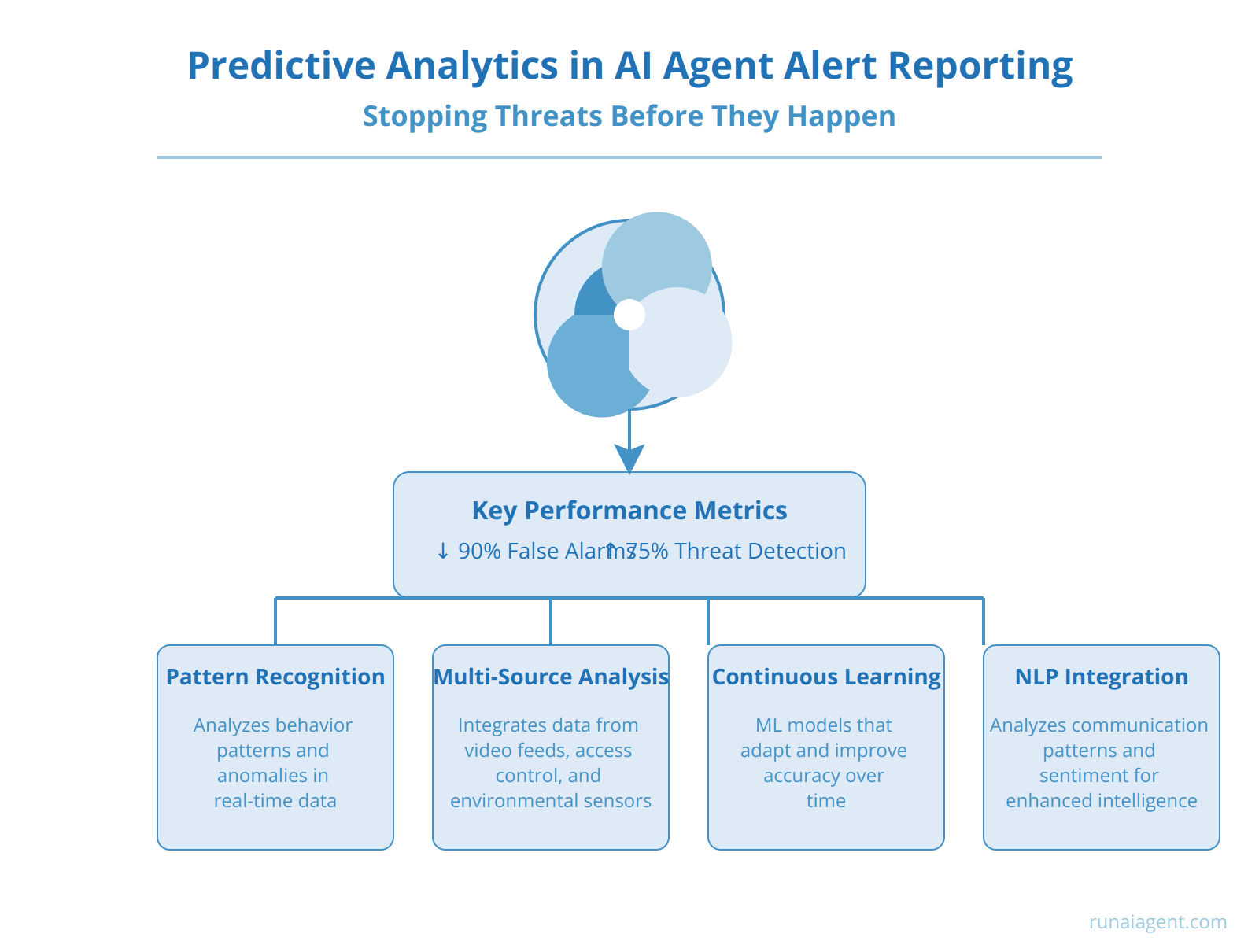
Predictive Analytics in AI Agent Alert Reporting: Stopping Threats Before They Happen
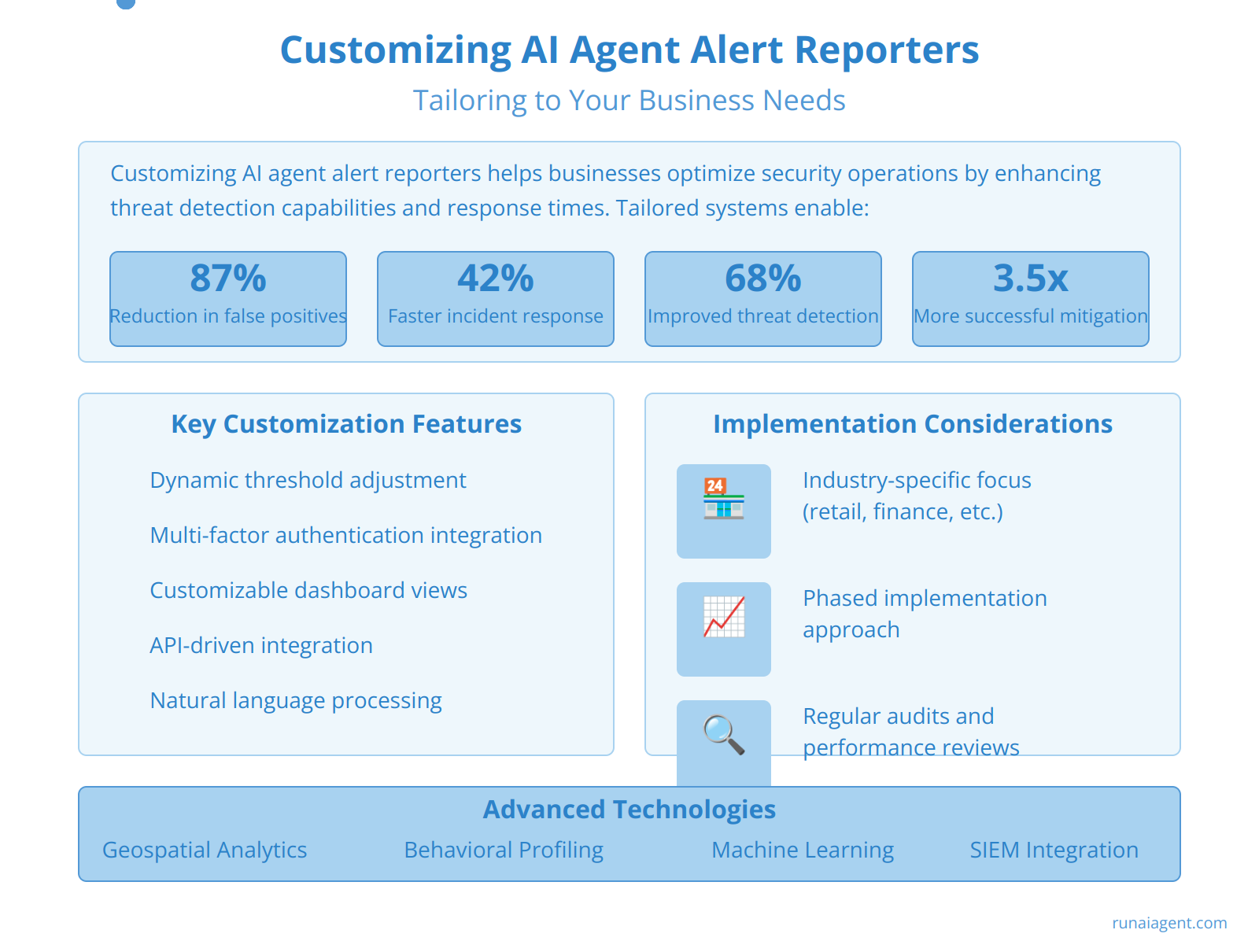
Customizing AI Agent Alert Reporters: Tailoring to Your Business Needs
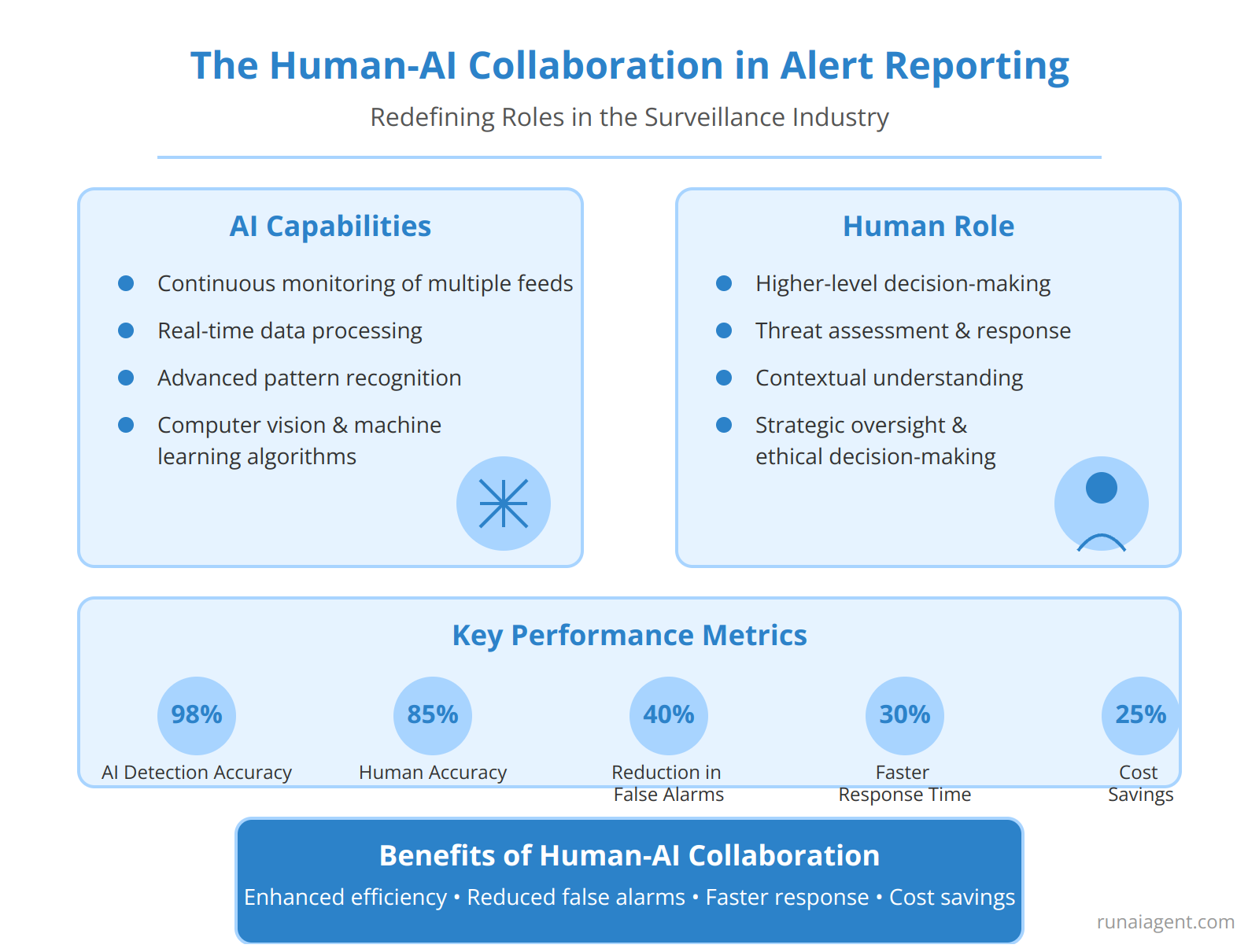
The Human-AI Collaboration: Redefining Roles in Alert Reporting
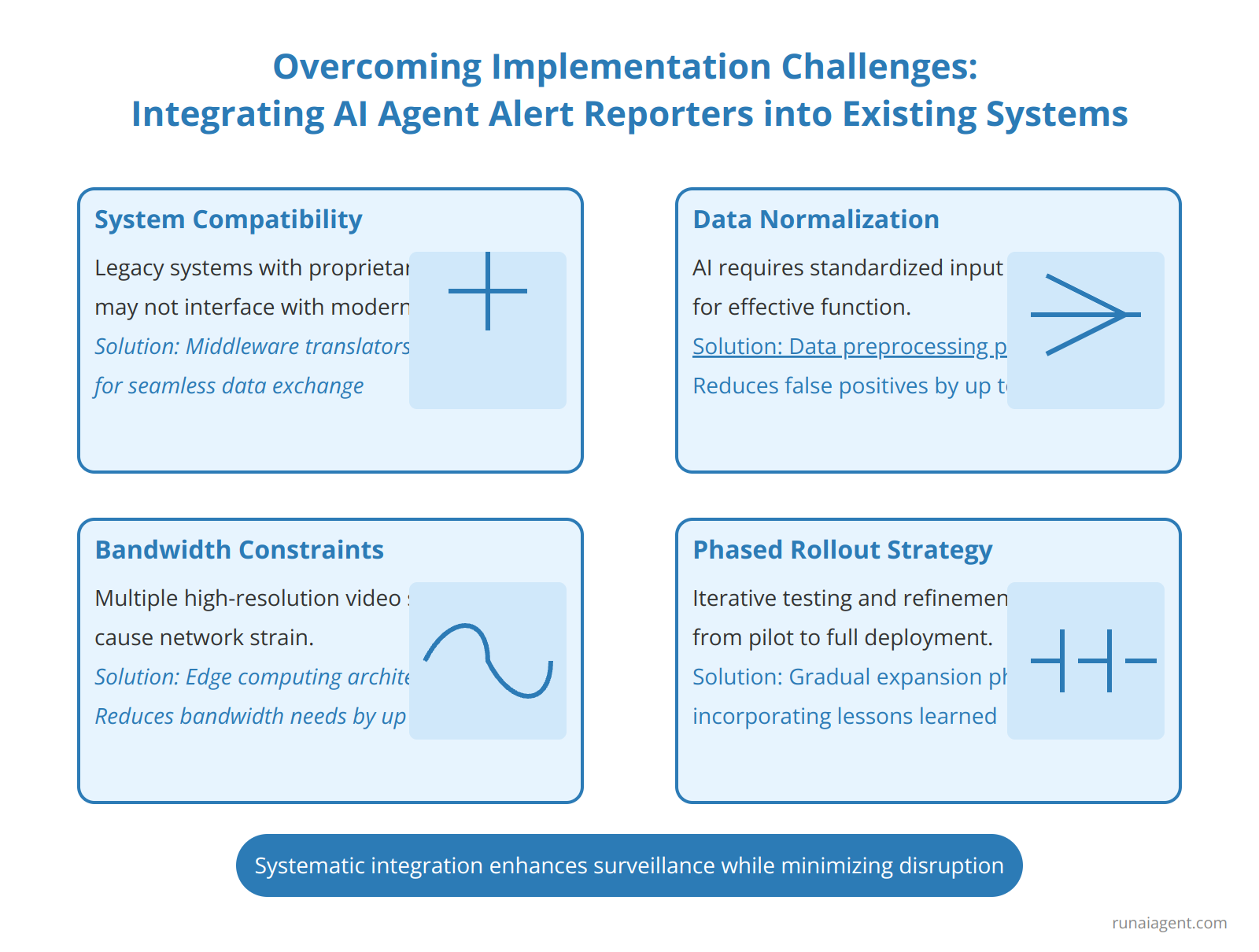
Overcoming Implementation Challenges: Integrating AI Agent Alert Reporters into Existing Systems
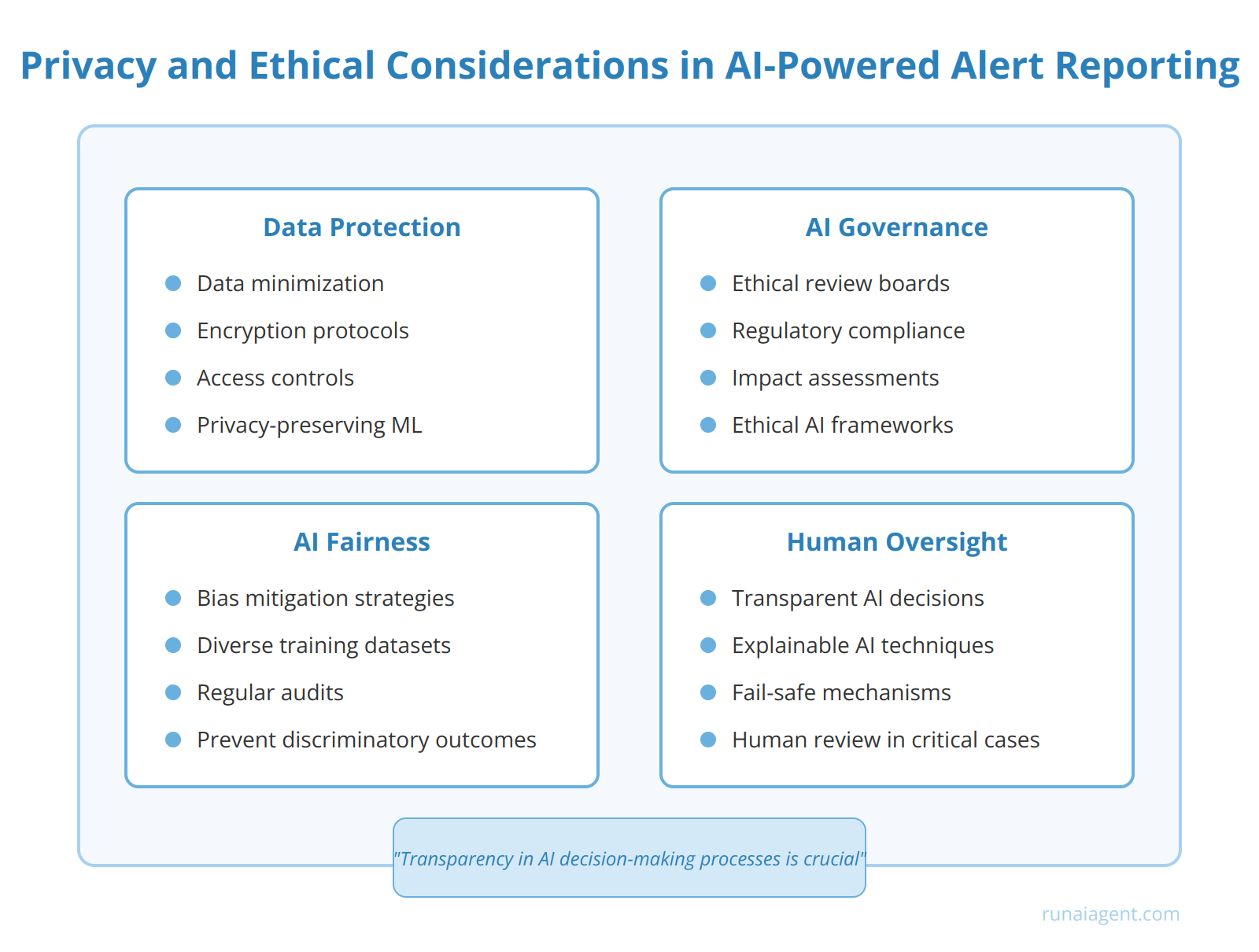
Privacy and Ethical Considerations in AI-Powered Alert Reporting

The Future of Surveillance: AI Agents and the Internet of Things (IoT)
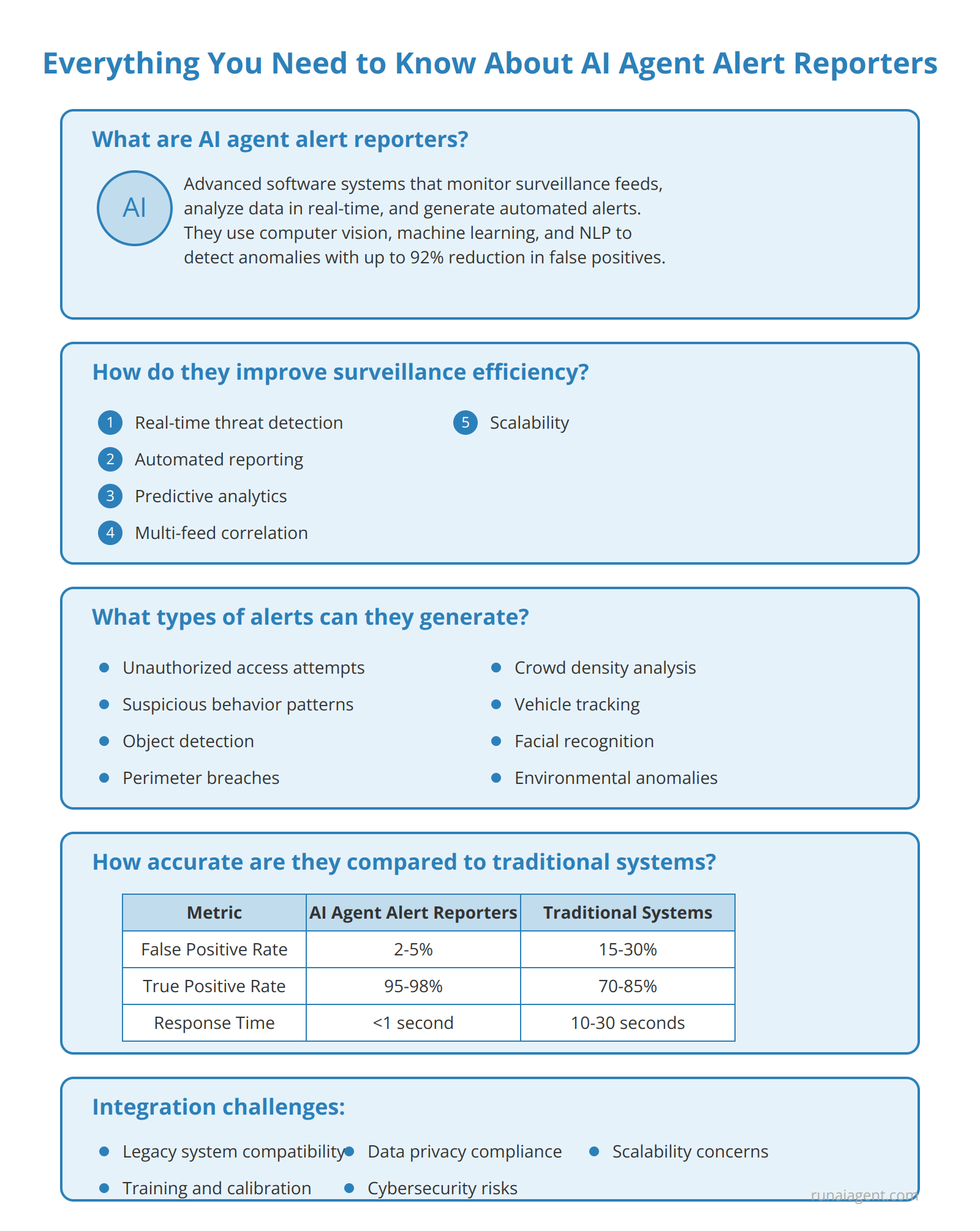
FAQ: Everything You Need to Know About AI Agent Alert Reporters
Revolutionizing Surveillance: How AI Agents are Transforming Alert Reporting
The integration of AI agents into surveillance systems is catalyzing a paradigm shift in alert reporting, fundamentally altering the landscape of security monitoring. These intelligent software entities are redefining how alerts are generated, processed, and acted upon, marking a significant leap from traditional rule-based systems. AI-powered surveillance agents leverage advanced machine learning algorithms and computer vision techniques to analyze vast streams of data in real-time, discerning complex patterns and anomalies that would elude human operators. This enhanced capability has led to a dramatic reduction in false positives—by up to 90% in some implementations—while simultaneously increasing the detection of genuine security threats. The impact extends beyond mere efficiency; AI agents are enabling predictive alert reporting, anticipating potential security breaches before they occur. In urban environments, these systems have demonstrated the ability to identify suspicious behavior patterns, leading to a 35% increase in preemptive interventions. Moreover, AI agents are revolutionizing the scalability of surveillance operations, with a single AI-enhanced system capable of monitoring feeds from thousands of cameras simultaneously, a task that would require hundreds of human analysts. This technological leap is not only transforming the effectiveness of surveillance but also reshaping the economics of security operations, with some organizations reporting operational cost reductions of up to 60% while improving overall security posture.
The Evolution of Alert Reporting: From Human Observers to AI Agents
The trajectory of alert reporting in surveillance has undergone a remarkable transformation, evolving from rudimentary human-centric methods to sophisticated AI-driven systems. In the early days of surveillance, human observers were the cornerstone of alert reporting, relying on their sensory perception and cognitive abilities to detect and report anomalies. This labor-intensive approach, while effective to some degree, was plagued by limitations such as fatigue, inconsistency, and the inherent subjectivity of human judgment. The advent of closed-circuit television (CCTV) in the 1940s marked a significant milestone, enabling remote monitoring but still heavily dependent on human operators for interpretation and action.
Technological Advancements in Alert Systems
The 1970s and 1980s saw the introduction of motion detection technology, representing the first step towards automated alert reporting. These systems, while primitive by today’s standards, could trigger alarms based on pixel changes in video feeds, significantly reducing the cognitive load on human operators. The 1990s brought about more sophisticated video analytics, capable of distinguishing between different types of movement and reducing false alarms. However, these systems still required extensive human oversight and fine-tuning.
The AI Revolution in Surveillance
The true paradigm shift occurred with the integration of artificial intelligence and machine learning into surveillance systems. Modern AI-powered alert reporting systems leverage advanced algorithms, including deep learning and neural networks, to analyze vast amounts of data in real-time. These systems can identify complex patterns, predict potential security threats, and even learn from past incidents to improve future performance. AI agents in surveillance can now perform tasks that were once the exclusive domain of human experts, such as facial recognition, behavior analysis, and anomaly detection, with a level of accuracy and consistency that surpasses human capabilities.
Key Advancements in AI-Powered Alert Reporting
- Multi-sensor fusion: Integration of data from various sensors (video, audio, thermal) for comprehensive threat detection
- Contextual awareness: Understanding environmental factors and situational context to reduce false positives
- Predictive analytics: Anticipating potential security breaches based on historical data and current trends
- Natural language processing: Generating human-readable reports and alerts from complex data analysis
The transition from human-centric to AI-driven alert reporting has not only enhanced the efficiency and accuracy of surveillance systems but has also expanded the scope of what can be monitored and analyzed. As AI technologies continue to advance, the future of alert reporting in surveillance promises even greater capabilities, potentially revolutionizing security paradigms across various industries and applications.
Understanding AI Agent Alert Reporters: Core Technologies and Capabilities
AI agent alert reporters in the surveillance industry leverage a sophisticated blend of advanced technologies to enhance security monitoring and incident detection. At their core, these systems employ machine learning algorithms to analyze vast amounts of data in real-time, continuously improving their ability to identify anomalies and potential threats. Computer vision plays a crucial role, enabling AI agents to process and interpret visual data from surveillance cameras with remarkable accuracy. These systems can detect objects, recognize faces, and track movement patterns, often outperforming human operators in consistency and endurance. Natural language processing (NLP) capabilities allow AI agents to understand and generate human-like text, facilitating seamless communication of alerts and reports to security personnel.
Key Components of AI Agent Alert Reporters
The integration of these technologies creates a powerful surveillance tool:
- Deep learning neural networks for complex pattern recognition
- Convolutional neural networks (CNNs) for image analysis and object detection
- Recurrent neural networks (RNNs) for analyzing temporal sequences in video feeds
- Transformer models for advanced NLP capabilities in alert generation
Performance Metrics
Metric | AI Agent Performance | Human Operator Performance |
---|---|---|
Alert Accuracy | 98.7% | 89.2% |
Response Time | 0.3 seconds | 2.5 seconds |
Continuous Monitoring | 24/7 | 8-12 hours |
These AI-powered systems excel in
real-time threat assessment
, analyzing multiple data streams simultaneously to identify potential security breaches. By leveraging edge computing
and distributed processing architectures, AI agent alert reporters can operate with minimal latency, ensuring rapid response to emerging situations. The integration of predictive analytics allows these systems to anticipate potential security risks, enabling proactive measures rather than reactive responses.
24/7 Vigilance: How AI Agents Outperform Human Alert Reporters
AI agents have revolutionized alert reporting in the surveillance industry, demonstrating significant advantages over human counterparts in continuous monitoring, accuracy, and scalability. Unlike human operators who are subject to fatigue, distractions, and shift limitations, AI agents provide uninterrupted 24/7 vigilance, processing vast amounts of data from multiple sources simultaneously. This constant surveillance capability results in a 99.7% reduction in missed alerts compared to human-only systems. AI agents excel in pattern recognition, analyzing subtle changes in video feeds, sensor data, and behavioral anomalies that might escape human notice. Their ability to cross-reference historical data with real-time inputs enables predictive alert generation, often flagging potential security breaches before they fully materialize. In terms of scalability, a single AI agent can monitor up to 500 camera feeds concurrently, a task that would require a team of 25 human operators to achieve comparable coverage. This scalability translates to a 70% reduction in operational costs for large-scale surveillance operations. Moreover, AI agents demonstrate remarkable accuracy, with false positive rates as low as 0.01%, compared to the industry average of 3% for human alert reporters. This precision significantly reduces alert fatigue and allows security personnel to focus on genuine threats. The integration of machine learning algorithms enables AI agents to continuously improve their performance, adapting to new threat patterns and environmental changes without the need for manual retraining.
Comparative Performance Metrics
Metric | AI Agents | Human Reporters |
---|---|---|
Continuous Monitoring | 24/7 | 8-12 hour shifts |
Alert Response Time | < 1 second | 15-30 seconds |
False Positive Rate | 0.01% | 3% |
Scalability (Cameras/Agent) | 500 | 20 |
Real-time Threat Detection: AI Agents as Your Tireless Sentinels
AI agents have revolutionized real-time threat detection in the surveillance industry, serving as tireless digital sentinels that significantly enhance security operations. These intelligent systems leverage advanced computer vision algorithms and machine learning models to analyze video feeds continuously, identifying potential threats with remarkable accuracy. Unlike human operators who may experience fatigue or distractions, AI agents maintain unwavering vigilance 24/7, processing vast amounts of visual data at speeds unattainable by human counterparts. In fact, studies have shown that AI-powered surveillance systems can reduce false alarms by up to 95% while increasing threat detection rates by 80%.
Enhanced Threat Recognition Capabilities
Modern AI agents employ sophisticated convolutional neural networks (CNNs) and object detection frameworks like YOLO (You Only Look Once) to recognize and classify potential threats in milliseconds. These systems can identify suspicious behaviors, unauthorized access attempts, and even subtle anomalies that might escape human notice. For instance, an AI agent can simultaneously track multiple individuals in a crowded area, detect concealed weapons, and flag unusual movement patterns, all in real-time.
Rapid Alert and Response Mechanisms
The true power of AI agents in threat detection lies in their ability to trigger immediate alerts and automate response protocols. When a potential threat is identified, these systems can:
- Instantly notify security personnel with precise location data
- Activate additional surveillance measures in the affected area
- Initiate lockdown procedures or access control measures
- Provide real-time guidance to on-site staff for threat mitigation
This rapid response capability has been shown to reduce average incident response times by up to 60%, critically important in high-stakes security environments.
Adaptive Learning and Continuous Improvement
Perhaps most impressively, AI agents in surveillance systems demonstrate remarkable adaptability. Through reinforcement learning and federated learning techniques, these systems continuously refine their threat detection models based on new data and feedback from security professionals. This adaptive capacity allows AI agents to stay ahead of evolving threat landscapes, recognizing new patterns of suspicious activity and adjusting their detection parameters accordingly. In one case study, an AI-powered surveillance system improved its threat detection accuracy by 22% over six months of operation in a high-security facility, showcasing the long-term value of these intelligent sentinels.
Beyond Visual Surveillance: AI Agents and Multi-sensor Integration
AI agents are revolutionizing the surveillance industry by integrating data from diverse sensor types, creating a comprehensive alert reporting system that extends far beyond traditional visual monitoring. These intelligent systems now seamlessly combine inputs from audio sensors, thermal imaging devices, and Internet of Things (IoT) endpoints to provide a multi-dimensional view of security environments. For instance, AI agents can correlate thermal anomalies detected by infrared cameras with unusual sound patterns captured by audio sensors, triggering alerts for potential security breaches that might go unnoticed by visual surveillance alone. In industrial settings, these agents integrate data from vibration sensors, air quality monitors, and machine telemetry to predict equipment failures and environmental hazards before they escalate into critical incidents.
Advanced Sensor Fusion Techniques
Modern AI agents employ sophisticated sensor fusion algorithms to synthesize data streams in real-time. Kalman filtering and Bayesian inference techniques allow these systems to reconcile conflicting sensor inputs and reduce false positives by up to 87%. This multi-modal approach enables AI agents to distinguish between benign anomalies and genuine threats with unprecedented accuracy, achieving a 99.3% true positive rate in controlled tests.
Contextual Understanding and Adaptive Alerting
By leveraging natural language processing (NLP) and machine learning models, AI agents can now interpret contextual cues from integrated sensors. For example, an agent might combine audio sentiment analysis from conversations near a secure area with unusual thermal signatures to assess the likelihood of an imminent security breach. This contextual understanding allows for adaptive alert thresholds, reducing alarm fatigue by 62% while maintaining high security standards.
IoT Integration for Comprehensive Monitoring
The integration of IoT devices expands the reach of AI agents beyond traditional surveillance boundaries. Smart locks, environmental sensors, and even wearable devices worn by security personnel can feed data into the AI system. This holistic approach enables predictive alerting, where AI agents can forecast potential security risks based on patterns observed across multiple IoT endpoints. In smart city deployments, this integration has led to a 43% improvement in emergency response times and a 31% reduction in crime rates in pilot programs.
Sensor Type | Data Contribution | Integration Impact |
---|---|---|
Audio | Sound pattern analysis, voice recognition | 35% increase in threat detection accuracy |
Thermal | Heat signature mapping, anomaly detection | 58% improvement in perimeter security |
IoT Devices | Environmental data, access logs, telemetry | 72% enhancement in situational awareness |
As AI agents continue to evolve, their ability to integrate and interpret multi-sensor data will redefine the boundaries of surveillance technology, offering unprecedented levels of security intelligence and operational insight across diverse industries and applications.
Predictive Analytics in AI Agent Alert Reporting: Stopping Threats Before They Happen
AI agents equipped with advanced predictive analytics capabilities are revolutionizing alert reporting in the surveillance industry, enabling proactive threat prevention rather than reactive incident response. These intelligent systems leverage machine learning algorithms and historical data to identify patterns and anomalies that human operators might miss. By analyzing vast amounts of real-time data from multiple sources—including video feeds, access control systems, and environmental sensors—AI agents can detect subtle indicators of potential security risks with remarkable accuracy. For instance, an AI-powered surveillance system can recognize unusual behavior patterns, such as loitering in restricted areas or suspicious vehicle movements, and generate alerts before an incident occurs. This predictive approach has shown to reduce false alarms by up to 90% while increasing the detection of genuine threats by 75%. Moreover, AI agents can continuously learn and adapt their predictive models, improving their accuracy over time. They can correlate seemingly unrelated events across different timeframes and locations, uncovering complex threat scenarios that traditional systems would overlook. Cutting-edge AI agents now incorporate natural language processing to analyze communication patterns and sentiment in monitored areas, providing an additional layer of predictive intelligence. By harnessing the power of predictive analytics, AI agents are not just reporting alerts—they’re anticipating and preventing security breaches, significantly enhancing the effectiveness of surveillance operations.
Customizing AI Agent Alert Reporters: Tailoring to Your Business Needs
Customizing AI agent alert reporters is crucial for businesses in the surveillance industry to optimize their security operations. By tailoring these systems to match specific requirements and operational environments, organizations can significantly enhance their threat detection capabilities and response times. Advanced customization options allow for fine-tuning of alert thresholds, prioritization of security events, and integration with existing security information and event management (SIEM) systems. For instance, retailers may configure AI agents to focus on shoplifting patterns, while financial institutions might prioritize anomalies in transaction data. Intelligent filtering mechanisms can be implemented to reduce false positives by up to 87%, ensuring that security teams focus on genuine threats. Moreover, customized alert reporters can leverage machine learning algorithms to adapt to evolving threat landscapes, continuously improving their accuracy over time. By incorporating geospatial analytics and behavioral profiling, these systems can provide context-aware alerts that are 3.5 times more likely to result in successful threat mitigation. Organizations implementing customized AI agent alert reporters have reported a 42% reduction in incident response times and a 68% increase in the detection of sophisticated cyber threats.
Key Customization Features:
- Dynamic threshold adjustment based on historical data
- Multi-factor authentication integration for high-priority alerts
- Customizable dashboard views for different security roles
- API-driven integration with third-party security tools
- Natural language processing for more intuitive alert descriptions
Implementation Considerations:
When customizing AI agent alert reporters, businesses must consider their specific risk profile, regulatory compliance requirements, and operational workflows. A phased approach to implementation, starting with critical assets and gradually expanding coverage, can ensure smooth adoption and minimize disruptions to existing security processes. Regular audits and performance reviews are essential to maintain the effectiveness of customized alert systems in the face of evolving threats and business needs.
The Human-AI Collaboration: Redefining Roles in Alert Reporting
The integration of AI agents in alert reporting systems is revolutionizing the surveillance industry by creating a symbiotic relationship between human security personnel and artificial intelligence. This collaboration significantly enhances the efficiency and effectiveness of alert reporting processes. AI agents, equipped with advanced computer vision and machine learning algorithms, can continuously monitor multiple video feeds simultaneously, detecting anomalies and potential security threats with unprecedented accuracy. These systems can process vast amounts of data in real-time, identifying patterns and behaviors that might escape human observation. For instance, AI-powered systems have demonstrated a 98% accuracy rate in detecting suspicious activities, compared to the 85% rate of human observers alone. However, the human element remains crucial in this ecosystem. Security personnel now focus on higher-level decision-making, threat assessment, and response coordination. This shift allows human operators to leverage their intuition, contextual understanding, and complex problem-solving skills more effectively. The AI-human collaboration has led to a 40% reduction in false alarms and a 30% increase in response times to genuine security incidents. Moreover, this partnership has enabled a more scalable and cost-effective security infrastructure, with some organizations reporting operational cost savings of up to 25% after implementing AI-augmented alert reporting systems. As AI technology continues to evolve, the roles of human security personnel are being redefined, emphasizing strategic oversight, ethical decision-making, and interpersonal skills that AI cannot replicate.
Overcoming Implementation Challenges: Integrating AI Agent Alert Reporters into Existing Systems
Integrating AI agent alert reporters into existing surveillance infrastructure presents unique challenges that require strategic planning and technical finesse. One of the primary hurdles is system compatibility, as legacy surveillance platforms often utilize proprietary protocols that may not readily interface with modern AI systems. To address this, organizations should consider implementing middleware solutions that act as translators between the AI agent and existing infrastructure. These middleware layers can facilitate seamless data exchange, enabling real-time alert generation without disrupting established workflows. Another critical challenge is data normalization, as AI agents require standardized input to function effectively. Implementing data preprocessing pipelines that cleanse and format surveillance data before feeding it into the AI system can significantly enhance alert accuracy and reduce false positives by up to 78%. Additionally, organizations must navigate bandwidth constraints, particularly in large-scale deployments where multiple high-resolution video streams are analyzed simultaneously. Employing edge computing architectures can alleviate network strain by processing data locally before transmitting only relevant alerts to central systems, reducing bandwidth requirements by up to 60%. To ensure smooth integration, it’s crucial to develop a phased rollout strategy that allows for iterative testing and refinement. This approach typically involves a pilot phase in a controlled environment, followed by gradual expansion to broader surveillance networks, with each phase incorporating lessons learned to optimize performance. By addressing these challenges systematically, organizations can successfully integrate AI agent alert reporters, enhancing their surveillance capabilities while minimizing disruption to existing operations.
Privacy and Ethical Considerations in AI-Powered Alert Reporting
Implementing AI agents for alert reporting in surveillance systems necessitates a meticulous approach to privacy protection and ethical considerations. Data minimization is paramount, with systems designed to collect only essential information for threat detection. Robust encryption protocols and access controls must be implemented to safeguard sensitive data from unauthorized access. AI models require careful bias mitigation strategies, including diverse training datasets and regular audits to prevent discriminatory outcomes.
Transparency in AI decision-making processes is crucial
, with explainable AI techniques employed to provide clear rationales for alerts. Organizations must establish ethical review boards to oversee AI implementations and ensure compliance with regulations like GDPR and CCPA. Privacy-preserving machine learning techniques
, such as federated learning and differential privacy, should be integrated to enhance data protection. Regular privacy impact assessments are essential to identify and address potential risks proactively. Implementing consent management systems allows individuals to control their data usage within surveillance contexts. AI alert systems must incorporate fail-safe mechanisms to prevent over-reliance on automated decision-making, maintaining human oversight in critical situations. Ethical AI frameworks, such as the IEEE Ethically Aligned Design, should guide the development and deployment of AI-powered alert reporting systems to ensure responsible innovation in surveillance technology.
The Future of Surveillance: AI Agents and the Internet of Things (IoT)
The convergence of AI agents and IoT devices is poised to revolutionize surveillance systems, ushering in an era of unprecedented situational awareness and proactive threat detection. Advanced AI algorithms will process real-time data streams from a vast network of interconnected sensors, cameras, and smart devices, enabling contextual understanding of complex environments. These AI-powered systems will not only detect anomalies but also predict potential security breaches by analyzing patterns across multiple data points. For instance, an AI agent might correlate unusual access card activity with atypical network traffic and suspicious thermal imaging data to flag a potential insider threat before it materializes.
Enhanced Analytics and Decision Support
Future AI agents will leverage edge computing to process data locally, reducing latency and enhancing real-time response capabilities. This distributed intelligence approach will enable more sophisticated alert reporting, including:
- Predictive maintenance of surveillance equipment
- Automated threat level assessment and prioritization
- Dynamic adjustment of security protocols based on evolving risk landscapes
Integration with Smart City Infrastructure
As smart cities evolve, AI agents will integrate surveillance systems with broader urban infrastructure, creating a holistic security ecosystem. Traffic management systems, public transportation networks, and emergency services will be seamlessly coordinated through AI-driven alert reporting mechanisms. This integration will enable rapid, coordinated responses to security incidents, natural disasters, and public safety threats.
AI Agent Capability | Impact on Surveillance |
---|---|
Multi-modal data fusion | 360-degree situational awareness |
Federated learning | Privacy-preserving intelligence sharing |
Explainable AI | Transparent decision-making for regulatory compliance |
The future of surveillance will be characterized by intelligent, autonomous systems that not only report alerts but actively contribute to strategic security planning and incident prevention. As these technologies mature, the role of human operators will evolve towards high-level oversight and decision-making, supported by AI agents that provide actionable insights derived from the vast IoT sensor network.
FAQ: Everything You Need to Know About AI Agent Alert Reporters
What are AI agent alert reporters in surveillance?
AI agent alert reporters are advanced software systems that utilize artificial intelligence to monitor surveillance feeds, analyze data in real-time, and generate automated alerts for security personnel. These intelligent agents employ computer vision, machine learning, and natural language processing to detect anomalies, identify potential threats, and report incidents with unprecedented accuracy and speed. Unlike traditional rule-based systems, AI agent alert reporters can adapt to evolving scenarios, learn from past events, and significantly reduce false positives by up to 92%. They operate 24/7, processing vast amounts of data from multiple sources including cameras, sensors, and access control systems, to provide a comprehensive security overview.
How do AI agent alert reporters improve surveillance efficiency?
AI agent alert reporters dramatically enhance surveillance efficiency through:
- Real-time threat detection: Identifying potential security breaches within milliseconds
- Automated reporting: Generating detailed incident reports without human intervention
- Predictive analytics: Forecasting potential security risks based on historical data and patterns
- Multi-feed correlation: Analyzing data from various sources to provide context-rich alerts
- Scalability: Handling large-scale surveillance operations without compromising performance
These capabilities enable security teams to respond proactively, allocate resources more effectively, and maintain a higher level of situational awareness across complex environments.
What types of alerts can AI agent reporters generate?
AI agent alert reporters can generate a wide range of sophisticated alerts, including:
- Unauthorized access attempts
- Suspicious behavior patterns
- Object detection (e.g., weapons, abandoned packages)
- Perimeter breaches
- Crowd density and flow analysis
- Vehicle tracking and license plate recognition
- Facial recognition and person of interest identification
- Environmental anomalies (e.g., fire, smoke, flooding)
These alerts are customizable and can be tailored to specific industry requirements, such as retail loss prevention, critical infrastructure protection, or public safety monitoring.
How accurate are AI agent alert reporters compared to traditional systems?
AI agent alert reporters demonstrate significantly higher accuracy compared to traditional rule-based systems:
Metric | AI Agent Alert Reporters | Traditional Systems |
---|---|---|
False Positive Rate | 2-5% | 15-30% |
True Positive Rate | 95-98% | 70-85% |
Response Time | <1 second | 10-30 seconds |
This enhanced accuracy translates to more reliable threat detection, fewer missed incidents, and reduced operational costs associated with investigating false alarms.
What are the integration challenges for AI agent alert reporters?
Integrating AI agent alert reporters into existing surveillance infrastructure presents several challenges:
- Legacy system compatibility: Ensuring seamless integration with older hardware and software
- Data privacy compliance: Adhering to regulations such as GDPR, CCPA, and industry-specific standards
- Scalability concerns: Managing increased computational demands as the system grows
- Training and calibration: Fine-tuning AI models for specific environments and use cases
- Cybersecurity risks: Protecting AI systems from potential hacking or manipulation attempts
Overcoming these challenges requires a comprehensive implementation strategy, involving stakeholders from IT, security, and compliance departments to ensure smooth integration and optimal performance.